Association between body mass index, missing data, and mortality risk among critically ill patients: the role of missing-data imputation
Introduction
Epidemiological research that uses database or medical records generated by clinicians to analyse relationships between outcomes plays a critical role in clinical practice (1,2). One important limitation of an epidemiological study design is the occurrence of missing data owing to various reasons during the data collection process (3). In such cases, most researchers ignore the missing data and perform a “complete-case analysis” (4,5), which can be a significant bias that weakens the results or conclusions of the research (6). Therefore, how to process missing data can be a critical issue in modern epidemiological research.
Body mass index (BMI, in kg/m2) is a basic physical characteristic used in almost all health-related research and is associated with important health-related outcomes such as mortality (7). Particularly, a lower BMI is associated with malnutrition (8), which has been linked to increased hospital mortality in critically ill patients who are admitted to the intensive care unit (ICU) (9). However, because accurate measurements of height and weight are necessary at the time of study to calculate BMI, many epidemiological types of research have missing data on BMI, which can affect the outcome (10-12). For example, when a patient was admitted to the ICU and required rapid resuscitation, BMI measurements might have been delayed and influenced by the rapid fluid resuscitation. Additionally, data might be missing owing to the human error of the nurse who recorded the height or weight of the patients admitted to the ICU. Therefore, multiple imputation for missing data is recommended to overcome such limitation (13); however, there is insufficient research on this topic.
This study investigated the association between BMI at the time of ICU admission and 90-day mortality risk of critically ill patients in complete-case datasets that ignores missing data, as well as in datasets with multiple imputation for missing data. The length of ICU and hospital stay of both datasets were also investigated.
We present the following article in accordance with the STROBE reporting checklist (available at http://dx.doi.org/10.21037/apm-20-203).
Methods
Design and ethical statement
This retrospective cohort study was approved by the Institutional Review Board (IRB) of Seoul National University Bundang Hospital (SNUBH) (IRB approval number: B-1806/474-105). Because of the retrospective design of the study, the requirement for informed consent was waived by the IRB. The study also conformed to the provisions of the Declaration of Helsinki (as revised in 2013).
Patients
This study included the medical records of patients aged ≥18 years who were admitted to the ICU at a single tertiary care academic hospital between January 2012 and December 2017. All ICU admission cases during the period were screened by medical record technicians who were blinded to the study objective. If one patient was admitted to the ICU more than twice during the period, only the last ICU admission case that would have been the most severe was included. Cases of patients aged <18 years were excluded, as well as cases with missing data other than BMI.
Data source
This study used data archived in the Bundang Hospital Electronic System for Total Care (BESTCare), an electronic medical record system of SNUBH (14). Additionally, the dates of death of all patients as of 16 May, 2018, including those lost to follow-up, were collected from the Statistics Korea database (http://kostat.go.kr/portal/eng). As a central government organization for statistics, Statistics Korea provides services related to overall planning and coordination of national statistics.
BMI at ICU admission (independent variable)
During the study period, the ICU of SNUBH used beds that can measure patient’s weight, which was generally assessed more than once per day. Unlike weight, height was directly measured if the patient could stand, or information provided by the patient was recorded if the patient could not stand. We used the weight or height recorded within 24 hours of ICU admission to calculate BMI at ICU admission. Therefore, if there was no record of BMI within 24 hours of ICU admission, it was considered missing data. Errors in BMI records were also considered missing data. There was a two-step process in identifying errors in BMI values. First, we screened patients with BMI values <2.5th or >97.5th percentile as outliers in the distribution of BMI data at ICU admission (15). Then, considering there might be extreme cases such as morbidly obese, severe cachexia, or scoliosis patients, we calculated the mean BMI values with standard deviation (SD) during the hospitalization period of patients who were screened by these outlier cut-off values (<2.5th or >97.5th percentile). If patients had a BMI value at ICU admission within 3 SD from the mean value of BMI during hospitalization, it was not considered as an error. For analysis, the BMI data were classified into four groups according to the Garrow classification (16): underweight (<20 kg/m2), normal (20.0–24.9 kg/m2), overweight (25–29.9 kg/m2), and obese (≥30 kg/m2).
