Investigation into molecular mechanisms and high-frequency core TCM for pulmonary fibrosis secondary to COVID-19 based on network pharmacology and data mining
Introduction
The spread of novel coronavirus pneumonia 2019 (COVID-19) has been declared as a global pandemic by the World Health Organization (WHO) (1). Almost 2.5 million cases of COVID-19 and more than 160,000 deaths till April 22, 2020, have been reported to WHO (2).
Similar to patients with SARS-CoV or MERS-CoV, pulmonary fibrosis (PF) secondary to COVID-19 raised a great attention (3). Evidence showed that some patients in the early stage of COVID-19 showed cellular fibromyxoid exudates and patchy inflammatory cellular infiltration in chest computed tomography images, which indicated that patients had the risk of PF (4,5); Some patients in intensive care unavoidably developed PF owing to the severe acute respiratory distress syndrome (ARDS) [12 (29%)] (6,7); Even many patients survived the acute phase of the illness, a substantial proportion of which died as a result of progressive PF (8). Although long-term follow-up studies will be required to establish the true prevalence of PF secondary to COVID-19. Given the huge number of individuals infected by COVID-19, the prevalence of PF secondary to COVID-19 will have a significant impact on health.
However, the molecular mechanisms underlying the development of PF secondary to COVID-19 are not well elaborated. Only a review study indicated the initiation and promotion of PF secondary to COVID-19 was related to abnormal immune mechanisms or an excessive cytokine storm (9). Except from the active anti-virus strategy against COVID-19, most studies still mainly focus on targeting cytokine storms or viral macro-and micro-vascular thromboembolism in the early stage of COVID-19 to prevent the development of PF (10,11). Thus antifibrotic therapy targeting PF secondary to COVID-19 is poorly defined. It should be noted, whether a novel antifibrotic drug would be beneficial in the context of COVID-19 related illness was brought to discuss by Peter M George: First the effect of antifibrotic compounds on viral eradication, and second, their effect on mitigating the cytokine storm that seems to be responsible for complications in severe COVID-19 (12).
The Traditional Chinese medicine (TCM) with multiple components and multiple targets has been highly recommended by the National Health Commission of the People's Republic of China as a therapeutic strategy for COVID-19 (13), for their effects on indirectly anti-SARS-CoV-2 and modulating cytokine storms (14,15). Interestingly, the effect of TCM on PF secondary to COVID-19 has also been studied, a research tried to demonstrate the efficacy of TCM against the PF, but only targeting fibrosis-related targets VEGFR or FGFR (16).
Recently, a new analytical method application in TCM, network pharmacology, was well demonstrated for elucidating the mechanisms of complex diseases, analyzing the interaction network of multiple factors such as protein target and genes, especially for discovering new therapeutic drugs (17,18). Therefore, this study aimed to systematically identify (I) molecular mechanisms of PF secondary to COVID-19 through COVID-19 differentially expressed gene (DEG) identification, “Candidate targets associated with PF secondary to COVID-19-Proteins interaction” network analysis, and enrichment pathway analysis; and (II) high-frequency core TCM targeting PF secondary to COVID-19 by data mining, “Key targets related to PF secondary to COVID-19-Ingredients” and “Key ingredients -Herbs” network analysis, and last validation through molecular docking the key ingredients to key targets related to PF secondary to COVID-19.
We presented the following article in accordance with the MDAR checklist (available at http://dx.doi.org/10.21037/apm-20-1384).
Methods
The study was conducted in accordance with the Declaration of Helsinki (as revised in 2013).
DEGs of COVID-19 selection
The DEGs in lung epithelial cells and primary human bronchial epithelial cells during SARS-CoV-2 infection were extracted from microarray data GSE147507 in the Gene Expression Omnibus (http://www.ncbi.nlm.nih.gov/geo/) database with a cutoff value of P<0.05 and |Log2FoldChange| ≥1 (19,20).
Known targets associated with PF screening
PF-associated known targets were obtained from the following databases after removing the duplicates: DisGeNET, Genecards, and Therapeutic Target Database (TTD). The species was all set to “Homo sapiens.”
DisGeNET is the largest public platform containing more than 380,000 associations between >16,000 genes and 13,000 diseases. It integrates data from expert-curated repositories, scientific literature, and genome-wide association study catalogs (21). The search strategy was as follows: Set the disease name as “pulmonary fibrosis” and then download file “all gene–disease associations” from DisGeNET.
Genecards (version 4.14) is an extensive platform that provides more than 7,000 human genes gathered from 150 web resources (22). The search strategy was as follows: Set the keyword as “pulmonary fibrosis,” download the file, and select the targets with a score >6.18 (two fold average score of all target genes) for the study.
