Biomarkers for the diagnosis of sepsis-associated acute kidney injury: systematic review and meta-analysis
Introduction
Sepsis is defined as organ dysfunction caused by the host’s adverse response to infection. One of the most common organs affected is the kidney, leading to acute kidney injury associated with sepsis (SA-AKI). In recent years, the incidence of sepsis has been increasing annually. A comprehensive assessment of 750 million people admitted to the hospital in the United States from 1979 to 2000 found that sepsis increased from 82.7 to 240.4 per 100,000 individuals, with an annual growth rate of 8.7% (1), and the inpatient mortality rate was as high as 17.9% (2). Secondary AKI significantly increased sepsis mortality. Sepsis is associated with up to 50% of AKI cases, and up to 60% of sepsis patients have AKI (3,4). Patients with sepsis combined with AKI had significantly higher mortality than those without AKI (5-7). In addition, sepsis-related patients with AKI have significantly increased mortality compared with patients with AKI of another etiology (6). Independent risk factors for the occurrence of AKI in sepsis include insufficient blood volume and exposure to toxic renal therapy, among others. Although the pathophysiological mechanism of AKI in sepsis is still not fully understood, the relationship between the two is close (8); some studies have shown that the inflammatory cascade reaction of sepsis is the cause of AKI (9).
To date, sepsis and acute kidney injury (AKI) have been diagnosed primarily based on urine volume and serum creatinine measurements. The commonly used diagnostic criteria are the RIFLE, AKIN and KDIGO standards (10-12). Since the late diagnosis of AKI based solely on urine volume and serum creatinine has been questioned, early diagnosis is of great clinical concern, so we need to provide more insights on this. Numerous studies have focused on the early identification of AKI biomarkers. Zhang et al. conducted a meta-analysis in 2016 (13), focusing on neutrophil gelatinase-associated lipocalin (NGAL), a plasma biomarker of human AKI, also known as lipid carrier protein-2. However, these researchers summarized only a partial biomarker of AKI in plasma; other biomarkers, such as urinary interleukin-18 and kim-1, have not been evaluated (14). There also were inconsistent findings regarding the research topic of biomarkers of sepsis-associated AKI.
Therefore, the following steps need to be taken: provide a ranking system for distinguishing disease in high-risk patients and determining prognosis. The purpose of this study was to compare biomarker levels in patients with AKI with samples from at-risk controls. This study intends to compare the predictive value of and differences in various indicators of AKI in patients with sepsis through literature data analysis and to explore the correlation between the biomarkers of patients with sepsis-associated AKI.
We present the following article in accordance with the PRISMA reporting checklist (available at http://dx.doi.org/10.21037/apm-20-1855).
Methods
Data sources and research choices
We manually searched the PubMed, Embase, Web of Science, Cochrane libraries and CNKI for articles on biomarkers of AKI in blood and urine samples released before January 30, 2021. We also searched the references of these articles for relevant papers to include in the study. Two researchers screened and evaluated all studies independently, and a third reviewer intervened when differences arose. For literature search, the terms used were “sepsis”, “acute kidney injury”, “biomarkers”, “diagnosis”.
Inclusion criteria
(I) the article is an original research paper on adult patients with sepsis, (II) the research concerns the association of clinical outcomes and biomarkers measured in blood or urine in an accurate value report (diagnosis of AKI in patients with risk and/or AKI mortality), (III) the paper describes the demographic variables of the study participants, and (IV) the paper is written in Chinese or English. We defined the inclusion of related studies by using PICOs (Patient, Intervention, Comparison and Outcome) criteria, in particular the design of studies to be included. P: Patients of sepsis. I: Patients of sepsis-associated AKI. C: Patients without sepsis-associated AKI. O: Diagnosis of sepsis associated kidney injury.
Exclusion criteria
(I) Articles written in languages other than Chinese or English, (II) articles unrelated to AKI, (III) articles that were not original studies, (IV) in vivo/in vitro studies, (V) pediatric studies, (VI) articles that analyzed biomarkers not measured in blood or urine, and (VII) articles that analyzed biomarkers for therapeutic monitoring.
Data extraction and quality assessment
We created Excel spreadsheets (Microsoft Corp., Redmond, WA, USA) to extract data from the included studies, and two researchers independently completed the data extraction. The average or median levels and standard deviation (SD) of AKI etiology and biomarkers in blood or urine were obtained. When the biomarkers were measured multiple times, only the measurements of the first day were extracted.
Research groups
First, the patients with sepsis were divided into two groups, namely, the AKI group and the non-AKI group, according to whether they had AKI status after admission.
