Exploring the mechanism of Shenqisherong pill against cervical spondylotic myelopathy by network pharmacology and molecular docking
Introduction
Cervical spondylotic myelopathy (CSM) is a common degenerative disease that causes spinal cord injury (1). It is triggered by the degeneration changes of the cervical intervertebral junction structures, such as disc herniation, posterior vertebral spur, ossification of the posterior longitudinal ligament, yellow ligament hypertrophy, or calcification that causes the cervical spinal cord to suffer from chronic progressive compression (1,2). CSM is the most common cause of spinal cord dysfunction in adults (3). It often causes pain and decreases the quality of life of patients. In severe cases, it could lead to disability (4). Also, it brings about a great economic burden on the family and society. Previous studies have been reported that the incidence and prevalence of CSM are at least 4.1 and 60.5 per 100,000, respectively, and will gradually increase as the global population ages, making it a growing public health concern (4,5).
Presently, the treatment of CSM is mainly divided into surgical and conservative approaches. A large number of studies have shown that the decompression of cervical spine surgery can prevent further development of symptoms, improve patients’ dysfunction, relieve pain, and improve the quality of life of patients (6-8). However, some studies have shown that there is no evidence that surgical treatment is better for mild to moderate myelopathy than conservative treatment. Thus, it is recommended that patients with mild symptoms be first treated conservatively (9,10). Furthermore, due to the risk of surgical treatment and complications post-surgery, a single surgical method cannot meet the clinical needs. Hence, a conservative treatment that can effectively control the condition and promote the recovery of patients is an urgent requisite.
Shenqisherong pill (SQSRP) was formulated based on the clinical experience of Professor Qi Shi, a well-known traditional Chinese medicine (TCM) practitioner in China and Shanghai Longhua Hospital. SQSRP is a representative TCM formula used clinically in Longhua Hospital to treat CSM for more than 20 years. It has provided a favorable therapeutic effect for patients with mild to moderate CSM. SQSRP is composed of Radix astragali (RA), Radix salviae Miltiorrhizae (RSM), Saline cistanche (SC), and musk. In addition, accumulating evidence has indicated that numerous active ingredients from the herbs of SQSRP had significant anti-inflammatory and neuroprotective effects (11-15). Our previous studies have shown that SQSRP reduces local inflammation, improves spinal cord ischemia or blood stasis, relieves continued spinal cord injury, and thus improves patients’ clinical symptoms and promotes recovery (16,17). It also exerts an adequate clinical effect in the treatment of patients with CSM in the early and middle stages and has been approved by the National Medical Products Administration for clinical trials (clinical trial acceptance number: CXZL1900016). However, the molecular mechanisms underlying SQSRP against CSM are yet unclear and need further investigation.
SQSRP, like other TCM formulas, has a complex composition with multi-component, multi-target, and multi-pathway synergy characteristics. Thus, these features and the mechanism of action are difficult to analyze using conventional experimental methods (18,19). TCM emphasizes dialectical treatment. Many diseases are often caused by multiple factors, and the symptoms can change dynamically with disease progression (20,21). Therefore, it is difficult to achieve curative effects by relying on a single drug component and a single target (22). Over the years, research on the mechanism of the efficacy of TCM has always been the focus and difficulty of TCM research. Network pharmacology is an emerging discipline based on systems biology theory and network analysis of biological systems (23). It is employed to explore the intervention and influence of drugs on disease networks (22,24). Intriguingly, network pharmacology emphasizes multi-pathway regulation of the signaling pathway, which is in accordance with the characteristics of TCM-based syndrome differentiation, multiple components, and multiple targets (25). Network pharmacology provides a novel method for the study of the efficacy of TCM and has been increasingly used in the research of the mechanism of TCM in treating various diseases; for example, the mechanism of Zhichan powder in the treatment of Parkinson’s disease and the multi-component synergy mechanism of Banxia xiexin decoction on irritable bowel syndrome (26,27).
In this study, we used a network pharmacological and molecular docking approach to explore the molecular mechanism of SQSRP in the treatment of CSM. Firstly, we screened and collected the related targets in CSM and the active ingredients of SQSRP through network pharmacology databases. Then, the interactions among the overlapping targets were deduced via STRING. Next, the overlapping targets were uploaded to the Omicshare analysis platform for Gene Ontology (GO) and Kyoto Encyclopedia of Genes and Genomes (KEGG) pathway analyses. Subsequently, the pharmacological data were integrated into the target-pathway (T-P) network and compound target-pathway (C-T-P) network to identify the key compounds and targets of SQSRP in the treatment of CSM. Finally, the molecular docking method was used to verify the binding effect of the compounds to the targets. The database or software used in this study is shown in Table 1, and the workflow is illustrated in Figure 1.
Table 1
Description of methods | Databases and software |
---|---|
Ingredients database building | TCMSP (http://www.tcmspw.com/tcmsp.php), TCMID (http://www.megabionet.org/tcmid/), Shanghai Institute of Organic Chemistry of CAS. Chemistry Database (http://www.organchem.csdb.cn) |
Screening for bioactive compounds | TCMSP (http://www.tcmspw.com/tcmsp.php) |
Screening of potential targets of bioactive compounds and diseases in database | TCMSP (http://www.tcmspw.com/tcmsp.php), UniProt (https://www.uniprot.org/), OMIM (https://omim.org/), GeneCards (https://www.genecards.org/), Bioinformatics and Evolutionary Genomics (http://bioinformatics.psb.ugent.be/webtools/Venn/) |
Construction of PPI network | STRING ver 11.0 (https://string-db.org/), Cytoscape (ver 3.7.2) |
GO and KEGG pathway enrichment analysis | Omnicshare (https://www.omicshare.com/tools/) |
Network construction | Cytoscape (ver 3.7.2) |
Molecular docking | PubChem (https://pubchem.ncbi.nlm.nih.gov/), Open Babel (ver 2.3.2), Protein Data Bank (http://www.rcsb.org/pdb), PyMOL (ver 2.3.4), AutoDockTools (ver 1.5.6), AutoDock Vina (ver 1.1.2) |
TCMSP, Traditional Chinese Medicine Systems Pharmacology; TCMID, Traditional Chinese Medicines Integrated Database; OMIM, Online Mendelian Inheritance in Man; PPI, protein-protein interaction; STRING, Search Tool for the Retrieval of Interacting Genes/Proteins; GO, Gene Ontology; KEGG, Kyoto Encyclopedia of Genes and Genomes.
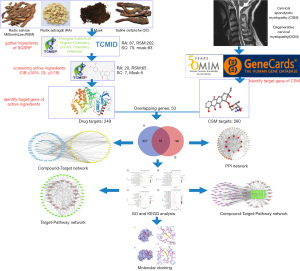
We present the following article in accordance with the MDAR reporting checklist (available at https://dx.doi.org/10.21037/apm-21-408).
Methods
Ingredients database building
The chemical ingredients of the four herbal medicines in SQSRP were collected from Traditional Chinese Medicines Systems Pharmacology (TCMSP) database (http://www.tcmspw.com/tcmsp.php) (28), Traditional Chinese Medicine Integrated Database (TCMID) (http://www.megabionet.org/tcmid) (29), Shanghai Institute of Organic Chemistry of CAS. Chemistry Database (http://www.organchem.csdb.cn), and related literature (30-32).
Screening for bioactive compounds
The components of TCM formulas are complex and diverse and may not be absorbed by the human body to achieve the desired treatment of the disease. Therefore, in order to screen out compounds with high activity in SQSRP, the collected components were imported into the TCMSP database to find the absorption, distribution, metabolism, and excretion (ADME) information. Oral bioavailability (OB) indicates the percentage of a drug being absorbed into the circulatory system and generates pharmacological effects after ingesting into the human body (33). The OB was calculated using the OBioavail 1.1 system and was set at a benchmark of ≥30% as OB less than 30% is regarded as low orally bioavailable drugs (34-36). The drug-likeness (DL) indicates the impression of a molecule’s pharmacodynamics in the body and determines molecules with similar characteristics and targets. The DL was calculated using the Tanimoto coefficient and was set at a benchmark of ≥0.18 (34,35). The compounds without ADME information were excluded, and the final list was reserved only for those components that met both OB ≥30% and DL ≥0.18. In addition, some compounds that did not meet the screening criteria but had crucial pharmacological effects were also retained for further analysis.
Screening of potential targets of bioactive compounds and diseases in databases
The protein target information of each bioactive compound was obtained from the TCMSP database. Compounds without target information were excluded. Subsequently, these protein targets were introduced into the UniProt database (https://www.uniprot.org/) to retrieve the corresponding gene symbol (37). The protein targets that were not identified in the database were removed.
CSM-related target genes were collected from the Online Mendelian Inheritance in Man (OMIM) (https://omim.org/) and GeneCards (https://www.genecards.org/) databases (38,39). The search keywords were as follows: cervical spondylotic myelopathy or degenerative cervical myelopathy. To date, all SQSRP-associated and CSM-associated target genes were collected, and the duplicate genes were deleted. Then, an online Venn mapping software (http://bioinformatics.psb.ugent.be/webtools/Venn/) was used to identify and visualize the overlapping genes between the compound and the CSM target genes. The study was conducted in accordance with the Declaration of Helsinki (as revised in 2013).
Construction of protein-protein interaction (PPI) network for overlapping genes
In order to explore the interaction between the protein targets of anti-CSM and identify the key proteins, the overlapping genes were imported into STRING 11.0 database (https://string-db.org/) (40), and species was set to “Homo sapiens” after “Multiple Proteins” was selected; the other parameters were default. The obtained protein interaction results were saved in TSV format. The node 1, node 2, and combined score information in the files were retained and imported into Cytoscape software to draw the interaction network (41). The Network Analyzer tool in Cytoscape software was used to analyze the network. The node size and color were set to reflect the size of the degree value, and the thickness of the edge was set to reflect the size of the combination score to obtain the final PPI network. To further identify the key proteins in the network, node 1 and node 2 in the export file were analyzed to obtain the number of adjacent nodes for each protein. Then, the bar graph of the top 30 proteins was visualized using the bar plot function in the R language.