90-day mortality and length of hospital and ICU stay (outcome variables)
The 90-day mortality was defined as patient death within 90 days of ICU admission. The length of ICU stay (days) was the number of days from the date of ICU admission to the date of ICU discharge or ICU death. The length of hospital stay (days) was calculated from the date of ICU admission to the date of hospital discharge or hospital death.
Potential covariates
The following potential covariates were considered associated with patient outcomes or BMI at ICU admission: (I) physical characteristics (sex and age); (II) Acute Physiology and Chronic Health Evaluation (APACHE) II; (III) socioeconomic status (SES)-related information (highest educational attainment (lower than high school/more than or equal to high school, lower than college/more than or equal to college), occupation (office worker, licensed job, house work, self-employed, student, military, or labourer/unemployed), marital status (never married, married or living with someone, divorced or separated, widowed), type of insurance (medical aid beneficiary program, national health insurance program); (IV) comorbidities at ICU admission (hypertension, diabetes mellitus, ischemic heart disease, cerebrovascular disease, chronic obstructive lung disease, liver disease, anaemia, cancer, chronic kidney disease, hyperlipidaemia); (V) admission through the emergency department; and (VI) type of admission department (internal medicine, neurologic centre, cardiothoracic surgical department, other surgical department). Comorbidity-related information was collected from the electronic medical record system based on the International Classification of Disease-10 codes. The patients in the medical aid beneficiary program are those who are classified as having low income, and most of their hospital charges are paid by the government. Moreover, for patients in the national health insurance program, approximately two-thirds of their hospital charges are covered by the government.
Study endpoints
The primary endpoint was to investigate the association between BMI and 90-day mortality risk in the complete-case group and multiple imputation group. The secondary endpoints consisted of determining how the length of ICU or hospital stay and BMI were associated with each group.
Statistical analysis
First, t-tests for continuous variables and chi-square tests for categorical variables were used to determine if there was a difference in each variable depending on whether BMI was missing or not.
Then, Little’s test was performed to determine the mechanism of missing BMI data. The result of Little’s test was P<0.001 at a significance level of 5%; thus, missing BMI values were not missing completely at random (MCAR) (17). We considered as many variables as possible that may be related to BMI to assume missing at random (MAR) in this study. To replace the missing BMI values, imputations were performed 10 times to generate 10 datasets with the fully conditional specification method by following the PROC MI procedures. All covariates (all physical characteristics, APACHE II, all SES-related information, all comorbidities, admission through emergency department or type of admission, and year of ICU admission) related to BMI or BMI missingness were included in the imputation model to improve accuracy in generating replacements of missing BMI values. Additionally, outcome variables such as survival time and length of ICU and hospital stay were included in the imputation model, considering that shorter stay in ICU or survival time might affect the missingness of BMI values. Among outcome variables, survival time was included in the imputation model after log transformation, whereas the length of hospital and ICU stay were included in the imputation model after log-log transformation (18). Using plots, we confirmed that the relationship between continuous BMI values and continuous variables (i.e., age, APACHE II, log survival time, and log-log length of ICU and hospital stay) was relatively linear. Finally, our imputation model for continuous BMI values is a linear regression on all covariates, log survival time, and log-log length of ICU and hospital stay. Then, for each of the 10 imputed datasets, we performed 10 analyses in each of the separate datasets. The relationship between the log relative hazard of 90-day mortality and BMI (a continuous variable) was presented through a restricted cubic spline (RCS) to identify linearity between BMI and 90-day mortality before and after missing data imputation. RCS in only one imputed dataset was provided as a result because all 10 RCSs in the 10 imputed datasets showed similar patterns. We performed univariable and multivariable Cox regression analyses to analyse the primary endpoint. All covariates (all physical characteristics, APACHE II, all SES-related information, all comorbidities, admission through emergency department, and type of admission) were included in the multivariable Cox regression model for adjustment. This Cox regression model was confirmed to satisfy the central assumption of the Cox proportional hazards model through the log-minus-log plot.
