The development and validation of a prediction model of lithium carbonate blood concentration by artificial neural network: a retrospective study
Highlight box
Key findings
• Artificial neural network has a good performance in predicting the blood concentration of Li carbonate in patients with bipolar disorder.
What is known and what is new?
• The therapeutic dose of Li carbonate is close to the toxic dose, and Li poisoning is prone to occur.
• Artificial neural network is accurate and effective in predicting the blood concentration of Li carbonate in patients with bipolar disorder. And it does not need more test or examination except for regular information.
What is the implication, and what should change now?
• Artificial neural network will help doctors in clinical practice to identify patients at high risk of Lithium intoxication.
Introduction
Bipolar disorder (BD) is a psychiatric disorder characterized by recurrent episodes of mania, hypomania, mixed states, and depression that are relatively common in clinical practice. Several epidemiological studies in the United States have shown that the prevalence of BD in the general population is about 1–1.3% (1,2). The prevalence in other countries may be similar (3), although some studies have shown that the prevalence of BD may have increased, even reaching 3.0–4.0% (4,5). A previous study has shown a lower prevalence of BD, with prevalence in Hong Kong and the United Kingdom of 0.184% and 0.357%, respectively, in 2018 (6). Lithium (Li) carbonate is a commonly used mood stabilizing drug for mentally ill patients, and it is often used for mental disorders such as BD. In our clinical practice, Li carbonate use is common, and some patients use it for prolonged periods. Since the therapeutic dose of Li carbonate is close to the toxic dose, the effective safety window of treatment is narrow, and Li poisoning is prone to occur. Some articles have pointed out that the safe range of Li carbonate blood concentration should be maintained at 0.60–1.20 mmol/L, and when the concentration is ≥1.50 mmol/L, the incidence of serious adverse reactions will increase significantly (7,8), including kidney failure, seizures, coma, and even death (9). Therefore, it is recommended to monitor the blood concentration of Li carbonate in each patient (10-12). There is evidence that the reference plasma concentration range for Li carbonate varies depending on whether the patient is in an acute or stable phase (13). The concentration reference value in the exacerbation period is slightly higher than that of the maintenance treatment in the stable period. Lithium is almost exclusively excreted via the kidney and the clearance of lithium decrease with aging. Renal insufficiency is associated with a considerable reduction in clearance of lithium and is considered a contraindication to its use (14).
Artificial neural network (ANN) is a dynamic information processing system that responds to continuous input by simulating the structure and function of a biological neural network (15,16). Back propagation (BP) network is currently the most mature and widely used neural network, which usually includes an input layer, an intermediate layer (hidden layer), and an output layer. The input layer is used for information input, the output layer is used to output the processing results of the information in the network, and the middle layer is located between the input layer and the output layer, and the input information is processed and passed to the output layer or the next middle layer. An ANN has strong nonlinear mapping ability, self-organization, self-adaptation, self-learning ability, and high fault tolerance and robustness. Neural networks avoid the disadvantage that pharmacokinetic analysis methods must rely on known models. They do not need to assume a specific pharmacokinetic model in advance, but only need to learn to establish the relationship between input and output based on the data provided to the network. Training a network system for prediction and judgment has unique advantages in pharmacokinetic research (17-19). In this study, 236 patients with mental disorders were used as the research cohort, and a BP neural network combined with the genetic algorithm was used to predict the blood concentration of Li carbonate, which provided the basis for the clinical rapid and effective formulation of individualized drug regimens. We present the following article in accordance with the TRIPOD reporting checklist (available at https://apm.amegroups.com/article/view/10.21037/apm-22-1237/rc).
Methods
This is a respective study using ANN to establish a prediction model for Li carbonate blood concentration in patients with BD.
Study population
In this study, patients with BD who were diagnosed and treated in our hospital from October 2016 to April 2021 were enrolled as the study participants. The inclusion criteria were as follows: (I) age ≥18 years; (II) meeting the diagnostic criteria for BD in the International Statistical Classification of Diseases and Related Health Problems (ICD-10) (20); (III) inpatients taking Li carbonate tablets or Li carbonate sustained-release tablets who had undergone blood concentration monitoring; (IV) liver and kidney function tests conducted before taking the drug; (V) clinical data was complete. The exclusion criteria were as follows: (I) combined with tumor; (II) combined with liver failure and renal failure; (III) pregnant and/or lactating women; (IV) drinking or smoking within 5 days before the detection of Li carbonate concentration. The study was conducted in accordance with the Declaration of Helsinki (as revised in 2013). The study was approved by the Ethics Committee of Tianjin Anding Hospital (No. 2021-25). Individual consent for this retrospective analysis was waived.