GO and KEGG pathway enrichment analysis of overlapping genes
To illustrate the role of anti-CSM target proteins in gene function and understand the potential mechanism of SQSRP against CSM, we performed GO function and KEGG pathway enrichment analysis of overlapping genes using Omicshare tools, a free online analysis platform (https://www.omicshare.com/tools/) (42).
Network construction
In order to explore the pharmacological mechanisms of SQSRP in the treatment of CSM, we constructed the regulatory network of SQSRP. Firstly, the interactions between compounds and overlapping targets were collected from the TCMSP database and the research results of the gene symbol to construct the interaction network of overlapping genes and compounds using Cytoscape 3.7.2 software. Then, combined with the data of 20 interested pathways from KEGG analysis, a T-P network and a C-T-P network were constructed using Cytoscape 3.7.2 software. Finally, the Network Analyzer tool was used to assess the topology of the network and identify the key target genes or compounds in the network.
Molecular docking
The compounds in Spatial Data File (SDF) file format were searched via the PubChem website (https://pubchem.ncbi.nlm.nih.gov/) and converted into Protein Data Bank (PDB) file using the Open Babel 2.3.2 software (43,44). The crystal structures of the receptor proteins were retrieved from the PDB database (http://www.rcsb.org/pdb). Then, water removal and ligand removal were performed on the receptor protein using the PyMOL 2.3.4 software, and the receptor protein was modified by hydrogenation and charge balance using AutoDockTools software (45,46). Subsequently, the receptor protein and small ligand molecules were converted into PDBQT format. The molecular docking of the receptor protein and small ligand molecules was carried out using the AutoDock Vina 1.1.2 software (Spacing (angstrom) =1, size_x =50, size_y =50, size_z =50 in each target) (47). The docking process was evaluated by the genetic algorithm. All docking run options were default values. Finally, the docking results with the lowest score were visualized using the PyMOL 2.3.4 software.
Results
Compounds of SQSRP
SQSRP consists of four herbal medicines, namely RA, RSM, SC, and musk. After removing the duplicate ingredients, a total of 447 compounds were identified in SQSRP, including 87 in RA, 202 in RSM, 75 in SC, and 83 in musk (Table S1).
Identification of SQSRP bioactive compounds
A total of 96 bioactive components (OB ≥30%, DL ≥0.18) were identified in four herbal medicines in SQSRP by ADME screening, including 20 in RA, 65 in RSM, 7 in SC, and 5 in musk. Additionally, two ingredients that did not meet the screening criteria were retained on the active ingredients list because of their specific therapeutic effects. For example, our previous studies have shown that echinacoside reduces the inflammatory response in a rat model of CSM via inhibition of excessive mitochondrial fission (48). Chen et al. showed that glycine has a neuroprotective role in neuron damage caused by oxygen-glucose deprivation by regulating the level of microRNA-301a (49). Some studies exhibited that testosterone had a neuroprotective effect and the ability to induce myelin regeneration in multiple sclerosis (50). The detailed information of the 96 active ingredients is shown in Table 2.
Table 2
Mol ID | Compound | OB (%) | DL | MW | AlogP | Hdon | Hacc | Caco-2 | BBB | FASA- | HL | Herb |
---|---|---|---|---|---|---|---|---|---|---|---|---|
MOL001601 | 1,2,5,6-tetrahydrotanshinone | 38.75 | 0.36 | 280.34 | 2.98 | 0 | 3 | 0.96 | 0.39 | 0.33 | 18.05 | RSM |
MOL001659 | Poriferasterol | 43.83 | 0.76 | 412.77 | 7.64 | 1 | 1 | 1.44 | 1.03 | 0.22 | 5.34 | RSM |
MOL001771 | Poriferast-5-en-3beta-ol | 36.91 | 0.75 | 414.79 | 8.08 | 1 | 1 | 1.45 | 1.14 | 0 | 5.07 | RSM |
MOL001942 | Isoimperatorin | 45.46 | 0.23 | 270.3 | 3.65 | 0 | 4 | 0.97 | 0.66 | 0.27 | −1.44 | RSM |
MOL002222 | Sugiol | 36.11 | 0.28 | 300.48 | 4.99 | 1 | 2 | 1.14 | 0.7 | 0.27 | 14.62 | RSM |
MOL002651 | Dehydrotanshinone II A | 43.76 | 0.4 | 292.35 | 4.22 | 0 | 3 | 1.02 | 0.52 | 0.33 | 23.71 | RSM |
MOL002776 | Baicalin | 40.12 | 0.75 | 446.39 | 0.64 | 6 | 11 | −0.85 | −1.74 | 0.36 | 17.36 | RSM |
MOL000569 | Digallate | 61.85 | 0.26 | 322.24 | 1.53 | 6 | 9 | −0.76 | −1.52 | 0.43 | 5.29 | RSM |
MOL000006 | Luteolin | 36.16 | 0.25 | 286.25 | 2.07 | 4 | 6 | 0.19 | −0.84 | 0.39 | 15.94 | RSM |
MOL006824 | α-amyrin | 39.51 | 0.76 | 426.8 | 7.35 | 1 | 1 | 1.37 | 1.2 | 0.23 | 3.06 | RSM |
MOL007036 | 5,6-dihydroxy-7-isopropyl-1,1-dimethyl-2,3-dihydrophenanthren-4-one | 33.77 | 0.29 | 298.41 | 4.38 | 2 | 3 | 1.19 | 0.8 | 0.29 | 14.91 | RSM |
MOL007041 | 2-isopropyl-8-methylphenanthrene-3,4-dione | 40.86 | 0.23 | 264.34 | 4.16 | 0 | 2 | 1.23 | 0.81 | 0.43 | 14.89 | RSM |
MOL007045 | 3α-hydroxytanshinoneIIa | 44.93 | 0.44 | 310.37 | 3.56 | 1 | 4 | 0.53 | 0.22 | 0.3 | 23.78 | RSM |
MOL007048 | (E)-3-[2-(3,4-dihydroxyphenyl)-7-hydroxy-benzofuran-4-yl] acrylic acid | 48.24 | 0.31 | 312.29 | 3.21 | 4 | 6 | 0.18 | −0.89 | 0.4 | 8.87 | RSM |
MOL007049 | 4-methylenemiltirone | 34.35 | 0.23 | 266.36 | 4.33 | 0 | 2 | 1.25 | 0.87 | 0.38 | 14.6 | RSM |
MOL007050 | 2-(4-hydroxy-3-methoxyphenyl)-5-(3-hydroxypropyl)-7-methoxy-3-benzofurancarboxaldehyde | 62.78 | 0.4 | 356.4 | 3.58 | 2 | 6 | 0.35 | −0.73 | 0.24 | 7.89 | RSM |
MOL007051 | 6-o-syringyl-8-o-acetyl shanzhiside methyl ester | 46.69 | 0.71 | 628.64 | −1.13 | 5 | 16 | −1.73 | −2.08 | 0.22 | 9.94 | RSM |
MOL007058 | Formyltanshinone | 73.44 | 0.42 | 290.28 | 3.36 | 0 | 4 | 0.54 | −0.28 | 0.41 | 24.12 | RSM |
MOL007059 | 3-beta-Hydroxymethyllenetanshiquinone | 32.16 | 0.41 | 294.32 | 3.16 | 1 | 4 | 0.38 | −0.48 | 0.36 | 22.51 | RSM |
MOL007061 | Methylenetanshinquinone | 37.07 | 0.36 | 278.32 | 4.26 | 0 | 3 | 1.03 | 0.46 | 0.36 | 24.33 | RSM |
MOL007063 | Przewalskin a | 37.11 | 0.65 | 398.49 | 2.25 | 1 | 6 | −0.26 | −0.69 | 0.38 | 1.63 | RSM |
MOL007064 | Przewalskin b | 110.32 | 0.44 | 330.46 | 3.18 | 1 | 4 | 0.34 | 0.22 | 0.32 | 2.17 | RSM |
MOL007068 | Przewaquinone B | 62.24 | 0.41 | 292.3 | 2.99 | 1 | 4 | 0.39 | −0.45 | 0.38 | 24.94 | RSM |
