Mortality prediction using clinical and laboratory features in elderly patients with severe community-acquired pneumonia
Introduction
Severe community-acquired pneumonia (SCAP), which is characterized by high morbidity, rapid disease progression, and high mortality, is a serious public health problem, particularly in elderly patients (1). The incidence of pneumonia increases with age, and death usually results from physiological changes that occur with age and chronic medical comorbidities (2-4). However, a controversial issue regarding the effect of aging on the pathogenic model of CAP has recently emerged. CAP patients have decreased cellular immune function (5), and T cell proliferation is decreased in elderly individuals (6). The Pneumonia Severity Index (PSI), which is associated with poor prognosis, is inversely correlated with several parameters related to T cell regulatory function (5,7). For elderly patients with SCAP, mortality is high among those who require an intensive care unit (ICU) stay (8,9). The in-hospital mortality among CAP patients has been estimated to be 10–12%. However, when SCAP is taken into consideration and ICU admission is required, the mortality rate rises to 50% (8,10).
The management of SCAP in elderly patients is often challenging, and risk stratification may be helpful to guide clinical decision making. Several recent studies have suggested that the mortality of elderly patients with SCAP is influenced by complex interactions among factors such as age, gender, tachycardia, effusions, and functional status (11-13). Although numerous prognostic indices for pneumonia are evaluated in elderly patients, a good index for mortality prediction remains a challenge. In addition, few studies have been performed to assess the role of a prediction model for mortality during the early stages of SCAP in elderly patients.
Based on the above information, this prospective, observational study was conducted to evaluate clinical and laboratory features (such as underlying disease and T cell subsets) for predicting mortality in elderly patients with SCAP. Subsequently, a prognostic model for mortality was developed and validated, which may help to optimize the management of these patients. We present the following article in accordance with the STARD reporting checklist (available at https://dx.doi.org/10.21037/apm-21-2537).
Methods
Ethics
This study conformed to the Declaration of Helsinki (as revised in 2013) and was approved by the ethics committee of the Fifth Affiliated Hospital, Sun Yat-sen University (2016, No. K15-1). Written informed consent was obtained from the patients or their guardians before inclusion in the study. All patients were censored at the date of death or at hospital discharge, whichever came first.
Patients
Elderly patients with SCAP who were admitted to our hospital and required an ICU stay between January 2017 and December 2018 were recruited for the study. Inclusion and exclusion criteria were then used to assess these patients, as outlined below.
Inclusion criteria: (I) age ≥65 years; (II) met the diagnostic criteria for SCAP given below; (III) ICU stay >24 h; (IV) appropriate laboratory tests, such as T cell subsets, were performed. Exclusion criteria: (I) other communicable lung diseases (such as tuberculosis and Pneumocystis carinii pneumonia, among others); (II) suffered from autoimmune diseases, connective tissue diseases, inflammatory diseases, cardiomyopathy, or valvular heart disease; (III) mental health conditions, such as schizophrenia or mood disorders; (IV) non-pneumonia-caused death.
According to the guidelines for the diagnosis and treatment of CAP issued by the Chinese Medical Association (2016 edition) (14), the severe form of CAP was defined if the patient met at least one of the following criteria: required tracheal intubation and mechanical ventilation; experienced septic shock and still needed vasoactive drugs after active fluid resuscitation; minor criteria (at least 3) including respiratory rate ≥30 bpm, oxygenation index ≤250 mmHg, infiltration in multiple lung lobes, or disturbance of consciousness and (or) disorientation.
Data collection
The data collection teams were trained in qualitative interviewing prior to data collection. At the time of admission, the following clinical and laboratory data were collected: (I) clinical features, such as comorbidities, invasive mechanical ventilation use, length of hospital stay, duration from symptom onset to treatment, aspiration occurrence, presence of multilobar pneumonia, and etiological index; (II) laboratory examinations, such as biochemical analyses, blood gas analysis, inflammation index, N-terminal pro-B-type natriuretic peptide (NT-pro BNP), and peripheral blood T-lymphocyte subsets; and (III) scores on the Acute Physiology and Chronic Health Evaluation II (APACHE II), the Sequential Organ Failure Assessment (SOFA), the PSI, and the CURB-65 (confusion, urea, respiration, blood pressure, age ≥65 years). Subsequently, all patients were given antibiotic treatment according to the guidelines (empiric treatment) of the Chinese Medical Association (14) or the results of susceptibility testing.
