Predictive value of machine learning in diagnosing cognitive impairment in patients with Parkinson’s disease: a systematic review and meta-analysis
Highlight box
Key findings
• Machine learning performed potential value for diagnosing PD patients with early cognitive impairment.
What is known and what is new?
• Machine learning for predicting PD with cognitive impairment (PD-CI) exists some debates.
• Although there is certain heterogeneity, machine learning still could be used as a potential diagnostic tool for PD patients with early cognitive impairment (PD-CI).
What is the implication, and what should change now?
• This study provides a theoretical basis for scoring systems of PD-CI with machine learning in the future. More large-scale, multicenter, and multi-ethnic studies need to be conducted in the future for diagnosing PD-CI with machine learning.
Introduction
Parkinson’s disease (PD) is a common disorder of neurodegeneration. It has a reported prevalence of >6 million worldwide, a figure that is 2.5 times larger than that of the past generation, and it has become a major cause of neurological disability (1,2). The incidence rate of PD is not high in those aged <50 years; however, it increases rapidly as age increases, and a study has reported that the incidence rate is the highest among those aged 80 years (3). The median age-standardized incidence of PD in developed countries is 14 per 100,000 people per year, and most PD patients are aged 65 years or older (4). The prevalence of age-adjusted PD has been reported to be lower in Africa than in Europe and the Americas (5-7); however, that of Asia has been found to be identical to that of Europe and the Americas (8). There are some differences in incidence depending on location; however, patients with PD experience a deterioration in body function, and a decline in mobility and cognition, which requires multidisciplinary management, such as drug treatments and rehabilitation therapies (9,10). The decreasing body function, declin mobility and cognition, drug treatments and rehabilitation therapies all of these create a heavy social and financial burden, especially among the elderly (11).
Cognitive decline, which, depending on the severity, ranges from PD with mild cognitive impairment to PD with dementia, is the most common and essential non-motor symptom of PD (12). Recent studies report that the prevalence of dementia is 46% at 10, even 6 years within the progression of PD (13,14). Thus, the early identification of PD patients with a cognitive defect in clinical practice would achieve prompt interventions.
Currently, in clinical practice, the cognitive impairments were mainly diagnosed via clinical interviews by experienced neurologists, which performs unsatisfactorily, and some other related tools, such as individual biomarkers and imaging evidence (15). The underlining reason may be these tools present different part of features of PD-CI. Given the heterogeneity of PD-CI, it may be difficult to replace these tools with each other, not to mention the specific contribution of each of these tools to predicting PD-CI with traditional analysis methods respectively. Due to the complexity and enormity of cognitive scores and image results, it is also hard to handle these data by traditional analysis methods. Recently, machine learning has been applied to the medical field for pre-diagnosis, and some investigators have used this advanced method to predict PD with cognitive impairment (PD-CI). ML can precisely model the relationship between inputs and outputs, and thus generate invisible data. On the other hand, ML make the analysis easier when dealing with complicated and huge data. However, machine learning includes multiple models with differential variables, which leads to certain heterogeneity. Consequently, debate continues as to whether machine learning should be used to diagnose PD-CI (16). The current systematic review and meta-analysis sought to provide a direction for the development and update of the scoring systems used in the diagnosis of PD -CI. We present the following article in accordance with the PRISMA-DTA reporting checklist (available at https://apm.amegroups.com/article/view/10.21037/apm-22-1396/rc) (17).
Methods
The research scheme for the current research was registered with PROSPERO (registration No. CRD42022353619).
Literature retrieval
A search was performed of the PubMed, Cochrane, Embase, and Web of Science databases. Original articles, published from the inception of the databases to 6 August 2022, on the performance of machine learning in diagnosing PD-CI were retrieved. We searched for the relevant articles using keywords plus subject headings. Details of our search strategies are available online in the supplementary materials (Table S1).
