Development and validation of a risk prediction model for post-traumatic stress disorder symptoms in patients with acute myocardial infarction in China
Introduction
Post-traumatic stress disorder (PTSD) refers to the clinical manifestations of traumatic experiences, increased alertness, persistent avoidance, or numbness after an individual experiences a major physical or psychological stimulus, which is a potentially traumatic event. The effects of PTSD include psychological disturbance and reduced treatment compliance, which in turn affects the patient’s disease prognosis (1). Studies have shown that the incidence of PTSD symptoms among acute myocardial infarction (AMI) patients within 12 months after discharge is 3.00–19.00% (2), and the incidence of PTSD symptoms in Chinese AMI patients from the acute phase to 3 months after discharge is 20.40–33.10% (3). A meta-analysis (4) found that the incidence of PTSD symptoms due to heart disease ranged from 0% to 38.00% and was highly dependent on the tools used for assessment. The condition of PTSD symptoms affects patients’ physical and mental health, and reduces their quality of life. Patients with PTSD symptoms have reported an approximately 20.0% lower health-related quality of life (HRQL) at follow-up. Individuals with a combination of PTSD and depression or anxiety are potentially faced with a poor HRQL as a longer-term outcome of their heart disease (5). In addition, PTSD symptoms increase the recurrence rate of AMI, hospitalization rate, and mortality rate of affected patients, incurring a major public health burden (6). Therefore, screening and early intervention of PTSD symptoms risk in AMI are of great importance. A international study has found that there is a potential causal relationship between sleep disorders and mental illness. This suggests that abnormal sleep patterns may be a sign of mental illness (7). A previous study has shown that the symptoms of PTSD in patients with AMI may be related to the patient’s age, gender, and personality characteristics (8). Hippocampal damage, serum cortisol, C-reactive protein are predictors of PTSD symptoms (9-11). However, there are currently no guidelines or risk prediction models to guide clinical personnel in reducing or preventing PTSD symptoms in patients with AMI. Based on Chinese and international literature and research group discussions, according to the clinical and biochemical index of accessible principle, this study explored the risk factors of PTSD symptoms in AMI and established its risk prediction model, in order to provide a convenient tool and evaluation method for the prevention and early intervention of PTSD symptoms in AMI. We present the following article in accordance with the TRIPOD reporting checklist (available at https://apm.amegroups.com/article/view/10.21037/apm-22-881/rc).
Methods
Study population and data collection
This is a single-center, observational, cross-sectional study. A total of 359 eligible patients from a hospital in Shandong Province from April 2021 to June 2022 were enrolled as the research cohort. Among them, 287 AMI patients from April 2021 to March 2022 were enrolled as the modeling group. According to their psychological status 30 days after discharge, the patients were divided into a PTSD symptoms group (n=92) and a non-PTSD symptoms group (n=195). A total of 72 patients with AMI from April to June 2022 were enrolled as the validation group. The participant inclusion criteria were as follows: age 18–80 years; AMI was diagnosed clinically for the first time and followed up; and a state of consciousness and lucidity. The exclusion criteria were as follows: severe cardiac, cerebral, and renal complications; patients who had experienced other major catastrophic events (earthquake, serious illness, family changes) in the last six months; respiratory system infection, urinary system infection, and other serious infectious diseases; and inability or unwillingness to cooperate with the scale tester. This study was approved by the Ethics Committee of Weifang Medical University Affiliated Hospital (No. wffy-2022-ky-147) and informed consent was taken from all the patients. All included patients voluntarily agreed to participate in this study. The study was conducted in accordance with the Declaration of Helsinki (as revised in 2013). The Demographic questionnaire, Athens insomnia scale and Disease fear progression Scale were distributed before discharge, and the civilian version was distributed 30 days after discharge to collect data.
Variables and measures
Demographic data
By consulting Chinese and international literature, consulting 5 clinical nursing experts in cardiovascular medicine, and incorporating hospital feasibility analysis, the design mainly included the following contents. The demographic information: age, gender, education level, marital status, occupation, drinking history, smoking history, body mass index (BMI, kg/m2), and family monthly income. Disease factors: history of hypertension, history of hyperlipidemia, history of diabetes, history of out-of-hospital cardiac arrest, grading of cardiac function, and pain intensity. Treatment factors: length of stay, number of stents, and postoperative clinical events. Laboratory test indicators: low density lipoprotein, high density lipoprotein, triglyceride, fasting blood glucose, total cholesterol, creatine kinase isoenzyme, and left ventricular ejection fraction (LVEF) value.