To analyse the secondary endpoint, we used multivariable generalized linear regression analysis. In this model, the length of ICU and hospital stay, a dependent variable, did not follow normality (Kolmogorov-Smirnov test: P<0.05); thus, the Poisson distribution was assumed, and the log link function was used. Results of the Cox regression analysis were presented as hazard ratios (HRs) with 95% confidence intervals (CIs), whereas results of the generalized linear regression analysis were presented as exponentiated regression (Exp.) coefficients with 95% CIs. In all models, the normal group (20–25 kg/m2) was set as the control group for the BMI group analysis. The results from each analysed dataset were combined using Rubin’s rules.
The rms package of R was used for RCS (version 3.3.2 with R packages), and SAS software version 9.4 (SAS Institute Inc., Cary, NC, USA) was used for all other analyses, including multiple imputation for missing values of BMI at ICU admission. For all analyses, P<0.05 was considered statistically significant.
Results
Between January 2012 and December 2017, a total of 30,398 patients were admitted to the ICU 40,533 times. Among the admission cases, 10,135 were excluded, and only the episodes of the last ICU admissions were included. Among the 30,398 patients, 5,440 patients aged <18 years were excluded, as well as 30 patients with missing or incomplete data other than BMI. Finally, 24,928 patients were examined, among whom 5916 (23.7%) were in the BMI missing group and 19,012 (76.3%) were in the complete-case group (Figure 1). In the BMI missing group, 2,987 patients had missing data on weight at ICU admission, 3,199 had missing data on height, and 270 had missing data on both height and weight.
BMI missing group versus complete-case group
Results of comparing the characteristics between the BMI missing group and complete-case group at ICU admission are shown in Table 1. Most characteristics showed a difference between both groups. The 90-day mortality in the BMI missing group was 1,355/5,916 (22.9%), which was significantly higher than 1,840/19,012 (9.7%) in the complete-case group (P<0.001). Moreover, the mean (SD) length of hospital stay in the BMI missing group was 8.3 days (15.5), which was significantly shorter than 12.2 days (20.6) in the complete-case group (P<0.001).
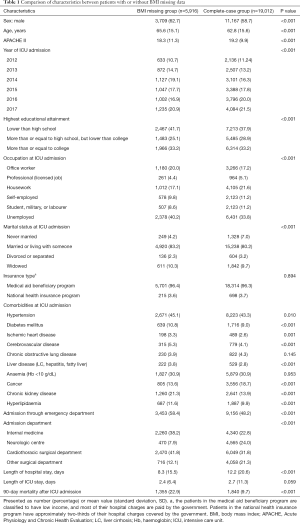
Full table
BMI distribution before and after multiple imputation
Table 2 shows the BMI distribution of the complete-case group (n=19,012; before multiple imputation) and multiple imputation group (n=24,928; after multiple imputation). The mean BMI (SD) of the complete-case group was 23.8 (3.8) and that of the multiple imputation group was 23.7 (4.4). In terms of subgroup distribution, the complete-case group had underweight (14.9%), normal (50.3%), overweight (29.5%), and obese (5.2%) patients. The multiple imputation group also had underweight (15.5%), normal (49.2%), overweight (30.0%), and obese (5.3%) patients. Figure S1 shows these distributions in graphs.