Inclusion criteria
Studies were included in the meta-analysis if they met the following inclusion criteria: (I) included PD patients; (II) related to a case-control, cohort, nest case-control, or case-cohort study; (III) included a fully constructed machine-learning prediction model; (IV) did or did not have external validation; and (V) examined different machine-learning studies published with the same data set.
Exclusion criteria
Articles were excluded from the meta-analysis if they met any of the following exclusion criteria: (I) related to a meta-analysis, review, guideline, or expert opinion; (II) only analyzed predictive factors, and did not construct a complete machine-learning model; (III) lacked certain outcome indicators for the predictive accuracy of the risk model [i.e., the receiver operating receiver operating characteristic (ROC) curve, concordance-index (c-index), sensitivity, specificity, accuracy, recovery, precision, confusion matrix, diagnosis 4-grid table, F1 score, and calibration curve]; (IV) had small sample sizes (<50 cases); and (V) only validated mature neuropsychological assessment..
Literature screening and data extraction
We imported the retrieved literature into Endnote. After Endnote’s duplicate identification strategies had been employed, any duplicate articles were manually deleted. Some additional articles were excluded in the title and abstract screening. Next, the full text of the articles deemed to be preliminarily eligible were downloaded and reviewed, and the original studies that met the above-mentioned criteria were selected for inclusion in the systematic analysis.
Information of the included articles were analyzed using a spreadsheet, including the title, first author, study design, geographical location, year of publication (prospective vs. retrospective), patient source (single center vs. multicenter), diagnostic criteria for cognitive impairment, patient numbers in data sets (total, training, and testing sets), internal and external validation (random split vs. n-folds cross validation if internal validation), overfitting method, procedures adopted for missing data, methods of feature screening, model names, predictor types, predictive performance measures [accuracy, specificity, sensitivity, positive and negative predictive accuracy, and c-statistic or area under (the ROC) curve and relevant 95% confidence intervals (CIs)]. Two researchers (i.e., RL, and XZ) independently extracted the described information from the selected articles and performed the cross-validation. If there were discrepancies, a 3rd investigator (i.e., TY) made the determination.
Assessment of risk of bias
The risk of bias in each of the prognostic models developed by the studies was assessed by researchers (i.e., MS and JJ) using PROBAST. A prevalence bias tool was used to systematically evaluate the prognostic or diagnostic predictive models (18,19). This tool examined 4 aspects (i.e., participants, predictors, outcomes, and analysis), and each aspect was examined and marked “yes”, “unclear”, or “no” on the basis of the features of the included study. A classification of “no”, indicated a high risk of bias, while a classification of “yes” indicated a low risk of bias. The overall risk of bias was considered low when all the aspects were marked low risk. The overall risk of bias was considered high if only 1 of the given aspects was marked high risk. The evaluation was visually displayed using Excel 2019.
Outcome index
Our systematic review employed the C-index as the outcome measure to determine the overall accuracy of the response model. The C-index cannot be used to determine the prediction accuracy of a machine-learning model for PD-CI patients if the sample proportion is too small (e.g., <10%). Our systematic review of the outcome measures also examined the sensitivity and specificity of the machine-learning models.
Data synthesis and statistical analysis
We conducted a meta-analysis of the metrics (C-index and accuracy) to evaluate the machine-learning models. If the C-index did not include the 95% CIs and standard errors, we used Debray et al.’s approach to estimate the standard errors (20). In addition, a bivariate mixed-effects model (21) was used for the meta-analysis of sensitivity and specificity. If there was a lack of accuracy in the original study, we calculated accuracy by combining the number of samples of each molecular subtype and the number of modeling samples according to the sensitivity and specificity. Given the differences in the variables included in the learning models of the different original studies and the inconsistent parameters, we prioritized the use of the random-effects model in the meta-analysis. The meta-analysis in this study was implemented in R4.2.0 (R Development Core Team, Vienna, http://www.R-project.org).