Athens insomnia scale
Soldatos et al. designed the Athens insomnia scale in 2000 for self-assessment of sleep disorders (12). This scale is an internationally recognized sleep quality self-test scale. There are 8 items in total, and each item is evaluated by a Likert 4-level numerical scoring method. The cumulative score of each item is the total score, with a total score less than 4 being no sleep disorder, 4–6 being suspicious of a sleep disorder, and above 6 being a sleep disorder. Cronbach’s α coefficient is 0.887–0.89 and the retest correlation coefficient is 0.88–0.89.
Disease fear progression scale
The simplified version of the Fear of Disease Progression Questionnaire (FoP-Q-SF) had 12 items. A Likert 5-level scoring method was used to calculate the total score of the 12 items. The total score ranged from 12 to 60 points. The higher the score, the more serious the patient’s fear. A score ≥34 reached the clinically defined level, indicating a psychological dysfunction of fear of disease progression. In 2015, Chinese researchers created a translated and culturally adjusted version of the FoP-Q-SF. The research fields of this scale have been extended to AMI, diabetes, and other disease factors, all of which have been reported to have good reliability and validity (13). The Chinese version of the scale includes 2 dimensions: physiological health, and social and family function (14). The physical health dimension mainly refers to patients’ worries and fears about their own health conditions. The social family function dimension mainly refers to patients’ worries and fears about their social and family participation in the future.
Civilian version of PTSD list
The civilian version of the PTSD list (PCL-C) is mainly used to report the PTSD symptoms of cases in the past 1 month. On a scale of 17 to 85, the higher the score, the higher the risk of PTSD. A single item score of ≥3 is considered the critical value of positive symptoms. Those who satisfied at least 1 item in the reexperience symptom group were positive, as were those who satisfied at least 2 items in the avoidance symptom group and hypervigilance symptom group. The Chinese version of this scale suggests 38 points as the demarcation standard (15). Cronbach’s α coefficient is 0.88–0.94, and the retest correlation coefficient is 0.708 (16).
Statistical analysis
The software SPSS 26.0 (IBM Corp, Armonk, NY, USA) was used for statistical analysis. The count data were described by case/percentage and χ2 test was performed. Normal measurement data were described by mean ± standard deviation, and and t-test is adopted. Non-normal distribution measurement data were described by median and quartile, and inter-group row rank sum test. P<0.05 was considered statistically significant, and the factor with Statistical significance in univariate analysis was entered into Logistic regression analysis, and the significant influencing factor was finally obtained to construct the prediction model. The R Studio software (The R Foundation for Statistical Computing, Vienna, Austria) was used to build a risk prediction model. The Hosmer-Lemeshow test and a receiver operating characteristic (ROC) curve were used to evaluate the fitting and diagnostic efficacy of the prediction model for PTSD symptoms.
Results
In the modeling group, PTSD symptoms occurred in 92 of 287 patients (32.06%). The total score and average score of each dimension of the PCL-C of PTSD are shown in Table 1. The total score and scores of other dimensions in the PTSD symptoms group were significantly higher than those in the non-PTSD symptoms group (P<0.001).
Table 1
Characteristic | PTSD group (n=92), mean ± SD | Non-PTSD group (n=195), mean ± SD | t-value | P value |
---|---|---|---|---|
Recurrence | 13.85±1.24 | 10.70±1.08 | 21.866 | <0.001 |
Avoidance and numbness | 19.00±2.19 | 12.20±0.75 | 23.639 | <0.001 |
Hypervigilance | 13.74±1.82 | 9.10±0.70 | 28.879 | <0.001 |
Total score | 46.59±4.96 | 32.00±2.39 | 26.804 | <0.001 |
PTSD, post-traumatic stress disorder.
Univariate analysis of PTSD symptoms in AMI. In the modeling group, in the analysis of PTSD symptoms in patients with AMI and PTSD symptoms in patients with AMI-related factors including gender, marital status, smoking history, family income, history of diabetes mellitus, LVEF value, creatine kinase isoenzyme, insomnia score, and the fear of disease progression, the difference was statistically significant (P<0.05), as shown in Table 2.
