Development of a prognostic nomogram based on objective blood test data for patients with advanced cancer undergoing palliative care
Highlight box
Key findings
• Serum calcium (>2.65 mmol/L), neutrophil count (<2 and >7 mmol/L), urea (>7.6 nmol/L), and glutamic oxalacetic transaminase (>40 U/L) were identified and incorporated into a nomogram to predict the 30-day survival of patients with advanced cancer.
What is known and what is new?
• Most survival models mainly contain subjective variables. Few studies have used nomograms that are entirely based on objective blood test parameters to predict the survival of patients with advanced cancer.
• A completely objective prognostic nomogram of 30-day survival for patients with advanced cancer was developed and validated, which was characterized by simplicity, objectivity, and ease of use.
What is the implication, and what should change now?
• With the advantages of being easily available and accurately measured, blood data and other objective indicators are becoming more important in the construction of prognosis models. More objective indicators need to be validated and incorporated in multicenter, prospective prognosis studies.
Introduction
The Global Cancer Statistics 2020 displayed the global cancer burden is expected to be 28.4 million cases in 2040, a 47% rise from 2020 (1). The global need for palliative care to alleviate the suffering of advanced cancer patients is greater than ever. Countless amounts of time and money have been used to treat advanced cancer patients every year (2), who no longer respond well to curative or life-prolonging treatments. Application of quality care indicators suggests that some interventions near the end of life (e.g., chemotherapy) may indicate poor patient care (3). Thus, early palliative care is needed to eliminate the torment caused by overtreatment or improper treatment, and to reduce the inefficient use of medical resources.
In palliative care practice, the focus is on predicting how long patients are expected to live rather than predicting their response to further treatment (4). Accurate prognosis estimation can help assess overall survival (OS) and provide early palliative care. In addition, prognosis awareness can relieve anxiety associated with prognostic uncertainty of patients and caregivers, assist therapeutic decision-makings, and facilitate the patient and the caregivers in advanced care planning (ACP) (5,6). Since the Working Group of the Research Network of the European Association for Palliative Care recommended the use of some indicators, including clinician prediction of survival (CPS), biochemical indicators, clinical signs, and psychosocial variables, to predict the survival of advanced patients (7), numerous prognostic models have been developed, evolved, and validated (8). However, most of them have incorporated subjective variables such as the patient’s symptoms, performance status, and the CPS, which are greatly dependent on the evaluator’s experience and competence (9). Recent research revealed that the accuracy of the palliative prognostic (PaP) score will be improved when the CPS, a well-known subjective measure, is excluded from the composite score (10).
With the advantages of being unbiased and authentic, objective biomarkers could potentially reveal the current status of patients and assist clinicians in decision making. Meanwhile, more extensive use of objective variables, such as laboratory findings and vital signs, has been strongly recommended when developing prognostic models (11). Completely objective prediction tools have emerged recently (12-14). However, most of these identified individual predictive factors, but failed to calculate survival probability (15). Furthermore, some of these tools are not feasible and appropriate for clinical use.
Nomograms, used to evaluate and visualize the survival probability of patients via a simple and clear figure, have been used to predict survival rates in patients with different cancers (16,17). However, few studies have used nomograms that are entirely based on objective blood test parameters to predict the survival of patients with advanced cancer. Hence, this current investigation identified the independent prognostic factors and developed and validated a novel nomogram using completely objective blood parameters for advanced cancers patients in a palliative care setting. We present this article in accordance with the TRIPOD reporting checklist (available at https://apm.amegroups.com/article/view/10.21037/apm-22-1058/rc).
Methods
Study design
This retrospective study was conducted at Hunan Cancer Hospital , China. The article does not contain any clinical trials with human participants nor animals. The study was conducted in accordance with the Declaration of Helsinki (as revised in 2013). The study was approved by the Hunan Cancer Hospital Research Ethics Committee (No. 12034209) and individual consent for this retrospective analysis was waived.
Patients
Patients with advanced cancer who were admitted to the palliative care unit between January 2020 and December 2021 were retrospectively reviewed. The palliative care unit aims to provide integrated ongoing support (including symptom control, psychological support, mental comforts, etc.) after the diagnosis of a life-limiting condition (for example cancer), rather than curing the disease.
The following inclusion criteria were applied: (I) patients who were diagnosed with any type of stage III or IV cancer by more than two physicians according to The TNM Staging System; (II) patients who had a definite outcome of either hospital discharge or death; and (III) patients who were aged 18 years and older. Patients who received chemoradiotherapy at least once from the time of data collection to death were excluded due to the effects of treatment on survival.
Demographic and biochemical data were collated from case records. Biochemical data was recorded from blood tests within one week after the first admission. If patients had a later readmission, only the initial test values were considered. Survival days were calculated from the date of first blood test to the date of death. Any case with more than 3 missing data or unknown survival time was censored. After enrollment, all patients were randomly grouped into either a training set or a validation set according to a 3:1 ratio. The predictive nomogram was constructed using the training set based on demographic data and results of the first laboratory tests after admission, and then validated using the validation set.
Data collection
Demographic characteristic data were collected, including age, gender, primary cancer, metastasis, body mass index (BMI), and overall survival (OS). The following parameters were assessed: serum natrium (Na; mmol/L), serum calcium (Ca; mmol/L), serum potassium (K; mmol/L), serum chloride (Cl; mmol/L), urea (BUN; mmol/L), uric acid (UA; mmol/L), alanine aminotransferase (ALT; U/L), glutamic oxalacetic transaminase (GOT; U/L), creatinine (Cr; mmoI/L), total bilirubin (Tbil; mmoI/L), albumin (Alb; G/L), red blood cell count (RBC; 1012/L), platelet count (PLT; 109/L), leukocyte count (WBC; 109/L), lymphocyte count (LY; 109/L), neutrophil count (NEUT; 109/L), hemoglobin (Hb; G/L), the neutrophil/lymphocyte ratio (NLR), platelet/lymphocyte ratio (PLR), and prognostic nutritional index (PNI). The PNI was calculated as follows: [lymphocyte count] × 5 + [albumin concentration].