MOL007069 | Przewaquinone c | 55.74 | 0.4 | 296.34 | 3.31 | 1 | 4 | 0.42 | −0.3 | 0.32 | 23.7 | RSM |
MOL007070 | (6S,7R)-6,7-dihydroxy-1,6-dimethyl-8,9-dihydro-7H-naphtho[8,7-g]benzofuran-10,11-dione | 41.31 | 0.45 | 312.34 | 2.34 | 2 | 5 | −0.06 | −0.68 | 0.32 | 22.54 | RSM |
MOL007071 | Przewaquinone f | 40.31 | 0.46 | 312.34 | 2.07 | 2 | 5 | −0.09 | −0.9 | 0.29 | 22.45 | RSM |
MOL007077 | Sclareol | 43.67 | 0.21 | 308.56 | 4.27 | 2 | 2 | 0.84 | 0.51 | 0.27 | 4.71 | RSM |
MOL007079 | Tanshinaldehyde | 52.47 | 0.45 | 308.35 | 3.83 | 0 | 4 | 0.57 | −0.07 | 0.32 | 23.49 | RSM |
MOL007081 | Danshenol B | 57.95 | 0.56 | 354.48 | 2.59 | 1 | 4 | 0.53 | 0.11 | 0.3 | 4.28 | RSM |
MOL007082 | Danshenol A | 56.97 | 0.52 | 336.41 | 2.01 | 1 | 4 | 0.33 | −0.01 | 0.34 | 5.15 | RSM |
MOL007085 | Salvilenone | 30.38 | 0.38 | 292.4 | 4.26 | 0 | 2 | 1.46 | 1.07 | 0.35 | 20.81 | RSM |
MOL007088 | cryptotanshinone | 52.34 | 0.4 | 296.39 | 3.44 | 0 | 3 | 0.95 | 0.51 | 0.29 | 17.3 | RSM |
MOL007093 | dan-shexinkum d | 38.88 | 0.55 | 336.41 | 2.83 | 1 | 4 | 0.67 | −0.15 | 0.35 | 30 | RSM |
MOL007094 | Danshenspiroketallactone | 50.43 | 0.31 | 282.36 | 3.24 | 0 | 3 | 0.88 | 0.51 | 0.34 | 15.19 | RSM |
MOL007098 | Deoxyneocryptotanshinone | 49.4 | 0.29 | 298.41 | 4.32 | 1 | 3 | 0.85 | 0.24 | 0.3 | 27.17 | RSM |
MOL007100 | Dihydrotanshinlactone | 38.68 | 0.32 | 266.31 | 2.77 | 0 | 3 | 1.26 | 0.81 | 0.38 | 5.42 | RSM |
MOL007101 | DihydrotanshinoneI | 45.04 | 0.36 | 278.32 | 2.86 | 0 | 3 | 0.95 | 0.43 | 0.4 | 18.32 | RSM |
MOL007105 | Epidanshenspiroketallactone | 68.27 | 0.31 | 284.38 | 2.37 | 0 | 3 | 0.9 | 0.61 | 0.33 | 1.77 | RSM |
MOL007107 | C09092 | 36.07 | 0.25 | 286.5 | 5.98 | 1 | 1 | 1.63 | 1.54 | 0.25 | −0.16 | RSM |
MOL007108 | Isocryptotanshi-none | 54.98 | 0.39 | 296.39 | 3.59 | 0 | 3 | 0.93 | 0.34 | 0.3 | 31.92 | RSM |
MOL007111 | Isotanshinone II | 49.92 | 0.4 | 294.37 | 4.66 | 0 | 3 | 1.03 | 0.45 | 0.3 | 24.73 | RSM |
MOL007115 | Manool | 45.04 | 0.2 | 304.57 | 5.5 | 1 | 1 | 1.28 | 1.16 | 0.28 | 5.81 | RSM |
MOL007118 | Microstegiol | 39.61 | 0.28 | 298.46 | 4.75 | 1 | 2 | 1.05 | 0.99 | 0.33 | 4.52 | RSM |
MOL007119 | Miltionone I | 49.68 | 0.32 | 312.39 | 3.33 | 1 | 4 | 0.35 | −0.11 | 0.35 | 41.49 | RSM |
MOL007120 | Miltionone II | 71.03 | 0.44 | 312.39 | 2.14 | 1 | 4 | 0.62 | 0.03 | 0.28 | 2.91 | RSM |
MOL007121 | Miltipolone | 36.56 | 0.37 | 300.43 | 2.74 | 1 | 3 | 0.5 | 0.17 | 0.3 | 1.7 | RSM |
MOL007122 | Miltirone | 38.76 | 0.25 | 282.41 | 4.73 | 0 | 2 | 1.23 | 0.87 | 0.32 | 14.82 | RSM |
MOL007123 | Miltirone II | 44.95 | 0.24 | 272.32 | 0.77 | 1 | 4 | 0.04 | −0.25 | 0.35 | 2.24 | RSM |
MOL007124 | Neocryptotanshinone ii | 39.46 | 0.23 | 270.35 | 3.61 | 1 | 3 | 0.76 | 0.16 | 0.32 | 26.98 | RSM |
MOL007125 | Neocryptotanshinone | 52.49 | 0.32 | 314.41 | 3.01 | 2 | 4 | 0.35 | −0.13 | 0.28 | 14.46 | RSM |
MOL007127 | 1-methyl-8,9-dihydro-7H-naphtho[5,6-g]benzofuran-6,10,11-trione | 34.72 | 0.37 | 280.29 | 3.21 | 0 | 4 | 0.5 | −0.27 | 0.33 | 37.89 | RSM |
MOL007130 | Prolithospermic acid | 64.37 | 0.31 | 314.31 | 2.77 | 4 | 6 | 0.1 | −0.75 | 0.42 | 8.82 | RSM |
MOL007132 | (2R)-3-(3,4-dihydroxyphenyl)-2-[(Z)-3-(3,4-dihydroxyphenyl)acryloyl]oxy-propionic acid | 109.38 | 0.35 | 360.34 | 2.69 | 5 | 8 | −0.33 | −1.02 | 0.41 | 2.01 | RSM |
MOL007140 | (Z)-3-[2-[(E)-2-(3,4-dihydroxyphenyl)vinyl]-3,4-dihydroxy-phenyl]acrylic acid | 88.54 | 0.26 | 314.31 | 2.82 | 5 | 6 | −0.09 | −0.77 | 0.43 | 4.31 | RSM |
MOL007141 | Salvianolic acid g | 45.56 | 0.61 | 340.3 | 2.2 | 4 | 7 | −0.14 | −0.97 | 0.45 | 2.4 | RSM |
MOL007142 | Salvianolic acid j | 43.38 | 0.72 | 538.49 | 3.78 | 6 | 12 | −0.82 | −2.14 | 0.44 | 5.77 | RSM |
MOL007143 | Salvilenone I | 32.43 | 0.23 | 270.4 | 2.88 | 1 | 2 | 1.13 | 0.77 | 0.3 | 1 | RSM |
MOL007145 | Salviolone | 31.72 | 0.24 | 268.38 | 4.05 | 1 | 2 | 1.04 | 0.72 | 0.36 | 0.33 | RSM |
MOL007149 | NSC 122421 | 34.49 | 0.28 | 300.48 | 4.99 | 1 | 2 | 1.08 | 0.63 | 0.29 | 14.56 | RSM |
MOL007150 | (6S)-6-hydroxy-1-methyl-6-methylol-8,9-dihydro-7H-naphtho[8,7-g]benzofuran-10,11-quinone | 75.39 | 0.46 | 312.34 | 2.42 | 2 | 5 | 0.03 | −0.74 | 0.29 | 23.45 | RSM |
MOL007151 | Tanshindiol B | 42.67 | 0.45 | 312.34 | 2.34 | 2 | 5 | 0.05 | −0.63 | 0.33 | 22.25 | RSM |
MOL007152 | Przewaquinone E | 42.85 | 0.45 | 312.34 | 2.34 | 2 | 5 | −0.04 | −0.65 | 0.32 | 22.44 | RSM |
MOL007154 | Tanshinone iia | 49.89 | 0.4 | 294.37 | 4.66 | 0 | 3 | 1.05 | 0.7 | 0.31 | 23.56 | RSM |
MOL007155 | (6S)-6-(hydroxymethyl)-1,6-dimethyl-8,9-dihydro-7H-naphtho[8,7-g]benzofuran-10,11-dione | 65.26 | 0.45 | 310.37 | 3.57 | 1 | 4 | 0.44 | −0.31 | 0.29 | 23.48 | RSM |
MOL007156 | Tanshinone VI | 45.64 | 0.3 | 296.34 | 2.44 | 2 | 4 | 0.48 | −0.28 | 0.38 | 15.21 | RSM |
MOL000211 | Mairin | 55.38 | 0.78 | 456.78 | 6.52 | 2 | 3 | 0.73 | 0.22 | 0.26 | 8.87 | RA |
MOL000239 | Jaranol | 50.83 | 0.29 | 314.31 | 2.09 | 2 | 6 | 0.61 | −0.22 | 0.29 | 15.5 | RA |
MOL000296 | Hederagenin | 36.91 | 0.75 | 414.79 | 8.08 | 1 | 1 | 1.32 | 0.96 | 0 | 5.35 | RA |
MOL000033 | (3S,8S,9S,10R,13R,14S,17R)-10,13-dimethyl-17-[(2R,5S)-5-propan-2-yloctan-2-yl]-2,3,4,7,8,9,11,12,14,15,16,17-dodecahydro-1H-cyclopenta[a]phenanthren-3-ol | 36.23 | 0.78 | 428.82 | 8.54 | 1 | 1 | 1.45 | 1.09 | 0 | 5.22 | RA |
MOL000354 | Isorhamnetin | 49.6 | 0.31 | 316.28 | 1.76 | 4 | 7 | 0.31 | −0.54 | 0.32 | 14.34 | RA |
MOL000371 | 3,9-di-O-methylnissolin | 53.74 | 0.48 | 314.36 | 2.89 | 0 | 5 | 1.18 | 0.63 | 0 | 9 | RA |
MOL000374 | 5’-hydroxyiso-muronulatol-2’,5’-di-O-glucoside | 41.72 | 0.69 | 642.67 | −0.95 | 9 | 16 | −2.47 | −3.62 | 0 | 2.52 | RA |
MOL000378 | 7-O-methylisomucronulatol | 74.69 | 0.3 | 316.38 | 3.38 | 1 | 5 | 1.08 | 0.84 | 0 | 2.98 | RA |
MOL000379 | 9,10-dimethoxypterocarpan-3-O-β-D-glucoside | 36.74 | 0.92 | 462.49 | 0.74 | 4 | 10 | −0.63 | −1.5 | 0 | 13.06 | RA |
MOL000380 | (6aR,11aR)-9,10-dimethoxy-6a,11a-dihydro-6H-benzofurano[3,2-c]chromen-3-ol | 64.26 | 0.42 | 300.33 | 2.64 | 1 | 5 | 0.93 | 0.55 | 0 | 8.49 | RA |
MOL000387 | Bifendate | 31.1 | 0.67 | 418.38 | 2.56 | 0 | 10 | 0.15 | −0.06 | 0 | 17.96 | RA |
MOL000392 | Formononetin | 69.67 | 0.21 | 268.28 | 2.58 | 1 | 4 | 0.78 | 0.02 | 0 | 17.04 | RA |
MOL000398 | Isoflavanone | 109.99 | 0.3 | 316.33 | 2.42 | 2 | 6 | 0.53 | 0.17 | 0 | 15.51 | RA |