The TTD database provides information about the known and explored therapeutic proteins and targets, the targeted disease, pathway information, and corresponding drugs directed at each of these targets; all information provided is fully referenced (23). The search strategy was as follows: Set the keyword as “pulmonary fibrosis” at “Search Drugs and Targets by Disease or ICD Identifier” and download the file.
Identification of candidate targets associated with PF secondary to COVID-19 and protein-protein interaction (PPI)
Candidate targets associated with PF secondary to COVID-19 were identified by mapping the DEGs of COVID-19 and known targets associated with PF. Then, the three-dimensional structure of the candidate targets was retrieved and downloaded from the RCSB PDB database (http://www.rcsb.org/) in the “PDB” file format (24).
The proteins interacting with these candidate targets were obtained from the STRING database, which defined known and predicted interactions of proteins with four categories of confidence scores: highest (0.9), high (0.7), medium (0.4), and low (0.1) (25). Based on these, the score ≥0.7 was set to identify PPI of these candidate targets (26).
Construction and analysis of PPI network of candidate targets
The PPI network of candidate targets was constructed by importing the data into the Cytoscape 3.61 software, which helped in data integration and network visualization (27). Then, parameters, such as the degree in the network, were calculated using its plugin NetworkAnalyzer. The higher the degree value of the node (candidate target/protein), the more important the role in the network (28). Thus, nodes with the highest degree value were identified as the key targets of PF secondary to COVID-19.
Enrichment analysis for candidate targets associated with PF secondary to COVID-19
The online Gene Ontology (GO) enrichment analysis was performed by inputting all the candidate targets associated with PF secondary to COVID-19 into the g:Profiler server (https://biit.cs.ut.ee/gprofiler/gost), setting the organism as “Homo sapiens” (29). The GO enrichment analysis can classify gene functions into three aspects: molecular functions, biological processes, and cellular components.
The Kyoto Encyclopedia of Genes and Genomes (KEGG) pathway enrichment analysis was performed by inputting all the candidate targets into the DAVID server (https://david.ncifcrf.gov/, v6.8), setting the organism as “Homo sapiens.” The KEGG analysis can explore the biological pathways or functions of the targets submitted (30). The pathways or functions with a higher enrichment score and a smaller P value were recommended as the main mechanisms of PF secondary to COVID-19.
Data mining of high-frequency core TCM acting on the key targets related to PF secondary to COVID-19
Predicted ingredients from data extraction
The predictive ingredients acting on the key targets related to PF secondary to COVID-19 were obtained from the TCM Systems Pharmacology Database (TCMSP, http://tcmspw.com/tcmsp.php). TCMSP is a systematic pharmacology platform designed for herbs, targets, and chemicals with ADME-related properties (absorption, distribution, metabolism, and excretion) (31). The search strategy was as follows: Set the keyword as “the key target” at the “target name” module and then download each predicted ingredient in the “mol2” file format with oral bioavailability (OB) ≥20% and drug-likeness (DL) ≥0.10 (26). Then a “Key targets related to PF secondary to COVID-19 - Predicted ingredients” network was built using Cytoscape 3.61 software.
Key ingredients identification
The frequency of these predicted ingredients was analyzed by searching the recorded in PubMed database (https://www.ncbi.nlm.nih.gov/pubmed) using the keywords “(the predicted ingredients[Title]) AND Pulmonary Fibrosis[Title]” (28). Hence, the key ingredients were identified according to their frequency sorted from high to low.
Core herb identification
The herbs containing these key ingredients were also obtained from the TCMSP database using the following search strategy: Set the keyword as “the key ingredients” in the “chemical name” module and download. The core herb for treating PF secondary to COVID-19 was identified based on the largest cumulative ingredients frequency recorded in PubMed. Then, a “key ingredients-Herbs” network was built using Cytoscape 3.61 software.
Molecular docking validation the interaction between key ingredients and key targets related to PF secondary to COVID-19
The binding affinities of the ligand (the key ingredient) and the receptor (the key target) were predicted by computer simulation and calculation. The web server, SwissDock (http://www.swissdock.ch/), is dedicated to carrying out receptor-ligand docking simulation intuitively based on the protein-ligand docking program EADock DSS (32). SwissDock was used in this study for molecular docking analysis to validate the interaction between the key ingredients and the key targets by uploading a ligand structure as “mol2” file and a receptor structure as “PDB” file; the binding affinity results were returned via email.
Statistical analysis
The data were analyzed with R Package and Cytoscape 3.61 software. P<0.05 was considered as a statistically significant value.
The flowchart of the network pharmacology approach used in this study
The flowchart of the network pharmacology approach used in this study is presented in Figure 1.