Statistical analysis
Normally distributed numeric variables were expressed as the mean ± standard deviation, while non-normally distributed variables were expressed as the median and interquartile range (IQR, 25th to 75th percentile). Categorical variables were described as frequency and percentage. Variables were compared between the survival and mortality groups through univariate logistic regression analysis. Multivariate logistic regression (enter method) was performed, and a predictive model for mortality in elderly SCAP patients was developed. To determine the performance of the model, receiver operating characteristic (ROC) curves were generated and the area under the ROC curve (AUROC) was determined. Subsequently, the threshold, sensitivity, specificity, accuracy, positive likelihood ratio (PLR), and negative likelihood ratio (NLR) were calculated. SPSS version 24.0 was used for statistical analysis, and a P value of <0.05 was considered to indicate a significant difference.
Results
Patient characteristics
A total of 133 patients were recruited for possible analysis. Of these, 13 were excluded because they refused to participate in the study, and the remaining 120 (96 men, 80.0%) were included and classified into a survival group (n=61) and a mortality group (n=59). The median age of the 120 patients was 70 (range, 65–79) years. Univariate analysis showed that there were no significant differences between the groups in age (P=0.067) and gender (P=0.715). Coronary heart disease was the most common comorbidity (51.67%), followed by chronic obstructive pulmonary disorder (COPD, 33.33%), type 2 diabetes mellitus (25.83%), and cerebrovascular events (21.67%). The median APACHE II and SOFA scores were 18 (range, 14.25–21.75) and 4 (range, 3–8), respectively.
In addition, Klebsiella pneumoniae (K. pneumoniae) [survival (n=6) and mortality (n=12) groups], Pseudomonas aeruginosa [survival (n=5) and mortality (n=8) groups], Acinetobacter baumannii (A. baumannii) [survival (n=4) and mortality (n=6) groups], and Escherichia coli (E. coli) [survival (n=4) and mortality (n=2) groups] were the 4 most common etiological agents. Three strains (K. pneumoniae, n=2; A. baumannii, n=1; E. coli, n=1) were isolated from blood cultures, and the others were isolated from sputum samples.
Univariate analysis
Tables 1,2 show the clinical and laboratory features of the survival and mortality groups. Based on univariate analysis (survival vs. mortality groups), significant differences were observed in the distributions of the history of COPD [odds ratio (OR) =5.276, P<0.001], chronic renal insufficiency (OR =2.870, P=0.034), PSI risk (P=0.005; class IV vs. classes I–III: OR=8.854, P=0.006; class V vs. classes I–III: OR =13.600, P=0.001), CD3+ T cell count (β=−0.010, P<0.001), CD3+CD4+ T cell count (β=−0.013, P<0.001), CD3+CD8+ T cell count (β=−0.015, P<0.001), CD3+CD4+/CD3+CD8+ T cell ratio (β=−0.719, P=0.006), APACHE II scores (β=0.083, P=0.010), SOFA scores (β=0.180, P=0.011), CURB-65 scores (β=0.493, P=0.006), and NT-pro BNP (β<0.000, P=0.016). However, other variables, such as the oxygenation index, procalcitonin, total peripheral blood lymphocyte counts, duration of mechanical ventilation, and length of ICU stay had no significant differences between the two groups (all P>0.05).