MOL000417 | Calycosin | 47.75 | 0.24 | 284.28 | 2.32 | 2 | 5 | 0.52 | −0.43 | 0 | 17.1 | RA |
MOL000422 | Kaempferol | 41.88 | 0.24 | 286.25 | 1.77 | 4 | 6 | 0.26 | −0.55 | 0 | 14.74 | RA |
MOL000433 | FA | 68.96 | 0.71 | 441.45 | 0.01 | 7 | 13 | −1.5 | −2.59 | 0 | 24.81 | RA |
MOL000438 | (3R)-3-(2-hydroxy-3,4-dimethoxyphenyl)chroman-7-ol | 67.67 | 0.26 | 302.35 | 3.13 | 2 | 5 | 0.96 | 0.34 | 0 | 2.9 | RA |
MOL000439 | Isomucronulatol-7,2'-di-O-glucosiole | 49.28 | 0.62 | 626.67 | −0.68 | 8 | 15 | −2.22 | −3.36 | 0 | 0.93 | RA |
MOL000442 | 1,7-Dihydroxy-3,9-dimethoxy pterocarpene | 39.05 | 0.48 | 314.31 | 3.11 | 2 | 6 | 0.89 | −0.04 | 0 | 7.95 | RA |
MOL000098 | Quercetin | 46.43 | 0.28 | 302.25 | 1.5 | 5 | 7 | 0.05 | −0.77 | 0.38 | 14.4 | RA & SC |
MOL000358 | Beta-sitosterol | 36.91 | 0.75 | 414.79 | 8.08 | 1 | 1 | 1.32 | 0.99 | 0.23 | 5.36 | SC |
MOL005320 | Arachidonate | 45.57 | 0.2 | 304.52 | 6.41 | 1 | 2 | 1.27 | 0.58 | 0.26 | 7.56 | SC |
MOL005384 | Suchilactone | 57.52 | 0.56 | 368.41 | 3.73 | 0 | 6 | 0.82 | 0.28 | 0.28 | 9.03 | SC |
MOL007563 | Yangambin | 57.53 | 0.81 | 446.54 | 2.6 | 0 | 8 | 0.67 | 0.01 | 0.14 | 3.61 | SC |
MOL008871 | Marckine | 37.05 | 0.69 | 475.69 | 5.3 | 3 | 5 | 0.86 | −0.1 | 0.17 | 13.56 | SC |
MOL008875 | Echinacoside | 3.14 | 0.38 | 786.81 | −1.36 | 12 | 20 | −3.11 | −4.2 | 0.32 | SC | |
MOL000953 | Cholesterol | 37.87 | 0.68 | 386.73 | 7.38 | 1 | 1 | 1.43 | 1.13 | 0.2 | 4.52 | Musk |
MOL000737 | Morin | 46.23 | 0.27 | 302.25 | 1.5 | 5 | 7 | 0 | −0.77 | 0.41 | 15.51 | Musk |
MOL010919 | 17-beta-estradiol | 12.41 | 0.32 | 272.42 | 3.81 | 2 | 2 | 0.95 | 0.46 | 0.27 | Musk | |
MOL001232 | TES | 12.93 | 0.35 | 288.47 | 3.33 | 1 | 2 | 0.68 | 0.29 | 0.26 | Musk | |
MOL000050 | GLY | 48.74 | 0 | 75.08 | −0.98 | 3 | 3 | −0.56 | −1.03 | 0 | 11.95 | Musk |
SQSRP, Shenqisherong Pill; ADME, absorption, distribution, metabolism, and excretion; OB, oral bioavailability; DL, drug-likeness; MW, molecular weight; AlogP, Atomic log P; Hdon, hydrogen-bond donors; Hacc, hydrogen-bond acceptors; Caco-2, caco-2 permeability; BBB, blood-brain barrier; FASA-, fractional negative surface area; HL, half-life; RSM, Radix Salviae Miltiorrhiza; RA, Radix Astragali; SC, Saline Cistanche.
Target gene identification of bioactive compounds and disease
After screening by the TCMSP database and the UniProt database, a total of 249 target genes corresponding to 84 compounds were collected (Table S2). As listed in Table S3, a total of 280 CSM-related target genes were identified from the OMIM and GeneCards databases. The Venn diagram (Figure 2) showed 53 overlapping genes between 280 CSM-related and 249 compounds-related genes. These overlapping molecules represent potential target genes for SQSRP in the treatment of CSM.
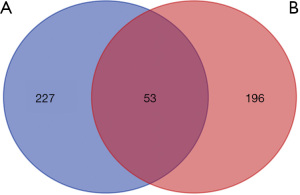
Analysis of PPI network for overlapping genes
In order to explore the correlation among the 53 anti-CSM-related targets, a PPI network was constructed. The nodes in the PPI network represent proteins, and edges represent interactions between the proteins. The size of the node and the shade of the color represent the degree value. The higher the degree value of a node, the more critical its role in the network. The detailed information on 53 target genes in the PPI network is shown in Table S4.
As shown in Figure 3, the network contains a large number of interlaced lines, indicating a strong correlation among these targets. This phenomenon indicates that SQSRP has a synergistic effect on multiple closely related targets in the treatment of CSM. The visualization results of the degree values of the targets in the network are shown in Figure 4. The top ten targets are interleukin 6 (IL-6), AKT serine/threonine kinase 1 (Akt1), C-X-C motif chemokine ligand 8 (CXCL8), transcription factor AP-1 (JUN), vascular endothelial growth factor A (VEGFA), interleukin-1 beta (IL-1B), C-C motif chemokine 2 (CCL2), mitogen-activated protein kinase 8 (MAPK8), mitogen-activated protein kinase 1 (MAPK1), and intercellular adhesion molecule 1 (ICAM1); these occupy a critical position in the entire network and are speculated as the key targets for SQSRP efficacy in the treatment of CSM.
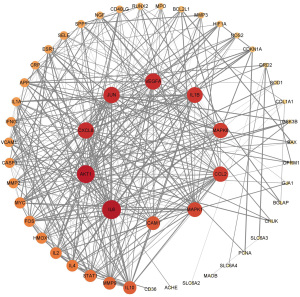
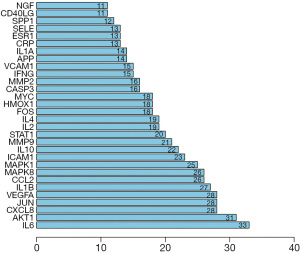
GO and KEGG pathway enrichment analysis of overlapping genes
The results of GO enrichment analysis include three categories: biological process (BP), molecular function (MF), and cellular component (CC). The data of BP, MF, and CC enrichment analysis of the 53 anti-CSM target genes were collected from Omicshare, and the top 15 significantly enriched terms in the three categories (P<0.05) were visualized using the Omicshare tools (Figure 5A-5C). RichFactor refers to the ratio of the number of genes located in the term entry among the differentially expressed genes to the total number of genes located in the term entry among all genes. The larger the RichFactor, the higher the degree of enrichment. The size of the bubble indicates the number of genes. The larger the bubble, the greater the number of genes enriched to the term. The shade of the bubble color indicates the level of significance; the redder the color, the greater the concentration of the term. Our results indicated that SQSRP might be responsive to oxygen-containing compound, cytokine stimulus, toxic substances, and inflammatory reaction via signaling receptor binding, cytokine receptor binding, cytokine activity, and growth factor receptor binding in the membrane raft, membrane region, extracellular region, axon, and neuron part to exert an anti-CSM potential.
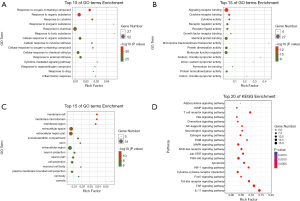
KEGG pathway enrichment analysis of overlapping genes was performed using the Omicshare online analysis platform at P<0.05 for screening; consequently, a total of 132 signaling pathways were obtained. Literature screening retrieved 20 KEGG pathways visualized using the Omicshare tools (Figure 5D), and the relevant information of those pathways are listed in Table 3. The current results indicated that the molecular mechanisms of SQSRP against CSM were closely related to these 20 signaling pathways.