To improve the efficiency of data collection and avoid any bias, a structured electronic extraction form was specially designed for the study. Data were obtained by communicating effectively with medical workers and double checking with them. All collectors had completed research courses (including medical statistics) and received training on how to extract medical data. The data were collected and analyzed anonymously. All the raw data analyzed during this study are included in the website: https://cdn.amegroups.cn/static/public/apm-22-1058-01.xlsx.
Statistical analysis
Enumeration data are described as N (%), measurement data are described as mean ± standard deviation (SD) or median (inter-quartile range, IQR). Univariable analyses were performed for each factor through Cox proportional hazard regression. Variables with P values less than 0.05 were selected into multiple Cox regression. Subsequently, factors with prognostic significance in multiple Cox regression were used for survival prediction and a nomogram was built to visualize the model.
The discrimination and calibration were obtained to evaluate the predictive performance of the nomogram. Discrimination evaluates how well the predicted risks distinguish between patients with and without disease (death). The discrimination properties were evaluated by means of survival analysis, receiver operating characteristic curve (ROC), under the ROC curve (AUC), and the Harrell’s concordance index (C-index). The score of each participant was calculated based on the nomogram model, which was then used in ROC curve analyses to identify the points of the highest prognostic accuracy of 30 days. Patients were divided into different risk groups and survival analysis was compared. The C-index value ranges from 0 to 1, and a value of 0.5 indicates no discrimination, whereas a value of 1.0 indicates perfect discrimination. Calibration evaluates the reliability of the estimated risks, which was used to graphically evaluate the calibration of the nomogram in both the training and validation sets. The model was performed by comparing the predicted probability of survival versus the actual probability of survival, again using 1000 bootstrap re-samples to reduce the overfit bias. The predictions falling on a 45-degree diagonal line indicate a well-calibrated model. Discrimination and calibration alone cannot inform us whether the model is beneficial for clinical decision-making (18). Thus, the decision curve analysis (DCA) has been developed to summarize the performance of the model in supporting decision making. DCA is a statistical method to evaluate whether a model is useful in supporting clinical decisions, and it was applied to evaluate the net benefit from the perspective of clinical consequences in present study. The ROC curve, survival curves, calibration, and DCA curve were compared between the training set and the validation set.
Statistical analysis was performed using Statistical Package for the Social Sciences (SPSS) software (Version 19.0) and R statistical software (rms package and Survival package). P values <0.05 were considered statistically significant.
Results
Patient characteristics
After careful inspection, 13 patients were excluded due to absent blood data and 16 were excluded due to the missed survival time. Ultimately, a total of 162 patients were enrolled in training set (Figure 1). The patient characteristics are listed in Table 1. The median age was 55 [49, 65] years in training set. Lung cancer was the most common primary cancer, followed by colorectal cancer.
Table 1
Characteristics | Training set (n=162), n (%) | Validation set (n=54), n (%) |
---|---|---|
Age (year), median [IQR] | 55 [49, 65] | 57 [50, 65] |
Male | 87 (53.7) | 28 (51.9) |
Primary cancer diagnoses | ||
Lung cancer | 46 (28.4) | 15 (27.7) |
Colorectal cancer | 23 (14.2) | 7 (13.0) |
Liver/biliary ducts cancer | 12 (7.4) | 8 (14.8) |
Gynecological oncology | 11 (6.8) | 4 (7.4) |
Breast cancer | 9 (5.6) | 6 (11.1) |
Oral cancer | 8 (4.9) | 4 (7.4) |
Gastric cancer | 7 (4.3) | 4 (7.4) |
Esophageal cancer | 6 (3.7) | 2 (3.7) |
Pancreatic cancer | 5 (3.0) | 1 (1.9) |
Nasopharynx cancer | 4 (2.5) | 2 (3.7) |
Renal cancer | 4 (2.5) | – |
Othersa | 27 (16.7) | 1 (1.9) |
Metastatic site | ||
Bone | 66 (40.7) | 21 (38.8) |
Lymph | 48 (29.6) | 10 (18.5) |
Liver | 47 (29) | 9 (16.7) |
Lung | 31 (19.1) | 14 (26.0) |
Median survival (IQR days) | 17 [8, 37]* | 21 [10, 46] |
a, leukemia [2], tonsil cancer [2], prostate cancer [3], head and neck cancer [3], middle ear cancer [1], olfactory nerve cancer [1], peritoneal cancer [3], laryngeal cancer [2], myeloma [2], glioma [3], lymph cancer [3], connective tissue cancer [2], pleural cancer [1]. IQR, interquartile range.
Variables associated with overall survival (OS) of patients
Univariable Cox regression analyses revealed that serum natrium, serum calcium, leukocyte count, neutrophil count, uric acid, urea, glutamic oxalacetic transaminase, total bilirubin, and neutrophil/lymphocyte ratio were significant associated with OS (Table 2). Multivariate Cox proportional hazards regression analyses demonstrated that serum calcium (>2.65 mmol/L), neutrophil count (<2 and >7 mmol/L), urea (>7.6 nmol/L), and glutamic oxalacetic transaminase (>40 U/L) were independent risk factors for OS (Figure 2).