Table 1
Categorical variables | Survival group (n=61), n (%) | Mortality group (n=59), n (%) | Total (n=120), n (%) | β | OR (95% CI) | P value |
---|---|---|---|---|---|---|
Gender (male) | 48 (78.69) | 48 (81.36) | 96 (80.00) | 0.167 | 1.182 (0.482–2.898) | 0.715 |
Malignant tumor | 9 (14.75) | 10 (16.95) | 19 (15.83) | 0.165 | 1.179 (0.442–3.146) | 0.742 |
Type 2 diabetes mellitus | 15 (24.59) | 16 (27.12) | 31 (25.83) | 0.132 | 1.141 (0.504–2.586) | 0.752 |
Coronary heart disease | 29 (47.54) | 33 (55.93) | 62 (51.67) | 0.337 | 1.401 (0.683–2.874) | 0.358 |
COPD | 10 (16.39) | 30 (50.85) | 40 (33.33) | 1.663 | 5.276 (2.258–12.325) | <0.001 |
Cerebrovascular conditions | 13 (21.31) | 13 (22.03) | 26 (21.67) | 0.043 | 1.043 (0.438–2.487) | 0.923 |
Chronic renal insufficiency | 7 (11.48) | 16 (27.12) | 23 (19.17) | 1.054 | 2.870 (1.083–7.605) | 0.034 |
Aspiration | 14 (22.95) | 8 (13.56) | 22 (18.33) | −0.641 | 0.527 (0.203–1.368) | 0.188 |
Multilobar pneumonia | 57 (93.44) | 58 (98.31) | 115 (95.83) | 1.404 | 4.070 (0.441–37.535) | 0.216 |
Fever | 35 (57.38) | 41 (69.49) | 76 (63.33) | 0.526 | 1.692 (0.798–3.587) | 0.170 |
Pathogen | 29 (47.54) | 33 (55.93) | 62 (51.67) | 0.337 | 1.401 (0.683–2.874) | 0.358 |
PSI risk | ||||||
Class I–III | 17 (27.87) | 2 (3.39) | 19 (15.83) | 0.005 | ||
Class IV | 24 (39.34) | 25 (42.37) | 49 (40.83) | 2.181 | 8.854 (1.845–42.498) | 0.006 |
Class V | 20 (32.79) | 32 (54.24) | 52 (43.33) | 2.610 | 13.600 (2.835–65.244) | 0.001 |
OR, odds ratio; CI, confidence interval; COPD, chronic obstructive pulmonary disorder; PSI, Pneumonia Severity Index.
Table 2
Continuous variables | Survival group (n=61), median [IQR] | Mortality group (n=59), median [IQR] | Total (n=120), median [IQR] | β | OR (95% CI) | P value |
---|---|---|---|---|---|---|
Age, years | 69 [65–77] | 72 [66–83] | 70 [65.25–79] | 0.042 | 1.043 (0.997–1.090) | 0.067 |
Duration from symptom onset to treatment initiation (hours) | 4 [2–9] | 3 [2–8] | 3.5 [2–8] | −0.009 | 0.991 (0.951–1.034) | 0.680 |
Creatinine (μmol/L) | 91 [63.5–127] | 119 [77–193] | 103 [65.5–166] | 0.001 | 1.001 (0.999–1.003) | 0.318 |
ALT (U/L) | 19 [12–27.5] | 24 [11–45] | 21 [12–36.75] | 0.009 | 1.009 (0.996–1.022) | 0.183 |
WBC (109/L) | 10.5 [6–14.6] | 11.5 [6.9–14.8] | 10.95 [6.4–14.675] | 0.024 | 1.024 (0.975–1.076) | 0.347 |
Platelets (109/L) | 196 [144–278.5] | 203 [138–271] | 197 [139–274] | 0.000 | 1.000 (0.996–1.003) | 0.825 |
Lymphocyte count (109/L) | 1.07 [0.815–1.385] | 0.79 [0.63–1.22] | 0.965 [0.7125–1.3275] | −0.212 | 0.809 (0.548–1.195) | 0.288 |
Procalcitonin (µg/L) | 1.32 [0.295–8.82] | 2.24 [0.42–7.58] | 1.72 [0.405–7.805] | 0.000 | 1.000 (0.979–1.022) | 0.995 |