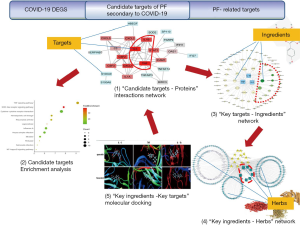
Results
190 DEGs of COVID-19 identified
A total of 190 DEGs of COVID-19 were identified (Table S1), including 116 in lung epithelial cells and 74 in primary human bronchial epithelial cells. All these DEGs were found to be related mainly to inflammatory cytokines, such as interleukin 6 (IL-6), IL-1β, and tumor necrosis factor (TNF). The results are shown in Figure 2.
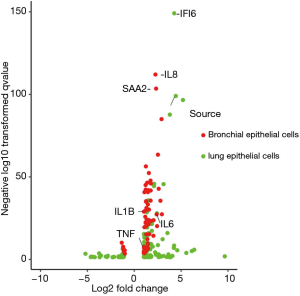
1,561 PF-associated known targets screened
A total of 432 PF–associated known targets were obtained from the DisGeNET database, 1,375 targets from the Genecards database, and 5 targets from the TTD database. Removing 249 duplicates, 1,561 PF-associated known targets were obtained (available online: https://cdn.amegroups.cn/static/public/APM-20-1384-1.pdf).
29 candidate targets associated with PF secondary to COVID-19 and key targets of IL-6, TNF, and IL-1β identified
A total of 29 candidate targets associated with PF secondary to COVID-19 were identified by mapping the COVID-19-related DEGs and PF-associated targets, including inflammatory cytokines IL-6, IL-1β, and TNF.
Figure 3 shows the PPI network of candidate targets, which includes 25 nodes and 65 edges. The nodes of IL-6, TNF, and IL-1β were identified as the three key targets of PF secondary to COVID-19 due to their highest degree value calculated using the Network Analyzer plugin. Table 1 presents detailed information.
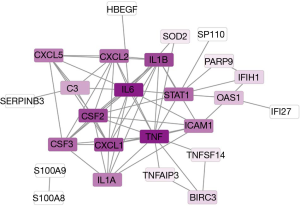
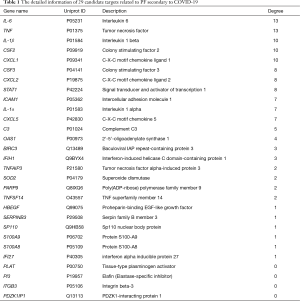
Full table
Results of GO and KEGG enrichment analysis for candidate targets
The molecular mechanisms of PF secondary to COVID-19 were identified through GO enrichment (molecular functions, biological processes, and cellular components) and KEGG pathway analyses.
Figure 4 shows the results of GO enrichment analysis. The top seven GO molecular function results were most related to cytokine or receptor activity, such as cytokine receptor binding, cytokine activity, and signaling receptor binding. The top seven GO biological process results were mostly related to the response to a stimulus, including response to an external stimulus, abiotic stimulus, or an immune response.
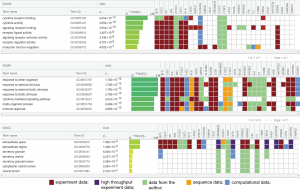
Figure 5 presents the results of the KEGG pathway enrichment analysis. The KEGG pathway of candidate targets is most enriched in the TNF signaling pathway, NOD-like receptor signaling pathway, cytokine-cytokine receptor interaction pathway, and NF-κB signaling pathway.
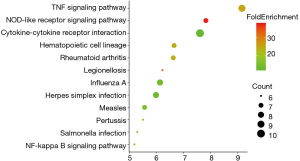
High-frequency TCM targeting treatment for PF secondary to COVID-19
13 key ingredients. A total of 76 predicted ingredients were obtained. Figure 6 shows the “The key targets-predicted ingredients” network. Ten predicted ingredients were found to act on all three key targets (IL-6, TNF, and IL-1β). Also, 24 predicted ingredients acted on two of these key targets. Further, 13 key ingredients were identified with OB ≥20% and DL ≥0.10. They were quercetin, epigallocatechin-3-gallate, emodin, triptolide, resveratrol, paeoniflorin, ginsenoside, astilbin, matrine, puerarin, kaempferol, apigenin, and tectorigenin, according to the frequency recorded in PubMed from 7 to 1. Table 2 provides detailed information on these 13 key ingredients.