Table 2
Variables | N (%) | Median [IQR] | HR (95.0% CI) | W | P value |
---|---|---|---|---|---|
Age (reference: <50 years) | 40 (24.7) | 20 [10, 30] | |||
50–60 | 59 (36.4) | 23 [8, 48] | 0.81 (0.54, 1.22) | −1.015 | 0.310 |
>60 | 63 (38.9) | 12 [7, 30] | 0.92 (0.62, 1.38) | −0.4 | 0.688 |
Gender (reference: male) | 87 (53.7) | 18 [9, 35] | |||
Female | 78 (46.3) | 15 [7, 38] | 1.01 (0.74, 1.39) | 0.09 | 0.928 |
BMI (reference: 18.5–24.9 kg/m2) | 84 (51.9) | 17 [6, 40] | |||
<18.5 | 59 (36.4) | 16 [10, 36] | 0.998 (0.71, 1.4) | −0.01 | 0.992 |
25–29.9 | 18 (11.1) | 17 [10, 34] | 0.99 (0.60, 1.67) | −0.003 | 0.997 |
≥30 | 1 (0.6) | – | 0.59 (0.08, 4.23) | −0.528 | 0.598 |
Na (reference: 135–146 mmol/L) | 76 (46.9) | 21 [10, 49] | |||
<135 | 85 (52.5) | 15 [7, 30] | 1.3 (0.95, 1.78) | 1.636 | 0.109 |
>146 | 1 (0,6) | – | 14.1 (1.85, 107.4) | 2.551 | 0.011 |
K (reference: 3.5–5.1 mmol/L) | 120 (74.1) | 20 [9, 40] | |||
<3.5 | 28 (17.3) | 15 [6, 37] | 1.25 (0.82, 1.89) | 1.04 | 0.2983 |
>5.1 | 14 (8.6) | 11 [6, 17] | 1.72 (0.98, 3.0) | 1.894 | 0.06 |
Cl (reference: 101–109 mmol/L) | 44 (27.2) | 30 [10, 54] | |||
<101 | 111 (68.5) | 14 [8, 30] | 1.4 (0.98,1.99) | 1.873 | 0.061 |
>109 | 7 (4.3) | 18 [7, 78] | 1.1 (0.49, 2.42) | 0.205 | 0.838 |
Ca (reference: 2.2–2.65 mmol/L) | 53 (32.7) | 20 [10, 42] | |||
<2.2 | 100 (61.7) | 18 [8, 40] | 1.11 (0.79. 1.55) | 0.615 | 0.539 |
>2.65 | 9 (5.6) | 8 [5, 20] | 2.549 (1.24, 5.23) | 2.554 | 0.011 |
ALB (reference: 34–48 G/L) | 69 (42.6) | 20 [10, 49] | |||
<34 | 89 (54.9) | 15 [8, 33] | 1.25 (0.91, 1.72) | 1.38 | 0.167 |
>48 | 4 (2.5) | 22 [11, 125] | 0.63 (0.22, 1.79) | −0.86 | 0.388 |
RBC (reference: 4×1012–6.5×1012/L) | 40 (24.7) | 15 [7, 44] | |||
<4×1012 | 122 (75.3) | 19 [8, 35] | 1.08 (0.75, 1.55) | 0.414 | 0.679 |
WBC (reference: 4×109–10×109/L) | 69 (42.6) | 22 [9, 43] | |||
<4×109 | 15 (9.3) | 37 [14, 60] | 0.89 (0.51, 1.57) | −0.39 | 0.696 |
>10×109 | 78 (48.1) | 12 [8, 29] | 1.64 (1.17, 2.29) | 2.89 | 0.0039 |
LY (reference: 0.8×109–4×109/L) | 85 (52.5) | 18 [9, 46] | |||
<0.8×109 | 77 (47.5) | 16 [7, 31] | 1.31 (0.96, 1.79) | 1.69 | 0.091 |
NEUT (reference: 2×109–7×109/L) | 59 (36.4) | 30 [14, 60] | |||
<2×109 | 7 (4.3) | 14 [5, 56] | 1.636 (0.74, 3.6) | 1.22 | 0.22 |
>7×109 | 96 (59.3) | 12 [7,28] | 1.91 (1.37, 2.68) | 3.78 | <0.001 |
PLT (reference: 100×109–300×109/L) | 91 (56.2) | 20 [8, 40] | |||
<100×109 | 18 (11.1) | 14 [5, 26] | 1.62 (0.97, 2.71) | 1.856 | 0.064 |
>300×109 | 53 (32.7) | 17 [9, 38] | 1.1 (0.78, 1.55) | 0.549 | 0.583 |
Hb (reference: 120–160 G/L) | 29 (17.9) | 14 [9, 51] | |||
<120 | 133 (82.1) | 17 [8, 36] | 0.16 (0.78, 1.76) | 0.777 | 0.437 |
UA (reference: 208.3–428.4 mmol/L) | 94 (58.0) | 21 [10, 47] | |||
<208.3 | 35 (21.6) | 20 [9, 38] | 1.167 (0.79, 1.72) | 0.766 | 0.444 |
>428.4 | 33 (20.4) | 10 [5, 22] | 1.77 (1.18, 2.65) | 2.78 | 0.005 |
Urea (reference: 2.8–7.6 nmol/L) | 77 (47.5) | 27 [11, 55] | |||
<2.8 | 18 (11.1) | 26 [9, 47] | 1.19 (0.71, 1.99) | 0.656 | 0.512 |
>7.6 | 67 (41.4) | 10 [5, 24] | 1.95 (1.40, 2.74) | 3.905 | <0.001 |
ALT (reference: 0–42 U/L) | 109 (67.3) | 20 [10, 43] | |||
>42 | 53 (32.7) | 12 [5, 33] | 1.353 (0.97, 1.88) | 1.791 | 0.073 |
GOT (reference: 0–40 U/L) | 103 (63.6) | 22 [9, 54] | |||
>40 | 59 (36.4) | 13 [6, 30] | 1.99 (1.41, 2.82) | 3.93 | <0.001 |
Cr (reference: 64–104 mmoI/L) | 33 (20.4) | 17 [8, 30] | |||
<64 | 92 (56.8) | 22 [10, 46] | 0.83 (0.56, 1.24) | −0.9 | 0.363 |
>104 | 37 (22.8) | 10 [5, 27] | 1.43 (0.89, 2.30) | 1.48 | 0.139 |
Tbil (reference: 3–20 mmoI/L) | 118 (72.8) | 21 [9, 42] | |||
<3 | 2 (1.2) | – | 0.60 (0.14, 2.3) | −0.806 | 0.42 |
>20 | 42 (25.9) | 11 [5, 29] | 1.52 (1.06, 2.17) | 2.283 | 0.022 |
PNI (reference: ≥45) | 24 (14.8) | 30 [11, 53] | |||
<45 | 138 (85.2) | 16 [8, 35] | 1.4 (0.9, 2.2) | 1.522 | 0.128 |
NLR (reference: <5) | 39 (24.1) | 30 [14, 67] | |||
>5 | 123 (75.9) | 14 [7, 30] | 1.78 (1.22, 2.59) | 3.007 | 0.0026 |
PLR (reference: <170.5) | 42 (25.9) | 16 [7, 30] | |||
>170.5 | 120 (74.1) | 18 [8, 40] | 0.71 (0.50, 1.02) | −1.856 | 0.065 |