Results
Study selection
A systematic literature search was carried out, and 1,787 articles from the above-mentioned databases were initially retrieved. Next, 64 duplicate articles were removed, and after a screening of the titles and abstracts, 38 articles remained. A review of the bibliographies revealed no additional relevant articles. The full texts of the 38 screened articles were then reviewed, and 6 more articles were removed because they fell outside the area of research interest. For further details of our inclusion strategies, see Figure 1. Following a thorough and careful review of the full texts of the remaining articles, 32 studies were ultimately included in the meta-analysis.
Characteristics of the included literature
There was a total of 10,778 participants in the included studies of whom, 2,270 had cognitive defects. Most of the countries that applied prognostic models were in the United States, United Kingdom, and China, and had been published from 2016 to 2022 (Table S2). The diagnostic criteria and the grouping of different studies also differed (https://cdn.amegroups.cn/static/public/apm-22-1396-1.xlsx).
Characteristics of the included prediction models
The most commonly used predictors were neuroimaging, Minimum Mental State Examination (MMSE), Montreal Cognitive Assessment (MoCA), age, sex, disease duration, and composite scores (Table S2). The modeling variables were mainly derived from the clinical features combined with brain magnetic resonance imaging (MRI), which showed that radiomics had significant application value in the diagnosis of PD-CI.
Risk of bias assessment
The supplementary table (available online: https://cdn.amegroups.cn/static/public/apm-22-1396-2.xlsx) shows the risk of bias and assessment results for each research model based on the 4 aspects of PROBAST, and also provides an overall summary of the aspect assessment for the included articles. Collectively, almost all of the results for the models had a high risk of bias. Of the 51 prognostic models, 11 were validated. However, only 2 models had undergone external validation with an independent team. Given the defects caused by the original research, only 2 studies applied prognostic models for which external validation had been performed (Figure 2).
Meta-analysis of prognostic models
Subsequently, 19 meta-analyses of the C-statistics were performed for the 51 prognostic models, and the total C-index values in the training and testing sets were 0.857 (0.842–0.873) and 0.845 (0.804–0.886), respectively. We conducted a subgroup analysis according to the type of machine learning (for further details, see Table 1 and Figure 3). We also summarized the sensitivity and specificity of machine learning in PD-CI diagnosis. The meta-analysis results revealed that the sensitivity of the training set was 0.77 (95% CI: 0.72–0.81) and that of the testing sets was 0.85 (95% CI: 0.82–0.88), and the specificity of the training set was 0.83 (95% CI: 0.80–0.85), and that of the testing sets was 0.74 (95% CI: 0.70–0.78). For further details, see Tables 2,3 and Figure 4.
Table 1
Model | Training sets | Testing sets | |||
---|---|---|---|---|---|
n | C-index (95% CI) | n | C-index (95% CI) | ||
ANN | 4 | 0.872 (0.810–0.934) | 1 | 0.911 (0.839–0.983) | |
Boosting | 4 | 0.840 (0.744–0.937) | 2 | 0.770 (0.684–0.856) | |
Cox | 3 | 0.783 (0.693–0.872) | 3 | 0.819 (0.776–0.862) | |
DT | 6 | 0.788 (0.713–0.863) | – | – | |
KNN | 1 | 0.958 (0.929–0.987) | – | – | |
LDA | 2 | 0.803 (0.773–0.834) | – | – | |
LR | 9 | 0.907 (0.887–0.927) | 2 | 0.926 (0.910–0.942) | |
NB | 10 | 0.856 (0.819–0.892) | – | – | |
RF | 7 | 0.865 (0.833–0.897) | 3 | 0.809 (0.742–0.877) | |
SVM | 13 | 0.882 (0.845–0.918) | 3 | 0.850 (0.755–0.946) | |
Overall | 51 | 0.857 (0.842–0.873) | 14 | 0.845 (0.804–0.886) |
ANN, artificial neural networks; Cox, Cox proportional-hazards model; DT, Decision Tree; KNN, K-Nearest Neighbor; LDA, Linear Discriminant Analysis; LR, logistic regression; NB, Naïve Bayes; RF, Random Forest; SVM, support vector machines.