CD3+ T cell count (cells/µL) | 605 [480–679.5] | 316 [238–368] | 407.5 [311–616] | −0.010 | 0.990 (0.986–0.993) | <0.001 |
CD3+CD4+ T cell count (cells/µL) | 446 [334–495] | 168 [112–216] | 240.5 [158–456] | −0.013 | 0.987 (0.983–0.991) | <0.001 |
CD3+CD8+ T cell count (cells/µL) | 173 [145.5–223] | 106 [91–150] | 147.5 [101.25–184.25] | −0.015 | 0.985 (0.978–0.993) | <0.001 |
CD3+CD4+/CD3+CD8+ T cell ratio | 2.21 [1.755–2.925] | 1.48 [1.14–1.92] | 1.87 [1.365–2.4875] | −0.719 | 0.487(0.291–0.817) | 0.006 |
Oxygenation index | 160 [120.5–224.5] | 155 [118–202] | 155.5 [120.25–210] | −0.002 | 0.998 (0.993–1.003) | 0.437 |
Duration of mechanical ventilation (days) | 0 [0–6.5] | 0.3 [0–8] | 0 [0–7] | 0.020 | 1.021 (0.980–1.063) | 0.330 |
Length of ICU stay (days) | 6 [2–12.5] | 3 [0–14] | 4.5 [0–13.75] | 0.011 | 1.011 (0.987–1.036) | 0.357 |
Total length of hospital stay (days) | 17 [10.5–29.5] | 15 [5–26] | 15.5 [9–28.75] | 0.001 | 1.001 (0.987–1.014) | 0.925 |
APACHE II | 17 [13–20] | 19 [15–23] | 18 [14.25–21.75] | 0.083 | 1.086 (1.020–1.156) | 0.010 |
SOFA | 4 [3–6] | 5 [4–8] | 4 [3–8] | 0.180 | 1.197 (1.042–1.375) | 0.011 |
CURB-65 | 2 [1–3] | 3 [2–3] | 2 [1.25–3] | 0.493 | 1.637 (1.149–2.334) | 0.006 |
NT-pro BNP | 786 [204.5–3,568.5] | 4,280 [1,040–12,800] | 2,500 [524.75–6,460] | 0.000 | 1.000 (1.000–1.000) | 0.016 |
IQR, interquartile range; OR, odds ratio; CI, confidence interval; ALT, alanine aminotransferase; WBC, white blood cell; ICU, intensive care unit; APACHE, Acute Physiology and Chronic Health Evaluation; SOFA, Sequential Organ Failure Assessment; CURB-65, confusion, urea, respiration, blood pressure (age ≥65 years); NT-pro BNP, N-terminal pro-B-type natriuretic peptide.
Multivariate analysis and the prediction model
Multivariate analysis showed that COPD (β=2.061, P=0.008) and CD3+CD4+ T cell count (β=−0.019, P=0.017) (Table 3) were associated with the death of elderly patients with SCAP. On the basis of the multivariate logistic regression analysis, a mortality prediction model (adjusted for gender and age) was established for elderly patients with SCAP:
Table 3
Characteristics | Univariate analysis | Multivariate analysis | |||||
---|---|---|---|---|---|---|---|
β | Risk ratio & 95% CI | P value | β | Risk ratio & 95% CI | P value | ||
Gender (male)† | 0.167 | 1.182 (0.482–2.898) | 0.715 | 0.194 | 1.215 (0.265–5.569) | 0.802 | |
Age† | 0.042 | 1.043 (0.997–1.090) | 0.067 | 0.021 | 1.021 (0.941–1.107) | 0.621 | |
COPD | 1.663 | 5.276 (2.258–12.325) | 0.000 | 2.061 | 7.853 (1.733–35.583) | 0.008 | |
Chronic renal insufficiency | 1.054 | 2.870 (1.083–7.605) | 0.034 | −0.090 | 0.914 (0.153–5.471) | 0.921 | |
PSI risk | 0.005 | 0.341 | |||||
Class IV | 2.181 | 8.854 (1.845–42.498) | 0.006 | 1.876 | 6.525 (0.525–81.107) | 0.145 | |