Medical treatment
After the patient was admitted to the hospital for a definite diagnosis, the initial dose of lithium carbonate was 150 mg/time, once a day; gradually increased to 300 mg/time, twice a day; the final effective dose was increased to a maximum of 1,500 mg/day, taken in 2 divided doses. The time for taking the medicine was immediately after meals, and the time for taking the medicine with dinner was 17:00. During the same period, according to the patient’s condition, an appropriate combination of quetiapine, duloxetine, olanzapine, sodium valproate, and other drugs was implemented.
Lithium concentration tests
The half-life of Li is about 20 hours and the time to steady state is 5–7 days. Therefore, the blood Li concentration was measured 7 days after reaching the target dose. Detection method: In the early morning, 12 hours after the last dose before the test and in the fasting state, 2 mL of venous blood was drawn, and the Li concentration was determined by the phosphatase method. The detection instrument was as follows: Hitachi automatic biochemical detector 7600 (Hitachi, Tokyo, Japan). The Li concentration determination kit was provided by Sino-Beijing Biotechnology Co., Ltd. (Beijing, China).
Data collection
We collected patient demographic data, including age and gender; physical examination information, including height and weight; laboratory test results, including liver and renal function, and lithium concentrations; medication information, including lithium carbonate usage, concomitant medications, and dose; and information on comorbidities and adverse reactions. Liver and renal function were tested just before lithium carbonate administration.
Statistical analysis and artificial neural network analysis
The ANN fitting module of SPSS 26.0 (IBM Corp., Armonk, NY, USA) was used for modeling and prediction. Data grouping: Using the random number table method, the Li concentration data of 236 patients were randomly divided into 2 groups: 195 cases in the training group and 41 cases in the test group.
Neural network input variable screening
A total of 195 pieces of steady-state whole blood concentration data were used as output variables, patient gender, age, weight, height, daily dose of Li carbonate within 7 days before the measurement of Li concentration, laboratory test results before concentration measurement, and combined medication, and so on, were used as input variables, and the optimal subset regression analysis method was used to screen the influencing factors of Li carbonate blood drug concentration as the input variables of the neural network.
BP neural network
The BP neural network was used for modeling, the hidden layer adopted the tangent S-shaped activation function, the output layer adopted the linear activation function, and the training method adopted the momentum method. The measured value of Li concentration in the training group was used as the output variable, and the influencing factors of Li concentration in “neural network input variable screening” were used as input variables. NeuroSolutions 5.0 full-featured trial software (NeuroDimension, Inc., Gainesville, FL, USA) was used to train the ANN by using genetic algorithm and momentum methods, such as the number of hidden layer nodes, step size, momentum factor, and so on, to be optimized. The network training termination condition was that the mean square error (MSE) of the network was less than a given value of 0.01; or the cross-validation data MSE had started to increase; or reached a predetermined evolutionary generation of 100. The maximum number of training times of the network was 1,000 times, and other parameters were default values. Finally, the nonlinear mapping model between the original data and the Li concentration was obtained.
Model validation
The test group data was used as the validation data, the selected ANN was used to estimate the Li concentration of the test group, the correlation between the estimated value of Li concentration and the measured value was investigated, and a correlation scatter plot was made, and the correlation coefficient close to 1 is considered good. At the same time, the prediction error (PE) was calculated, the mean prediction error (MPE) was used to evaluate the accuracy of the model, the mean absolute prediction error (MAE) was used to evaluate the precision of the model, and the rate of data with relative prediction error ≤10% was calculated.