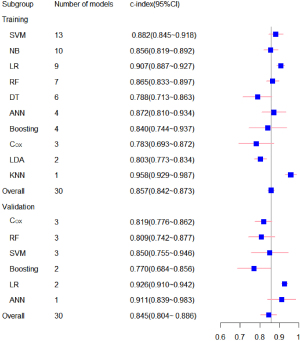
Table 2
Model | Training sets | Training sets | |||
---|---|---|---|---|---|
n | Sensitivity (95% CI) | n | Specificity (95% CI) | ||
ANN | 4 | 0.77 (0.68–0.84) | 4 | 0.93 (0.89–0.95) | |
Boosting | 9 | 0.66 (0.61–0.70) | 9 | 0.79 (0.71–0.85) | |
RF | 8 | 0.74 (0.68–0.79) | 8 | 0.79 (0.74–0.84) | |
SVM | 10 | 0.83 (0.72–0.90) | 10 | 0.85 (0.81–0.89) | |
Overall | 41 | 0.77 (0.72–0.81) | – | 0.83 (0.80–0.85) |
PD-CI, Parkinson’s Disease-Cognitive Impairment; ANN, artificial neural networks; RF, Random Forest; SVM, support vector machines.
Table 3
Model | Testing sets | Testing sets | |||
---|---|---|---|---|---|
n | Sensitivity (95% CI) | n | Specificity (95% CI) | ||
RF | 4 | 0.85 (0.79–0.89) | 4 | 0.74 (0.70–0.78) | |
SVM | 3 | 0.83 (0.71–0.90) | 3 | 0.77 (0.65–0.86) | |
Boosting | 2 | 0.82 (0.73–0.89) | 2 | 0.76 (0.53–0.92) | |
ANN | 1 | 1 | 1 | 0.6 | |
Cox | 1 | 0.87 | 1 | 0.72 | |
Overall | 11 | 0.85 (0.82–0.88) | – | 0.74 (0.70–0.78) |
PD-CI, Parkinson’s Disease-Cognitive Impairment; RF, Random Forest; SVM, support vector machines; ANN, artificial neural networks; Cox, Cox proportional-hazards model.
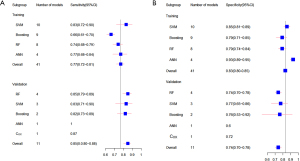
Discussion
This meta-analysis of 32 original studies indicated that machine learning could potentially be an ideal tool for predicting PD-CI, and the total C-index of all models was 0.857 (0.842–0.873). For PD patients with and without cognitive impairment, the overall models in this study had an ideal accuracy with a sensitivity and specificity >70%. In addition, the present study suggested that the accuracy of the testing sets was not significantly lower than that of the training set, which indicated that the machine-learning models had an ideal value in real world. Thus, machine learning may be applied as a potential tool for the identification of PD-CI.
At present, in the systematic review of machine learning for predicting PD, the applied fields, such as motor symptoms, pathology, and pathogenesis, have achieved good results (22,23). A similar investigation was conducted on the use of machine learning in the diagnosis of Alzheimer’s disease (24,25). Inspired by this previous research, we examined the value of machine learning in predicting PD-CI.
In the included original studies, the main variables in the models were generally clinical features and neuroimaging. In recent years, the PD patterns of cognitive neural substrates have been identified using MRI techniques, which can be applied as prediction tools in PD-CI detection; however, few studies have applied such techniques to validate the exact sensitivity and specificity of MRI techniques in predicting PD-CI (12). A previous review and meta-analysis noted that further investigations of potential predictors are needed using multi-modal MRI technology, targeted biomarkers for different cognitive domains, and predictive algorithms (26). The current research used machine learning to build a pre-diagnostic model that contained MRI and some other predictable elements, such as representative biomarkers, and cognitive domain tests (as necessary). This type of model should achieve more accurate results than using the above predictors without machine learning.