N, the number of patients; HR, hazard ratio; CI, confidence interval; W, Wald value; Na, serum natrium; Ca, serum calcium; K, serum potassium; Cl, serum chloride; ALB, albumin; RBC, red blood cell count; WBC, leukocyte count; LY, lymphocyte count; NEUT, neutrophil count; PLT, platelet count; Hb, hemoglobin; UA, uric acid; ALT, alanine aminotransferase; GOT, glutamic oxalacetic transaminase; Cr, creatinine; TBil, total bilirubin; NLR, neutrophil/lymphocyte; PLR, platelet/lymphocyte; PNI, prognostic nutritional index.
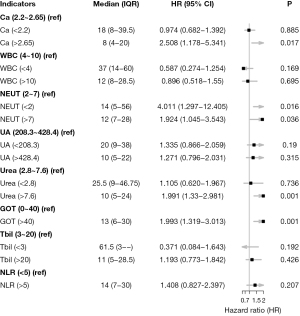
The construction and evaluation of a nomogram
The four prognostic factors (serum calcium, neutrophil count, urea, and glutamic oxalacetic transaminase) were incorporated to establish a predictive model to predict the 30-day mortality of advanced cancer patients (Figure 3). This model was visualized using a nomogram, which transforms the complex regression equation into a visual graph to facilitate ease of reading the prediction model. The basic principle of the nomogram is to build a multi-factor regression model (such as Cox regression), assign scores to each value according to its regression coefficient, and present the score in appropriate proportions. The point line represents the single score of each variable under different values. The use of the model is illustrated with an assumptive patient with serum calcium ≤2.65 mmol/L, neutrophil count of 2×109–7×109/L, urea ≤7.6 nmol/L, and glutamic oxalacetic transaminase ≤40 U/L upon admission. Points for serum calcium, neutrophil count, urea, and glutamic oxalacetic transaminase were all 0. The total points added up to 0 for this patient, which represents approximately 0.65 survival probability of 30 days. The score of each participant was calculated based on the nomogram model, with the best cutoff point being 88 for distinguishing 30-day survival. Patients were divided into 2 risk groups based on the cutoff value of 88, namely, a high-mortality risk group within 30 days (score >88, n=77, 47.5%) and a low-mortality risk group within 30 days (score ≤88, n=85, 52.5%). Log-rank test showed that there was a significant difference between the two sets (P<0.001; Figure 4).
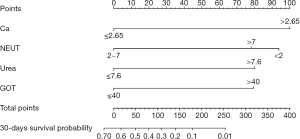
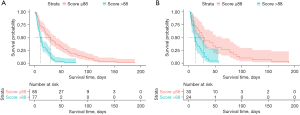
The performance of nomogram was assessed using the C-index, the AUC, and calibration plots. The final model was moderately calibrated with a concordance index of 0.69 (SE =0.022) in the training set and 0.71 (SE =0.032) in the validation set. The AUC of the ROC showed that the accuracy, sensitivity, and specificity were 0.755, 0.596, and 0.812, respectively, in training set, while the accuracy was 0.70 in the validation set (Figure 5), indicating medium discrimination of the model. The calibration plot for OS showed that the calibration curve was close to the diagonal reference line, which is the line indicating good calibration, suggesting an optimal agreement between the prediction by the nomogram and actual observation (Figure 6). The DCA is a statistical method to evaluate whether a model is useful in supporting clinical decisions, which was also applied to evaluate the net benefit from the perspective of clinical consequences. As expected, the DCA yielded a wide range of risk thresholds, at which the clinical net benefits would be obtained by using our nomogram (Figure 7).
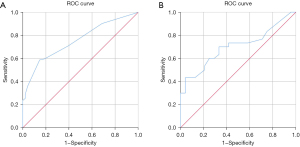
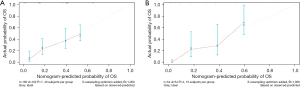
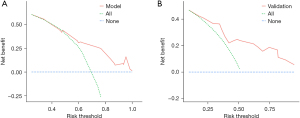
Discussion
Prognosis remains an important part of patient care and the main determinant of clinical decision-making for advantage cancer patients in the terminal stages of the disease (19). Accurate prediction of prognosis will ensure patients avoid futile treatment and minimize the burden of unnecessary hospital visits in patients with a short life expectancy. When validated survival prognosis tools are applied correctly, it may serve to improve the accuracy of prediction and improve patient care (8). Although the nomogram constructed currently should be considered a valid tool, with good calibration and discrimination, it appears to be more applicable in the end-of-life period (days, weeks, months), but not earlier as originally expected. In addition, the nomogram is not suitable for advanced cancer patients who were undergoing or who are planning to receive chemoradiotherapy.