We extracted the average blood and urine biomarker concentrations from the different subgroups. The sensitivity, specificity, true positives, false positives, false negatives, true negatives, area under the ROC curve and other indicators were collected. In addition, the data on demographic variables (age, gender and number of participants in each subgroup), diagnostic criteria for sepsis, diagnostic criteria for AKI, AKI mortality rate, time of sample collection, and sample type were collected.
The quality of all studies was assessed according to the quality assessment of QUADAS-2, a diagnostic accuracy study score, and the content was customized according to QUADAS-2 (15) guidelines. The risk of bias and applicability concerning graphs/abstracts were assessed using Review Manager version 5.3 (Cochrane Collaboration, Oxford, UK).
Statistical analysis
Revman 5.3 software was used, and the chi-squared test was used for heterogeneity analysis. Heterogeneity was interpreted as an I2 value =0% as no heterogeneity, 25%= low heterogeneity, 50%= moderate heterogeneity, and 75%= high heterogeneity. If no heterogeneity was observed between studies (P>0.1), a fixed-effect model was adopted. If there was heterogeneity between studies (P<0.1), a random-effect model was selected. The sources of heterogeneity of included studies main were different diagnostic criteria for sepsis and AKI, time of sample collection and patients came from different departments. Then, the weighted sensitivity, specificity, positive likelihood ratio, negative likelihood ratio, diagnostic odds ratio and 95% CI of each study were calculated by using Revman 5.3 software, and the SROC curve was calculated and summarized to indicate the accuracy of the diagnostic test and reflect the SROC.
The closer the curve is to the upper left corner, the higher the value is, and thus the higher the accuracy of the diagnostic test is; the results are represented by a forest chart.
Results
Literature retrieval
Literature retrieval produced a total of 1,227 articles from the database: PubMed, 444 articles; Web of Science, 512 articles; Embase, 259 articles; Cochrane library, 9 articles; and 3 articles from the reference lists of the articles included in the study. Studies were primarily excluded by reviewing titles and abstracts for the following factors: in vitro/animal studies (n=434), duplications (n=423), articles other than original studies (comments, editorials or case reports, n=90), and articles (n=30) that were not related to the incidence or mortality of AKI. After initial screening, 95 articles remained for full-text review. Of these, six articles contained insufficient data. For four of these articles, we attempted to contact the author; however, copies of the full text were not available. The remaining 42 articles were used for the meta-analysis (Figure 1).
Research characteristics and quality assessment
Demographic variables included in the study are summarized in Table 1. A total of 42 articles involving 4,189 patients were included in this meta-analysis. In 25% of the studies, AKI was diagnosed based on the AKIN criteria. Other standards, such as RIFLE and KDIGO, are used with the AKIN standard. The mean age was 55 to 79 years, and the mortality rate was 30% to 66%. Blood and urine were collected 30 minutes after admission and 72 hours after AKI diagnosis. In terms of sample type, 50% of the studies used biomarkers in urine. Fifty percent used biomarkers in the blood. Blood and urine were used in 3 articles.
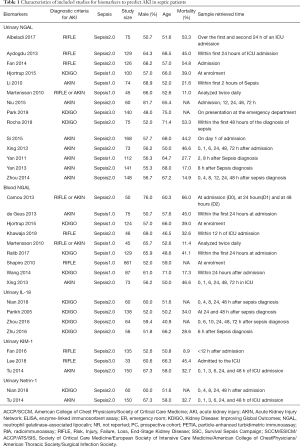
Full table
Biomarker characteristics associated with AKI diagnosis
We conducted a meta-analysis of 22 blood biomarkers related to the diagnosis of AKI in sepsis (Table 1). Biomarkers such as NGAL, KIM-1, and IL-18, were collected, among which NGAL (16-38) had the highest levels, and among the biomarkers with significant diagnostic value among subgroups, sensitivity and specificity were also high (Table 2). However, general heterogeneity was shown. We conducted an impact analysis to check the sensitivity of the results. The impact analysis suggested that the heterogeneity may be caused by the limited number of studies. By removing the outliers studies, we observed a strong effect of the biomarkers. The biomarkers NGAL, KIM1 and IL-18 were analyzed by subgroup. Since most of the included studies were case-control studies, we excluded other study design types. Markers of endothelial dysfunction, including sTM, C protein, E selectin, and PAI-1, showed diagnostic significance (39,40). Among them, C protein, E selectin and PAI-1 have been found to have lower predictive value for AKI than sTM (41-43); therefore, they were not included in the table. The coagulation function marker ATIII also showed diagnostic significance, as did markers of inflammatory response, including KIM-1 (44), IL-18 (38,45-48), and OPG (49), and estradiol as a marker of hormone response level (50).
