Risk prediction of in-hospital mortality among patients with type 2 diabetes mellitus and concomitant community-acquired pneumonia
Introduction
Diabetes mellitus (DM) is a metabolic disease characterized by chronic hyperglycemia. It is caused by impaired insulin secretion and/or impaired insulin utilization (1). Most patients have type 2 DM (T2DM). In the past decade, the overall prevalence of DM among Chinese adults has been between 9.7% and 11.6% (2-4). DM has become a severe disease and poses a significant economic burden on the Chinese Government and its citizens (5).
Respiratory tract infection is an infectious disease typically found in patients with DM (6); it is particularly common in hospitalized patients aged >60 years (7). Specifically, community-acquired pneumonia (CAP) is an important respiratory infection. Previous studies have shown that patients with DM exhibit impaired immune function and lung tissue injury, and their respiratory tracts are prone to pathogen colonization (8-10). Thus, DM is a risk factor for CAP and can also impact its prognosis. DM can increase the morbidity of CAP, exacerbate the disease, and increase the rate of complications and mortality risk (8,11-13). Furthermore, patients with DM and concomitant CAP exhibit distinct clinical features compared with patients without DM (11,12), they were more commonly mental confusion and more serious imaging findings on admission, but less likely to have clinical features such as cough and purulent sputum. Therefore, it is crucial to search for effective mortality predictors in DM patients with CAP, especially those who are easily identified or tested in the clinical setting, and to conduct mortality risk assessments among such patients. This will facilitate the identification of patients who require more active treatment.
There are existing clinical CAP prediction scores, such as the pneumonia severity index (PSI) and CURB-65, intended for the general population (14-18). There are also clinical scores for pneumonia for specific pathogens or specific populations, but to the best of our knowledge, there is currently a lack of clinical prediction scores for pneumonia in patients with DM (19,20). Therefore, the establishment of a simple and reliable CAP prediction score for patients with DM is of clinical significance. It will aid clinicians in better decision-making and allow patients with DM to receive more appropriate treatment. Based on this, we conducted a retrospective study in two centers in Shenyang, China. And we present the following article in accordance with the STROBE reporting checklist (available at http://dx.doi.org/10.21037/apm-20-1489).
Methods
Study design and data source
The study population was inpatients with a confirmed diagnosis of CAP and concomitant T2DM in the Shengjing Hospital of China Medical University and The First Affiliated Hospital of China Medical University in Shenyang, Liaoning Province, China, between September 2009 and October 2019. Case screening and data extraction at the Shengjing Hospital of China Medical University were performed using RealOne ISCI from Neusoft, whereas at The First Affiliated Hospital of China Medical University, they were performed using YIDUCLOUD. RealOne ISCI and YIDUCLOUD are intelligent platforms for electronic medical records, which can screen cases through specific criteria. Extracted data were manually verified and supplemented and did not contain any patients’ identity information. Patients’ clinical features were retrospectively analyzed, and an observational cohort study was conducted (Figure 1). The inclusion criterion was a confirmed diagnosis of CAP. Diagnostic criteria were based on the Diagnosis and Treatment of Community Acquired Pneumonia in Adults 2016, published by the Chinese Thoracic Society, for concomitant T2DM (21), and on the Standards of Medical Care for type 2 Diabetes in China 2019, published by the Chinese Diabetes Society (22). The exclusion criteria were as follows: type 1 DM; admission due to non-infectious pulmonary diseases, such as pulmonary embolism and pulmonary heart disease; active pulmonary tuberculosis; treatment outcome not observed; and age <18 years. All inpatients who meet the criteria are included in this study, whose number is 1, 360. All procedures performed in this study involving human participants were in accordance with the Declaration of Helsinki (as revised in 2013). The present study was examined and approved by the ethics committee of the institutions. Because of the retrospective nature of the research, the requirement for informed consent was waived.