Class V | 2.610 | 13.600 (2.835–65.244) | 0.001 | 2.006 | 7.436 (0.368–150.167) | 0.191 | |
CD3+ T cell count (cells/µL) | −0.010 | 0.990 (0.986–0.993) | 0.000 | 0.002 | 1.002 (0.988–1.016) | 0.794 | |
CD3+CD4+ T cell count (cells/µL) | −0.013 | 0.987 (0.983–0.991) | 0.000 | −0.019 | 0.981 (0.966–0.997) | 0.017 | |
CD3+CD8+ T cell count (cells/µL) | −0.015 | 0.985 (0.978–0.993) | 0.000 | 0.004 | 1.004 (0.984–1.024) | 0.727 | |
CD3+CD4+/CD3+CD8+ T cell ratio | −0.719 | 0.487 (0.291–0.817) | 0.006 | 0.596 | 1.815 (0.974–3.384) | 0.061 | |
APACHE II | 0.083 | 1.086 (1.020–1.156) | 0.010 | 0.001 | 1.001 (0.873–1.147) | 0.992 | |
SOFA | 0.180 | 1.197 (1.042–1.375) | 0.011 | 0.010 | 1.011 (0.729–1.401) | 0.950 | |
CURB-65 | 0.493 | 1.637 (1.149–2.334) | 0.006 | −0.062 | 0.940 (0.381–2.320) | 0.893 | |
NT-pro BNP | 0.000 | 1.000 (1.000–1.000) | 0.016 | 0.000 | 1.000 (1.000–1.000) | 0.312 |
†, gender and age were adjusted for risk factors in the multivariate logistic regression. SCAP, severe community-acquired pneumonia; CI, confidence interval; COPD, chronic obstructive pulmonary disorder; PSI, Pneumonia Severity Index; APACHE, Acute Physiology and Chronic Health Evaluation; SOFA, Sequential Organ Failure Assessment; CURB-65, confusion, urea, respiration, blood pressure (age ≥65 years); NT-pro BNP, N-terminal pro-B-type natriuretic peptide.
where p is the probability of poor SCAP prognosis (death), x1 is gender (male =1, female =0), x2 is age, x3 is the presence of COPD (with COPD =1, without COPD =0), and x4 is the CD3+CD4+ T cell count (cells/µL).
Performance of the prediction model
The probability of death (p) was calculated for each patient with the prediction model and subsequently evaluated using ROC analysis (Figure 1 and Table 4). The data suggested that the composite model had an AUROC of 0.915 [95% confidence interval (CI): 0.858–0.972]. Using a P value >0.539 as the cut-off, the sensitivity, specificity, and accuracy for mortality were 91.53%, 86.89%, and 78.41%, and the PLR and the NLR were 6.979 and 0.098, respectively.
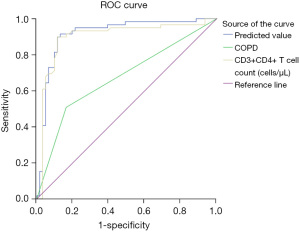
Table 4
Criteria | AUROC (95% CI) | Threshold | Sensitivity (%) | Specificity (%) | Youden’s index (%) | PLR | NLR |
---|---|---|---|---|---|---|---|
Prediction model | 0.915 (0.858–0.972) | 0.539 | 91.53 | 86.89 | 78.41 | 6.979 | 0.098 |
COPD | 0.672 (0.575–0.77) | 0.500 | 50.85 | 83.61 | 34.45 | 3.102 | 0.588 |
CD3+CD4+ T cells (cells/µL) | 0.898 (0.832–0.964) | 240.500 | 89.83 | 88.52 | 78.36 | 7.828 | 0.115 |
SCAP, severe community-acquired pneumonia; AUROC, area under the receiver operating characteristic curve; CI, confidence interval; PLR, positive likelihood ratio; NLR, negative likelihood ratio; COPD, chronic obstructive pulmonary disorder.