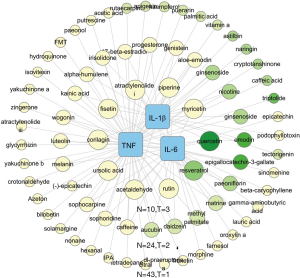
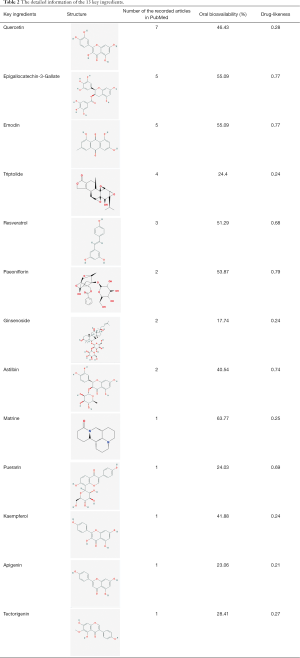
Full table
Polygonum cuspidatum as a core herb. A total of 258 herbs containing these key ingredients were acquired (available online: https://cdn.amegroups.cn/static/public/APM-20-1384-2.pdf). The “Key ingredients–Herbs” network was constructed using the Cytoscape 3.61 software (Figure 7). Nine herbs were found to contain four key ingredients, of which Polygonum cuspidatum was defined as the core herb with therapeutic effects on PF secondary to COVID-19 because it contained the key ingredients with the largest frequency recorded in the PubMed database.
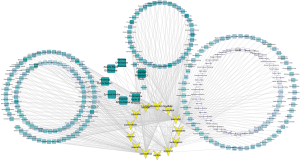
Molecular docking validation
The results on molecular docking showed that the key ingredients of quercetin and resveratrol were well docked to the key targets of PF secondary to COVID-19 (IL-6, TNF, and IL-1β), having several interactions with amino acid residues (Figure 8), and their docking fullFitness energy ranged from ‒2,666.9993 to ‒1,510.0823 (kcal/mol), their docking estimated ΔG ranged from ‒7.798237 to ‒6.6433935 (kcal/mol). The docking affinity information are shown in Table 3.
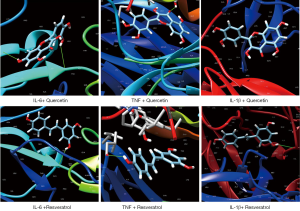
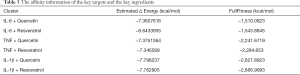
Full table
Discussion
The SARS-CoV2 has caused the epidemic of COVID-19, threatening millions worldwide (33). The PF secondary to COVID-19 is likely to increase rapidly in accompany with the global prevalence of COVID-19. Furthermore, a positive correlation was found between the duration of the illness caused by SARS-CoV and the degree of interstitial fibrosis (34). Hence, the molecular mechanisms of PF secondary to COVID-19 and novel therapeutic strategies targeting this disease critically need to be explored.
The results of this study showed that the molecular mechanisms of PF secondary to COVID-19 were related mainly to the cytokine or receptor activity and inflammatory pathways, including the TNF signaling pathway and the NF-κB signaling pathway. Further, IL-6, TNF, and IL-1β were identified as the key targets related to PF secondary to COVID-19. The high-frequency core TCM targeting these key targets (IL-6, TNF, IL-1β) included the ingredients of quercetin, epigallocatechin-3-gallate, emodin, triptolide, resveratrol, and the herb of Polygonum cuspidatum. Of which quercetin and resveratrol were well validated by their docking to IL-6, TNF, and IL-1β protein respectively.
In fact, the alterations in cytokine expression and function in animals and patients were regarded as the mechanisms of PF over the past 40 years (35,36). Most of the findings described a propensity for a variety of cytokines (including IL-6, TNF, and IL-1β) were highly associated with the fibrosis (37,38). Pro-inflammatory cytokine IL-6 has been known to play an important role in lung repair responses following viral or chemical insults (39). A previous study demonstrated that the blockade of IL-6 trans-signaling attenuated PF (40). TNF-α, another cytokine with inflammatory and fibrotic properties, stimulated collagen synthesis and promoted the proliferation of fibroblasts, contributing to the development of PF (41). The blocking of TNF-α promoted the attenuation of fibrosis in rodents (42). Evidence also showed that IL-1β stimulated fibroblast proliferation and chemotaxis, thereby inducing PF and inflammation (43).
Interestingly, the serum levels of IL-6 in 29 COVID-19 patients were showed positively correlated with the severity of COVID-19 according to an analysis (44). Studies demonstrated IL-6 played an important role in cytokine release syndrome (also known as cytokine storm) (45), which was an important reason leading to the death of patients with severe COVID-19. Chi Zhang believed that the blocking of the signal transduction pathway of IL-6 might serve as a new method for treating the cytokine storm, rescuing patients with COVID-19 (42). Especially, the levels of IL-6, TNF, and IL-1β were found to notably increase in patients with COVID-19 (46). Thus, IL-6, TNF, and IL-1β might serve as new molecular markers and a therapeutic strategy targeting PF secondary to COVID-19 and COVID-19.