Table 2
Characteristic | PTSD group (n=92) | Non-PTSD group (n=195) | P value |
---|---|---|---|
Gender, n (%) | |||
Men | 24 (26.1) | 147 (75.4) | <0.001 |
Women | 68 (73.9) | 48 (24.6) | |
Marital status, n (%) | |||
Married | 41 (44.6) | 123 (63.1) | 0.003 |
Unmarried/divorced/widow | 51 (55.4) | 72 (36.9) | |
Smoking history, n (%) | |||
Yes | 67 (72.8) | 118 (60.5) | 0.042 |
No | 25 (27.2) | 77 (39.5) | |
Monthly household income, n (%) | |||
<$300 | 18 (19.6) | 16 (8.2) | |
$300–750 | 53 (57.6) | 123 (63.1) | 0.019 |
>$750 | 21 (22.8) | 56 (28.7) | |
History of diabetes, n (%) | |||
Yes | 70 (76.1) | 106 (54.4) | <0.001 |
No | 22 (23.9) | 89 (45.6) | |
LVEF, n (%) | |||
<50% | 69 (75.0) | 106 (54.4) | <0.001 |
≥50% | 23 (25.0) | 89 (45.6) | |
Creatine kinase isoenzyme, U/L, M (P25, P75) | 62.0 (52.9, 67.3) | 37.5 (28.9, 45.5) | <0.001 |
Insomnia score, M (P25, P75) | 5.0 (4.0, 7.0) | 4.0 (3.0, 5.0) | <0.001 |
Fear of disease progression, points, M (P25, P75) | 34.5 (23.0, 40.0) | 21.0 (20.0, 23.0) | <0.001 |
PTSD, post-traumatic stress disorder; LVEF, left ventricular ejection fraction.
Multivariate analysis of PTSD symptoms in AMI. A total of 9 variables with statistical significance in single factor analysis were included. The independent variables were assigned as follows: gender: 0= male, 1= female; marriage: 1= spouse 2= divorce/death of spouse; monthly household income: 1= less than $300, 2= $300–750 , 3= more than $750; smoking history: 0= no,1= yes; history of diabetes: 0= no, 1= yes; and LVEF: 0=≥50%, 1=<50%. Continuous variables, including creatine kinase isoenzymes, insomnia scores, and fear of disease progression, were entered as original values. Binary logistic regression analysis was used to construct a logistic regression model. The results showed that gender, diabetes history, creatine kinase isoenzyme, insomnia score and fear of disease progression score were independent risk factors for PTSD in patients with AMI. The prediction model was finally established as P=ex/(1+ex), where E is the natural logarithm, X=−10.500+ (1.201 × gender) + (0.829 × diabetes history) + (0.045 × creatine kinase isoenzyme) + (0.715 × insomnia score) + (0.119 × fear of disease progression), as shown in Table 3.
Table 3
Variable | Regression coefficient | SE | Wald χ2 | OR (95% CI) | P value |
---|---|---|---|---|---|
Constant | −10.500 | 1.226 | 73.352 | – | <0.001 |
Gender | 1.201 | 0.392 | 9.441 | 3.325 (1.543–7.163) | 0.002 |
History of diabetes | 0.829 | 0.412 | 4.052 | 2.292 (1.022–5.138) | 0.044 |
Creatine kinase isoenzyme | 0.045 | 0.012 | 14.728 | 1.046 (1.022–1.071) | <0.001 |
Insomnia score | 0.715 | 0.164 | 19.045 | 2.045 (1.483–2.820) | <0.001 |
Fear of disease progression | 0.119 | 0.023 | 26.213 | 1.126 (1.076–1.179) | <0.001 |
PTSD, post-traumatic stress disorder; OR, odds ratio; CI, confidence interval.