Clinician prediction of survival (CPS), Palliative Performance Scale (PPS) (20), PaP, and the Palliative Prognostic Index (PPI) (21) are the most commonly used prognostic tools at present. The accuracy of CPS, to a large degree, depends on the physician's experience and ability, and it often overestimates the rates of survival by 20–30% (22,23). The PPS and PPI are mainly composed of objective indicator like CPS, performance score, self-care ability, consciousness, oral intake, and presence of signs and symptoms. Some biological parameters like white blood cell, bilirubin, lymphocyte ratio, transaminase, and C-reactive protein were added to formulate prediction models such as PaP, Delirium-PaP (D-PaP) (24), New Chinese prognostic scale (New-ChPs) (25), Prognosis in Palliative care Study (PiPS) (26), Objective Prognostic Score (OPS) (27) and PROgnostic Model for Advanced Cancer (PRO-MAC) (28). Most of them have an acceptable accuracy rate ranging from 52–89%. By comparison, completely objective tools have a relatively higher prediction accuracy. Hamano et al. developed Objective Prognostic Index for advanced cancer (OPI-AC) based on laboratory findings and vital signs alone using a fractional polynomial model (12), with accuracy of 0.77, 0.81, 0.90, 0.90, and 0.92 for the 7-day, 14-day, 30-day, 56-day, and 90-day models, respectively. OPPS (13) was developed by incorporating heart rate, white blood cells, platelets, serum creatinine level, serum potassium level, and history of chemotherapy, with an accuracy of 0.82. Niki et al. (29) developed the WPCBAL model (based on six laboratory test values including white blood cell, platelet, C-reactive protein, blood urea nitrogen, aspartate aminotransferase, and lactase dehydrogenase) and the WPBAL model (C-reactive protein is removed) to predict the mortality within two weeks with high specificity and sensibility. Miyagi et al. (9) formulated a tool solely based on routine blood test data, including total bilirubin, creatinine, urea/creatinine ratio, aspartate aminotransferase, albumin, total leukocyte count, differential lymphocyte count, and PLR, with accuracy of 0.87.
It is generally accepted that deaths occurring within 30 days is an indicator of the quality of cancer care (4). In the present study, we developed and validated a novel easy-to-use nomogram based on blood test data to predict the 30-day survival probability. Only four blood variables were included: serum calcium, urea, neutrophil count, and glutamic oxalacetic transaminase, which are simple to calculate and easily obtained from routine blood tests. The model was tested regarding calibration and discrimination. The calibration plot for the OS showed an optimal agreement between the prediction by the nomogram and actual observation. The C-index of 0.69 and the AUC at 30 days (0.76) are at a moderate level, which does not seem so ideal compared with other prognostic tools. This may be caused by the small number of subjects included in training set. However, important properties for prognostic tools to be user-friendly include convenience and simplicity (30). Many prognostic tools with higher accuracy are rarely used in practice due to multiple complex variables and the time required to calculate scores. Our nomogram model was constructed with 4 simple and accessible variables. Rather than using continuous variable, they were transformed into categorical variables, which is convenient for scoring. The validation set presented a satisfactory calibration and discrimination of 0.71, which ensured reliable performance in practice.
Our study demonstrated that high serum calcium was related with shorter OS. High levels of serum calcium, such as hypercalcemia of malignancy, is most commonly mediated by tumoral production of parathyroid hormone-related protein or by cytokines activating osteoclast degradation of bone (31). Meanwhile, hypercalcemia is the presenting sign of cancer. Many of the pathways regulated by Ca2+ are of significance to the development and spread of several forms of cancer (32). For example, the association between higher serum calcium and ovarian cancer was confirmed in some prospective studies (33,34). Furthermore, the model revealed that serum calcium concentration was associated with lower odds of longevity (35). The results herein showed that low level and high level of neutrophil count were both related to poor prognosis. Neutrophil count is an important indicator in the Systemic Immune-Inflammation Index (SII) (36), which reflects the local immune response and systemic inflammation. Inflammation has been regarded as a trigger of cancer and a necessity for the maintenance of the cancer status (37), which is associated with a majority of cancer symptoms and survival (38). Expression of high levels of neutrophils has been shown to lead to detrimental outcomes (39). Patients with low neutrophil counts have low immunity and poor prognosis. Neutrophils are crucial for the human innate immunity, and it serves as a measure for the immune system’s functionality (40). Low neutrophil counts might be caused by disease or anticancer treatment, which in turn increases the patient’s risk of infections. It has also been demonstrated that low preoperative neutrophil/lymphocyte (NLR) values have a negative prognostic impact on survival and recurrence to primary oral squamous cell carcinomas, similarly to high ratios (41). It has been suggested that urea is grade A evidence for prediction, while other parameters like some transaminases are grade B indicators (11,42). Transaminases have been shown to have prognostic value in advanced cancer patients. (43) Our results confirmed that glutamic oxalacetic transaminase is an independent predictor of survival, which was consistent with previous findings (12,25,44). Elevations of serum glutamic oxalacetic transaminase may relate to liver parenchymal damage, due to hepatotoxic effects of early chemotherapy agents and liver metastasis. The level of serum urea could strongly response the renal function, which was shown to be significantly correlated with death within 1–2 weeks, both in prospective and retrospective research (43,45). The raised serum urea concentrations, to a great degree, are leaded by decreased glomerular filtration rate, due to renal dysfunction as progressive disease malignancy.