Results
Baseline characteristics
A total of 236 patients with BD were included in this study, including 88 males and 148 females, aged 18–75 years {median age 38 [30, 52] years}, weight 43–83 kg, mean 61.7±8.1 kg. The daily dose of Li carbonate before Li concentration testing was 150–1,200 mg. Among the 236 patients, 85 were also taking zopiclone, 71 were on quetiapine, 53 were on lorazepam, 48 were on olanzapine, 30 were on sodium valproate, and 29 were on alprazolam. There were 114 cases of hypertension, among whom 92 cases were taking metoprolol and 19 cases were taking nifedipine. There were 23 patients with diabetes mellitus: 17 patients were taking metformin, and 8 patients were taking acarbose. A total of 20 patients were taking statins.
ANN input variables
The optimal subset regression analysis method was used with Li concentration as the dependent variable. When subsets consisted of age, concentration before daily dose, alanine aminotransferase (ALT), aspartate aminotransferase (AST), albumin (ALB), total bilirubin (TBIL), creatinine (Cr) and co-administered zopiclone, quetiapine, pyridoxine, lorazepam, olanzapine, sodium valproate, metoprolol, and statins (1 for combined use, 0 for non-combined use), the established regression equation had the largest corrected R2 (68.3%), the minimum estimated standard error (SE =3.049), and this variable set was the best subset as the input variable of the neural network. There were 195 cases in the training group and 41 cases in the test group. The baseline data and Li concentration comparison of the 2 groups are shown in Table 1.
Table 1
Parameters | Training group (n=195) | Testing group (n=41) | t/χ2 value | P value |
---|---|---|---|---|
Age (years) | 38 [29, 50] | 39 [32, 54] | 0.641 | 0.507 |
Male (n) | 71 | 17 | 0.370 | 0.543 |
Smoking, n (%) | 21 (10.8) | 8 (19.5) | 2.403 | 0.121 |
Alcohol, n (%) | 33 (16.9) | 10 (24.5) | 1.268 | 0.260 |
Weight (kg) | 61.5±8.6 | 62.9±9.1 | 0.938 | 0.349 |
SBP (mmHg) | 131.7±10.4 | 132.4±11.8 | 0.382 | 0.703 |
WBC (×109/L) | 7.2±2.5 | 7.5±2.9 | 0.679 | 0.498 |
HB (g/L) | 144.3±8.7 | 143.5±9.6 | 0.526 | 0.600 |
PLT (×109/L) | 203.8±29.3 | 199.9±32.5 | 0.760 | 0.448 |
ALT (U/L) | 27.4±6.9 | 25.9±7.4 | 1.249 | 0.213 |
TBIL (μmol/L) | 14.2±3.5 | 14.9±4.2 | 1.123 | 0.263 |
CR (μmol/L) | 73.1±14.6 | 74.2±15.7 | 0.433 | 0.666 |
TC (mmol/L) | 5.7±1.3 | 5.8±1.7 | 0.423 | 0.673 |
TG (mmol/L) | 2.2±0.6 | 2.3±0.9 | 0.881 | 0.380 |
GLU (mmol/L) | 6.1±1.4 | 6.2±1.8 | 0.394 | 0.694 |
Data are shown as median [range], n, n (%), or mean ± SD. SBP, systolic blood pressure; WBC, white blood cell; HB, hemoglobin; PLT, platelet; ALT, alanine aminotransferase; TBIL, total bilirubin; CR, creatinine; TC, total cholesterol; TG, triglyceride; GLU, glucose.
Back propagation ANN
The network parameters were optimized by genetic algorithm and momentum method, and a 3-layer BP neural network was established. The hidden layer adopted the tangent S-type activation function, and the output layer adopted the linear activation function. In the input layer, the input variables in order of significance were age, daily dose before concentration measurement, ALT, AST, ALB, TBIL, Cr, and combined use of zopiclone, quetiapine, lorazepam, olanzapine, sodium valproate, metoprolol, and statins, with a total of 14 nodes. The number of hidden layer nodes was determined to be 13 according to the results of trial and error. The output layer was 1 node, that is, the output variable was the Li concentration. The neural network structure diagram is shown in Figure 1. The performance of the established ANN was verified, and the results were as follows: MSE =0.00439, gradient magnitude =0.00052, number of verification checks =0, and the correlation coefficient =0.973.