The Hosmer–Lemeshow test model fitting effect showed P=0.785. The sensitivity and specificity of the model were tested by the area under the curve (AUC), as shown in Figure 1. The optimal critical value of the model was determined by the maximum value of the Youden index. The AUC of this model was 0.910 (95% CI: 86.90% to 95.10%), the maximum value of the Youden index was 0.751, the optimal critical value was 0.587, the sensitivity was 0.870, and the specificity was 0.881 (details in Figure 1A). A total of 72 patients with AMI who met the inclusion criteria were selected as the research cohort, and the model was used for clinical validation. In the validation group, there were 32 cases of PTSD symptoms, with the actual incidence of 44.44%. The risk model predicted 17 cases of PTSD symptoms, 15 cases of misjudgment, sensitivity 70.83%; The risk model predicted that there were non-PTSD symptoms in 33 cases, but 7 cases were misjudged, with specificity of 68.75%. The total accuracy of the model is 69.44% (details in Figure 1B).
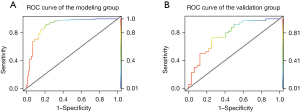
Discussion
In this study, the incidence of PTSD symptoms in patients with AMI was 32.06%. International study (2) have shown that the incidence of PTSD symptoms in AMI is between 3% and 19%. A Chinese study has shown that the incidence of PTSD symptoms in patients with AMI is 20.4–33.1% (3). The different incidence of PTSD symptoms in AMI patients in China and internationally may be related to the differences in the study target population, PTSD symptoms assessment tools, and follow-up time.
The results of this study showed that women had a higher incidence of PTSD symptoms than men. When faced with the stress of disease, female patients are more likely to experience PTSD symptoms than male patients, which is similar to the results of Fonkoue and Kobayashi et al. (17,18). An international study shows that there is a significant correlation between the size of the amygdala and PTSD symptoms when individuals are stressed (19). Compared with that of men, the amygdala of women has a more persistent and sensitive response to negative stimuli (20) and is more likely to produce intense emotions such as fear. Compared to men, women’s amygdalae are more responsive to ongoing negative stimuli. This indicates that females are more likely to show higher emotional disorders when stimulated by the same negative things as males. Women are twice as likely as men to develop symptoms of PTSD, and the reason may be due to interactions between sex hormones and stress peptides, the study said (21). In addition, due to the difference in sex hormones (22), women are more sensitive to painful stimuli and are more likely to perceive physical and mental harm brought by diseases. In clinical work, our medical staff should focus on the psychological problems of female AIM patients, actively communicate with patients who do not express themselves readily, and reduce their negative emotions, so as to reduce the risk of PTSD symptoms.
The results of this study showed that the risk of PTSD symptoms in patients with a diabetes history was 2.292 times higher than that in those with no history of diabetes. The symptoms of PTSD were associated with elevated diabetes distress and more frequent exercise and self-blood glucose testing, which is similar to the results of Arigo et al. (23). There are 2 predominant stress factors of PTSD symptoms: diagnosis of trauma (acute stressors) and trauma-related medical treatment (chronic stressors) (24). In patients with type 2 diabetes, diabetes is a chronic stressor wherein long-term high blood sugar and insulin resistance can accelerate glucolipid metabolic disorders, cause patients to experience systemic tissue organ dysfunction and failure, and even cause a variety of chronic complications (25), all of which increase the economic and psychological burden of patients prone to PTSD symptoms. In addition, studies have shown that the symptoms of PTSD interact with the metabolic severity of diabetes (26). Diabetics are more prone to PTSD symptoms and diabetes aggravates the symptoms of PTSD symptoms (27), mainly because a stable glucose level is essential for brain metabolism and neuronal activity. Chronic high glucose levels and rapid glucose fluctuations in diabetics can lead to neuronal damage, undesirable mental health outcomes, and other neurological and cognitive deficits that can easily manifest as symptoms of PTSD. Therefore, clinicians should continuously detect and control blood sugar, especially that of diabetics, to reduce their physical and psychological injury, thus reducing the occurrence of PTSD symptoms and improving their quality of life.
Creatine kinase isoenzyme is an important factor in the occurrence of PTSD symptoms in patients with AMI. The results of this study showed that the total value of creatine kinase isoenzyme in the PTSD symptoms group was significantly higher than that in non-PTSD symptoms group. The main reason is that creatine kinase isoenzyme is a typical biomarker of AMI, and its level can reflect the degree of myocardial damage, representing the severity of the patient’s condition to a certain extent (28). In the follow-up treatment, when there is a conflict between physical health and executive ability, psychological pressure is easily generated. Therefore, it is necessary to strengthen the monitoring and treatment of creatine kinase isoenzyme in clinical practice to prevent the occurrence of PTSD symptoms and improve the physical and mental health of patients.