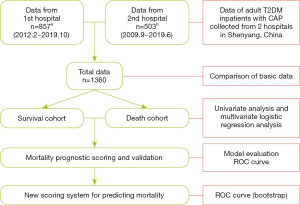
Study methods
Data of the selected cases, including personal history, medical history, laboratory and imaging findings on admission, and treatment outcomes, were collected. If the data of the same variable from two hospitals has different units, the data would be converted to the same unit. Based on the patients’ treatment outcomes (deceased, improved and cured), the cohort was divided into two cohorts: deceased in one cohort, improved or cured in the other cohort. General characteristics, relevant laboratory parameters, and treatment outcomes of the two groups were compared. Factors affecting the prognosis of patients with T2DM and concomitant CAP were analyzed based on the following parameters: age; pulse rate, respiratory rate, and blood pressure on admission; mental confusion from disease onset to admission; duration of DM (years); DM-related complications; history of chronic diseases; fasting glucose on admission; glycated hemoglobin A1c (HbA1c); routine blood test on admission; arterial blood gas analysis; serum ion; serum albumin; and blood urea nitrogen. We selected several common DM-related complications including diabetic nephropathy, diabetic foot, diabetic retinopathy and diabetic peripheral neuropathy.
The two composite parameters, neutrophil-lymphocyte ratio (NLR) and platelet-lymphocyte ratio (PLR), can be used to predict the severity of pneumonia (23). Thus, these two parameters were also included in the analysis.
Statistical analysis
R Project for Statistical Computing software (version 3.6.1) was used for statistical processing. In particular, RMS 5.1-4 software was employed for univariate and multivariate analyses. Quantitative data were expressed as mean ± standard deviation. Qualitative and categorical data were expressed as count (proportion). Stochastic single imputation was used to complete the missing values for certain variables. Segmentation was applied to the quantitative and qualitative data based on reference values and clinical experience, and one-way analysis of variance was used for the analysis. Based on the results of the univariate analysis, a stepwise multivariate analysis was performed on the relevant variables selected based on clinical experience and their odds ratio (OR) values (from high to low). Statistical significance was set at P<0.05.
Results
General patient characteristics
A total of 1,360 (males, n=850, 62.5%; females, n=510, 37.5%) patients were enrolled in the cohort. The death cohort comprised 129 patients (9.5%), and the survival cohort comprised 1,231 patients (90.5%). The mean length of hospital stay of the death cohort and the survival cohort was 16.28±27.27 and 29.35±72.28 days, respectively. The death cohort had an older average age and men were more likely to die seemingly. Their vital signs, such as consciousness, pulse and diastolic blood pressure, were worse on admission. They had higher fasting glucose, NLR and PLR, and other worse laboratory findings on admission. Besides, they were prone to multilobar infiltrates and pleural effusion in imaging findings. Furthermore, they had more concomitant diseases such as diabetic nephropathy, chronic renal failure, cerebrovascular disease and neoplastic disease. The specific measurement results are listed in Table 1.
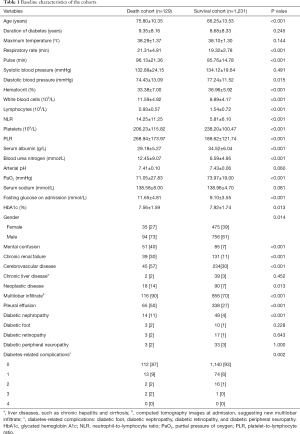
Full table
In the above variables, the missing data in pulmonary imaging is 0.74%, in serum albumin is 0.66%, in fasting glucose on admission is 11.18%, in HbA1c is 35.07%, in arterial pH and PaO2 are 29.12%, in serum sodium is 0.07%, in blood urea nitrogen is 0.29%, and other variables have no missing data.
Univariate analysis on disease severity in patients with T2DM and concomitant CAP
Univariate analysis was performed on 19 variables from Table 1, including age, NLR, arterial blood pH, and duration of DM, based on P values and clinical experience. The results of the univariate analysis showed that age ≥65 years, male sex, pulse rate ≥125 bpm, systolic blood pressure <90 mmHg or diastolic blood pressure ≤60 mmHg, mental confusion, NLR ≥4, PLR >200, lymphocyte count <0.8×109/L, serum sodium ≤130 mmol/L, arterial blood pH ≤7.35, partial pressure of oxygen <60 mmHg, fasting glucose on admission ≥9 mmol/L, multilobar infiltrate confirmed by chest computed tomography (CT) on admission, pleural effusion, chronic renal insufficiency, diabetic nephropathy, and cerebrovascular disease were risk factors affecting disease prognosis (Table 2).