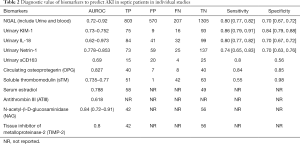
Full table
Systematic assessment of biomarkers associated with AKI diagnosis
For urinary NGAL, a total of 1,470 patients with sepsis were included in 14 studies. True positive AKI was diagnosed in 496 cases, false positive in 180 cases, false negative in 119 cases and true negative in 675 cases (Figure 2A). For blood NGAL, a total of 1,358 patients with sepsis were included in 9 studies. True positive AKI was diagnosed in 283 cases, false positive in 381 cases, false negative in 86 cases and true negative in 608 cases (Figure 2B). For urinary interleukin-18, a total of 4 studies were searched and included, there were 84 cases of true positive, 41 cases of false positive, 32 cases of false negative and 99 cases of true negative (Figure 2C). For urinary KIM-1, a total of 3 studies were included in the search, there were 124 cases of true positive, 37 cases of false positive, 21 cases of false negative and 194 cases of true negative (Figure 2D), For urinary Netrin-1, a total of 2 studies were searched and included, there were 73 cases of true positive, 59 cases of false positive, 25 cases of false negative and 137 cases of true negative (Figure 2E). The sensitivity of urinary NGAL was 0.81 [0.77, 0.84], and the specificity was 0.79 [0.76, 0.82] (Figure 3A). The sensitivity of blood NGAL was 0.77 [0.72, 0.81], and the specificity was 0.61 [0.58, 0.65] (Figure 3B). The sensitivity of urinary interleukin-18 was 0.80 [0.77, 0.82], with a specificity of 0.70 [0.67, 0.72] (Figure 3C). The sensitivity of urinary KIM-1 was 0.86 [0.79, 0.91], with a specificity of 0.84 [0.79, 0.88] (Figure 3D). The sensitivity of urinary Netrin-1 was 0.74 [0.65, 0.83], with specificity of 0.70 [0.63, 0.76] (Figure 3E).
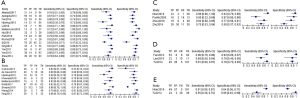
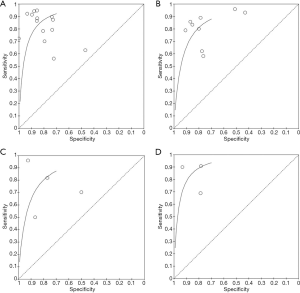
Risk of bias
The risk of bias and the adjustment factors among the studies are summarized in Figure 4. Patent selection, flow and timing of most articles had low risk of bias.
Sensitivity and false positive rate
According to the sensitivity and false positive rate, the sequence was urinary KIM-1 > urinary NGAL > urinary IL-18> blood NGAL (Figure 5).
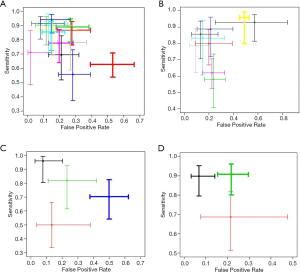
SROC and the diagnostic ratio (DOR) of urinary NGAL, blood NGAL, IL-18 and KIM-1
According to the sample size, the SROC values of urinary NGAL, blood NGAL, urinary IL-18 and urinary KIM-1 were 0.907, 0.857, 0.861 and 0.931, respectively (Figure 6). The sequence was urinary KIM-1 > urinary NGAL > blood NGAL > urinary IL-18. Without sample weights, the SROCs of urinary NGAL, blood NGAL, urinary IL-18 and urinary KIM-1 were 0.874, 0.853, 0.839 and 0.911, respectively (Figure 7). The SROC of urinary KIM-1 ranked first, followed by the other biomarkers: urinary KIM-1 > urinary NGAL > blood NGAL > urinary IL-18. The diagnostic odds ratios of urinary NGAL, blood NGAL, urinary IL-18 and urinary KIM-1 were 3.01 (2.26, 3.76), 2.70 (2.06, 3.35), 2.55 (0.83, 4.28) and 3.54 (2.15, 4.93), respectively (Figure 8). Due to the small number of urinary Netrin-1 studies, an SROC calculation could not be completed.
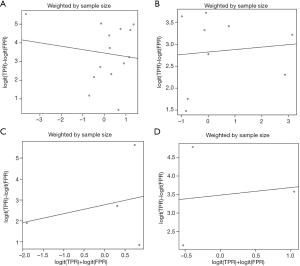
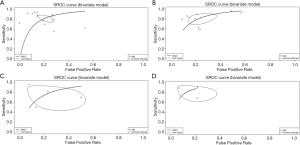
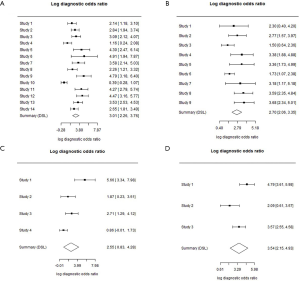
Discussion
In this meta-analysis, we summarized novel biomarkers associated with AKI diagnosis in high-risk groups (previously creatinine, urea nitrogen, cystatin C, etc.) and biomarkers associated with AKI mortality. Forty-nine studies involving 4,189 patients were identified by searching several databases and screening articles. We found significant increases in NGAL, urinary interleukin-18, KIM-1, and urinary Netrin-1 in the blood of patients with AKI. Although urinary sCD163, serum sex hormone levels and soluble thromboregulatory proteins are also significantly different between AKI patients and high-risk patients, the number of studies on these biomarkers is small, so the results cannot be meta-analyzed.