The DCA showed that the nomogram was good within most of the reasonable threshold probability range, indicating that the feasibility of the model would bring net benefit in clinical practice. It is common for advanced cancer patients and physicians to make decisions to furthest improve quality of life. Many measures such as hydration, parenteral/enteral nutrition, and anticancer treatments are dependent on survival. Thus, an accurate prognostic assessment is essential to guarantee that patients with different death-risk status make the best decisions to minimize the risk of overtreatment or undertreatment.
Many studies have demonstrated that malnutrition is a significant independent predictor of OS in patients with advanced cancer (46-48). Prognostic nutritional index (PNI) is an important indicator of nutritional status and systemic immune competence, and has attracted attention as a prognostic biomarker (49). A plethora of research has demonstrated that PNI is associated with adverse prognosis (50), worse progression-free survival, and poor OS (51). However, as far as we know, there is no survival prediction tool for advanced cancer patients that included PNI as a predictive item. Thus, we measured the PNI value and expected to incorporate PNI into our model. Unfortunately, there was no significant relationship between PNI score and survival, as shown in a previous study (52). In addition, vital signs like heart rate (53) and its variability (53), handgrip strength (HGS) (54), systolic pressure, and phase angle (55), were all demonstrated to be associated with survival. Further research to include these objective indicators into the survival prediction model is warranted in the future.
Limitations
There were certain limitations in our study. First, this is a retrospective study, which was performed in a single institution with only a small group enrolled into the analysis. Future prospective, multicenter, and large sample studies are warranted. Meanwhile, the subjects who were selected into this study were those who had complete blood tests data and survival time, which may lead to selective bias. It unclear whether there was a difference in performance conditions between patients who received blood tests and those who did not. In addition, the collecting of laboratory data was an invasive procedure. Not every patient had these data nor accepted invasive operations due to their frail condition. Furthermore, the applicability and the feasibility of our model to outpatients and nursing home residents requires further validation.
Conclusions
A simple and objective prognostic nomogram model predicting the 30-day survival of patients with advanced cancer was developed and validated in present study, with adequate calibration and discrimination properties. Our model is characterized by simplicity, objectivity, and ease of use, which is expected to aid clinical prognostication and facilitate individualized evaluation of advanced cancer. Further validation warranted in future prospective, multicenter ,and large sample studies.
Acknowledgments
The authors are grateful to the participating patients and to all other co-investigators who contributed to this study.
Funding: This study was funded by the Science and Technology Innovation Plan (No. 2021SK51120) and Scientific Research Project of Health Commission of Hunan Province (No. B202314057983). The sponsor played no role in the study design; collection, analysis, and interpretation of the data; writing of the report; nor the decision to submit the article for publication.
Footnote
Reporting Checklist: The authors have completed the TRIPOD reporting checklist. Available at https://apm.amegroups.com/article/view/10.21037/apm-22-1058/rc
Data Sharing Statement: available at https://apm.amegroups.com/article/view/10.21037/apm-22-1058/dss
Peer Review File: available at https://apm.amegroups.com/article/view/10.21037/apm-22-1058/prf
Conflicts of Interest: All authors have completed the ICMJE uniform disclosure form (available at https://apm.amegroups.com/article/view/10.21037/apm-22-1058/coif). The authors reported the funding from the Science and Technology Innovation Plan (No. 2021SK51120) and Scientific Research Project of Health Commission of Hunan Province (No. B202314057983).
Ethical Statement: The authors are accountable for all aspects of the work in ensuring that questions related to the accuracy or integrity of any part of the work are appropriately investigated and resolved. This study was conducted in accordance with the Declaration of Helsinki (as revised in 2013) and was approved by the Hunan Cancer Hospital Research Ethics Committee (No. 12034209). Individual consent for this retrospective analysis was waived.
Open Access Statement: This is an Open Access article distributed in accordance with the Creative Commons Attribution-NonCommercial-NoDerivs 4.0 International License (CC BY-NC-ND 4.0), which permits the non-commercial replication and distribution of the article with the strict proviso that no changes or edits are made and the original work is properly cited (including links to both the formal publication through the relevant DOI and the license). See: https://creativecommons.org/licenses/by-nc-nd/4.0/.
References
- Sung H, Ferlay J, Siegel RL, et al. Global Cancer Statistics 2020: GLOBOCAN Estimates of Incidence and Mortality Worldwide for 36 Cancers in 185 Countries. CA Cancer J Clin 2021;71:209-49. [Crossref] [PubMed]
- Newland JA. Do not resuscitate. Nurse Pract 2015;40:12. [Crossref] [PubMed]
- Pobar I, Job M, Holt T, et al. Prognostic tools for survival prediction in advanced cancer patients: A systematic review. J Med Imaging Radiat Oncol 2021;65:806-16. [Crossref] [PubMed]
- Feng C, Yu H, Lei H, et al. A prognostic model using the neutrophil-albumin ratio and PG-SGA to predict overall survival in advanced palliative lung cancer. BMC Palliat Care 2022;21:81. [Crossref] [PubMed]
- Scarpi E, Nanni O, Maltoni M. Development and Validation of the PaP Score Nomogram for Terminally Ill Cancer Patients. Cancers (Basel) 2022;14:2510. [Crossref] [PubMed]
- Lee SH, Lee JG, Choi YJ, et al. Prognosis palliative care study, palliative prognostic index, palliative prognostic score and objective prognostic score in advanced cancer: a prospective comparison. BMJ Support Palliat Care 2021;bmjspcare-2021-003077.