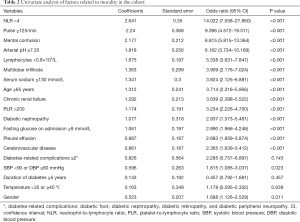
Full table
Multivariate analysis on disease severity in patients with T2DM and concomitant CAP
Based on the results of the univariate analysis, as well as clinical experience, stepwise Akaike information criterion multivariate analysis was conducted on the following 12 variables (in descending OR values): NLR, pulse rate, mental confusion, arterial blood pH, multilobar infiltrate, serum sodium, age, diabetic nephropathy, fasting glucose on admission, pleural effusion, cerebrovascular disease, and DM complications. The results showed that pulse rate ≥125 bpm, arterial blood pH ≤7.35, age ≥65 years, mental confusion, NLR ≥4, multilobar infiltrate, serum sodium ≤130 mmol/L, diabetic nephropathy, and fasting glucose on admission ≥9 mmol/L were independent predictors of disease severity (Table 3).
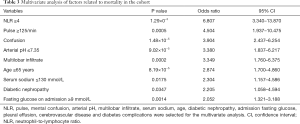
Full table
Construction of a prediction model for disease severity in patients with T2DM and concomitant CAP
Based on the results of the multivariate analysis, we constructed a novel prediction score. The prediction rule, which we coined T2DMCAP, was based on nine variables: pulse rate, arterial blood pH, age, mental confusion, NLR, multilobar infiltrate, serum sodium, diabetic nephropathy, and fasting glucose on admission. Each item of the T2DMCAP score was counted as one point, and the total score was nine points. The total score was the summed score of all items (Table 4). Sensitivity, specificity, Positive Predictive Value (PPV) and Negative Predictive Value (NPV) of T2DMCAP are shown in Table 5. In the cohort of the present study, the area under the curve (AUC) of T2DMCAP, CURB-65, and the PSI was 0.864, 0.788, and 0.854, respectively. After bootstrap validation (n=2,000), the AUC of the above three scores was 0.858, 0.774, and 0.831, respectively (Figure 2). It was evident from the AUC that, of the three prediction rules, the prediction ability of T2DMCAP was significantly superior to that of CURB-65 and slightly superior to that of the PSI.
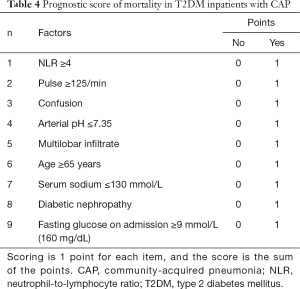
Full table
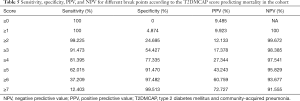
Full table
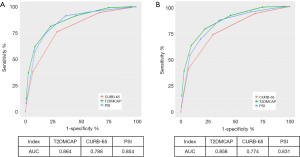
Discussion
The PSI is a commonly used prediction score for CAP in clinical practice (14). It contains 18 items, stratifies patients into five classes, and can effectively predict patient mortality risk. CURB-65 is another commonly used clinical score that stratifies patients into different groups, based on disease severity, in a simple and effective manner (15). When comparing the T2DMCAP score that we established with the two aforementioned prediction scores, the CURB-65 score lacked DM-related parameters, whereas the PSI score only included one DM-related parameter: blood glucose. In the present study, bootstrap validation showed that the prediction score T2DMCAP was significantly superior to that of CURB-65 and slightly superior to that of the PSI. In addition, T2DMCAP was able to identify the mortality risk in different patients (Table 6). Compared with the PSI, which uses 18 variables and a complex scoring method, T2DMCAP uses only nine variables and a simple scoring method. Thus, T2DMCAP has simpler requirements and better clinical usability.
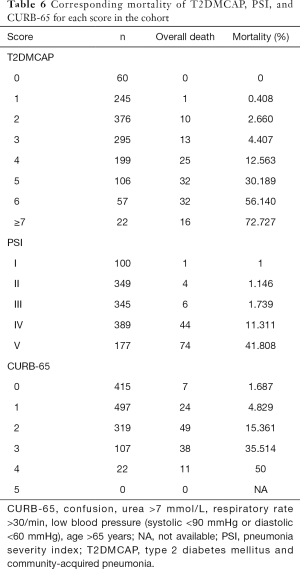
Full table
DM is a chronic disease that can involve multiple organs. In our clinical prediction model, T2DMCAP, the patient’s baseline condition, pneumonia-related parameters, and DM-related parameters were considered collectively. The patient’s baseline condition was represented by age, mental confusion, pulse rate and serum sodium; the patient’s inflammatory status was represented by the NLR, arterial blood pH, and lung CT imaging; and the status of the patient’s DM control was represented by fasting glucose on admission and diabetic nephropathy. The validation results showed that patients with a score ≥4 warranted greater levels of clinical monitoring, and active treatment should be provided (Table 6). Nonetheless, further investigations are required to determine whether these patients will benefit from active treatment, and what type of treatment modalities would be most effective.