Neutrophil gelatinase-related lipid carrier protein (NGAL) is a member of the lipid carrier protein superfamily and is expressed by neutrophils and various epithelial cells (51). As a proximal tubular injury-related protein, NGAL is expressed in injured renal tubular epithelial cells and has a high effect size for the diagnosis of AKI (assessed by a combination odds ratio). NGAL in blood and urine samples is the most widely studied biomarker for the early prediction of AKI, and Zhang et al. (13) reported the value of the blood and urinary NGAL biomarker in the diagnosis and prognosis of sepsis-associated AKI. They report that NGAL not only is an effective predictor of AKI during sepsis but also has potential predictive value for RRT and mortality. However, this study did not distinguish the different diagnostic characteristics of urinary NGAL and blood NGAL. In our meta-study, it was found that the diagnostic value of urinary NGAL was slightly higher than that of blood NGAL. Haase-Fielitz et al. (52) also conducted a systematic evaluation, identifying 58 articles and including >16,500 patients with AKI, and found that both plasma and urinary NGAL could predict AKI and its severity, and the total AUC in different clinical environments was 0.79–0.87. However, this study involved a wide range of AKI patients, and a separate study is still needed for patients with sepsis, a special condition. Therefore, a summary of the results of our study shows that plasma and urinary NGAL have good diagnostic accuracy for sepsis-associated AKI (AUC is 0.86 and 0.90, respectively).
Kidney damage molecule-1 (KIM-1) is a type I transmembrane glycoprotein that has an immunoglobulin and mucin domain structure; KIM-1 is also a kind of proximal renal tubular injury-related protein that is expressed in renal tubular epithelial cells; it is not expressed in normal renal tissues or urine tests but is expressed at very high levels after ischemic or toxic damage in rodents and in the dedifferentiation of renal proximal tubule epithelial cells. AKI can be diagnosed early because urine excretion increases significantly 2 to 8 h after injury. It has been reported in the literature that urinary KIM-1 elevation has the highest specificity for the recognition of renal injury and can distinguish prerenal AKI from acute tubular necrosis (38). In addition, hyperuria and KIM-1 expression were prospectively assessed in a cohort of 201 hospitalized AKI patients and were associated with adverse clinical outcomes (death and need for dialysis) in AKI patients (53). In our study, 124 cases of true positive, 37 cases of false positive, 21 cases of false negative and 194 cases of true negative after Kim-1 diagnosis of AKI were found, with a sensitivity of 0.86 [0.79, 0.91] and a specificity of 0.84 [0.79, 0.88]. Our study found a ranking of urinary Kim-1 > urinary NGAL > blood NGAL > urinary IL-18 according to the SROC curve area. This indicates that urinary Kim-1 has high diagnostic value. However, as the sample size is still too small, further studies with a large sample size are needed in clinical practice.
IL-18 is a pro-inflammatory cytokine expressed conditionally in late inserted cells in the distal convoluted tubule, connecting tubules to collecting tubules in healthy human kidneys. In addition, these cells contain the three main components needed to release the active pro-inflammatory cytokine IL-18, namely, pro-IL-18, P2X7 and intracellular protease caspase-1 (54), which are converted to IL-18 in its active form and then leave the cell and enter the urine, for example, in AKI (55). In the first cross-sectional study of people with a variety of kidney diseases, urine levels of IL-18 were significantly higher and the diagnosis of ATN was highly sensitive and specific for groups with PA, UTI, chronic kidney disease, and normal kidney function compared with the healthy control group. IL-18 can be used as a marker of proximal renal tubular injury in ATN (44). Furthermore, urinary IL-18 was significantly upregulated and predicted mortality during mechanical ventilation before serum creatinine increased in patients with AKI acute respiratory distress syndrome (56). Early urine measurements of IL-18 were associated with AKI severity and mortality; however, in prospective analyses, IL-18 showed no ability to predict the subsequent development of AKI. Our study showed that the diagnosis of sepsis-associated AKI with urinary IL-18 was a true negative in 84 cases, false positive in 41 cases, false negative in 32 cases, and true negative in 99 cases, with a sensitivity of 0.72 [0.63, 0.80] and a specificity 0.71 [0.62, 0.78]. Considering that IL-18 is a pro-inflammatory cytokine that plays an important role in sepsis, IL-18 measurement may also be affected by many coexisting variables, such as endotoxemia, inflammation and autoimmune diseases; therefore, its sensitivity and specificity are affected.