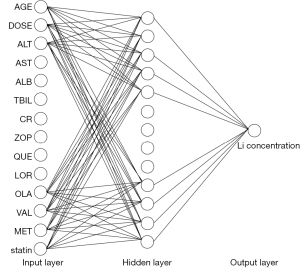
Concentration prediction using back propagation ANN
Model application validation was performed on the patients in the test group, and the age of the patients, daily dose before concentration measurement, ALT, AST, ALB, TBIL, Cr, the combined use of zopiclone, quetiapine, lorazepam, olanzapine, sodium valproate, metoprolol, and statins were collected as input layer variables to predict the serum Li concentration of patients, and compare it with the actual concentration. The correlation coefficient r=0.9883, r2=0.9767, P<0.001, as shown in Figure 2. The prediction accuracy is shown in Table 2, the prediction error range was −0.05 to 0.07 mmol/L, and the deviation range was −18.52% to 13.04%, the mean absolute error was 0.03, and the mean prediction error deviation was between −10% and 10% in 33 cases (80.5%).
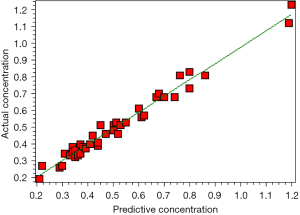
Table 2
AC (mmol/L) | PC (mmol/L) | D (%) |
---|---|---|
0.76 | 0.81 | −6.17 |
0.80 | 0.83 | −3.61 |
0.21 | 0.19 | 10.53 |
0.22 | 0.27 | −18.52 |
0.29 | 0.26 | 11.54 |
0.34 | 0.38 | −10.53 |
0.45 | 0.51 | −11.76 |
0.50 | 0.51 | −1.96 |
0.80 | 0.73 | 9.59 |
0.86 | 0.81 | 6.17 |
1.20 | 1.23 | −2.44 |
0.44 | 0.39 | 12.82 |
0.47 | 0.46 | 2.17 |
0.51 | 0.53 | −3.77 |
0.35 | 0.33 | 6.06 |
0.33 | 0.33 | 0.00 |
0.34 | 0.35 | −2.86 |
0.50 | 0.48 | 4.17 |
0.62 | 0.57 | 8.77 |
0.68 | 0.7 | −2.86 |
0.35 | 0.32 | 9.37 |
0.38 | 0.38 | 0.00 |
0.36 | 0.33 | 9.09 |
0.41 | 0.4 | 2.50 |
0.42 | 0.45 | −6.67 |
0.52 | 0.46 | 13.04 |
0.53 | 0.51 | 3.92 |
0.6 | 0.61 | −1.64 |
1.19 | 1.12 | 6.25 |
0.31 | 0.34 | −8.82 |
0.37 | 0.34 | 8.82 |
0.35 | 0.36 | −2.78 |
0.37 | 0.4 | −7.50 |
0.55 | 0.53 | 3.77 |
0.7 | 0.68 | 2.94 |
0.67 | 0.68 | −1.47 |
0.74 | 0.68 | 8.82 |
0.3 | 0.27 | 11.11 |
0.39 | 0.37 | 5.41 |
0.44 | 0.41 | 7.32 |
0.61 | 0.56 | 8.93 |
ANN, artificial neural network; AC, actual concentration; PC, predictive concentration; D, deviation.
Discussion
The effective plasma concentration range of Li carbonate is relatively narrow. The commonly used starting dose of 125–250 mg/d is safe for most people, but it may be excessive for a few patients. Moreover, in the process of gradually increasing the dose, some patients may be more sensitive, and a slight increase in the dose can lead to excessive blood concentration and adverse reactions (7). Therefore, it is of great clinical significance to predict the blood drug concentration of patients before taking medication (21). In this study, through retrospective analysis, an ANN was used to establish a model for predicting the blood concentration of Li carbonate, and it was verified in randomly selected cases. The results showed that the correlation coefficient r2 between the predicted blood concentration and the actual blood concentration reached 0.9767, the prediction error range was −0.05–0.07 mmol/L, the deviation range was −18.52–13.04%, the mean absolute error was 0.03, and the prediction error deviation of 80.5% was between −10% and 10%.