In previously published studies, CAP prediction scores have demonstrated the importance of certain parameters, such as age, mental confusion, pulse rate, serum sodium, arterial blood pH, and multilobar infiltrate when assessing disease severity, supporting the findings of the present study (14-18). However, our research focused on the T2DM population specifically among patients with CAP. Previous studies have shown that numerous factors might adversely affect the prognosis of these patients. In the present study, we attempted to analyze the factors present in patients with T2DM that would affect the prognosis of CAP. The results showed that hyperglycemia observed on admission, concurrent diabetic nephropathy, and a high NLR significantly affected patient prognosis.
HbA1c reflects recent glycemic control in patients (1). A high HbA1c increases the hospitalization risk of patients with DM owing to pneumonia (13). However, in the present study, a high HbA1c was not a risk factor for patient prognosis. Previous clinical studies from Portugal and Taiwan have shown that a high HbA1c is not associated with the prognosis of infectious diseases in patients with DM (24,25). Hyperglycemia is common among patients with DM and concomitant CAP (11,26). In a previously published study, hyperglycemia was reported in 67% of hospitalized pneumonia patients and 81% of hospitalized pneumonia patients who had a new diagnosis of DM (27). Hyperglycemia on admission may be caused by acute stress, chronic hyperglycemia, or both (28). HbA1c, however, only reflects the patient’s recent blood glucose status, but not their acute stress. Therefore, fasting blood glucose levels on admission can reflect both baseline blood glucose levels and the acute stress status of patients with DM, while minimizing the effect of diet on blood glucose. In the present study, we demonstrated that a high fasting blood glucose level was an independent risk factor for patient mortality. Furthermore, previous studies also showed that hyperglycemia on admission significantly increased the incidence of complications and the mortality rate of CAP patients (28). For every 1 mmol/L increase in the blood glucose of patients with T2DM on admission, the 30-day mortality rate increased by 3.2%, and the 90-day mortality rate increased by 1.8% (29).
In addition, infection can decrease insulin levels and increase cortisol, catecholamine, and glucagon concentrations in patients with DM, which result in increased gluconeogenesis and glycogen breakdown, as well as reduced glucose utilization by tissue. Therefore, hyperglycemia is more common among patients with DM and concomitant CAP, and these patients are susceptible to concurrent ketoacidosis (8). CAP may cause hypoxia in patients, which in turn increases the risk of consequent lactic acidosis (25). Acidosis will lower the pH of arterial blood. A lowering of arterial blood pH results in more severe disease conditions (14-17), an observation which was confirmed in the present study. Thus, patients with lowered arterial blood pH should receive active treatment to restore acid-base homeostasis.
In the present study, we analyzed the impact of several DM complications on the prognosis of CAP, including diabetic nephropathy, diabetic foot, diabetic retinopathy, and diabetic peripheral neuropathy. The results showed that only diabetic nephropathy was an independent risk factor for patient prognosis. The number of DM complications differed between the two patient groups, but the difference was not statistically significant after segmentation. Previous studies have also shown that diabetic nephropathy and renal insufficiency are risk factors impacting the prognosis of CAP in patients with DM (11,12); renal insufficiency is also a risk factor for the prognosis of CAP patients without DM (14). A survey on the causes of death among patients with DM in Japan between 2001 and 2010 showed that, among patients with poor glycemic control, the lifespan of patients with concurrent diabetic nephropathy was shortened the most (30). It is currently believed that impaired renal function in patients with diabetic nephropathy further exacerbates metabolic dysregulation, malnutrition, and immune dysfunction. Furthermore, the reduced secretion of erythropoietin causes anemia, which renders the patient more susceptible to pulmonary infection and worse prognosis (31,32). Therefore, among other DM complications, diabetic nephropathy has a significant adverse impact on the prognosis of CAP. Additional attention should be paid to patients with concurrent diabetic nephropathy, especially those undergoing dialysis.