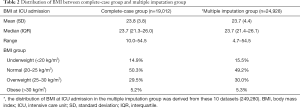
Full table
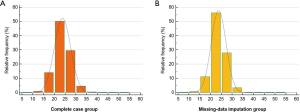
90-day mortality before and after missing-data imputation
Figure 2 shows the log relative hazard of 90-day mortality based on BMI in the complete-case group (A; before missing-data imputation) and multiple imputation group in one imputed dataset (B; after missing-data imputation). Compared with BMI in the complete-case group, BMI after missing-data imputation showed a more gradual and linear pattern between BMI and 90-day mortality. Table 3 shows the results of the univariable and multivariable Cox regression analyses for 90-day mortality in both groups. While the 90-day mortality risk of underweight patients in the complete-case group increased by 1.49 times (HR: 1.49, 95% CI: 1.34–1.66; P<0.001), the 90-day mortality risk of underweight patients in the multiple imputation group increased by 1.36 times (HR: 1.36, 95% CI: 1.24–1.49; P<0.001) compared with that of normal BMI patients. The 90-day mortality risk of overweight patients decreased in both the complete-case group (HR: 0.82, 95% CI: 0.72–0.94; P=0.003) and multiple imputation group (HR: 0.81, 95% CI: 0.72–0.90; P<0.001) compared with that of normal BMI patients. The 90-day mortality risk of overweight patients compared with that of normal BMI patients was not significantly different in either the complete-case group (P=0.246) or the multiple imputation group (P=0.292).
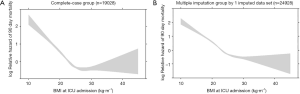
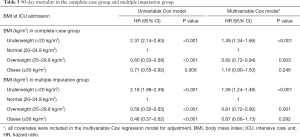
Full table
Length of hospital and ICU stay before and after missing-data imputation
Table 4 shows the results of the generalized linear regression analysis for the length of ICU and hospital stay according to BMI in the complete-case group and multiple imputation group. The length of ICU stay of underweight patients in the complete-case group and multiple imputation group increased by 44% (Exp. coefficient: 1.44, 95% CI: 1.41–1.47; P<0.001) and 45% (Exp. coefficient: 1.45, 95% CI: 1.38–1.52; P<0.001), respectively. The length of ICU stay of overweight patients in the complete-case group and multiple imputation group decreased by 22% (Exp. coefficient: 0.78, 95% CI: 0.76–0.80; P<0.001) and 26% (Exp. coefficient: 0.74, 95% CI: 0.71–0.76; P<0.001), respectively. The length of ICU stay of obese patients in the complete-case group and multiple imputation group decreased by 6% (Exp. coefficient: 0.94, 95% CI: 0.91–0.98; P=0.006) and 21% (Exp. coefficient: 0.79, 95% CI: 0.75–0.84; P<0.001), respectively.
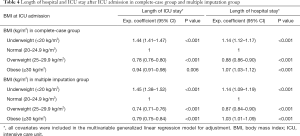
Full table
The length of hospital stay of underweight patients in the complete-case group and multiple imputation group increased by 14% (Exp. coefficient: 1.14, 95% CI: 1.12–1.17; P<0.001) and 14% (Exp. coefficient: 1.14, 95% CI: 1.09–1.19; P<0.001), respectively. The length of hospital stay of overweight patients in the complete-case group and multiple imputation group decreased by 12% (Exp. coefficient: 0.88, 95% CI: 0.86–0.90; P<0.001) and 13% (Exp. coefficient: 0.87, 95% CI: 0.84–0.90; P<0.001), respectively, whereas that of obese patients increased by 7% (Exp. coefficient: 1.07, 95% CI: 1.03–1.12; P<0.001) and 3% (Exp. coefficient: 1.03, 95% CI: 1.01–1.09; P<0.001), respectively.