Netrin-1 is a laminin-related neuronal guidance molecule that is not expressed or is barely expressed in the tubular epithelial cells of normal kidneys. At present, a large number of studies have shown that Netrin-1 is highly expressed in renal tubular epithelial cells under various pathological conditions of the kidney and plays an important role in protecting the kidney by inhibiting the inflammatory response, among other mechanisms. In addition, Netrin-1 can be detected in urine within a short period of time; therefore, it may play an early role in the prediction and diagnosis of acute renal injury. Through a urine test in patients with AKI, the level of Netrin-1 was found to change in 38 of the studies analyzed (9); compared with that in healthy people, the urinary Netrin-1 level in patients with AKI increased significantly, and the increase was most obvious in the sepsis group, showing that the early identification of urinary Netrin-1 levels had a certain clinical significance in AKI and may become a new diagnostic index of early AKI. In our study, the sensitivity and specificity of Netrin-1 after aggregation were shown to be 0.74 [0.65, 0.83] and 0.70 [0.63, 0.76], respectively. There were 73 cases of true positive, 59 cases of false positive, 25 cases of false negative and 137 cases of true negative. Due to the small amount of literature, the diagnostic value of Netrin-1 still needs to be further studied.
This systematic evaluation and meta-analysis may facilitate the further study of sepsis-associated AKI diagnosis in a variety of different ways. First, it established the diagnostic sequence of sepsis-associated AKI biomarkers, ranked as urinary KIM-1 > urinary NGAL > blood NGAL > urinary IL-18, according to the SROC curve area. Second, the diagnostic characteristics of a variety of biomarkers were demonstrated. The same biomarker has different manifestations in blood and urine, among which the diagnostic value of urinary NGAL is slightly higher than that of blood NGAL. Third, these biomarkers represent the pathophysiological process of sepsis-associated AKI. In our meta-analysis, the diagnosis of sepsis-associated AKI was associated with inflammation, endothelial dysfunction, coagulation and even hormones. Although some nonquantitative methods were not included in this meta-analysis, they are still worth exploring.
There are limitations to this meta-analysis. First, although subgroup analyses of different biomarkers diagnosed with AKI were performed, heterogeneity could not be explained for each biomarker. Various types of controls were used in the study, and study inclusion was associated with biomarker measures and different treatments of AKI. Due to the limited information, we cannot carry out further analysis. Second, each biomarker included a limited number of studies, which hinders the reproducibility of the results. The number of studies for each biomarker should be considered when evaluating the effectiveness of the ranking system. Third, only studies involving two or more biomarkers were included in this meta-analysis. Therefore, not considering promising biomarkers assessed in a single study may limit the study of whole-blood or urine biomarkers.
Conclusions
This systematic evaluation and meta-analysis outlined the diagnostic characteristics of sepsis-associated AKI blood and urine biomarkers. According to the SROC curve area, the diagnostic sequence of sepsis-associated AKI biomarkers was urinary KIM-1 > urinary NGAL > blood NGAL > urinary IL-18. This sequence, provided by evaluating the effect size of sepsis-associated biomarkers in AKI diagnosis, can be used as a reference for the further study of AKI biomarkers and clinical diagnosis.
Acknowledgments
We thank all the staff for their valuable contribution to the study.
Funding: This project was supported by grants from the Songjiang District Science and Technology Project (No. 19SJKJGG92), the 3rd Sansheng TCP Young and Middle-aged Scientific Research Funding, Shanghai science and technology committee scientific and technological support project (No. 18411950600 and No. 18411950602), Clinical Research Innovation Plan of Shanghai General Hospital (CTCCR-2016B01) and Wu jieping medical foundation (No. 320.6750.18546).
Footnote
Reporting Checklist: The authors have completed the PRISMA reporting checklist. Available at http://dx.doi.org/10.21037/apm-20-1855
Conflicts of Interest: All authors have completed the ICMJE uniform disclosure form (available at http://dx.doi.org/10.21037/apm-20-1855). The authors have no conflicts of interest to declare.
Ethical Statement: The authors are accountable for all aspects of the work in ensuring that questions related to the accuracy or integrity of any part of the work are appropriately investigated and resolved.