The NLR is currently used for predicting the prognosis of many diseases, including infections, cardiovascular diseases, certain cancers, and diabetes complications (33-38). The NLR is a composite marker that can be easily measured and has a short testing time, facilitating its use in clinical practice. Neutrophils are important immune cells that play a crucial role in the body’s response to infection. Neutrophil count increases during infection and thus represents the extent of inflammation. Lymphocytes are also important immune cells. Studies have shown that the peripheral blood lymphocyte count has a significant positive correlation with the level of cellular immunity in the body (39). The peripheral blood lymphocyte count is negatively correlated with the level of cellular immunity during infection or inflammation (40). Furthermore, lymphocyte counts of deceased patients are significantly lower than those of surviving patients (41), indicating that immune function can be used to assess the prognosis of CAP patients. The NLR represents the ratio of the two aforementioned leukocyte subtypes, which effectively overcomes the drawbacks of absolute values that may be affected by factors such as dehydration. It also reflects both the intrinsic cause (immune function) and extrinsic cause (extent of inflammation) of the disease, and exhibits a higher degree of clinical significance when compared with the independent parameters. A previous study showed that the NLR is superior to other inflammation-based indices or scores in predicting mortality in patients with pneumonia (42). Other studies have reported that the NLR in patients with DM is higher than that in patients without DM (43), and that the increase in the NLR is more prominent when DM complications and infections are present (35-38,44). The patients included in the present study had T2DM and concomitant CAP, and the NLR were generally higher than normal reference values (45). This suggests the utility of the NLR as a predictive marker for disease severity in patients with T2DM and concomitant CAP.
Previous studies have found that platelets can trigger an inflammatory response and affect a diverse array of immune cells; the number of platelets decreases when the body is undergoing infection and inflammation (33). Thrombocytopenia is associated with disseminated intravascular coagulation and severe sepsis, and is an indicator of disease progression or poor prognosis in pneumonia patients (46). Thrombocytopenia is also listed as one of the diagnostic criteria for severe CAP in the American CAP guidelines (47). In the present study, the platelet count significantly differed between the two patient groups, but it was not an independent predictor of disease severity. In addition, although the PLR also significantly differed between the two patient groups, the OR (3.234) was significantly lower than that of the NLR (14.022). Other studies have also shown that PLR is less effective than the NLR in evaluating the severity of pneumonia (23). Thus, the PLR was not included in the multifactor analysis in the present study.
The data in the present study were collected from two grade 3A hospitals in Shenyang, China; this dual-center study design was a strength of our study. Nonetheless, our study also had several limitations. First, most patients had taken their own medications or received outpatient treatment prior to admission, and the use of diabetes medications had also been adjusted in some patients after disease onset. Therefore, the status of blood glucose control on admission may be different from their usual condition. This was an inevitable influencing factor in the present study. Second, as the present study only included inpatients, the score should not be applied to outpatients. In addition, we did not exclude patients who restricted treatment escalation (e.g., refused to be rescued), nor did we consider the impact of such restrictions on mortality. Finally, this was a retrospective study, and population-based data were not used. The severity of patients’ conditions might also vary among different tertiary hospitals in the same area, thus leading to an inevitable selection bias. It should also be emphasized that the applicability of the T2DMCAP score still needs to be validated in more cohorts with T2DM and concomitant CAP. To verify its accuracy, future studies should utilize this score to predict patient mortality.
In summary, DM increases the risk and severity of CAP (11-13). Multiple factors can predict disease severity; age, change in state of consciousness, pulse rate, arterial blood pH, serum sodium, NLR, initial fasting blood sugar, multilobar involvement, and concurrent diabetic nephropathy were independent predictors of patient mortality. In addition, a simple and easy-to-use mortality risk prediction score, T2DMCAP, was constructed for patients with T2DM and concomitant CAP to predict the disease severity of hospitalized patients. The score can be used for prognostic assessment and clinical decision-making for T2DM patients with CAP.