Given the important role of cytokine IL-6, TNF, and/or IL-1β as a promising therapeutic strategy against PF secondary to COVID-19, the high-frequency core TCM targeting these targets were identified, including quercetin, epigallocatechin-3-gallate, emodin, triptolide, and resveratrol. In fact, it has been confirmed that quercetin strikingly attenuated bleomycin-induced PF in vivo by reducing the levels of IL-6, TNF-α, and IL-1β in the bronchoalveolar lavage fluid (47,48). Especially, it abolished the resistance to apoptosis caused by TNF-related apoptosis-inducing ligand in idiopathic pulmonary fibrosis (49). These findings strikingly supported the molecular docking results of our study that quercetin well docked to IL-6, TNF, and IL-1β, which were identified as the key targets of PF secondary to COVID-19.
Another high-frequency core TCM, epigallocatechin-3-gallate, also decreased the levels of IL-6, TNF-α, IL-1β, and NF-κB to modulate PF (50,51). Similar antifibrotic effects of high-frequency core TCM emodin, a major active component of P. cuspidatum, have also been reported (52,53). These key ingredients all shared antifibrotic effects by decreasing the levels of IL-6, TNF-α, IL-1β, and NF-κB, further supporting the cytokine activity and inflammatory pathways, molecular mechanisms of PF secondary to COVID-19 identified in our study.
P. cuspidatum, containing four key ingredients, was considered as the core herb with a therapeutic effect against PF secondary to COVID-19 in this study. Also, the antifibrotic effect of P. cuspidatum on the progression of PF in rats has been revealed (54). Especially, the major active component of P. cuspidatum, emodin, has been reported to decrease the levels of IL-6, TNF-α, IL-1β, and NF-κB, as discussed the above (52,53), directly supporting the findings on molecular mechanisms of PF secondary to COVID-19.
Besides, the molecular docking results of this study validated the interaction between the key ingredient resveratrol and key targets IL-6, TNF-α, and IL-1β in PF secondary to COVID-19. Resveratrol was demonstrated to inhibit PF by regulating miR-21 through MAPK/AP-1 pathways (55) and suppressing oxidative stress or transforming growth factor-β1 signaling (56). However, whether resveratrol has effects on anti-PF by reducing the levels of IL-6, TNF-α, or IL-1β needs further investigation.
Although, network pharmacology and data mining have been become an emerging discipline in the research of TCM. However, they are still in the early stage of development and exist some limitations (14). For example, network pharmacology uses computer network screening to achieve target selection, and some results do not have enough experimental data support (57). Also, There are still some objective conditions that the known compound targets do not fully correspond to the complex mechanisms of TCM formulae (58). In the same way, more corresponding experiments are still needed to support our results further.
Conclusions
This study systematically showed that the molecular pathways of PF secondary to COVID-19 were mainly related to the TNF signaling pathway, NOD-like receptor signaling pathway, cytokine or receptor activity, and NF-κB signaling pathway. Of these, reduced levels of IL-6, TNF, and IL-1β were identified as the targets for PF secondary to COVID-19 therapy. The use of high-frequency core TCM, including quercetin, epigallocatechin-3-gallate, emodin, triptolide, resveratrol, and herb P. cuspidatum, might serve as a therapeutic TCM strategy against PF secondary to COVID-19. On the basis of antiviral treatment, this study provided new ideas for the discovery of small molecular compounds with potential therapeutic effects on novel COVID-19.
Acknowledgments
Funding: This research were supported by the key scientific research projects of the Science and Technology Commission of Shanghai (No.17401901100) and Science and Technology Innovation Project of Traditional Chinese Medicine of Shanghai Municipal Commission of Health (No.ZYKC201701007).
Footnote
Reporting Checklist: The authors have completed the MDAR checklist. Available at http://dx.doi.org/10.21037/apm-20-1384
Conflicts of Interest: All authors have completed the ICMJE uniform disclosure form (available at http://dx.doi.org/10.21037/apm-20-1384). The authors have no conflicts of interest to declare.
Ethical Statement: The authors are accountable for all aspects of the work in ensuring that questions related to the accuracy or integrity of any part of the work are appropriately investigated and resolved. The authors declare that the study conformed to the provisions of the Declaration of Helsinki (as revised in 2013).