Currently, there are several cut-offs for PD-CI in neuropsychological examinations, and a few global scales for cognitive screening (15). Overall, the sensitivity and specificity of pre-diagnostic values using these diverse testing techniques are inadequate. Importantly, changes in PD-CI patients in terms of cognitive performance are largely restricted to certain relevant domains associated with human cognition, but may also include function alteration after long-term follow-up progression. Some researchers have indicated that speech issues appear during the transition to dementia in PD patients, especially in the presentation of problems in the comprehension and production of speech (27). However, these aspects have not been fully and intelligently characterized in PD, and current tests have only been designed to test the sentence comprehension and oral fluency of the subjects (28).
Many investigators have reported that the underlying neuropathology of certain speech problems associated with the temporal lobe and executive-frontal disorders explain speech problems (29). As such, the primary cause leading to disorders may be dopaminergic or cholinergic dysfunction in the described areas (30). Consequently, a combination of radiomics and other different types of markers (e.g., related biomarkers) may perform well, as they use multi-dimensional information. Further, several investigations have explored the combination of machine learning with the above indicators for the diagnosis of Alzheimer’s disease, and reported an increase in the sensitivity and specificity of diagnosing Alzheimer’s disease (25,31). Regrettably, almost no qualified studies that combine the above indicators based on machine learning for the pre-diagnosis of PD-CI have been conducted, but several recent studies have confirmed that neuroimaging and other sensitive predictors, such as quantitative electroencephalograms (qEEG), based on machine learning improve prognostic accuracy (32,33).
In summary, the diagnosis of PD-CI based on brain MRI and cognitive scale assessment tools has certain defects. We encourage and advocate for the application of machine learning. We undertook the first comprehensive and systematic analysis of the application of machine learning in PD-CI. Our findings may lead to advances in digital therapy in this field, and also provide theoretical evidence for the subsequent development of machine-learning models for the diagnosis of this disease.
However, the study also had some limitations. First, it included a variety of models, each of which had a different diagnostic performance. According to previous studies and analyses, PD-CI can be affected by education level and environment, which causes certain heterogeneity. Second, some of the models were only tested with small sample sizes. Third, due to the particularity of this disease, some the original studies were not multicenter studies. Fourth, there is no international consensus on the diagnosis of PD-CI, which also caused certain selective biases. Finally, since the progression of PD-CI is slow and heterogenic, we may need to establish a longitudinal multi-model to enhance the diagnosis accuracy.
Conclusions
Our systematic review showed that machine learning could be used as a prospective diagnostic tool for PD patients with early cognitive impairment, and our findings provide a theoretical basis for the development and update of relevant scoring systems in the future. However, given the high risk of bias in the modeling process, more large-scale, multicenter, and multi-ethnic studies need to be conducted in the future with more effective predictors, especially non-invasive or minimally invasive predictors, to enable the early identification and prediction of this disease.
Acknowledgments
We would like to thank the researchers and study participants for their contributions.
Funding: None.
Footnote
Reporting Checklist: The authors have completed the PRISMA-DTA reporting checklist. Available at https://apm.amegroups.com/article/view/10.21037/apm-22-1396/rc
Conflicts of Interest: All authors have completed the ICMJE uniform disclosure form (available at https://apm.amegroups.com/article/view/10.21037/apm-22-1396/coif). The authors have no conflicts of interest to declare.
Ethical Statement: The authors are accountable for all aspects of the work in ensuring that questions related to the accuracy or integrity of any part of the work are appropriately investigated and resolved.