Table 3
Pathway ID | Pathway | Number | Target genes |
---|---|---|---|
ko04657 | IL-17 signaling pathway | 15 | GSK3B, MAPK1, MMP9, MAPK8, CCL2, IFNG, IL4, IL1B, IL6, MMP3, CASP3, CXCL8, FOS, JUN, CHUK |
ko04668 | TNF signaling pathway | 15 | SELE, ICAM1, MAPK1, MMP9, MAPK8, CCL2, IL1B, IL6, AKT1, MMP3, VCAM1, CASP3, FOS, JUN, CHUK |
ko04151 | PI3K-Akt signaling pathway | 14 | GSK3B, MAPK1, COL1A1, IL2, VEGFA, IL4, SPP1, CDKN1A, NGF, IL6, MYC, AKT1, BCL2L1, CHUK |
ko04010 | MAPK signaling pathway | 12 | MAPK1, MAPK8, VEGFA, IL1A, IL1B, NGF, MYC, AKT1, CASP3, FOS, JUN, CHUK |
ko04620 | Toll-like receptor signaling pathway | 11 | MAPK1, MAPK8, STAT1, SPP1, IL1B, IL6, AKT1, CXCL8, FOS, JUN, CHUK |
ko04060 | Cytokine-cytokine receptor interaction | 11 | IL1B, IL2, IFNG, IL1A, IL6, CCL2, CD40LG, IL10, NGF, CXCL8, IL4 |
ko04660 | T cell receptor signaling pathway | 11 | MAPK1, IL2, IFNG, AKT1, GSK3B, JUN, FOS, CD40LG, IL10, CHUN, IL4 |
ko04210 | Apoptosis | 10 | BAX, MAPK1, MAPK8, NGF, AKT1, CASP3, FOS, BCL2L1, JUN, CHUK |
ko04630 | Jak-STAT signaling pathway | 10 | IL2, IFNG, IL4, STAT1, CDKN1A, IL6, IL10, MYC, AKT1, BCL2L1 |
ko04621 | NOD-like receptor signaling pathway | 10 | MAPK1, MAPK8, CCL2, STAT1, IL1B, IL6, CXCL8, BCL2L1, JUN, CHUK |
ko04066 | HIF-1 signaling pathway | 9 | NOS2, MAPK1, HMOX1, HIF1A, IFNG, VEGFA, CDKN1A, IL6, AKT1 |
ko04915 | Estrogen signaling pathway | 8 | MMP2, ESR1, MAPK1, MMP9, OPRM1, AKT1, FOS, JUN |
ko04068 | FoxO signaling pathway | 7 | MAPK8, MAPK1, IL10, AKT1, CHUN, IL6, CDKN1A |
ko04012 | ErbB signaling pathway | 7 | GSK3B, MAPK1, MAPK8, CDKN1A, MYC, AKT1, JUN |
ko04722 | Neurotrophin signaling pathway | 7 | GSK3B, BAX, MAPK1, MAPK8, NGF, AKT1, JUN |
ko04064 | NF-kappa B signaling pathway | 7 | ICAM1, CD40LG, IL1B, VCAM1, CXCL8, BCL2L1, CHUK |
ko04062 | Chemokine signaling pathway | 7 | GSK3B, MAPK1, CCL2, STAT1, AKT1, CXCL8, CHUK |
ko04014 | Ras signaling pathway | 7 | MAPK1, MAPK8, VEGFA, NGF, AKT1, BCL2L1, CHUK |
ko04024 | cAMP signaling pathway | 6 | MAPK1, MAPK8, AKT1, DRD2, FOS, JUN |
ko04920 | Adipocytokine signaling pathway | 4 | MAPK8, CD36, AKT1, CHUK |
CSM, cervical spondylotic myelopathy; SQSRP, Shenqisherong Pill.
Results of network construction-based analysis
According to the TCMSP database and the research results of the gene symbol, 56 compounds that interacted with the 53 overlapping genes were identified. The interaction network of 53 overlapping genes and 56 compounds, containing 109 nodes and 476 edges, was constructed using Cytoscape 3.7.2 (Figure 6). To screen out the crucial active components and key targets of SQSRP against CSM, T-P and C-T-P networks were also constructed using Cytoscape 3.7.2 software. The nodes in the networks represent interacting protein target genes, compounds, or pathways. The edges represent the interactions between the nodes. The degree value of the nodes represents the number of interacting genes, compounds, or pathways. The higher the degree value of the node, the more critical the corresponding genes, compounds, or pathways are for the treatment of CSM.
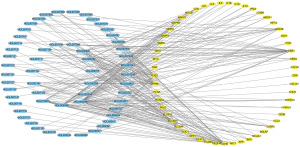
The degree value of the compounds or genes was specifically set, and the topology of the network was analyzed to screen out the crucial active components and key targets of SQSRP against CSM. The degree values of the nodes in the network are shown in Table 4. As shown in Figure 6 and Figure 7, four active ingredients (quercetin, luteolin, 17-beta-estradiol, and kaempferol) have high degree values. In the T-P network, we found that Akt1 and MAPK1 have high degree values, 16 and 15, respectively (Figure 8). In addition, IL-17, TNF, PI3K-Akt, and MAPK signaling pathways have high degree values, 15, 15, 14, and 12, respectively (Figures 7,8). Thus, it can be speculated that these compounds, targets, and pathways have a pharmacological effect in the treatment of CSM by SQSRP. Further analysis showed that 41/56 compounds are connected to at least two targets, and 36/53 targets are connected to at least two compounds (Figure 3), i.e., the same active ingredient may act on different targets, and different active ingredients may act on the same target. These data indicated that SQSRP exerts its medicinal effect in CSM treatment through a multi-component, multi-target, and multi-pathway synergistic effect.
Table 4
Compound | Value | Gene | Value |
---|---|---|---|
MOL000098 | 38 | ACHE | 32 |
MOL000006 | 19 | OPRM1 | 31 |
MOL010919 | 17 | ESR1 | 25 |
MOL000422 | 13 | NOS2 | 14 |
MOL000392 | 9 | SLC6A4 | 13 |
MOL007154 | 8 | GSK3B | 12 |
MOL007100 | 7 | SLC6A3 | 9 |
MOL007145 | 6 | CASP3 | 6 |
MOL000358 | 5 | JUN | 6 |
MOL000378 | 5 | CDKN1A | 4 |
MOL000354 | 5 | BCL2L1 | 4 |
MOL007124 | 5 | AKT1 | 4 |
MOL007111 | 5 | DRD2 | 4 |
MOL007108 | 5 | VCAM1 | 3 |
MOL007049 | 5 | SELE | 3 |
MOL000371 | 4 | BAX | 3 |
MOL007094 | 4 | MAOB | 3 |
MOL007093 | 4 | SLC6A2 | 3 |
MOL007041 | 4 | IFNG | 3 |
MOL000417 | 3 | ICAM1 | 3 |
MOL000380 | 3 | HMOX1 | 3 |
MOL007122 | 3 | MAPK1 | 3 |
MOL007119 | 3 | MMP9 | 3 |
MOL007105 | 3 | MMP2 | 3 |
MOL007098 | 3 | IL1B | 2 |
MOL007088 | 3 | SOD1 | 2 |
MOL007061 | 3 | STAT1 | 2 |
MOL007050 | 3 | MYC | 2 |
MOL002651 | 3 | FOS | 2 |
MOL002222 | 3 | CD40LG | 2 |
MOL007155 | 2 | IL4 | 2 |
MOL007130 | 2 | IL2 | 2 |
MOL007127 | 2 | APP | 2 |
MOL007121 | 2 | IL6 | 2 |
MOL007107 | 2 | IL10 | 2 |
MOL007079 | 2 | VEGFA | 2 |
MOL007069 | 2 | BGLAP | 1 |
MOL007059 | 2 | NGF | 1 |
MOL007045 | 2 | CD36 | 1 |
MOL007036 | 2 | RUNX2 | 1 |
MOL001601 | 2 | SPP1 | 1 |
MOL000737 | 1 | CHUK | 1 |
MOL000433 | 1 | CRP | 1 |
MOL000296 | 1 | MPO | 1 |
MOL000239 | 1 | IL1A | 1 |
MOL007156 | 1 | COL1A1 | 1 |
MOL007152 | 1 | CXCL8 | 1 |
MOL007151 | 1 | CCL2 | 1 |
MOL007150 | 1 | GJA1 | 1 |
MOL007143 | 1 | HIF1A | 1 |
MOL007132 | 1 | MMP3 | 1 |
MOL007125 | 1 | MAPK8 | 1 |
MOL007120 | 1 | PCNA | 1 |
MOL007085 | 1 | ||
MOL007081 | 1 | ||
MOL007070 | 1 |
C-T, compound-target.
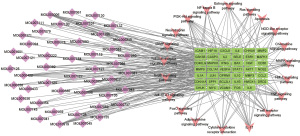
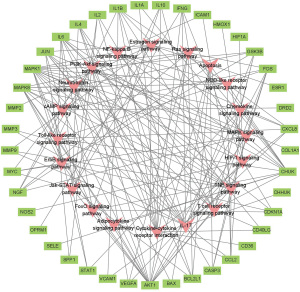
Molecular docking
We used molecular docking studies to investigate the putative interaction between active ingredients in SQSRP and key anti-CSM targets. The binding energy and docking parameters are shown in Table 5. The lower the energy value, the better the combination effects. The two docking results had the lowest scores (Figure 9). Figure 9A shows the binding mode between receptor protein Akt1 and Luteolin ligand small molecule. Ala230, Lys179, Thr291, and Glu278 form hydrogen bonds with small ligand molecules. The amino acid residues Tyr229, Phe438, Leu156, ALA177, Val164, Asp292, and Met281 form hydrophobic interactions with the small ligand molecules. Figure 9B shows the binding mode between the receptor protein MAPK1 and the small ligand molecule 17-beta-estradiol. The amino acid residues Asp167 and Lys54 interact with the small ligand molecule via hydrogen bonds. The amino acid residues, Val39, Ile31, Gln105, Met108, Glu109, Thr110, Leu156, and Cys166, interact with small ligand hydrophobic molecules. These docking results showed that the active compounds had a high affinity with key targets, especially luteolin and 17-beta-estradiol.
Table 5
Protein | Grid_size | Docking score (kcal/mol) | |||
---|---|---|---|---|---|
17-beta-estradiol | kaempferol | luteolin | quercetin | ||
AKT1 | 50×50×50 | −8.7 | −8.0 | −8.7 | −8.7 |
MAPK1 | 50×50×50 | −8.9 | −8.4 | −8.3 | −8.0 |
AKT1, AKT serine/threonine kinase 1; MAPK1, mitogen-activated protein kinase 1.