The results of this study showed that sleep disturbance was an independent risk factor for PTSD symptoms in patients with AMI, and patients with a higher insomnia score had a higher risk of developing PTSD symptoms. Sleep is important to normal cognitive function (29). The occurrence of sleep disorders and circadian rhythm disorders in patients will fundamentally affect the neuroendocrine, immune, and autonomic systems, resulting in the breakdown of biological behavioral adaptation mechanisms and increased stress sensitivity and vulnerability. Therefore, sleep may play a causal role in the development of PTSD symptoms (30). In addition, sleep disorders often appear together with PTSD symptoms, and patients with poor sleep quality are prone to PTSD symptoms (31). In clinical work, it is necessary to pay attention to the sleep assessment of patients, any sleep-related problems that they mention, provide targeted guidance, take measures to ameliorate the adverse mood and sleep disorders of patients, and strengthen their sleep management.
The results of this study show that patients with high fear of disease progression are prone to develop PTSD symptoms, and the 2 are positively correlated, which is consistent with the research results of Chinese researchers (32,33). An international study of 763 patients with heart disease found that patients with higher fear of disease had significantly more severe PTSD symptoms at 1-month follow-up (34). The reason may be that AMI is a potential life-threatening event which can cause subjective feelings of fear and threat to those affected, which are manifested as strong fear and helpless post-traumatic perception, which increases the risk of PTSD symptoms in patients with heart disease (35). In addition, fear of disease progression is strongly correlated with social pressure, and a higher level of fear of disease progression can reflect future disease risk (36). Therefore, in future early intervention studies, medical staff should focus on the level of fear of recurrence in patients with AMI and intervene as soon as possible to effectively prevent the occurrence of PTSD symptoms.
In this study, the Hosmer–Lemeshow model was used to evaluate the goodness of fit, and AUC was used to evaluate the prediction model. When the AUC was less than 0.7, the prediction efficiency is low; when the AUC was between 0.7 and 0.9, the prediction efficiency was medium; an AUC >0.9 indicates high prediction efficiency (37). In this model, the AUC for internal verification was 0.910, and in clinical verification, the maximum value of Youden index was 0.751, the optimal critical value was 0.587, the sensitivity was 0.870, and the specificity was 0.881. In external clinical validation, the sensitivity and specificity were 70.83% and 68.75%, respectively. The total accuracy rate was 69.44%. Both internal and external validation indicated that the model was effective in predicting and identifying patients with PTSD symptoms. Therefore, this model can be applied to clinical practice.
Conclusions
In this study, female patients with AMI, diabetes, insomnia, high creatine kinase isoenzyme, and high fear of disease progression were found to be prone to PTSD symptoms. This does not indicate that male patients should be disregarded. Therefore, medical staff should carry out early assessment and screening for the influencing factors of PTSD symptoms, implement timely and effective intervention, and improve the disease prognosis of patients. The limitation of this study is that it was only conducted during a period of time without longitudinal investigation, suggesting that future researchers can explore the changes of patients’ PTSD symptoms status over a longer span of time.
Acknowledgments
Funding: None.
Footnote
Reporting Checklist: The authors have completed the TRIPOD reporting checklist. Available at https://apm.amegroups.com/article/view/10.21037/apm-22-881/rc
Data Sharing Statement: Available at https://apm.amegroups.com/article/view/10.21037/apm-22-881/dss
Conflicts of Interest: All authors have completed the ICMJE uniform disclosure form (available at https://apm.amegroups.com/article/view/10.21037/apm-22-881/coif). The authors have no conflicts of interest to declare.
Ethical Statement: The authors are accountable for all aspects of the work in ensuring that questions related to the accuracy or integrity of any part of the work are appropriately investigated and resolved. This study was approved by the Ethics Committee of Weifang Medical University Affiliated Hospital (No. wffy-2022-ky-147) and informed consent was taken from all the patients. The study was conducted in accordance with the Declaration of Helsinki (as revised in 2013).
Open Access Statement: This is an Open Access article distributed in accordance with the Creative Commons Attribution-NonCommercial-NoDerivs 4.0 International License (CC BY-NC-ND 4.0), which permits the non-commercial replication and distribution of the article with the strict proviso that no changes or edits are made and the original work is properly cited (including links to both the formal publication through the relevant DOI and the license). See: https://creativecommons.org/licenses/by-nc-nd/4.0/.