Open Access Statement: This is an Open Access article distributed in accordance with the Creative Commons Attribution-NonCommercial-NoDerivs 4.0 International License (CC BY-NC-ND 4.0), which permits the non-commercial replication and distribution of the article with the strict proviso that no changes or edits are made and the original work is properly cited (including links to both the formal publication through the relevant DOI and the license). See: https://creativecommons.org/licenses/by-nc-nd/4.0/.
References
- Cucinotta D, Vanelli M. WHO Declares COVID-19 a Pandemic. Acta bio-medica Acta Biomed 2020;91:157-60. [PubMed]
- WHO Director-General's opening remarks at the media briefing on COVID-19 - 22 April 2020. Available online: https://www.who.int/dg/speeches/detail/who-director-general-s-opening-remarks-at-the-media-briefing-on-covid-19--22-april-2020
- Spagnolo P, Balestro E, Aliberti S, et al. Pulmonary fibrosis secondary to COVID-19: a call to arms? Lancet Respir Med 2020;8:750-2. [Crossref] [PubMed]
- Tian S, Hu W, Niu L, et al. Pulmonary Pathology of Early-Phase 2019 Novel Coronavirus (COVID-19) Pneumonia in Two Patients With Lung Cancer. J Thorac Oncol 2020;15:700-4. [Crossref] [PubMed]
- Xu Z, Shi L, Wang Y, et al. Pathological findings of COVID-19 associated with acute respiratory distress syndrome. Lancet Respir Med 2020;8:420-2. [Crossref] [PubMed]
- Dai H, Zhang X, Xia J, et al. High-resolution Chest CT Features and Clinical Characteristics of Patients Infected with COVID-19 in Jiangsu, China. Int J Infect Dis 2020;95:106-12. [Crossref] [PubMed]
- Huang C, Wang Y, Li X, et al. Clinical features of patients infected with 2019 novel coronavirus in Wuhan, China. Lancet 2020;395:497-506. [Crossref] [PubMed]
- Ngai JC, Ko FW, Ng SS, et al. The long-term impact of severe acute respiratory syndrome on pulmonary function, exercise capacity and health status. Respirology 2010;15:543-50. [Crossref] [PubMed]
- Wang J, Wang BJ, Yang JC, et al. Advances in the research of mechanism of pulmonary fibrosis induced by Corona Virus Disease 2019 and the corresponding therapeutic measures. Zhonghua Shao Shang Za Zhi 2020;36:691-7. [PubMed]
- Kavianpour M, Saleh M, Verdi J. The role of mesenchymal stromal cells in immune modulation of COVID-19: focus on cytokine storm. Stem Cell Res Ther 2020;11:404. [Crossref] [PubMed]
- Manolis AS, Manolis TA, Manolis AA, et al. COVID-19 Infection: Viral Macro- and Micro-Vascular Coagulopathy and Thromboembolism/Prophylactic and Therapeutic Management. J Cardiovasc Pharmacol Ther 2021;26:12-24. [Crossref] [PubMed]
- George PM, Wells AU, Jenkins RG. Pulmonary fibrosis and COVID-19: the potential role for antifibrotic therapy. Lancet Respir Med 2020;8:807-15. [Crossref] [PubMed]
- Notice on the issuance of a new coronavirus pneumonia diagnosis and treatment plan (for trial implementation of the seventh edition). China: 2020.
- Zhou Z, Chen B, Chen S, et al. Applications of Network Pharmacology in Traditional Chinese Medicine Research. Evid Based Complement Alternat Med 2020;2020:1646905 [Crossref] [PubMed]
- Hopkins AL. Network pharmacology. Nat Biotechnol 2007;25:1110-1. [Crossref] [PubMed]
- Bian YQ, Ma J, Ren Y, et al. Discovery of intervention effect of Chinese herbal formulas on COVID-19 pulmonary fibrosis treated by VEGFR and FGFR inhibitors. Zhongguo Zhong Yao Za Zhi 2020;45:1481-7. [PubMed]
- Li S, Zhang B. Traditional Chinese medicine network pharmacology: theory, methodology and application. Chin J Nat Med 2013;11:110-20. [Crossref] [PubMed]
- Yu M, Song X, Yang W, et al. Identify the Key Active Ingredients and Pharmacological Mechanisms of Compound XiongShao Capsule in Treating Diabetic Peripheral Neuropathy by Network Pharmacology Approach. Evid Based Complement Alternat Med 2019;2019:5801591 [Crossref] [PubMed]