Discussion
This study showed that the association of BMI at ICU admission with 90-day mortality might be different according to the statistical handling of missing data, such as multiple imputation. This tendency was similar for the length of ICU or hospital stay. Moreover, results of the Cox regression model suggested that the complete-case analysis, ignoring missing BMI data, may have led to a slight overestimation of the 90-day mortality risk in the underweight group (<20 kg/m2) compared with that in the normal group (20–24.9 kg/m2). However, in terms of the length of ICU and hospital stay, our findings suggested the potential underestimation of the effect of BMI in the overweight group (25–29.9 kg/m2) and obese group (≥30 kg/m2) compared with that in the normal group (20–24.9 kg/m2). Above all, the present study suggested that replacing missing BMI values by multiple imputation modelling could be an acceptable procedure to reduce bias.
The most important issue in this study was the missing mechanism of BMI at ICU admission because it was closely related to the effect of missing BMI values on outcomes such as mortality or length of ICU and hospital stay. In Table 1, many differences were noted between the complete-case group and missing BMI group, indicating that an analysis of just the complete-case group could cause a significant bias (19). In detail, the distribution of BMI before and after multiple imputation modelling showed that the proportions of the underweight, overweight, and obese groups were slightly increased after multiple imputation modelling, compared with the complete-case group. In general, both underweight and obese critically ill patients had a higher risk of clinical outcomes after ICU admission (20,21), and our data suggested that the missing BMI value at ICU admission occurred more in underweight or obese patients.
A recent study by Sharafoddini et al. reported that the presence or absence of laboratory data could be a potential predictor for in-hospital and 30-day mortality in critically ill patients after ICU admission (22). The results of this study were similar to those of our study with respect to the clinical importance of missing data in critically ill patients, and missing data could result in a biased prediction of mortality in critically ill patients. Another study by Engerström et al. also suggested that missing data could affect the performance of the Simplified Acute Physiology Score (SAPS)-3 mortality prediction model in Swedish Intensive Care Registry (23). In addition to these previous findings (22,23), our study focused on missing BMI data at ICU admission, and we found that missing BMI data could affect the prediction of outcomes in critically ill patients.
Our study result of increased mortality in the multiple imputation group compared with that in the complete-case group is consistent with the results of an epidemiological study based on the recent Scottish National Diabetes Register (11). This implies that more patients with severe conditions were distributed in the multiple imputation group. Meanwhile, the mean value of the APACHE II scores was lower in the BMI missing group than in the complete-case group, and the length of hospital stay was also shorter in the BMI missing group than in the complete-case group in this study. Comprehensively, this suggests that multiple imputation group might have included many patients of relatively very high or very low severity. Hence, relatively healthier patients who were transferred from the ICU to the wards might have had missing BMI data owing to short admission duration. In contrast, BMI data might have been missing in patients who died soon after ICU admission or in severely ill patients for whom the medical staff could not afford the time to measure and record BMI.
Our institution recently reported that the lower the BMI, the greater the risk of postoperative mortality and mortality after ICU admission (24,25). However, those studies performed a complete-case analysis that ignored missing BMI values. Consequently, as BMI decreased, mortality increased rapidly in RCS in previous studies. However, in the present study, the complete-case group had RCS that depicted a slightly steeper slope with a lower BMI compared with RCS in the multiple imputation group, as shown in Figure 2. This difference in RCSs shows that complete-case analysis may produce an overestimated risk of the mortality of underweight patients in the ICU.
This study has some limitations. First, because this was a retrospective cohort study, there were many confounders that needed to be controlled by multivariable adjustment. Second, it was conducted at a single centre, which limits the generalisability of its findings. For example, as a characteristic of the Korean population, the proportion of morbidly obese (BMI >40 kg/m2) patients is relatively low at 0.1% compared with 5.4% reported in studies of other regions (26). Third, some data on height may have been inaccurate in patients who could not stand to measure height. In these patients, height at ICU admission was recorded by self-report by patients. Because self-report by patients is known to be biased (27), it might be a limitation in this study. Fourth, the fully conditional specification method we used would result in biased estimates if the imputation model was mis-specified. Finally, estimated values calculated in the multiple imputation model were based on the observed data in this study. Therefore, the imputed BMI data can be biased from unobserved properties and assumptions in the model. Although we performed Little’s test to show that the missing mechanism of BMI was not MCAR, the possibility of MNAR being the missing mechanism is still possible in this study. Therefore, to assume MAR as a missing mechanism in this study, we considered maximum variables related to missing BMI values in the multiple imputation modelling.