Open Access Statement: This is an Open Access article distributed in accordance with the Creative Commons Attribution-NonCommercial-NoDerivs 4.0 International License (CC BY-NC-ND 4.0), which permits the non-commercial replication and distribution of the article with the strict proviso that no changes or edits are made and the original work is properly cited (including links to both the formal publication through the relevant DOI and the license). See: https://creativecommons.org/licenses/by-nc-nd/4.0/.
References
- Global, regional, and national burden of neurological disorders, 1990-2016: a systematic analysis for the Global Burden of Disease Study 2016. Lancet Neurol 2019;18:459-80. [Crossref] [PubMed]
- Dorsey ER, Sherer T, Okun MS, et al. The Emerging Evidence of the Parkinson Pandemic. J Parkinsons Dis 2018;8:S3-8. [Crossref] [PubMed]
- Bower JH, Maraganore DM, McDonnell SK, et al. Influence of strict, intermediate, and broad diagnostic criteria on the age- and sex-specific incidence of Parkinson’s disease. Mov Disord 2000;15:819-25. [Crossref] [PubMed]
- Hirtz D, Thurman DJ, Gwinn-Hardy K, et al. How common are the "common" neurologic disorders? Neurology 2007;68:326-37. [Crossref] [PubMed]
- Winkler AS, Tütüncü E, Trendafilova A, et al. Parkinsonism in a population of northern Tanzania: a community-based door-to-door study in combination with a prospective hospital-based evaluation. J Neurol 2010;257:799-805. [Crossref] [PubMed]
- Dotchin C, Msuya O, Kissima J, et al. The prevalence of Parkinson’s disease in rural Tanzania. Mov Disord 2008;23:1567-672. [Crossref] [PubMed]
- Okubadejo NU, Bower JH, Rocca WA, et al. Parkinson’s disease in Africa: A systematic review of epidemiologic and genetic studies. Mov Disord 2006;21:2150-6. [Crossref] [PubMed]
- Zhang ZX, Roman GC, Hong Z, et al. Parkinson’s disease in China: prevalence in Beijing, Xian, and Shanghai. Lancet 2005;365:595-7. [Crossref] [PubMed]
- Rubenis J. A rehabilitational approach to the management of Parkinson’s disease. Parkinsonism Relat Disord 2007;13:S495-7. [Crossref] [PubMed]
- Radder DLM, Nonnekes J, van Nimwegen M, et al. Recommendations for the Organization of Multidisciplinary Clinical Care Teams in Parkinson’s Disease. J Parkinsons Dis 2020;10:1087-98. [Crossref] [PubMed]
- Global, regional, and national burden of Parkinson’s disease, 1990-2016: a systematic analysis for the Global Burden of Disease Study 2016. Lancet Neurol 2018;17:939-53. [Crossref] [PubMed]
- Aarsland D, Creese B, Politis M, et al. Cognitive decline in Parkinson disease. Nat Rev Neurol 2017;13:217-31. [Crossref] [PubMed]
- Williams-Gray CH, Mason SL, Evans JR, et al. The CamPaIGN study of Parkinson’s disease: 10-year outlook in an incident population-based cohort. J Neurol Neurosurg Psychiatry 2013;84:1258-64. [Crossref] [PubMed]
- Pigott K, Rick J, Xie SX, et al. Longitudinal study of normal cognition in Parkinson disease. Neurology 2015;85:1276-82. [Crossref] [PubMed]
- Aarsland D, Batzu L, Halliday GM, et al. Parkinson disease-associated cognitive impairment. Nat Rev Dis Primers 2021;7:47. [Crossref] [PubMed]
- Severson KA, Chahine LM, Smolensky LA, et al. Discovery of Parkinson’s disease states and disease progression modelling: a longitudinal data study using machine learning. Lancet Digit Health 2021;3:e555-64. [Crossref] [PubMed]