Open Access Statement: This is an Open Access article distributed in accordance with the Creative Commons Attribution-NonCommercial-NoDerivs 4.0 International License (CC BY-NC-ND 4.0), which permits the non-commercial replication and distribution of the article with the strict proviso that no changes or edits are made and the original work is properly cited (including links to both the formal publication through the relevant DOI and the license). See: https://creativecommons.org/licenses/by-nc-nd/4.0/.
References
- Martin GS, Mannino DM, Eaton S, et al. The epidemiology of sepsis in the United States from 1979 through 2000. N Engl J Med 2003;348:1546-54. [Crossref] [PubMed]
- Gotts JE, Matthay MA. Sepsis: pathophysiology and clinical management. BMJ 2016;353:i1585. [Crossref] [PubMed]
- Uchino S, Kellum JA, Bellomo R, et al. Beginning and Ending Supportive Therapy for the Kidney (BEST Kidney) Investigators. Acute renal failure in critically ill patients: a multinational, multicenter study. JAMA 2005;294:813-8. [Crossref] [PubMed]
- Bagshaw SM, Lapinsky S, Dial SCooperative Antimicrobial Therapy of Septic Shock (CATSS) Database Research Group, et al. Acute kidney injury in septic shock: clinical outcomes and impact of duration of hypotension prior to initiation of antimicrobial therapy. Intensive Care Med 2009;35:871-81. [Crossref] [PubMed]
- Yegenaga I, Hoste E, Van Biesen W, et al. Clinical characteristics of patients developing ARF due to sepsis/systemic inflammatory response syndrome: results of a prospective study. Am J Kidney Dis 2004;43:817-24. [Crossref] [PubMed]
- Bagshaw SM, Uchino S, Bellomo R, et al. Beginning and Ending Supportive Therapy for the Kidney (BEST Kidney) Investigators. Septic acute kidney injury in critically ill patients: clinical characteristics and outcomes. Clin J Am Soc Nephrol 2007;2:431-9. [Crossref] [PubMed]
- Mehta RL, Bouchard J, Soroko SBProgram to Improve Care in Acute Renal Disease (PICARD) Study Group, et al. Sepsis as a cause and consequence of acute kidney injury: Program to Improve Care in Acute Renal Disease. Intensive Care Med 2011;37:241-8. [Crossref] [PubMed]
- Perner A, Cecconi M, Cronhjort M, et al. Expert statement for the management of hypovolemia in sepsis. Intensive Care Med 2018;44:791-8. [Crossref] [PubMed]
- Morrell ED, Kellum JA, Pastor-Soler NM, et al. Septic acute kidney injury: molecular mechanisms and the importance of stratification and targeting therapy. Crit Care 2014;18:501. [Crossref] [PubMed]
- Mehta RL, Kellum JA, Shah SV, et al. Acute Kidney Injury Network. Acute Kidney Injury Network: report of an initiative to improve outcomes in acute kidney injury. Crit Care 2007;11:R31. [Crossref] [PubMed]
- Bellomo R, Ronco C, Kellum JA, et al. Acute Dialysis Quality Initiative workgroup. Acute renal failure - definition, outcome measures, animal models, fluid therapy and information technology needs: the Second International Consensus Conference of the Acute Dialysis Quality Initiative (ADQI) Group. Crit Care 2004;8:R204-12. [Crossref] [PubMed]
- KDIGO. Kidney Disease: Improving Global Outcomes (KDIGO) Acute Kidney Injury Work Group. KDIGO Clinical Practice Guideline for Acute Kidney Injury. Kidney Int Suppl 2012;2:1-138.
- Zhang A, Cai Y, Wang PF, et al. Diagnosis and prognosis of neutrophil gelatinase-associated lipocalin for acute kidney injury with sepsis: a systematic review and meta-analysis. Critical Care 2016;20:41. [Crossref] [PubMed]
- Poston J T, Koyner JL. Sepsis associated acute kidney injury. BMJ 2019;364:k4891. [Crossref] [PubMed]
- Whiting PF, Rutjes AW, Westwood ME, et al. QUADAS-2: a revised tool for the quality assessment of diagnostic accuracy studies. Ann Intern Med 2011;155:529-36. [Crossref] [PubMed]
- Albeladi FI, Algethamy H. Urinary Neutrophil Gelatinase-Associated Lipocalin as a Predictor of Acute Kidney Injury, Severe Kidney Injury, and the Need for Renal Replacement Therapy in the Intensive Care Unit. Nephron Extra 2017;7:62-77. [Crossref] [PubMed]
- Khawaja S, Jafri L, Siddiqui I, et al. The utility of neutrophil gelatinase-associated Lipocalin (NGAL) as a marker of acute kidney injury (AKI) in critically ill patients. Biomark Res 2019;7:4. [Crossref] [PubMed]
- Park HS, Kim JW, Lee KR, et al. Urinary neutrophil gelatinase-associated lipocalin as a biomarker of acute kidney injury in sepsis patients in the emergency department. Clin Chim Acta 2019;495:552-5. [Crossref] [PubMed]
- Md Ralib A, Mat Nor MB, Pickering JW. Plasma Neutrophil Gelatinase Associated Lipocalin Diagnosed Acute Kidney Injury in Patients With Systemic Inflammatory Disease And Sepsis. Nephrology (Carlton) 2017;22:412-9. [Crossref] [PubMed]
- da Rocha EP, Gabriela YL, Motta SB, et al. Urinary Neutrophil Gelatinase-Associated Lipocalin Is Excellent Predictor of Acute Kidney Injury in Septic Elderly Patients. Aging and Disease 2018;9:182-91. [Crossref] [PubMed]
- Aydogdu M, Gursel G, Sancak B, et al. The use of plasma and urine neutrophil gelatinase associated lipocalin (NGAL) and Cystatin C in early diagnosis of septic acute kidney injury in critically ill patients. Dis Markers 2013;34:237-46. [Crossref] [PubMed]
- Li PZ, Xu WX. Prediction of acute kidney injury complicated by sepsis with neutronphil gelatinase-associated lipocalin as an early marker. Chin J Lab Med 2010;33:492-6.