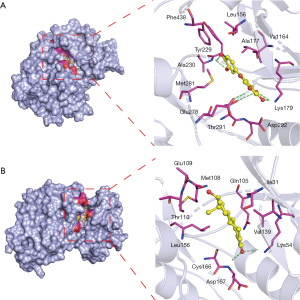
Discussion
Network pharmacology can comprehensively and systematically analyze the role of drug molecules in diseases and has been increasingly used in the research of mechanisms underlying TCM formulas (51). In this study, the active ingredients of SQSRP were analyzed using the network pharmacological method—the C-T network was constructed, and the interaction between the compounds and the targets was analyzed. The C-T network showed that the therapeutic effect of SQSRP on CSM was closely related to 56 active ingredients and 53 target genes. The analysis of the degree value of compounds in the network found that the key active ingredients were quercetin, luteolin, 17-beta-estradiol, and kaempferol. Quercetin is a natural antioxidant that has been used as an anti-inflammatory drug (52,53). Several animal experiments have shown that quercetin reduces neuroinflammation and apoptosis after spinal cord injury and protects the neurons (21,52,54,55). Luteolin is a natural flavonoid compound with various pharmacological activities, such as anti-inflammatory, anti-allergic, and anti-tumor (56). Reportedly, luteolin protects the nerves by reducing oxidative stress and inhibiting inflammatory response and apoptosis after spinal cord injury (57). In addition, it exerts an analgesic effect on both acute and inflammatory pain as well as neuropathic pain (58). 17-beta-estradiol is the active ingredient in musk. Studies have shown that 17-beta-estradiol reduces secondary damage after spinal cord injury in rats (59,60). This neuroprotective effect could be attributed to increased Kir4.1 and glutamate transporter one expression in astrocytes by 17-beta-estradiol, thereby improving the homeostasis of potassium and glutamate (61). Kaempferol is an active flavonoid substance with anti-inflammatory, antioxidant, and anti-viral effects that can alleviate LPS-induced neuroinflammation and BBB dysfunction in mice by inhibiting HMGB1 release and downregulating TLR4/MyD88 pathway (62). In addition, many compounds, such as formononetin in RA, tanshinone IIA in RSM, have anti-inflammatory and neuroprotective effects in the C-T network (63,64). Thus, these findings suggested that the main active ingredients in SQSRP have therapeutic effects on CSM.
In order to explore the correlation among 56 CSM-related target genes, the PPI network was constructed (Figure 3). Further analysis found that target genes, such as IL-6, Akt1, CXCL8, JUN, VEGFA, IL-1b, CCL2, MAPK8, MAPK1, and ICAM1 occupied an important position in the network, indicating that these target genes were vital to the action of SQSRP against CSM. To illustrate the role of 53 target genes in gene function and signaling pathways, we performed GO function enrichment and KEGG pathway enrichment analyses. The current study demonstrated that SQSRP was mainly involved in the regulation of nitrogen compounds, reactive oxygen species, cytokines, lipids, and inflammation. The results of KEGG enrichment analysis and T-P network (Figures 5D and 8) showed that these 20 signaling pathways were closely related to the therapeutic effects of SQSRP against CSM and PI3K-Akt, MAPK, IL-17, and TNF were the pivotal signaling pathways. In the pathological process of CSM, progressive compression can cause chronic hypoxic-ischemic damage to the spinal cord tissue, triggering a series of inflammatory reactions and leading to neuron and oligodendrocyte apoptosis. Several studies have shown that the PI3K-Akt signaling pathway after spinal cord injury significantly reduces apoptosis and protects the nerves (65,66). Thus, the MAPK pathway is critical for the pathogenesis of neuropathic pain and spinal cord apoptosis, which is closely related to the occurrence and development of CSM (67,68). Furthermore, chronic persistent neuropathic pain could be alleviated by suppressing the expression of IL-17A in the IL-17 signaling pathway (69). The pro-inflammatory cytokine TNF-α mediates the recruitment of inflammatory cells into injured spinal cord tissues, and by inhibiting the expression of TNF-α, the inflammation in CSM is reduced (70). The current research suggested that the signaling pathways mentioned above may play crucial roles in the inflammatory response, apoptosis, and neuroprotection and exert the therapeutic effects of SQSRP against CSM.
The results of the C-T-P network analysis indicated that 17-beta-estradiol, kaempferol, luteolin, and quercetin are the pivotal active ingredients and Akt1 and MAPK1 are the critical targets of SQSRP against CSM. Akt1, Akt2, and Akt3 and isoforms of the Akt kinase (71). The Akt kinase plays a vital role in the body’s physiological and pathological signaling mechanism as it is involved in cellular functions, such as cell survival, proliferation, migration, and gene expression (72). Some studies have shown that the upregulation of Akt1 activation and activation of the PI3K/Akt pathway contributes to the neuroprotective effect during spinal cord injury (73-76). The MAPK1, also known as ERK2, is a member of the MAP kinase signal transduction pathway. The activation of MAPK1 provides neuroprotection during spinal cord injury (77-79). Molecular docking showed that luteolin and 17-beta-estradiol tightly bind to Akt1 and MAPK1, respectively, suggesting that the critical components of SQSRP could reduce nerve injury by regulating inflammation, reducing stress response, and reducing neuronal apoptosis.
Nevertheless, the present study has several limitations. First, network pharmacology is an approach to discover the network of drug actions through a combination of drug-target networks and biological networks (22). However, this qualitative analysis of the interaction of “drug-component-target-disease” is limited in explaining the overall compatibility mechanism of the TCM compounds as the databases might show discrepancies due to numerous sources of information and theoretical and experimental data (80).
Second, the TCM formula contains a variety of herbs and could be prescribed in different ratios upon the rationale of the physician and the perceived syndrome of the patients. The different ratios of the herbs and the solvent used as a vehicle of the prescription may alter the concentrations of the compounds in the body after consumption, and uncertainty to the concentrations absorbed in the body may occur due to the pharmacokinetics of absorption, distribution, metabolism, excretion, and toxic effects (ADMET) parameters in the body (81). Nonetheless, the dose-efficacy correlation between the compounds and disease is critical, and it is difficult to quantify the effect of the compounds with the current network pharmacology (82). Therefore, further validation experiments on drug concentration are required to accurately reflect the effective components of SQSRP in the treatment of CSM.
Third, the TCM formula is composed of various herbs that play different roles during the treatment according to the principles of TCM theories and therapies (83). However, the results showed that some of the compounds are relatively low in the original herbs, thereby implying that they only exert theoretical significance and do not contribute to the clinical therapeutic value of TCM towards this disease.
Fourth, several active ingredients such as quercetin, kaempferol, and β-sitosterol were found in many herbs and exhibited pharmacological actions on the target disease. Owing to this phenomenon, the specificity of the medicinal activity of these herbs is yet controversial. However, natural products usually act through the modulation of multiple targets rather than a single, specific target. This synergistic action of natural products could address multiple targets of diseases and contribute to the holistic approach of TCM (84). Nevertheless, bioinformatics analysis and further in vivo and in vitro pharmacological experimental validations are imperative to support this hypothesis (85).
In summary, the results of network pharmacological analysis indicated that the anti-CSM effect of SQSRP was achieved through multi-compound, multi-target, and multi-pathway synergy. The C-T-P network and KEGG enrichment analysis identified 20 signaling pathways closely related to the anti-CSM effect of SQSRP and four key signaling pathways in the network. Next, we verified the interactions between these compounds and targets, as well as targets and targets. The results of network pharmacology provided a theoretical basis for the subsequent extraction of active ingredients from SQSRP to treat CSM.
Conclusions
In this study, for the first time, the potential mechanism of SQSRP against CSM was investigated using network pharmacology. We identified the therapeutic effects of SQSRP against CSM that were effectuated by reducing inflammation, inhibiting apoptosis, and protecting neurons (Figure 10). The molecular mechanisms were closely related to 20 pathways, and PI3K-Akt, MAPK, IL-17, and TNF might be the critical signaling pathways (Figure 8). Four key compounds and two key targets were identified by comprehensive analysis. These targets were further verified by molecular docking. To elucidate the mechanism of action of SQSRP in the treatment of CSM, we conducted an in vivo pharmacokinetic study, determined the pharmacokinetic characteristics of the active compounds by liquid mass spectrometry, and observed the anti-inflammatory and inhibition of apoptosis effects of the active ingredients at different concentrations through in vivo and in vitro models.
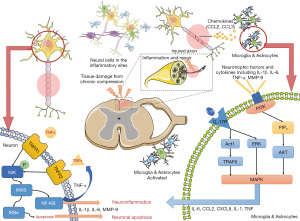
Acknowledgments
Funding: This study was supported by the National Natural Science Foundation of China (No. 81930116, 81804115, 81873317, 82074454), the National Key R&D Plan (No. 2018YFC1704302), and Shanghai Natural Science Foundation (No. 20ZR1459000).
Footnote
Reporting Checklist: The authors have completed the MDAR reporting checklist. Available at https://dx.doi.org/10.21037/apm-21-408
Conflicts of Interest: All authors have completed the ICMJE uniform disclosure form (available at https://dx.doi.org/10.21037/apm-21-408). The author reports fundings from the National Key R&D Plan (No. 2018YFC1704302), the National Natural Science Foundation of China (No. 82074454, 81930116, 81873317, and 81804115) and Shanghai Natural Science Foundation (No. 20ZR1459000).
Ethical Statement: The authors are accountable for all aspects of the work in ensuring that questions related to the accuracy or integrity of any part of the work are appropriately investigated and resolved. The study was conducted in accordance with the Declaration of Helsinki (as revised in 2013).
Open Access Statement: This is an Open Access article distributed in accordance with the Creative Commons Attribution-NonCommercial-NoDerivs 4.0 International License (CC BY-NC-ND 4.0), which permits the non-commercial replication and distribution of the article with the strict proviso that no changes or edits are made and the original work is properly cited (including links to both the formal publication through the relevant DOI and the license). See: https://creativecommons.org/licenses/by-nc-nd/4.0/.