References
- Nachshol M, Lurie I, Benyamini Y, et al. Role of psychosocial factors in long-term adherence to secondary prevention measures after myocardial infarction: a longitudinal analysis. Ann Epidemiol 2020;52:35-41. [Crossref] [PubMed]
- Jacquet-Smailovic M, Tarquinio C, Alla F, et al. Posttraumatic Stress Disorder Following Myocardial Infarction: A Systematic Review. J Trauma Stress 2021;34:190-9. [Crossref] [PubMed]
- Cao X, Wu J, Gu Y, et al. Post-traumatic Stress Disorder and Risk Factors in Patients With Acute Myocardial Infarction After Emergency Percutaneous Coronary Intervention: A Longitudinal Study. Front Psychol 2021;12:694974. [Crossref] [PubMed]
- Vilchinsky N, Ginzburg K, Fait K, et al. Cardiac-disease-induced PTSD (CDI-PTSD): A systematic review. Clin Psychol Rev 2017;55:92-106. [Crossref] [PubMed]
- Allabadi H, Alkaiyat A, Zahdeh T, et al. Posttraumatic stress disorder predicts poor health-related quality of life in cardiac patients in Palestine. PLoS One 2021;16:e0255077. [Crossref] [PubMed]
- Lima BB, Hammadah M, Pearce BD, et al. Association of Posttraumatic Stress Disorder With Mental Stress-Induced Myocardial Ischemia in Adults After Myocardial Infarction. JAMA Netw Open 2020;3:e202734. [Crossref] [PubMed]
- Sun X, Liu B, Liu S, et al. Sleep disturbance and psychiatric disorders: a bidirectional Mendelian randomisation study. Epidemiol Psychiatr Sci 2022;31:e26. [Crossref] [PubMed]
- Kong Y, Tian X, Zhang Q, et al. Research progress of PTSD in patients with acute myocardial infarction. General Nursing 2022;20:2776-9.
- Tanriverdi B, Gregory DF, Olino TM, et al. Hippocampal Threat Reactivity Interacts with Physiological Arousal to Predict PTSD Symptoms. J Neurosci 2022;42:6593-604. [Crossref] [PubMed]
- Bielas H, Meister-Langraf RE, Schmid JP, et al. C-reactive protein as a predictor of posttraumatic stress induced by acute myocardial infarction. Gen Hosp Psychiatry 2018;53:125-30. [Crossref] [PubMed]
- Schaffter N, Ledermann K, Pazhenkottil AP, et al. Serum cortisol as a predictor for posttraumatic stress disorder symptoms in post-myocardial infarction patients. J Affect Disord 2021;292:687-94. [Crossref] [PubMed]
- Soldatos CR, Dikeos DG, Paparrigopoulos TJ. Athens Insomnia Scale: validation of an instrument based on ICD-10 criteria. J Psychosom Res 2000;48:555-60. [Crossref] [PubMed]
- Liu J, Qi Y, Si Q, et al. Application progress of fear disease progression-related scale in the evaluation of patients with chronic diseases. Nursing Research 2020;34:3272-6.
- Wu Q, Ye Z, Li L, et al. Chinese version and reliability and validity analysis of the simplified scale of cancer patients’ fear of disease progression. Chinese Journal of Nursing 2015;50:1515-9.
- Chen S, Gao XP, Li L, et al. Preliminary evaluation of reliability and validity of PTSD symptom checklist 90. Chinese Mental Health Journal 2005;13-6. [Crossref]
- Yang X, Yang H, Liu Q, et al. Research on the validity, reliability and influencing factors of the civilian version of PTSD. Chinese Journal of Health Psychology 2007;6-9.