- Niu KY, Yang F, Yang XY, et al. Diagnostic value of urinary livertype fatty acid binding proteins and urinary neutrophil gelatinase-associated lipocalin in severe sepsis patients with acute kidney injury. Clin Focus 2015;5:536-9.
- Shapiro NI, Trzeciak S, Hollander JE, et al. The diagnostic accuracy of plasma neutrophil gelatinase-associated lipocalin in the prediction of acute kidney injury in emergency department patients with suspected sepsis. Ann Emerg Med 2010;56:52-9.e1. [Crossref] [PubMed]
- Wang HX, Mu HB, Zheng RQ, et al. Neutrophil gelatinaseassociated lipocalin for the early diagnosis of sepsis in patients with acute kidney injury. J Clin Med Pract 2014;18:183-4.
- Xing HB, Lv T, Sheng PP, et al. The diagnostic value of new biomarkers in sepsis patients with acute kidney injury. Chin J Crit Care Med 2013;33:507-10.
- Yan J, Xu HY, Zang D, et al. Significance of early diagnosis of urinary neutrophil gelatinase-associated lipocalin and urinary interleukin-18 in patients with sepsis complicated with acute kidney injury. Suzhou Univ J Med Sci 2011;31:785-8.
- Yan J, Zang ZD. Prediction of acute kidney injury complicated by sepsis with sTREM-1 and NGAL as early marker. Chin J Nephrol 2013;29:423-8.
- Hjortrup PB, Haase N, Treschow F, et al. Predictive value of NGAL for use of renal replacement therapy in patients with severe sepsis. Acta Anaesthesiol Scand 2015;59:25-34. [Crossref] [PubMed]
- Fan H, Zhao Y, Zhu JH, et al. Urine neutrophil gelatinase-associated lipocalin in septic patients with and without acute kidney injury. Ren Fail 2014;36:1399-403. [Crossref] [PubMed]
- Camou F, Oger S, Paroissin C, et al. Plasma neutrophil gelatinase-associated lipocalin (NGAL) predicts acute kidney injury in septic shock at ICU admission. Ann Fr Anesth Reanim 2013;32:157-64. [Crossref] [PubMed]
- Nga HS, Medeiros P, Menezes P, et al. Sepsis and AKI in clinical emergency room patients: the role of urinary NGAL. Biomed Res Int 2015;2015:413751 [PubMed]
- Mårtensson J, Bell M, Oldner A, et al. Neutrophil gelatinase-associated lipocalin in adult septic patients with and without acute kidney injury. Intensive Care Med 2010;36:1333-40. [Crossref] [PubMed]
- de Geus HR, Betjes MG, Schaick R, et al. Plasma NGAL similarly predicts acute kidney injury in sepsis and nonsepsis. Biomark Med 2013;7:415-21. [Crossref] [PubMed]
- Zhou HQ, Chen MQ, Zhang HD, et al. sTREM-1 and NGAL levels for the early diagnosis of sepsis complicated by acute kidney injury. J Clin Exp Med 2014;21:1773-5.