- McInnes MDF, Moher D, Thombs BD, et al. Preferred Reporting Items for a Systematic Review and Meta-analysis of Diagnostic Test Accuracy Studies: The PRISMA-DTA Statement. JAMA 2018;319:388-96. [Crossref] [PubMed]
- Wolff RF, Moons KGM, Riley RD, et al. PROBAST: A Tool to Assess the Risk of Bias and Applicability of Prediction Model Studies. Ann Intern Med 2019;170:51-8. [Crossref] [PubMed]
- Moons KGM, Wolff RF, Riley RD, et al. PROBAST: A Tool to Assess Risk of Bias and Applicability of Prediction Model Studies: Explanation and Elaboration. Ann Intern Med 2019;170:W1-W33. [Crossref] [PubMed]
- Debray TP, Damen JA, Riley RD, et al. A framework for meta-analysis of prediction model studies with binary and time-to-event outcomes. Stat Methods Med Res 2019;28:2768-86. [Crossref] [PubMed]
- Reitsma JB, Glas AS, Rutjes AW, et al. Bivariate analysis of sensitivity and specificity produces informative summary measures in diagnostic reviews. J Clin Epidemiol 2005;58:982-90. [Crossref] [PubMed]
- Chandrabhatla AS, Pomeraniec IJ, Ksendzovsky A. Co-evolution of machine learning and digital technologies to improve monitoring of Parkinson’s disease motor symptoms. NPJ Digit Med 2022;5:32. [Crossref] [PubMed]
- Su C, Tong J, Wang F. Mining genetic and transcriptomic data using machine learning approaches in Parkinson’s disease. NPJ Parkinsons Dis 2020;6:24. [Crossref] [PubMed]
- Tzimourta KD, Christou V, Tzallas AT, et al. Machine Learning Algorithms and Statistical Approaches for Alzheimer’s Disease Analysis Based on Resting-State EEG Recordings: A Systematic Review. Int J Neural Syst 2021;31:2130002. [Crossref] [PubMed]
- Chang CH, Lin CH, Lane HY. Machine Learning and Novel Biomarkers for the Diagnosis of Alzheimer’s Disease. Int J Mol Sci 2021;22:2761. [Crossref] [PubMed]
- Hou Y, Shang H. Magnetic Resonance Imaging Markers for Cognitive Impairment in Parkinson’s Disease: Current View. Front Aging Neurosci 2022;14:788846. [Crossref] [PubMed]
- Hobson P, Meara J. Risk and incidence of dementia in a cohort of older subjects with Parkinson’s disease in the United Kingdom. Mov Disord 2004;19:1043-9. [Crossref] [PubMed]
- Robinson GA. Primary progressive dynamic aphasia and Parkinsonism: generation, selection and sequencing deficits. Neuropsychologia 2013;51:2534-47. [Crossref] [PubMed]
- Henry JD, Crawford JR. Verbal fluency deficits in Parkinson’s disease: a meta-analysis. J Int Neuropsychol Soc 2004;10:608-22. [Crossref] [PubMed]
- Pagonabarraga J, Kulisevsky J. Cognitive impairment and dementia in Parkinson’s disease. Neurobiol Dis 2012;46:590-6. [Crossref] [PubMed]
- Martí-Juan G, Sanroma-Guell G, Piella G. A survey on machine and statistical learning for longitudinal analysis of neuroimaging data in Alzheimer’s disease. Comput Methods Programs Biomed 2020;189:105348. [Crossref] [PubMed]
- Zhang J, Gao Y, He X, et al. Identifying Parkinson’s disease with mild cognitive impairment by using combined MR imaging and electroencephalogram. Eur Radiol 2021;31:7386-94. [Crossref] [PubMed]
- Tang C, Zhao X, Wu W, et al. An individualized prediction of time to cognitive impairment in Parkinson’s disease: A combined multi-predictor study. Neurosci Lett 2021;762:136149. [Crossref] [PubMed]
(English Language Editor: L. Huleatt)