- Maltoni M, Caraceni A, Brunelli C, et al. Prognostic factors in advanced cancer patients: evidence-based clinical recommendations--a study by the Steering Committee of the European Association for Palliative Care. J Clin Oncol 2005;23:6240-8. [Crossref] [PubMed]
- Simmons CPL, McMillan DC, McWilliams K, et al. Prognostic Tools in Patients With Advanced Cancer: A Systematic Review. J Pain Symptom Manage 2017;53:962-970.e10. [Crossref] [PubMed]
- Miyagi T, Miyata S, Tagami K, et al. Prognostic model for patients with advanced cancer using a combination of routine blood test values. Support Care Cancer 2021;29:4431-7. [Crossref] [PubMed]
- Hui D, Park M, Liu D, et al. Clinician prediction of survival versus the Palliative Prognostic Score: Which approach is more accurate? Eur J Cancer 2016;64:89-95. [Crossref] [PubMed]
- Reid VL, McDonald R, Nwosu AC, et al. A systematically structured review of biomarkers of dying in cancer patients in the last months of life; An exploration of the biology of dying. PLoS One 2017;12:e0175123. [Crossref] [PubMed]
- Hamano J, Takeuchi A, Yamaguchi T, et al. A combination of routine laboratory findings and vital signs can predict survival of advanced cancer patients without physician evaluation: a fractional polynomial model. Eur J Cancer 2018;105:50-60. [Crossref] [PubMed]
- Chen YT, Ho CT, Hsu HS, et al. Objective palliative prognostic score among patients with advanced cancer. J Pain Symptom Manage 2015;49:690-6. [Crossref] [PubMed]
- Demirelli B, Babacan NA, Ercelep Ö, et al. Modified Glasgow Prognostic Score, Prognostic Nutritional Index and ECOG Performance Score Predicts Survival Better than Sarcopenia, Cachexia and Some Inflammatory Indices in Metastatic Gastric Cancer. Nutr Cancer 2021;73:230-8. [Crossref] [PubMed]
- Deng GC, Lv Y, Yan H, et al. Nomogram to predict survival of patients with advanced and metastatic pancreatic Cancer. BMC Cancer 2021;21:1227. [Crossref] [PubMed]
- Smeijers S, Depreitere B. Prognostic scores for survival as decisional support for surgery in spinal metastases: a performance assessment systematic review. Eur Spine J 2021;30:2800-24. [Crossref] [PubMed]
- Gupta V, Coburn N, Kidane B, et al. Survival prediction tools for esophageal and gastroesophageal junction cancer: A systematic review. J Thorac Cardiovasc Surg 2018;156:847-56. [Crossref] [PubMed]
- Van Calster B, Wynants L, Verbeek JFM, et al. Reporting and Interpreting Decision Curve Analysis: A Guide for Investigators. Eur Urol 2018;74:796-804. [Crossref] [PubMed]
- Hui D. Prognostication of Survival in Patients With Advanced Cancer: Predicting the Unpredictable? Cancer Control 2015;22:489-97. [Crossref] [PubMed]
- Dzierżanowski T, Gradalski T, Kozlowski M. Palliative Performance Scale: cross cultural adaptation and psychometric validation for Polish hospice setting. BMC Palliat Care 2020;19:52. [Crossref] [PubMed]
- Morita T, Tsunoda J, Inoue S, et al. The Palliative Prognostic Index: a scoring system for survival prediction of terminally ill cancer patients. Support Care Cancer 1999;7:128-33. [Crossref] [PubMed]
- Cheon S, Agarwal A, Popovic M, et al. The accuracy of clinicians' predictions of survival in advanced cancer: a review. Ann Palliat Med 2016;5:22-9. [PubMed]
- Farinholt P, Park M, Guo Y, et al. A Comparison of the Accuracy of Clinician Prediction of Survival Versus the Palliative Prognostic Index. J Pain Symptom Manage 2018;55:792-7. [Crossref] [PubMed]
- Tarumi Y, Watanabe SM, Lau F, et al. Evaluation of the Palliative Prognostic Score (PaP) and routinely collected clinical data in prognostication of survival for patients referred to a palliative care consultation service in an acute care hospital. J Pain Symptom Manage 2011;42:419-31. [Crossref] [PubMed]
- Cui J, Zhou L, Wee B, et al. Predicting survival time in noncurative patients with advanced cancer: a prospective study in China. J Palliat Med 2014;17:545-52. [Crossref] [PubMed]
- Gwilliam B, Keeley V, Todd C, et al. Development of Prognosis in Palliative care Study (PiPS) predictor models to improve prognostication in advanced cancer: prospective cohort study. BMJ Support Palliat Care 2015;5:390-8. [Crossref] [PubMed]
- Suh SY, Choi YS, Shim JY, et al. Construction of a new, objective prognostic score for terminally ill cancer patients: a multicenter study. Support Care Cancer 2010;18:151-7. [Crossref] [PubMed]
- Hum A, Wong YKY, Yee CM, et al. PROgnostic Model for Advanced Cancer (PRO-MAC). BMJ Support Palliat Care 2020;10:e34. [Crossref] [PubMed]
- Niki K, Okamoto Y, Tabata Y, et al. A New Approach for Determining Short-Term, Objective Prognostic Predictive Methods for Terminal Cancer Patients Based on the Change Point of Laboratory Test Values. J Palliat Med 2018;21:529-32. [Crossref] [PubMed]
- Paiva CE, Paiva BSR, de Paula Pântano N, et al. Development and validation of a prognostic nomogram for ambulatory patients with advanced cancer. Cancer Med 2018;7:3003-10. [Crossref] [PubMed]