- Fonkoue IT, Michopoulos V, Park J. Sex differences in post-traumatic stress disorder risk: autonomic control and inflammation. Clin Auton Res 2020;30:409-21. [Crossref] [PubMed]
- Kobayashi I, Sledjeski EM, Delahanty DL. Gender and age interact to predict the development of posttraumatic stress disorder symptoms following a motor vehicle accident. Psychol Trauma 2019;11:328-36. [Crossref] [PubMed]
- Morey RA, Gold AL, LaBar KS, et al. Amygdala volume changes in posttraumatic stress disorder in a large case-controlled veterans group. Arch Gen Psychiatry 2012;69:1169-78. [Crossref] [PubMed]
- Andreano JM, Dickerson BC, Barrett LF. Sex differences in the persistence of the amygdala response to negative material. Soc Cogn Affect Neurosci 2014;9:1388-94. [Crossref] [PubMed]
- Lehner M, Skórzewska A, Wisłowska-Stanek A. Sex-Related Predisposition to Post-Traumatic Stress Disorder Development-The Role of Neuropeptides. Int J Environ Res Public Health 2021;19:314. [Crossref] [PubMed]
- Dang A. Characteristics and research status of female cardiovascular diseases. Chinese Journal of Cardiovascular and Cerebrovascular Diseases of the Elderly 2021;23:561-3.
- Arigo D, Juth V, Trief P, et al. Unique relations between post-traumatic stress disorder symptoms and patient functioning in type 2 diabetes. J Health Psychol 2020;25:652-64. [Crossref] [PubMed]
- Chen Y, Guo X, Zhao J, et al. Incidence and influencing factors of post-traumatic stress disorder of mothers with type I diabetes. Chinese Journal of Health Psychology 2015;23:842-5.
- Yu F, Xia M, Liu D. Effect of post-traumatic stress disorder on blood glucose and insulin resistance in patients with type 2 diabetes mellitus. Chinese Journal of Health Psychology 2019;27:1323-6.
- Wolf EJ, Bovin MJ, Green JD, et al. Longitudinal associations between post-traumatic stress disorder and metabolic syndrome severity. Psychol Med 2016;46:2215-26. [Crossref] [PubMed]
- Ribeiro ACAF, Batista TH, Ferrari MS, et al. The accentuation in post-traumatic stress disorder-like symptoms induced by diabetes in rats is not associated with a further increase in astrocyte activation in the hippocampus. Neurosci Lett 2021;762:136174. [Crossref] [PubMed]
- Zhi L, Yu H, Dong B. Changes of serum cTnI, CK-MB, CRP levels and myocardial microcirculation status in patients with myocardial infarction. Journal of Molecular Diagnostics and Therapy 2022;14:491-4.
- Wen F, Chen M, Zhao C, et al. Establishment of cognitive decline risk prediction model for elderly patients with stable coronary heart disease. Journal of Nursing Science 2021;36:21-6.
- Agorastos A, Olff M. Sleep, circadian system and traumatic stress. Eur J Psychotraumatol 2021;12:1956746. [Crossref] [PubMed]
- Brown B, Jones EC, Clark KP, et al. Sleep disturbances and post-traumatic stress disorder in women. Neuro Endocrinol Lett 2014;35:560-6. [PubMed]
- Dong J, Wang J, Yang H, et al. Mediating effect of fear of disease progression in patients with acute myocardial infarction between symptoms of cardiac discomfort and post-traumatic stress disorder. Chinese Journal of Behavioral Medicine and Brain Science 2021;30:806-10.
- Zeng K, Chen X, Tu Y, et al. Correlation analysis between fear of disease progression and symptoms of post-traumatic stress disorder in patients with acute myocardial infarction. Nursing Research 2020;34:380-3.
- Konrad B, Hiti D, Chang BP, et al. Cardiac patients’ perceptions of neighboring patients’ risk: influence on psychological stress in the ED and subsequent posttraumatic stress. BMC Emerg Med 2017;17:33. [Crossref] [PubMed]
- Fait K, Vilchinsky N, Dekel R, et al. Cardiac-disease-induced PTSD and Fear of illness progression: Capturing the unique nature of disease-related PTSD. Gen Hosp Psychiatry 2018;53:131-8. [Crossref] [PubMed]
- Thiele S, Goebel S, Kröger N, et al. Fear of disease progression and relevant correlates in acute leukemia patients prior to allogeneic hematopoietic stem cell transplantation. Psychooncology 2020;29:1248-54. [Crossref] [PubMed]
- Guo L, Ding Y, Zheng Z, et al. Construction of prediction model for influencing factors of perineal incision decision during delivery. Chinese Journal of Nursing 2019;54:1469-74.