According to the records of Li carbonate drug instructions, the reference range of blood drug concentration in the acute phase is 0.60–1.20 mmol/L, and the reference value of blood drug concentration in the maintenance phase was 0.40–0.80 mmol/L. In the process of using Li carbonate to stabilize mood in mentally ill patients, the blood concentration should be closely monitored to avoid Li poisoning (21). Due to the existence of individual differences, including differences in gender, age, body weight, underlying diseases, concomitant medication, dietary habits, and medication compliance, even patients with Li carbonate concentrations within the safe concentration range may experience toxic reactions. Elderly patients should receive a reduced dose due to their reduced clearance rate. Low body weight patients tend to have high levels of lithium in their bodies, and care should be taken to avoid lithium poisoning (12). Therefore, in clinical practice, in addition to monitoring the blood concentration of Li carbonate, it is also necessary to observe the clinical manifestations of patients, to ensure that they can receive individualized drug treatment, to ensure the efficacy of drug treatment, and to avoid adverse reactions. Adverse reactions caused by Li carbonate often involve multiple systems, and the aggravation of adverse reactions such as the digestive system and the nervous system is likely to be a precursor to Li poisoning (22). The 2017 “Consensus Guidelines for Therapeutic Drug Monitoring in Neuropsychopharmacology” mentioned that the ratio of drug concentration to dose (C/D) is inversely proportional to drug clearance, and higher C/D indicates slow drug clearance (23). This consensus concluded that differences in gender and age will have a significant impact on C/D. The C/D of female and elderly patients is significantly higher than that of males and patients under the age of 60, indicating that the clearance rate of Li carbonate in the body is reduced, and that it is easy for the drug to accumulate in the body (23). It is recommended to appropriately reduce the amount of Li carbonate when taking it (23). Since Li carbonate does not bind to plasma proteins and tissue proteins, it is widely distributed in the body, which may easily lead to higher concentrations in those with a lower body weight. Patients with mental illness often have multiple psychiatric problems and frequently require the use of multiple antipsychotic drugs at the same time. Concomitant use of other antipsychotic drugs is likely to interact with Li carbonate, especially those excreted by the kidneys.
In clinical practice, for BD patients, if not necessary, many indicators are generally not detected. At the same time, most patients need to use a combination of drugs to improve symptoms, such as quetiapine, zopiclone, olanzapine, and other drugs. In addition, these patients commonly take drugs for the treatment of comorbidities; as age gradually increases, the prevalence of chronic diseases increases, and they often need to take various drugs for the treatment of chronic diseases. Combining these factors, we incorporated the most used clinical indicators and combination drugs and the simplest information of patients to establish a prediction model of Li carbonate plasma concentration through ANN without increasing the number of patient testing items and testing times. This method is convenient for clinical use. This method can fully factor in the differences between patients, without considering the pharmacokinetic model, and directly establish the mapping relationship between the patient’s dose, physiological, pathological data, and the blood drug concentration. Clinicians/pharmacists can use the information directly to predict the blood drug concentration, which is easy to practice.
ANN accumulates “experience” by “learning” from a large amount of data, thereby making predictions on new data. The fundamental task of training a neural network is to ensure that the network model has good applicability to non-training samples (test samples) (19). In order to avoid the phenomenon of “over-training” in the training process of ANN and ensure high enough adaptability, the sample data is generally divided into 3 groups: Training set, used for model training, generally about 70% of the total sample data; Validation set, supervise the model performance during the training process, and stop training after reaching a certain standard, generally 15–20% of the data; Testing set: used to verify the adaptability of the network, the proportion is generally 15–20%. In the case of relatively small sample size, validation set is not necessary (24). Collecting enough samples is an important factor affecting whether an ANN can achieve a good prediction effect, but there is no unified conclusion about the sample size required to establish ANN for pharmacokinetic research. Usually, the more variables introduced into the ANN, the larger the sample size required. According to the experience of this study, the ANN is suitable for the pharmacokinetic study of Li carbonate, and the total sample size should be more than 200 cases. Before determining the input variables, the optimal subset regression analysis method can be used to find the best variable subset among all possible combinations of the input variables, and the regression equation can be established with fewer variables, so that the regression equation has the largest correction r2, and the smallest estimated standard error. The Mallows’ Cp statistic is closest to the number of input variables in the regression equation. In this study, after optimal subset regression analysis, age, daily dose before concentration measurement, ALT, AST, ALB, TBIL, Cr, and the combined use of zopiclone, quetiapine, lorazepam, olanzapine, sodium valproate, metoprolol and statins, a total of 14 nodes formed the best subset as input to the neural network variable. Among these variables, some variables have a certain relationship with the blood concentration of Li carbonate, such as old age, Cr, and so on, yet some may have nothing to do with the plasma concentration of Li carbonate. For example, quetiapine and olanzapine have no effect on the plasma concentration of Li carbonate (25,26).