- Karki S, Galiveeti S, Leung V. Hypercalcemia and Renal Mass: A Diagnostically Challenging Case. Cureus 2021;13:e16718. [Crossref] [PubMed]
- O'Grady S, Morgan MP. Calcium transport and signalling in breast cancer: Functional and prognostic significance. Semin Cancer Biol 2021;72:19-26. [Crossref] [PubMed]
- Schwartz GG, Tretli S, Vos L, et al. Prediagnostic serum calcium and albumin and ovarian cancer: A nested case-control study in the Norwegian Janus Serum Bank Cohort. Cancer Epidemiol 2017;49:225-30. [Crossref] [PubMed]
- Schwartz GG, Tretli S, Klug MG, et al. Women who develop ovarian cancer show an increase in serum calcium and a decrease in serum albumin. A longitudinal study in the Janus Serum Bank Cohort. Gynecol Oncol 2020;159:264-9. [Crossref] [PubMed]
- Yuan S, Baron JA, Michaëlsson K, et al. Serum calcium and 25-hydroxyvitamin D in relation to longevity, cardiovascular disease and cancer: a Mendelian randomization study. NPJ Genom Med 2021;6:86. [Crossref] [PubMed]
- Huang H, Liu Q, Zhu L, et al. Prognostic Value of Preoperative Systemic Immune-Inflammation Index in Patients with Cervical Cancer. Sci Rep 2019;9:3284. [Crossref] [PubMed]
- Laird BJ, McMillan DC, Fayers P, et al. The systemic inflammatory response and its relationship to pain and other symptoms in advanced cancer. Oncologist 2013;18:1050-5. [Crossref] [PubMed]
- Dupré A, Malik HZ. Inflammation and cancer: What a surgical oncologist should know. Eur J Surg Oncol 2018;44:566-70. [Crossref] [PubMed]
- Ocana A, Nieto-Jiménez C, Pandiella A, et al. Neutrophils in cancer: prognostic role and therapeutic strategies. Mol Cancer 2017;16:137. [Crossref] [PubMed]
- Thunström Salzer A, Niemiec MJ, Hosseinzadeh A, et al. Assessment of Neutrophil Chemotaxis Upon G-CSF Treatment of Healthy Stem Cell Donors and in Allogeneic Transplant Recipients. Front Immunol 2018;9:1968. [Crossref] [PubMed]
- Mattavelli D, Lombardi D, Missale F, et al. Prognostic Nomograms in Oral Squamous Cell Carcinoma: The Negative Impact of Low Neutrophil to Lymphocyte Ratio. Front Oncol 2019;9:339. [Crossref] [PubMed]
- Dolan RD, McSorley ST, Horgan PG, et al. The role of the systemic inflammatory response in predicting outcomes in patients with advanced inoperable cancer: Systematic review and meta-analysis. Crit Rev Oncol Hematol 2017;116:134-46. [Crossref] [PubMed]
- Okugawa Y, Shirai Y, Nodono H, et al. Objective Predictive Score as a Feasible Biomarker for Short-term Survival in TerminalIy Ill Patients with Cancer. Anticancer Res 2017;37:267-75. [Crossref] [PubMed]
- Li T, Copeland C, Le A. Glutamine Metabolism in Cancer. Adv Exp Med Biol 2021;1311:17-38. [Crossref] [PubMed]
- Durand JP, Mir O, Coriat R, et al. Validation of the Cochin Risk Index Score (CRIS) for life expectancy prediction in terminally ill cancer patients. Support Care Cancer 2012;20:857-64. [Crossref] [PubMed]
- Tan CS, Read JA, Phan VH, et al. The relationship between nutritional status, inflammatory markers and survival in patients with advanced cancer: a prospective cohort study. Support Care Cancer 2015;23:385-91. [Crossref] [PubMed]
- Triarico S, Rinninella E, Cintoni M, et al. Impact of malnutrition on survival and infections among pediatric patients with cancer: a retrospective study. Eur Rev Med Pharmacol Sci 2019;23:1165-75. [PubMed]
- Bullock AF, Greenley SL, McKenzie GAG, et al. Relationship between markers of malnutrition and clinical outcomes in older adults with cancer: systematic review, narrative synthesis and meta-analysis. Eur J Clin Nutr 2020;74:1519-35. [Crossref] [PubMed]
- Okadome K, Baba Y, Yagi T, et al. Prognostic Nutritional Index, Tumor-infiltrating Lymphocytes, and Prognosis in Patients with Esophageal Cancer. Ann Surg 2020;271:693-700. [Crossref] [PubMed]
- Luan C, Wang F, Wei N, et al. Prognostic nutritional index and the prognosis of diffuse large b-cell lymphoma: a meta-analysis. Cancer Cell Int 2020;20:455. [Crossref] [PubMed]
- Johannet P, Sawyers A, Qian Y, et al. Baseline prognostic nutritional index and changes in pretreatment body mass index associate with immunotherapy response in patients with advanced cancer. J Immunother Cancer 2020;8:e001674. [Crossref] [PubMed]
- Namikawa T, Maeda M, Yokota K, et al. Assessment of Systemic Inflammatory Response and Nutritional Markers in Patients With Trastuzumab-treated Unresectable Advanced Gastric Cancer. In Vivo 2020;34:2851-7. [Crossref] [PubMed]
- Mori I, Maeda I, Morita T, et al. Association Between Heart Rate and Reversibility of the Symptom, Refractoriness to Palliative Treatment, and Survival in Dyspneic Cancer Patients. J Pain Symptom Manage 2020;60:87-93. [Crossref] [PubMed]
- Kilgour RD, Vigano A, Trutschnigg B, et al. Handgrip strength predicts survival and is associated with markers of clinical and functional outcomes in advanced cancer patients. Support Care Cancer 2013;21:3261-70. [Crossref] [PubMed]
- Hui D, Dev R, Pimental L, et al. Association Between Multi-frequency Phase Angle and Survival in Patients With Advanced Cancer. J Pain Symptom Manage 2017;53:571-7. [Crossref] [PubMed]