In conclusion, our study showed that the occurrence of missing BMI data at ICU admission was not MCAR, and missingness could affect the prediction of 90-day mortality in critically ill patients. Particularly, missing BMI data had the potential to slightly overestimate the risk of 90-day mortality of underweight patients (BMI <20 kg/m2) at ICU admission. Our findings suggested that the lack of BMI data in critically ill patients admitted to the ICU might affect the predictive ability for mortality. Additionally, multiple imputation could be an appropriate statistical option to reduce the bias in critically ill patients.
Acknowledgments
Funding: None.
Footnote
Reporting Checklist: The authors have completed the STROBE reporting checklist. Available at http://dx.doi.org/10.21037/apm-20-203
Data Sharing Statement: Available at http://dx.doi.org/10.21037/apm-20-203
Conflicts of Interest: All authors have completed the ICMJE uniform disclosure form (available at http://dx.doi.org/10.21037/apm-20-203). The authors have no conflicts of interest to declare.
Ethical Statement: The authors are accountable for all aspects of the work in ensuring that questions related to the accuracy or integrity of any part of the work are appropriately investigated and resolved. This retrospective cohort study was approved by the Institutional Review Board (IRB) of Seoul National University Bundang Hospital (SNUBH) (IRB approval number: B-1806/474-105). Because of the retrospective design of the study, the requirement for informed consent was waived by the IRB. The study also conformed to the provisions of the Declaration of Helsinki (as revised in 2013).
Open Access Statement: This is an Open Access article distributed in accordance with the Creative Commons Attribution-NonCommercial-NoDerivs 4.0 International License (CC BY-NC-ND 4.0), which permits the non-commercial replication and distribution of the article with the strict proviso that no changes or edits are made and the original work is properly cited (including links to both the formal publication through the relevant DOI and the license). See: https://creativecommons.org/licenses/by-nc-nd/4.0/.
References
- Ligthelm RJ, Borzi V, Gumprecht J, et al. Importance of observational studies in clinical practice. Clin Ther 2007;29:1284-92. [Crossref]
- Yang W, Zilov A, Soewondo P, et al. Observational studies: going beyond the boundaries of randomized controlled trials. Diabetes Res Clin Pract 2010;88 Suppl 1:S3-9. [Crossref] [PubMed]
- Marston L, Carpenter JR, Walters KR, et al. Issues in multiple imputation of missing data for large general practice clinical databases. Pharmacoepidemiol Drug Saf 2010;19:618-26. [Crossref] [PubMed]
- Barnett AG, McElwee P, Nathan A, et al. Identifying patterns of item missing survey data using latent groups: an observational study. BMJ Open 2017;7:e017284. [Crossref] [PubMed]
- Pedersen AB, Mikkelsen EM, Cronin-Fenton D, et al. Missing data and multiple imputation in clinical epidemiological research. Clin Epidemiol 2017;9:157-66. [Crossref] [PubMed]
- Van Buuren S. Flexible imputation of missing data. Chapman and Hall/CRC; 2018.