- Clough E, Barrett T. The Gene Expression Omnibus Database. Methods Mol Biol 2016;1418:93-110. [Crossref] [PubMed]
- Blanco-Melo D, Nilsson-Payant BE, Liu WC, et al. Imbalanced Host Response to SARS-CoV-2 Drives Development of COVID-19. Cell 2020;181:1036-45.e9. [Crossref] [PubMed]
- Piñero J, Queralt-Rosinach N, Bravo À, et al. DisGeNET: a discovery platform for the dynamical exploration of human diseases and their genes. Database (Oxford) 2015;2015:bav028 [Crossref] [PubMed]
- Rebhan M, Chalifa-Caspi V, Prilusky J, et al. GeneCards: a novel functional genomics compendium with automated data mining and query reformulation support. Bioinformatics 1998;14:656-64. [Crossref] [PubMed]
- Wang Y, Zhang S, Li F, et al. Therapeutic target database 2020: enriched resource for facilitating research and early development of targeted therapeutics. Nucleic Acids Res 2020;48:D1031-41. [PubMed]
- Sussman JL, Lin D, Jiang J, et al. Protein Data Bank (PDB): database of three-dimensional structural information of biological macromolecules. Acta Crystallogr D Biol Crystallogr 1998;54:1078-84. [Crossref] [PubMed]
- Szklarczyk D, Morris JH, Cook H, et al. The STRING database in 2017: quality-controlled protein-protein association networks, made broadly accessible. Nucleic Acids Res 2017;45:D362-8. [Crossref] [PubMed]
- Song Y, Wang H, Pan Y, et al. Investigating the Multi-Target Pharmacological Mechanism of Hedyotis diffusa Willd Acting on Prostate Cancer: A Network Pharmacology Approach. Biomolecules 2019;9:591. [Crossref] [PubMed]
- Smoot ME, Ono K, Ruscheinski J, et al. Cytoscape 2.8: new features for data integration and network visualization. Bioinformatics 2011;27:431-2. [Crossref] [PubMed]
- Yu MX, Ma XQ, Song X, et al. Validation of the Key Active Ingredients and Anti-Inflammatory and Analgesic Effects of Shenjin Huoxue Mixture Against Osteoarthritis by Integrating Network Pharmacology Approach and Thin-Layer Chromatography Analysis. Drug Des Devel Ther 2020;14:1145-56. [Crossref] [PubMed]
- Raudvere U, Kolberg L, Kuzmin I, et al. g:Profiler: a web server for functional enrichment analysis and conversions of gene lists (2019 update). Nucleic Acids Res 2019;47:W191-8 [Crossref] [PubMed]
- Kanehisa M, Goto S. KEGG: kyoto encyclopedia of genes and genomes. Nucleic Acids Res 2000;28:27-30. [Crossref] [PubMed]
- Ru J, Li P, Wang J, et al. TCMSP: a database of systems pharmacology for drug discovery from herbal medicines. J Cheminform 2014;6:13. [Crossref] [PubMed]
- Bitencourt-Ferreira G, de Azevedo WF Jr. Docking with SwissDock. Methods Mol Biol 2019;2053:189-202. [Crossref] [PubMed]
- Jee Y. WHO International Health Regulations Emergency Committee for the COVID-19 outbreak. Epidemiol Health 2020;42:e2020013 [Crossref] [PubMed]
- Sun P, Qie S, Liu Z, et al. Clinical characteristics of hospitalized patients with SARS-CoV-2 infection: A single arm meta-analysis. J Med Virol 2020;92:612-7. [Crossref] [PubMed]
- Todd NW, Luzina IG, Atamas SP. Molecular and cellular mechanisms of pulmonary fibrosis. Fibrogenesis Tissue Repair 2012;5:11. [Crossref] [PubMed]
- Wynn TA. Cellular and molecular mechanisms of fibrosis. J Pathol 2008;214:199-210. [Crossref] [PubMed]
- Kim HR, Shin DY, Chung KH. A review of current studies on cellular and molecular mechanisms underlying pulmonary fibrosis induced by chemicals. Environ Health Toxicol 2018;33:e2018014 [Crossref] [PubMed]
- King TE Jr, Albera C, Bradford WZ, et al. Effect of interferon gamma-1b on survival in patients with idiopathic pulmonary fibrosis (INSPIRE): a multicentre, randomised, placebo-controlled trial. Lancet 2009;374:222-8. [Crossref] [PubMed]
- McGonagle D, Sharif K, O'Regan A, et al. The Role of Cytokines including Interleukin-6 in COVID-19 induced Pneumonia and Macrophage Activation Syndrome-Like Disease. Autoimmun Rev 2020;19:102537 [Crossref] [PubMed]