References
- Nouri A, Cheng JS, Davies B, et al. Degenerative Cervical Myelopathy: A Brief Review of Past Perspectives, Present Developments, and Future Directions. J Clin Med 2020;9:535. [Crossref] [PubMed]
- Matsunaga S, Komiya S, Toyama Y. Risk factors for development of myelopathy in patients with cervical spondylotic cord compression. Eur Spine J 2015;24:142-9. [Crossref] [PubMed]
- Tetreault LA, Rhee J, Prather H, et al. Change in Function, Pain, and Quality of Life Following Structured Nonoperative Treatment in Patients With Degenerative Cervical Myelopathy: A Systematic Review. Global Spine J 2017;7:42S-52S. [Crossref] [PubMed]
- Gibson J, Nouri A, Krueger B, et al. Degenerative Cervical Myelopathy: A Clinical Review. Yale J Biol Med 2018;91:43-8. [PubMed]
- Badhiwala JH, Ahuja CS, Akbar MA, et al. Degenerative cervical myelopathy - update and future directions. Nat Rev Neurol 2020;16:108-24. [Crossref] [PubMed]
- Haddas R, Lieberman I, Arakal R, et al. Effect of Cervical Decompression Surgery on Gait in Adult Cervical Spondylotic Myelopathy Patients. Clin Spine Surg 2018;31:435-40. [Crossref] [PubMed]
- Ross MN, Ross DA. Minimally Invasive Cervical Laminectomy for Cervical Spondylotic Myelopathy. Clin Spine Surg 2018;31:331-8. [Crossref] [PubMed]
- Badhiwala JH, Wilson JR. The Natural History of Degenerative Cervical Myelopathy. Neurosurg Clin N Am 2018;29:21-32. [Crossref] [PubMed]
- Kadaňka Z, Bednařík J, Novotný O, et al. Cervical spondylotic myelopathy: conservative versus surgical treatment after 10 years. Eur Spine J 2011;20:1533-8. [Crossref] [PubMed]
- Yoshimatsu H, Nagata K, Goto H, et al. Conservative treatment for cervical spondylotic myelopathy. prediction of treatment effects by multivariate analysis. Spine J 2001;1:269-73. [Crossref] [PubMed]
- Yan R, Chen R, Wang J, et al. Jingshu Keli and its Components Notoginsenoside R1 and Ginsenoside Rb1 Alleviate the Symptoms of Cervical Myelopathy through Kir3.1 Mediated Mechanisms. CNS Neurol Disord Drug Targets 2019;18:631-42. [Crossref] [PubMed]
- Yu S, Zhao G, Han F, et al. Muscone relieves inflammatory pain by inhibiting microglial activation-mediated inflammatory response via abrogation of the NOX4/JAK2-STAT3 pathway and NLRP3 inflammasome. Int Immunopharmacol 2020;82:106355 [Crossref] [PubMed]
- Yang C, Mo Y, Xu E, et al. Astragaloside IV ameliorates motor deficits and dopaminergic neuron degeneration via inhibiting neuroinflammation and oxidative stress in a Parkinson's disease mouse model. Int Immunopharmacol 2019;75:105651 [Crossref] [PubMed]
- Spagnuolo C, Moccia S, Russo GL. Anti-inflammatory effects of flavonoids in neurodegenerative disorders. Eur J Med Chem 2018;153:105-15. [Crossref] [PubMed]
- Crupi R, Impellizzeri D, Bruschetta G, et al. Co-Ultramicronized Palmitoylethanolamide/Luteolin Promotes Neuronal Regeneration after Spinal Cord Injury. Front Pharmacol 2016;7:47. [Crossref] [PubMed]
- Mo W, Shi Q, Wang YJ, et al. Clinical study on treatment of cervical spondylotic myelopathy with Yiqi Huayu Bushen formula. Shanghai Journal of Traditional Chinese Medicine 2008:41-2.
- Mo W, Shi Q, Ye XL, et al. Retrospective analysis of 109 cases of cervical spondylotic myelopathy treated with the method of Yiqi Huayu Bushen. Chinese Journal of Traditional Medical Traumatology and Orthopedics 2009;17:22-5.
- Cao H, Li S, Xie R, et al. Exploring the Mechanism of Dangguiliuhuang Decoction Against Hepatic Fibrosis by Network Pharmacology and Experimental Validation. Front Pharmacol 2018;9:187. [Crossref] [PubMed]
- Guo Q, Zheng K, Fan D, et al. Wu-Tou Decoction in Rheumatoid Arthritis: Integrating Network Pharmacology and In Vivo Pharmacological Evaluation. Front Pharmacol 2017;8:230. [Crossref] [PubMed]
- Zhou Z, Chen B, Chen S, et al. Applications of Network Pharmacology in Traditional Chinese Medicine Research. Evid Based Complement Alternat Med 2020;2020:1646905 [Crossref] [PubMed]
- Wang Y, Li W, Wang M, et al. Quercetin reduces neural tissue damage and promotes astrocyte activation after spinal cord injury in rats. J Cell Biochem 2018;119:2298-306. [Crossref] [PubMed]
- Hopkins AL. Network pharmacology. Nat Biotechnol 2007;25:1110-1. [Crossref] [PubMed]
- Hopkins AL. Network pharmacology: the next paradigm in drug discovery. Nat Chem Biol 2008;4:682-90. [Crossref] [PubMed]
- Boezio B, Audouze K, Ducrot P, et al. Network-based Approaches in Pharmacology. Mol Inform 2017; [Crossref] [PubMed]
- Shi XQ, Yue SJ, Tang YP, et al. A network pharmacology approach to investigate the blood enriching mechanism of Danggui buxue Decoction. J Ethnopharmacol 2019;235:227-42. [Crossref] [PubMed]
- Li J, Qi X, Sun Y, et al. Network Pharmacology Analysis on Zhichan Powder in the Treatment of Parkinson's Disease. Comb Chem High Throughput Screen 2020;23:28-40. [Crossref] [PubMed]
- Li B, Rui J, Ding X, et al. Exploring the multicomponent synergy mechanism of Banxia Xiexin Decoction on irritable bowel syndrome by a systems pharmacology strategy. J Ethnopharmacol 2019;233:158-68. [Crossref] [PubMed]
- Ru J, Li P, Wang J, et al. TCMSP: a database of systems pharmacology for drug discovery from herbal medicines. J Cheminform 2014;6:13. [Crossref] [PubMed]
- Huang L, Xie D, Yu Y, et al. TCMID 2.0: a comprehensive resource for TCM. Nucleic Acids Res 2018;46:D1117-20. [Crossref] [PubMed]
- Wang Y, Dong B, Xue W, et al. Anticancer Effect of Radix Astragali on Cholangiocarcinoma In Vitro and Its Mechanism via Network Pharmacology. Med Sci Monit 2020;26:e921162 [Crossref] [PubMed]
- Zhang C, Liao Y, Liu L, et al. A Network Pharmacology Approach to Investigate the Active Compounds and Mechanisms of Musk for Ischemic Stroke. Evid Based Complement Alternat Med 2020;2020:4063180 [PubMed]
- Luo Y, Feng Y, Song L, et al. A network pharmacology-based study on the anti-hepatoma effect of Radix Salviae Miltiorrhizae. Chin Med 2019;14:27. [Crossref] [PubMed]
- Zhao M, Chen Y, Wang C, et al. Systems Pharmacology Dissection of Multi-Scale Mechanisms of Action of Huo-Xiang-Zheng-Qi Formula for the Treatment of Gastrointestinal Diseases. Front Pharmacol 2019;9:1448. [Crossref] [PubMed]
- Yue SJ, Liu J, Feng WW, et al. System Pharmacology-Based Dissection of the Synergistic Mechanism of Huangqi and Huanglian for Diabetes Mellitus. Front Pharmacol 2017;8:694. [Crossref] [PubMed]
- Wang Y, Wei S, Gao T, et al. Anti-Inflammatory Effect of a TCM Formula Li-Ru-Kang in Rats With Hyperplasia of Mammary Gland and the Underlying Biological Mechanisms. Front Pharmacol 2018;9:1318. [Crossref] [PubMed]
- Hou T, Wang J, Zhang W, et al. ADME evaluation in drug discovery. 6. Can oral bioavailability in humans be effectively predicted by simple molecular property-based rules? J Chem Inf Model 2007;47:460-3. [Crossref] [PubMed]
- The UniProt Consortium. UniProt: the universal protein knowledgebase. Nucleic Acids Res 2017;45:D158-69. [Crossref] [PubMed]
- Amberger JS, Bocchini CA, Schiettecatte F, et al. OMIM.org: Online Mendelian Inheritance in Man (OMIM®), an online catalog of human genes and genetic disorders. Nucleic Acids Res 2015;43:D789-98. [Crossref] [PubMed]
- Stelzer G, Rosen N, Plaschkes I, et al. The GeneCards Suite: From Gene Data Mining to Disease Genome Sequence Analyses. Curr Protoc Bioinformatics 2016;54:1.30.1-1.30.33.