Acknowledgments
We thank Neusoft and Neusoft Research of Intelligent Healthcare Technology for their support with the data extraction, analysis, and evaluation by leveraging RealOne ISCI and RealMedSci, their clinic research platform and automatic medical analysis platform. The authors also thank the staff from the First Affiliated Hospital of China Medical University for their helpful contributions to the study.
Funding: The present study was supported by the National Key R&D Program of China (grant No. 2017YFC1309702).
Footnote
Reporting Checklist: The authors have completed the STROBE reporting checklist. Available at http://dx.doi.org/10.21037/apm-20-1489
Data Sharing Statement: Available at http://dx.doi.org/10.21037/apm-20-1489
Conflicts of Interest: All authors have completed the ICMJE uniform disclosure form (available at http://dx.doi.org/10.21037/apm-20-1489). The authors have no conflicts of interest to declare.
Ethical Statement: The authors are accountable for all aspects of the work in ensuring that questions related to the accuracy or integrity of any part of the work are appropriately investigated and resolved. All procedures performed in this study involving human participants were in accordance with the Declaration of Helsinki (as revised in 2013). The present study was examined and approved by the ethics committee of the institutions. Because of the retrospective nature of the research, the requirement for informed consent was waived.
Open Access Statement: This is an Open Access article distributed in accordance with the Creative Commons Attribution-NonCommercial-NoDerivs 4.0 International License (CC BY-NC-ND 4.0), which permits the non-commercial replication and distribution of the article with the strict proviso that no changes or edits are made and the original work is properly cited (including links to both the formal publication through the relevant DOI and the license). See: https://creativecommons.org/licenses/by-nc-nd/4.0/.
References
- Alberti KG, Zimmet PZ. Definition, diagnosis and classification of diabetes mellitus and its complications. Part 1: diagnosis and classification of diabetes mellitus provisional report of a WHO consultation. Diabet Med 1998;15:539-53. [Crossref] [PubMed]
- Yang W, Lu J, Weng J, et al. China National Diabetes and Metabolic Disorders Study Group. Prevalence of diabetes among men and women in China. N Engl J Med 2010;362:1090-101. [Crossref] [PubMed]
- Bui HD, Jing X, Lu R, et al. Prevalence of and factors related to microvascular complications in patients with type 2 diabetes mellitus in Tianjin, China: a cross-sectional study. Ann Transl Med 2019;7:325. [Crossref] [PubMed]
- Wang H, Wang M, Wang J, et al. Cost-effectiveness analysis of comprehensive intervention programs to control blood glucose in overweight and obese type 2 diabetes mellitus patients based on a real-world setting: Markov modeling. Ann Transl Med 2019;7:676. [Crossref] [PubMed]
- Mao W, Yip CW, Chen W. Complications of diabetes in China: health system and economic implications. BMC Public Health 2019;19:269. [Crossref] [PubMed]
- Hine JL, de Lusignan S, Burleigh D, et al. Association between glycaemic control and common infections in people with Type 2 diabetes: a cohort study. Diabet Med 2017;34:551-7. [Crossref] [PubMed]
- Lin W, Chen C, Guan H, et al. Hospitalization of elderly diabetic patients: characteristics, reasons for admission, and gender differences. BMC Geriatr 2016;16:160. [Crossref] [PubMed]
- Boyanova L, Mitov I. Antibiotic resistance rates in causative agents of infections in diabetic patients: rising concernsJ. Expert Rev Anti Infect Ther 2013;11:411-20. [Crossref] [PubMed]
- Frydrych LM, Fattahi F, He K, et al. Diabetes and Sepsis: Risk, Recurrence, and Ruination. Front Endocrinol (Lausanne) 2017;8:271. [Crossref] [PubMed]
- Lin CC, Chang CT, Li TC, et al. Objective evidence of impairment of alveolar integrity in patients with non-insulin-dependent diabetes mellitus using radionuclide inhalation lung scan. Lung 2002;180:181-6. [Crossref] [PubMed]
- Di Yacovo S, Garcia-Vidal C, Viasus D, et al. Clinical features, etiology, and outcomes of community-acquired pneumonia in patients with diabetes mellitus. Medicine (Baltimore) 2013;92:42-50. [Crossref] [PubMed]