- Fan H, Zhao Y, Sun M, et al. Urinary neutrophil gelatinase-associated lipocalin, kidney injury molecule-1, N-acetyl-β-D-glucosaminidase levels and mortality risk in septic patients with acute kidney injury. Arch Med Sci 2018;14:1381-6. [Crossref] [PubMed]
- Lee CW, Kou HW, Chou HS, et al. A combination of SOFA score and biomarkers gives a better prediction of septic AKI and in-hospital mortality in critically ill surgical patients: a pilot study. World J Emerg Surg 2018;13:41. [Crossref] [PubMed]
- Zhu L, Shi D. Early diagnostic value of neutrophil gelatinase-associated lipocalin and interleukin-18 in patients with sepsis-induced acute kidney injury. Zhonghua Wei Zhong Bing Ji Jiu Yi Xue 2016;28:718. [PubMed]
- Maddens B, Ghesquiere B, Vanholder R, et al. Chitinase-like Proteins are Candidate Biomarkers for Sepsis-induced Acute Kidney Injury. Mol Cell Proteomics 2012;11:M111.013094-M111.013094.
- Su L, Feng L, Liu C, et al. Diagnostic value of urine sCD163 levels for sepsis and relevant acute kidney injury: a prospective study. BMC Nephrol 2012;13:123. [Crossref] [PubMed]
- Katayama S, Nunomiya S, Koyama K, et al. Markers of acute kidney injury in patients with sepsis: the role of soluble thrombomodulin. Crit Care 2017;21:229. [Crossref] [PubMed]
- Bouchard J, Malhotra R, Shah S, et al. Levels of Protein C and Soluble Thrombomodulin in Critically Ill Patients with Acute Kidney Injury: A Multicenter Prospective Observational Study. PLoS One 2015;10:e0120770 [Crossref] [PubMed]
- Guo Juntien, Liu Qi, Xiao Dong. Study on the value of soluble thromboregulatory protein in the prediction of acute renal injury in sepsis. Chinese Emergency Medicine 2018:590-5.
- Tu Y, Wang H, Sun R, et al. Urinary netrin-1 and KIM-1 as early biomarkers for septic acute kidney injury. Renal Failure 2014;36:1559-63. [Crossref] [PubMed]
- Parikh CR, Abraham E, Ancukiewicz M, et al. Urine IL-18 Is an Early Diagnostic Marker for Acute Kidney Injury and Predicts Mortality in the Intensive Care Unit. J Am Soc Nephrol 2005;16:3046-52. [Crossref] [PubMed]
- Siew ED, Ikizler TA, Gebretsadik T, et al. Elevated Urinary IL-18 Levels at the Time of ICU Admission Predict Adverse Clinical Outcomes. Clin J Am Soc Nephrol 2010;5:1497-505. [Crossref] [PubMed]
- Nian Y, Hu J. Clinical value of urine netrin-1 and il-18 in early diagnosis of acute renal injury in sepsis. Heilongjiang Medical Science 2018;3.
- Ming-Ming Z, Zheng-Ying J, Li R. Value of Combined Detection of Cystatin C and IL-18 in the Early Prediction of Sepsis Patients Complicated With Acute Renal Injury. Chinese General Practice 2016.
- Schaalan M, Mohamed W. Predictive ability of circulating osteoprotegerin as a novel biomarker for early detection of acute kidney injury induced by sepsis. Eur Cytokine Netw 2017;28:52-62. [Crossref] [PubMed]
- Feng JY, Liu KT, Abraham E, et al. Serum Estradiol Levels Predict Survival and Acute Kidney Injury in Patients with Septic Shock- A Prospective Study. PLoS One 2014;9:e97967 [Crossref] [PubMed]
- Devarajan P. Neutrophil gelatinase-associated lipocalin—an emerging troponin for kidney injury. Nephrol Dial Transplant 2008;23:3737-43. [Crossref] [PubMed]
- Haase-Fielitz A, Haase M, Devarajan P. Neutrophil gelatinase-associated lipocalin as a biomarker of acute kidney injury: a critical evaluation of current status. Ann Clin Biochem 2014;51:335-51. [Crossref] [PubMed]
- Liangos O, Perianayagam MC, Vaidya VS, et al. Urinary N-Acetyl- beta -(D)-Glucosaminidase Activity and Kidney Injury Molecule-1 Level Are Associated with Adverse Outcomes in Acute Renal Failure. J Am Soc Nephrol 2007;18:904-12. [Crossref] [PubMed]
- Gauer S, Sichler O, Obermüller N, et al. IL-18 is expressed in the intercalated cell of human kidney. Kidney Int 2007;72:1081-7. [Crossref] [PubMed]
- Melnikov VY, Ecder T, Fantuzzi G, et al. Impaired IL-18 processing protects caspase-1-deficient mice from ischemic acute renal failure. J Clin Invest 2001;107:1145-52. [Crossref] [PubMed]
- Parikh CR, Abraham E, Ancukiewicz M, et al. Urine IL-18 is an early diagnostic marker for acute kidney injury and predicts mortality in the intensive care unit. J Am Soc Nephrol 2005;16:3046-52. [Crossref] [PubMed]