- von Mering C, Huynen M, Jaeggi D, et al. STRING: a database of predicted functional associations between proteins. Nucleic Acids Res 2003;31:258-61. [Crossref] [PubMed]
- Shannon P, Markiel A, Ozier O, et al. Cytoscape: a software environment for integrated models of biomolecular interaction networks. Genome Res 2003;13:2498-504. [Crossref] [PubMed]
- Liang L, Zhu JH, Chen G, et al. Prognostic Values for the mRNA Expression of the ADAMTS Family of Genes in Gastric Cancer. J Oncol 2020;2020:9431560 [Crossref] [PubMed]
- O'Boyle NM, Banck M, James CA, et al. Open Babel: An open chemical toolbox. J Cheminform 2011;3:33. [Crossref] [PubMed]
- Berman HM, Westbrook J, Feng Z, et al. The Protein Data Bank. Nucleic Acids Res 2000;28:235-42. [Crossref] [PubMed]
- Seeliger D, de Groot BL. Ligand docking and binding site analysis with PyMOL and Autodock/Vina. J Comput Aided Mol Des 2010;24:417-22. [Crossref] [PubMed]
- Cosconati S, Forli S, Perryman AL, et al. Virtual Screening with AutoDock: Theory and Practice. Expert Opin Drug Discov 2010;5:597-607. [Crossref] [PubMed]
- Trott O, Olson AJ. AutoDock Vina: improving the speed and accuracy of docking with a new scoring function, efficient optimization, and multithreading. J Comput Chem 2010;31:455-61. [PubMed]
- Zhou L, Yao M, Tian Z, et al. Echinacoside attenuates inflammatory response in a rat model of cervical spondylotic myelopathy via inhibition of excessive mitochondrial fission. Free Radic Biol Med 2020;152:697-714. [Crossref] [PubMed]
- Chen J, Zhuang Y, Zhang ZF, et al. Glycine confers neuroprotection through microRNA-301a/PTEN signaling. Mol Brain 2016;9:59. [Crossref] [PubMed]
- Collongues N, Patte-Mensah C, De Seze J, et al. Testosterone and estrogen in multiple sclerosis: from pathophysiology to therapeutics. Expert Rev Neurother 2018;18:515-22. [Crossref] [PubMed]
- Yuan H, Ma Q, Cui H, et al. How Can Synergism of Traditional Medicines Benefit from Network Pharmacology? Molecules 2017;22:1135. [Crossref] [PubMed]
- Fan H, Tang HB, Shan LQ, et al. Quercetin prevents necroptosis of oligodendrocytes by inhibiting macrophages/microglia polarization to M1 phenotype after spinal cord injury in rats. J Neuroinflammation 2019;16:206. [Crossref] [PubMed]
- Yang Y, Liu X, Wu T, et al. Quercetin attenuates AZT-induced neuroinflammation in the CNS. Sci Rep 2018;8:6194. [Crossref] [PubMed]
- Song Y, Liu J, Zhang F, et al. Antioxidant effect of quercetin against acute spinal cord injury in rats and its correlation with the p38MAPK/iNOS signaling pathway. Life Sci 2013;92:1215-21. [Crossref] [PubMed]
- Zhang Y, Yi B, Ma J, et al. Quercetin promotes neuronal and behavioral recovery by suppressing inflammatory response and apoptosis in a rat model of intracerebral hemorrhage. Neurochem Res 2015;40:195-203. [Crossref] [PubMed]
- Swaminathan A, Basu M, Bekri A, et al. The Dietary Flavonoid, Luteolin, Negatively Affects Neuronal Differentiation. Front Mol Neurosci 2019;12:41. [Crossref] [PubMed]
- Fu J, Sun H, Zhang Y, et al. Neuroprotective Effects of Luteolin Against Spinal Cord Ischemia-Reperfusion Injury by Attenuation of Oxidative Stress, Inflammation, and Apoptosis. J Med Food 2018;21:13-20. [Crossref] [PubMed]
- Hara K, Haranishi Y, Terada T, et al. Effects of intrathecal and intracerebroventricular administration of luteolin in a rat neuropathic pain model. Pharmacol Biochem Behav 2014;125:78-84. [Crossref] [PubMed]
- Kachadroka S, Hall AM, Niedzielko TL, et al. Effect of endogenous androgens on 17beta-estradiol-mediated protection after spinal cord injury in male rats. J Neurotrauma 2010;27:611-26. [Crossref] [PubMed]
- Siriphorn A, Dunham KA, Chompoopong S, et al. Postinjury administration of 17β-estradiol induces protection in the gray and white matter with associated functional recovery after cervical spinal cord injury in male rats. J Comp Neurol 2012;520:2630-46. [Crossref] [PubMed]
- Olsen ML, Campbell SC, McFerrin MB, et al. Spinal cord injury causes a wide-spread, persistent loss of Kir4.1 and glutamate transporter 1: benefit of 17 beta-oestradiol treatment. Brain 2010;133:1013-25. [Crossref] [PubMed]
- Cheng X, Yang YL, Yang H, et al. Kaempferol alleviates LPS-induced neuroinflammation and BBB dysfunction in mice via inhibiting HMGB1 release and down-regulating TLR4/MyD88 pathway. Int Immunopharmacol 2018;56:29-35. [Crossref] [PubMed]
- Li Z, Zeng G, Zheng X, et al. Neuroprotective effect of formononetin against TBI in rats via suppressing inflammatory reaction in cortical neurons. Biomed Pharmacother 2018;106:349-54. [Crossref] [PubMed]
- Yin X, Yin Y, Cao FL, et al. Tanshinone IIA attenuates the inflammatory response and apoptosis after traumatic injury of the spinal cord in adult rats. PLoS One 2012;7:e38381 [Crossref] [PubMed]
- Li H, Zhang X, Qi X, et al. Icariin Inhibits Endoplasmic Reticulum Stress-induced Neuronal Apoptosis after Spinal Cord Injury through Modulating the PI3K/AKT Signaling Pathway. Int J Biol Sci 2019;15:277-86. [Crossref] [PubMed]
- Zhang P, Zhang L, Zhu L, et al. The change tendency of PI3K/Akt pathway after spinal cord injury. Am J Transl Res 2015;7:2223-32. [PubMed]
- Zhou J, Lin W, Chen H, et al. TRESK contributes to pain threshold changes by mediating apoptosis via MAPK pathway in the spinal cord. Neuroscience 2016;339:622-33. [Crossref] [PubMed]
- Ji RR, Gereau RW 4th, Malcangio M, et al. MAP kinase and pain. Brain Res Rev 2009;60:135-48. [Crossref] [PubMed]
- Yao CY, Weng ZL, Zhang JC, et al. Interleukin-17A Acts to Maintain Neuropathic Pain Through Activation of CaMKII/CREB Signaling in Spinal Neurons. Mol Neurobiol 2016;53:3914-26. [Crossref] [PubMed]
- Esposito E, Cuzzocrea S. Anti-TNF therapy in the injured spinal cord. Trends Pharmacol Sci 2011;32:107-15. [Crossref] [PubMed]
- Di Lorenzo A, Fernández-Hernando C, Cirino G, et al. Akt1 is critical for acute inflammation and histamine-mediated vascular leakage. Proc Natl Acad Sci U S A 2009;106:14552-7. [Crossref] [PubMed]
- Paez J, Sellers WR. PI3K/PTEN/AKT pathway. A critical mediator of oncogenic signaling. Cancer Treat Res 2003;115:145-67. [Crossref] [PubMed]
- Bi J, Shen J, Chen C, et al. Role of melatonin in the dynamics of acute spinal cord injury in rats. J Cell Mol Med 2021;25:2909-17. [Crossref] [PubMed]
- Li XG, Du JH, Lu Y, et al. Neuroprotective effects of rapamycin on spinal cord injury in rats by increasing autophagy and Akt signaling. Neural Regen Res 2019;14:721-7. [Crossref] [PubMed]
- Lv X, Liang J, Wang Z. MiR-21-5p reduces apoptosis and inflammation in rats with spinal cord injury through PI3K/AKT pathway. Panminerva Med 2020; Epub ahead of print. [Crossref] [PubMed]
- Lu H, Zhang LH, Yang L, et al. The PI3K/Akt/FOXO3a pathway regulates regeneration following spinal cord injury in adult rats through TNF-α and p27kip1 expression. Int J Mol Med 2018;41:2832-8. [Crossref] [PubMed]
- Zhou CL, Li F, Wu XW, et al. Overexpression of miRNA-433-5p protects acute spinal cord injury through activating MAPK1. Eur Rev Med Pharmacol Sci 2020;24:2829-35. [PubMed]
- Zhang C, Wang MM, Zhang Y, et al. Downregulation of miRNA-127-5p aggravates spinal cord injury through activating MAPK1. Eur Rev Med Pharmacol Sci 2019;23:10617-22. [PubMed]
- Genovese T, Esposito E, Mazzon E, et al. Evidence for the role of mitogen-activated protein kinase signaling pathways in the development of spinal cord injury. J Pharmacol Exp Ther 2008;325:100-14. [Crossref] [PubMed]
- Zhang R, Zhu X, Bai H, et al. Network Pharmacology Databases for Traditional Chinese Medicine: Review and Assessment. Front Pharmacol 2019;10:123. [Crossref] [PubMed]
- Sun S, Wang Y, Wu A, et al. Influence Factors of the Pharmacokinetics of Herbal Resourced Compounds in Clinical Practice. Evid Based Complement Alternat Med 2019;2019:1983780 [Crossref] [PubMed]
- Luo TT, Lu Y, Yan SK, et al. Network Pharmacology in Research of Chinese Medicine Formula: Methodology, Application and Prospective. Chin J Integr Med 2020;26:72-80. [Crossref] [PubMed]
- Yang M, Chen JL, Xu LW, et al. Navigating traditional chinese medicine network pharmacology and computational tools. Evid Based Complement Alternat Med 2013;2013:731969 [Crossref] [PubMed]
- Zhou X, Seto SW, Chang D, et al. Synergistic Effects of Chinese Herbal Medicine: A Comprehensive Review of Methodology and Current Research. Front Pharmacol 2016;7:201. [Crossref] [PubMed]
- Li S, Zhang B. Traditional Chinese medicine network pharmacology: theory, methodology and application. Chin J Nat Med 2013;11:110-20. [Crossref] [PubMed]