This study had some limitations. First, this was a retrospective study, and many patients could not be included in the study due to incomplete data, which possibly cause a decrease in the representativeness of the sample. Second, this study has not been validated in a prospective cohort, and its clinical application value needs further research and observation. Third, this study did not make further attempts to predict the plasma concentration of Li carbonate with fewer input variables. Fourth, high correlation coefficients do not equal to high accuracy. Therefore, the results of this study should be carefully used in clinical practice. In future studies, variables unrelated to the plasma concentration of Li carbonate may be considered for removal from the model to further simplify the model.
Conclusions
Through this retrospective study, we established an ANN model with good performance in predicting the blood concentration of Li carbonate in patients with BD. It’s worthy to further investigate the correlation, accuracy, and precision of this model in future studies, to validate its usefulness in patients’ treatment.
Acknowledgments
Funding: None.
Footnote
Reporting Checklist: The authors have completed the TRIPOD reporting checklist. Available at https://apm.amegroups.com/article/view/10.21037/apm-22-1237/rc
Data Sharing Statement: Available at https://apm.amegroups.com/article/view/10.21037/apm-22-1237/dss
Conflicts of Interest: All authors have completed the ICMJE uniform disclosure form (available at https://apm.amegroups.com/article/view/10.21037/apm-22-1237/coif). The authors have no conflicts of interest to declare.
Ethical Statement: The authors are accountable for all aspects of the work in ensuring that questions related to the accuracy or integrity of any part of the work are appropriately investigated and resolved. The study was conducted in accordance with the Declaration of Helsinki (as revised in 2013). The study was approved by the Ethics Committee of Tianjin Anding Hospital (No. 2021-25). Individual consent for this retrospective analysis was waived.
Open Access Statement: This is an Open Access article distributed in accordance with the Creative Commons Attribution-NonCommercial-NoDerivs 4.0 International License (CC BY-NC-ND 4.0), which permits the non-commercial replication and distribution of the article with the strict proviso that no changes or edits are made and the original work is properly cited (including links to both the formal publication through the relevant DOI and the license). See: https://creativecommons.org/licenses/by-nc-nd/4.0/.
References
- Bebbington P, Ramana R. The epidemiology of bipolar affective disorder. Soc Psychiatry Psychiatr Epidemiol 1995;30:279-92. [Crossref] [PubMed]
- Kessler RC, McGonagle KA, Zhao S, et al. Lifetime and 12-month prevalence of DSM-III-R psychiatric disorders in the United States. Results from the National Comorbidity Survey. Arch Gen Psychiatry 1994;51:8-19. [Crossref] [PubMed]
- Weissman MM, Bland RC, Canino GJ, et al. Cross-national epidemiology of major depression and bipolar disorder. JAMA 1996;276:293-9. [Crossref] [PubMed]
- Steel Z, Marnane C, Iranpour C, et al. The global prevalence of common mental disorders: a systematic review and meta-analysis 1980-2013. Int J Epidemiol 2014;43:476-93. [Crossref] [PubMed]
- Godman B, Grobler C, Van-De-Lisle M, et al. Pharmacotherapeutic interventions for bipolar disorder type II: addressing multiple symptoms and approaches with a particular emphasis on strategies in lower and middle-income countries. Expert Opin Pharmacother 2019;20:2237-55. [Crossref] [PubMed]
- Ng VWS, Man KKC, Gao L, et al. Bipolar disorder prevalence and psychotropic medication utilisation in Hong Kong and the United Kingdom. Pharmacoepidemiol Drug Saf 2021;30:1588-600. [Crossref] [PubMed]
- Ott M, Stegmayr B, Salander Renberg E, et al. Lithium intoxication: Incidence, clinical course and renal function - a population-based retrospective cohort study. J Psychopharmacol 2016;30:1008-19. [Crossref] [PubMed]
- Hanyu S, Sugita N, Matsuda M, et al. Lithium intoxication-induced dysgeusia accompanied by glossalgia in a patient receiving lithium carbonate: a case report. J Med Case Rep 2020;14:149. [Crossref] [PubMed]
- Oruch R, Elderbi MA, Khattab HA, et al. Lithium: a review of pharmacology, clinical uses, and toxicity. Eur J Pharmacol 2014;740:464-73. [Crossref] [PubMed]
- Baird-Gunning J, Lea-Henry T, Hoegberg LCG, et al. Lithium Poisoning. J Intensive Care Med 2017;32:249-63. [Crossref] [PubMed]
- Malhi GS, Gessler D, Outhred T. The use of lithium for the treatment of bipolar disorder: Recommendations from clinical practice guidelines. J Affect Disord 2017;217:266-80. [Crossref] [PubMed]
- Rej S, Herrmann N, Gruneir A, et al. Blood Lithium Monitoring Practices in a Population-Based Sample of Older Adults. J Clin Psychiatry 2018;79:17m12095.