- Falguera M, Pifarre R, Martin A, et al. Etiology and outcome of community-acquired pneumonia in patients with diabetes mellitus. Chest 2005;128:3233-9. [Crossref] [PubMed]
- Kornum JB, Thomsen RW, Riis A, et al. Diabetes, glycemic control, and risk of hospitalization with pneumonia: a population-based case-control study. Diabetes Care 2008;31:1541-5. [Crossref] [PubMed]
- Fine MJ, Auble TE, Yealy DM, et al. A prediction rule to identify low-risk patients with community-acquired pneumonia. N Engl J Med 1997;336:243-50. [Crossref] [PubMed]
- Lim WS, van der Eerden MM, Laing R, et al. Defining community acquired pneumonia severity on presentation to hospital: an international derivation and validation study. Thorax 2003;58:377-82. [Crossref] [PubMed]
- Miyashita N, Matsushima T, Oka M, et al. The JRS guidelines for the management of community-acquired pneumonia in adults: an update and new recommendations. Intern Med 2006;45:419-28. [Crossref] [PubMed]
- Charles PG, Wolfe R, Whitby M, et al. Australian Community-Acquired Pneumonia Study Collaboration. Grayson ML. SMART-COP: a tool for predicting the need for intensive respiratory or vasopressor support in community-acquired pneumonia. Clin Infect Dis 2008;47:375-84. [Crossref] [PubMed]
- Ewig S, de Roux A, Bauer T, et al. Validation of predictive rules and indices of severity for community acquired pneumonia. Thorax 2004;59:421-7. [Crossref] [PubMed]
- Guo L, Wei D, Zhang X, et al. Clinical Features Predicting Mortality Risk in Patients With Viral Pneumonia: The MuLBSTA Score. Front Microbiol 2019;10:2752. [Crossref] [PubMed]
- Tashiro M, Fushimi K, Takazono T, et al. A mortality prediction rule for non-elderly patients with community-acquired pneumonia. BMC Pulm Med 2016;16:39. [Crossref] [PubMed]
- Cao B, Huang Y, She DY, et al. Diagnosis and treatment of community-acquired pneumonia in adults: 2016 clinical practice guidelines by the Chinese Thoracic Society, Chinese Medical Association. Clin Respir J 2018;12:1320-60. [Crossref] [PubMed]
- Jia W, Weng J, Zhu D, et al. Standards of medical care for type 2 diabetes in China 2019. Diabetes Metab Res Rev 2019;35:e3158. [Crossref] [PubMed]
- Lee JH, Song S, Yoon SY, et al. Neutrophil to lymphocyte ratio and platelet to lymphocyte ratio as diagnostic markers for pneumonia severity. Br J Biomed Sci 2016;73:140-2. [Crossref] [PubMed]
- Tsai SS, Huang JC, Chen ST, et al. Characteristics of Klebsiella pneumoniae bacteremia in community-acquired and nosocomial infections in diabetic patients. Chang Gung Med J 2010;33:532-9. [PubMed]
- Ferreira L, Moniz AC, Carneiro AS, et al. The impact of glycemic variability on length of stay and mortality in diabetic patients admitted with community-acquired pneumonia or chronic obstructive pulmonary disease. Diabetes Metab Syndr 2019;13:149-53. [Crossref] [PubMed]
- Chen PC, Liao WI, Wang YC, et al. An Elevated Glycemic Gap is Associated With Adverse Outcomes in Diabetic Patients With Community-Acquired Pneumonia. Medicine (Baltimore) 2015;94:e1456. [Crossref] [PubMed]
- MacIntyre EJ, Majumdar SR, Gamble JM, et al. Stress hyperglycemia and newly diagnosed diabetes in 2124 patients hospitalized with pneumonia. Am J Med 2012;125:1036.e17-23. [Crossref] [PubMed]
- McAlister FA, Majumdar SR, Blitz S, et al. The relation between hyperglycemia and outcomes in 2,471 patients admitted to the hospital with community-acquired pneumonia. Diabetes Care 2005;28:810-5. [Crossref] [PubMed]
- Kornum JB, Thomsen RW, Riis A, et al. Type 2 diabetes and pneumonia outcomes: a population-based cohort study. Diabetes Care 2007;30:2251-7. [Crossref] [PubMed]
- Nakamura J, Kamiya H, Haneda M, et al. Causes of death in Japanese patients with diabetes based on the results of a survey of 45,708 cases during 2001-2010: Report of the Committee on Causes of Death in Diabetes Mellitus. J Diabetes Investig 2017;8:397-410. [Crossref] [PubMed]
- Jin Z, Shandong Y, Xuenong X. Clinical characteristics of diabetic nephropathy patients complicated with pulmonary infections. Chinese Journal of Nosocomiology 2013;23:5428-30.