- Le TT, Karmouty-Quintana H, Melicoff E, et al. Blockade of IL-6 Trans signaling attenuates pulmonary fibrosis. J Immunol 2014;193:3755-68. [Crossref] [PubMed]
- Zhao Y, Tian B, Sadygov RG, et al. Integrative proteomic analysis reveals reprograming tumor necrosis factor signaling in epithelial mesenchymal transition. J Proteomics 2016;148:126-38. [Crossref] [PubMed]
- Altintas N, Erboga M, Aktas C, et al. Protective Effect of Infliximab, a Tumor Necrosis Factor-Alfa Inhibitor, on Bleomycin-Induced Lung Fibrosis in Rats. Inflammation 2016;39:65-78. [Crossref] [PubMed]
- Hoshino T, Okamoto M, Sakazaki Y, et al. Role of proinflammatory cytokines IL-18 and IL-1beta in bleomycin-induced lung injury in humans and mice. Am J Respir Cell Mol Biol 2009;41:661-70. [Crossref] [PubMed]
- Chen L, Liu HG, Liu W, et al. Analysis of clinical features of 29 patients with 2019 novel coronavirus pneumonia. Zhonghua Jie He He Hu Xi Za Zhi 2020;43:203-8. [PubMed]
- Zhang C, Wu Z, Li JW, et al. Cytokine release syndrome in severe COVID-19: interleukin-6 receptor antagonist tocilizumab may be the key to reduce mortality. Int J Antimicrob Agents 2020;55:105954 [Crossref] [PubMed]
- Ruan Q, Yang K, Wang W, et al. Clinical predictors of mortality due to COVID-19 based on an analysis of data of 150 patients from Wuhan, China. Intensive Care Med 2020;46:846-8. [Crossref] [PubMed]
- Baowen Q, Yulin Z, Xin W, et al. A further investigation concerning correlation between anti-fibrotic effect of liposomal quercetin and inflammatory cytokines in pulmonary fibrosis. Eur J Pharmacol 2010;642:134-9. [Crossref] [PubMed]
- Veith C, Drent M, Bast A, et al. The disturbed redox-balance in pulmonary fibrosis is modulated by the plant flavonoid quercetin. Toxicol Appl Pharmacol 2017;336:40-8. [Crossref] [PubMed]
- Hohmann MS, Habiel DM, Coelho AL, et al. Hogaboam CM. Quercetin Enhances Ligand-induced Apoptosis in Senescent Idiopathic Pulmonary Fibrosis Fibroblasts and Reduces Lung Fibrosis In Vivo. Am J Respir Cell Mol Biol 2019;60:28-40. [Crossref] [PubMed]
- Sriram N, Kalayarasan S, Sudhandiran G. Epigallocatechin-3-gallate augments antioxidant activities and inhibits inflammation during bleomycin-induced experimental pulmonary fibrosis through Nrf2-Keap1 signaling. Pulm Pharmacol Ther 2009;22:221-36. [Crossref] [PubMed]
- You H, Wei L, Sun WL, et al. The green tea extract epigallocatechin-3-gallate inhibits irradiation-induced pulmonary fibrosis in adult rats. Int J Mol Med 2014;34:92-102. [Crossref] [PubMed]
- Guan R, Zhao X, Wang X, et al. Emodin alleviates bleomycin-induced pulmonary fibrosis in rats. Toxicol Lett 2016;262:161-72. [Crossref] [PubMed]
- Tian SL, Yang Y, Liu XL, et al. Emodin Attenuates Bleomycin-Induced Pulmonary Fibrosis via Anti-Inflammatory and Anti-Oxidative Activities in Rats. Med Sci Monit 2018;24:1-10. [Crossref] [PubMed]
- Jin X, Luo X, Song K, et al. Effect of Huzhang on expression of lung fibroblasts' MMP-2/TIMP-1mRNA in pulmonary fibrosis rats. Zhongguo Zhong yao za zhi 2009;34:1574-7. [PubMed]
- Wang J, He F, Chen L, et al. Resveratrol inhibits pulmonary fibrosis by regulating miR-21 through MAPK/AP-1 pathways. Biomed Pharmacother 2018;105:37-44. [Crossref] [PubMed]
- Zhang YQ, Liu YJ, Mao YF, et al. Resveratrol ameliorates lipopolysaccharide-induced epithelial mesenchymal transition and pulmonary fibrosis through suppression of oxidative stress and transforming growth factor-beta1 signaling. Clin Nutr 2015;34:752-60. [Crossref] [PubMed]
- Wang Y, Gao XM, Zhang BL, et al. Building methodology for discovering and developing Chinese medicine based on network biology. Zhongguo Zhong Yao Za Zhi 2011;36:228-31. [PubMed]
- Xin WY, Bai M, Miao MS, et al. Chinese medicine research and the secondary development model of traditional Chinese medicine based on network pharmacology. Acta Chinese Medicine 2016;31:92-5.