- Malhi GS, Bell E, Outhred T, et al. Lithium therapy and its interactions. Aust Prescr 2020;43:91-3. [Crossref] [PubMed]
- Grandjean EM, Aubry JM. Lithium: updated human knowledge using an evidence-based approach. Part II: Clinical pharmacology and therapeutic monitoring. CNS Drugs 2009;23:331-49. [Crossref] [PubMed]
- Renganathan V. Overview of artificial neural network models in the biomedical domain. Bratisl Lek Listy 2019;120:536-40. [Crossref] [PubMed]
- Nair TM. Building and Interpreting Artificial Neural Network Models for Biological Systems. Methods Mol Biol 2021;2190:185-94. [Crossref] [PubMed]
- Bräm DS, Parrott N, Hutchinson L, et al. Introduction of an artificial neural network-based method for concentration-time predictions. CPT Pharmacometrics Syst Pharmacol 2022;11:745-54. [Crossref] [PubMed]
- Xu Y, Lou H, Chen J, et al. Application of a Backpropagation Artificial Neural Network in Predicting Plasma Concentration and Pharmacokinetic Parameters of Oral Single-Dose Rosuvastatin in Healthy Subjects. Clin Pharmacol Drug Dev 2020;9:867-75. [Crossref] [PubMed]
- Yamamura S, Kawada K, Takehira R, et al. Artificial neural network modeling to predict the plasma concentration of aminoglycosides in burn patients. Biomed Pharmacother 2004;58:239-44. [Crossref] [PubMed]
- Phillips ML, Kupfer DJ. Bipolar disorder diagnosis: challenges and future directions. Lancet 2013;381:1663-71. [Crossref] [PubMed]
- Sheikh M, Qassem M, Triantis IF, et al. Advances in Therapeutic Monitoring of Lithium in the Management of Bipolar Disorder. Sensors (Basel) 2022;22:736. [Crossref] [PubMed]
- Hlaing PM, Isoardi KZ, Page CB, et al. Neurotoxicity in chronic lithium poisoning. Intern Med J 2020;50:427-32. [Crossref] [PubMed]
- Hiemke C, Bergemann N, Clement HW, et al. Consensus Guidelines for Therapeutic Drug Monitoring in Neuropsychopharmacology: Update 2017. Pharmacopsychiatry 2018;51:9-62. [Crossref] [PubMed]
- Terziyan V, Kaikova O. Neural Networks With Disabilities: An Introduction to Complementary Artificial Intelligence. Neural Comput 2021;34:255-90. [Crossref] [PubMed]
- DeVane CL, Nemeroff CB. Clinical pharmacokinetics of quetiapine: an atypical antipsychotic. Clin Pharmacokinet 2001;40:509-22. [Crossref] [PubMed]
- Sun L, Yagoda S, Yao B, et al. Combination of Olanzapine and Samidorphan Has No Clinically Significant Effect on the Pharmacokinetics of Lithium or Valproate. Clin Drug Investig 2020;40:55-64. [Crossref] [PubMed]
(English Language Editor: J. Jones)