- He F, Wu X, Xia X, et al. Pneumonia and mortality risk in continuous ambulatory peritoneal dialysis patients with diabetic nephropathy. PLoS One 2013;8:e61497. [Crossref] [PubMed]
- Kartal O, Kartal AT. Value of neutrophil to lymphocyte and platelet to lymphocyte ratios in pneumonia. Bratisl Lek Listy 2017;118:513-6. [PubMed]
- Luo P, Li R, Yu S, et al. The Relationship between Neutrophil-to-Lymphocyte Ratio and Intracerebral Hemorrhage in Type 2 Diabetes Mellitus. J Stroke Cerebrovasc Dis 2017;26:930-7. [Crossref] [PubMed]
- Xu T, Weng Z, Pei C, et al. The relationship between neutrophil-to-lymphocyte ratio and diabetic peripheral neuropathy in Type 2 diabetes mellitus. Medicine (Baltimore) 2017;96:e8289. [Crossref] [PubMed]
- Yin Y, Wang J, Wang X, et al. Prognostic value of the neutrophil to lymphocyte ratio in lung cancer: A meta-analysis. Clinics(Sao Paulo) 2015;70:524-30. [Crossref] [PubMed]
- Vatankhah N, Jahangiri Y, Landry GJ, et al. Predictive value of neutrophil-to-lymphocyte ratio in diabetic wound healing. J Vasc Surg 2017;65:478-83. [Crossref] [PubMed]
- Huang W, Huang J, Liu Q, et al. Neutrophil-lymphocyte ratio is a reliable predictive marker for early-stage diabetic nephropathy. Clin Endocrinol (Oxf) 2015;82:229-33. [Crossref] [PubMed]
- Zhao B, Chen YY, Tan MQ. Value of lymphocyte count in assessing cellular immune function in patients with community-acquired pneumonia. Nan Fang Yi Ke Da Xue Xue Bao 2016;36:273-6. [PubMed]
- Ommen SR, Gibbons RJ, Hodge DO, et al. Usefulness of the lymphocyte concentration as a prognostic marker in coronary artery disease. Am J Cardiol 1997;79:812-4. [Crossref] [PubMed]
- Shi SJ, Li H, Liu M, et al. Mortality prediction to hospitalized patients with influenza pneumonia: PO(2) /FiO(2) combined lymphocyte count is the answer. Clin Respir J 2017;11:352-60. [Crossref] [PubMed]
- Shimoyama Y, Umegaki O, Inoue S, et al. The Neutrophil to Lymphocyte Ratio Is Superior to Other Inflammation-Based Prognostic Scores in Predicting the Mortality of Patients with Pneumonia. Acta Med Okayama 2018;72:591-3. [PubMed]
- Mertoglu C, Gunay M. Neutrophil-Lymphocyte ratio and Platelet-Lymphocyte ratio as useful predictive markers of prediabetes and diabetes mellitus. Diabetes Metab Syndr 2017;11 Suppl 1:S127-31. [Crossref] [PubMed]
- Demirdal T, Sen P. The significance of neutrophil-lymphocyte ratio, platelet-lymphocyte ratio and lymphocyte-monocyte ratio in predicting peripheral arterial disease, peripheral neuropathy, osteomyelitis and amputation in diabetic foot infection. Diabetes Res Clin Pract 2018;144:118-25. [Crossref] [PubMed]
- Forget P, Khalifa C, Defour JP, et al. What is the normal value of the neutrophil-to-lymphocyte ratio? BMC Res Notes 2017;10:12. [Crossref] [PubMed]
- Zhifang C. CAP and Study on coagulation in patients with diabetes mellitus and its clinical correlation factors. Journal of Internal Intensive Medicine 2015;21:224-5.
- Mandell LA, Wunderink RG, Anzueto A, et al. Infectious Diseases Society of America/American Thoracic Society consensus guidelines on the management of community-acquired pneumonia in adults. Clin Infect Dis 2007;44 Suppl 2:S27-72. [Crossref] [PubMed]