- NCD Risk Factor Collaboration (NCD-RisC). Worldwide trends in body-mass index, underweight, overweight, and obesity from 1975 to 2016: a pooled analysis of 2416 population-based measurement studies in 128·9 million children, adolescents, and adults. Lancet 2017;390:2627-42. [Crossref] [PubMed]
- Cereda E, Klersy C, Hiesmayr M, et al. Body mass index, age and in-hospital mortality: The NutritionDay multinational survey. Clin Nutr 2017;36:839-47. [Crossref] [PubMed]
- Pickkers P, de Keizer N, Dusseljee J, et al. Body mass index is associated with hospital mortality in critically ill patients: an observational cohort study. Crit Care Med 2013;41:1878-83. [Crossref] [PubMed]
- Razzaghi H, Tinker SC, Herring AH, et al. Impact of Missing Data for Body Mass Index in an Epidemiologic Study. Matern Child Health J 2016;20:1497-505. [Crossref] [PubMed]
- Read SH, Lewis SC, Halbesma N, et al. Measuring the Association Between Body Mass Index and All-Cause Mortality in the Presence of Missing Data: Analyses From the Scottish National Diabetes Register. Am J Epidemiol 2017;185:641-9. [Crossref] [PubMed]
- Tin Tin S, Elwood JM, Lawrenson R, et al. Missing data on body mass index in a breast cancer register: how is it associated with patient characteristics and clinical outcomes? N Z Med J 2017;130:21-9. [PubMed]
- Mishra GD, Dobson AJ. Multiple imputation for body mass index: lessons from the Australian Longitudinal Study on Women's Health. Stat Med 2004;23:3077-87. [Crossref] [PubMed]
- Yoo S, Lee KH, Lee HJ, et al. Seoul National University Bundang Hospital's Electronic System for Total Care. Healthc Inform Res 2012;18:145-52. [Crossref] [PubMed]
- Aguinis H, Gottfredson RK, Joo H. Best-practice recommendations for defining, identifying, and handling outliers. Organizational Research Methods 2013;16:270-301. [Crossref]
- Seidell J, Deurenberg P, Hautvast J, et al. Obesity and fat distribution in relation to health-current insights and recommendations. Overweight and fat distribution-Associations with aspects of morbidity 1987.
- Little RJ, Rubin DB. Statistical analysis with missing data. John Wiley & Sons; 2014.
- White IR, Royston P. Imputing missing covariate values for the Cox model. Stat Med 2009;28:1982-98. [Crossref] [PubMed]
- White IR, Carlin JB. Bias and efficiency of multiple imputation compared with complete-case analysis for missing covariate values. Stat Med 2010;29:2920-31. [Crossref] [PubMed]
- Aldawood A, Arabi Y, Dabbagh O. Association of obesity with increased mortality in the critically ill patient. Anaesth Intensive Care 2006;34:629-33. [Crossref] [PubMed]
- Yatabe T, Yamashita K, Yokoyama M. Lower body mass index is associated with hospital mortality in critically ill Japanese patients. Asia Pac J Clin Nutr 2016;25:534-7. [PubMed]
- Sharafoddini A, Dubin JA, Maslove DM, et al. A New Insight Into Missing Data in Intensive Care Unit Patient Profiles: Observational Study. JMIR Med Inform 2019;7:e11605. [Crossref] [PubMed]
- Engerström L, Nolin T, Mardh C, et al. Impact of Missing Physiologic Data on Performance of the Simplified Acute Physiology Score 3 Risk-Prediction Model. Crit Care Med 2017;45:2006-13. [Crossref] [PubMed]
- Oh TK, Lee J, Hwang JW, et al. Value of Preoperative Modified Body Mass Index in Predicting Postoperative 1-Year Mortality. Sci Rep 2018;8:4614. [Crossref] [PubMed]
- Oh TK, Lee J, Lee YJ, et al. Association between Modified Body Mass Index and 30-Day and 1-Year Mortality after Intensive Care Unit Admission: A Retrospective Cohort Study. J Clin Med 2018;7:81. [Crossref] [PubMed]
- Arabi YM, Dara SI, Tamim HM, et al. Clinical characteristics, sepsis interventions and outcomes in the obese patients with septic shock: an international multicenter cohort study. Crit Care 2013;17:R72. [Crossref] [PubMed]
- Rosenman R, Tennekoon V, Hill LG. Measuring bias in self-reported data. Int J Behav Healthc Res 2011;2:320-32. [Crossref] [PubMed]