To validate the performance of the predictive model, a set of 22 individuals were recruited for another prospective analysis. Characteristics such as gender, age, COPD, and CD3+CD4+ T cell count were collected, and P values using the model were calculated. Under the optimal value of 0.539 for predicting death in elderly SCAP patients, the model had a sensitivity, specificity, and accuracy of 84.62%, 88.89%, and 73.50%, and the PLR and the NLR were 7.615 and 0.173, respectively (Table 5).
Table 5
Criteria | Sensitivity (%) | Specificity (%) | Accuracy (%) | PLR | NLR |
---|---|---|---|---|---|
Expected performance | 91.53 | 86.89 | 78.41 | 6.979 | 0.098 |
Observed performance | 84.62 | 88.89 | 73.50 | 7.615 | 0.173 |
PLR, positive likelihood ratio; NLR, negative likelihood ratio.
Discussion
SCAP is a public health issue that is associated with substantial morbidity and mortality. Early recognition and rapid and appropriate treatment of the disease are critical for the reduction of hospital-resource consumption and overall mortality, and this situation may be more serious for elderly patients (15). The prognosis of CAP in elderly patients may be affected by various health issues, such as immune function, nutritional status, swallowing dysfunction, pathogenic changes, and antibiotic resistance (16-19), which may create difficulties in treatment and lead to a poor prognosis. Besides the above obstacles, heterogeneity among elderly patients with SCAP also poses significant challenges in predicting the clinical outcomes of this infectious disease (20). In addition, several studies have suggested that the functional status at admission can serve as a predictive index for mortality (8,21-24). Although there are several mortality prediction score systems available, none of them are exclusively aimed at elderly patients. Hence, a model to predict death in elderly patients with SCAP was developed in our study. Our findings suggest that this proposed composite scheme outperforms the prognostic performance of each individual variable.
Models based on individual variables tend to have limited value for predicting mortality in CAP patients. Therefore, several predictive models using multiple variables have been developed. However, because different variables were included, the predictive performances were different. Factors, such as comorbidities, age, gender, disease severity, organ function, and biomarkers, which might influence the outcome of SCAP, were included in this analysis. To enable accurate prediction of mortality in elderly patients with SCAP, we integrated multiple variables identified as risk factors of mortality into a single model. In our study, most of the patients were men (80.0%). Similar findings have been reported in other studies of CAP (2,25). Further analysis showed that several factors, such as COPD, chronic kidney disease, NT-pro BNP, APACHE II score, SOFA score, PSI score, CURB-65 score, and immune system compromise, were risk factors associated with mortality in elderly individuals with SCAP. In a prospective, multi-center cohort study involving 4,070 patients with CAP in 13 Spanish hospitals, older age (>65 years), COPD, and renal disease were associated with severe sepsis (26). Similarly, based on univariate analysis, COPD and chronic renal insufficiency were predicted to have an association with mortality. However, chronic renal insufficiency was not included in the final model. As a marker for evaluating the risk of heart failure, NT-pro BNP is used to assess heart function. Additional data suggest that elderly individuals with SCAP are at a significant risk of inflammation-mediated acute cardiac events (27). However, NT-pro BNP was not included in the final multivariate analysis.
Diagnostic tools, such as APACHE II, SOFA, PSI, and CURB-65, were also assessed in our study and were all proven to have a significant association with patient outcome. APACHE II and SOFA are good scoring systems that are widely used in the ICU for predicting mortality. CURB-65 is a tool for assessing pneumonia severity on the basis of consciousness, serum urea level, respiratory rate, blood pressure, and age. However, existing data suggest that CURB-65 is not a good tool for mortality prediction in patients with SCAP (28). The PSI uses more relevant prognostic variables than CURB-65 and is an important scoring system for the assessment of severity, admission status, and prognosis prediction in patients with CAP (29), which suggests that PSI is more appropriate in clinical decision making for the comprehensive evaluation of patients with CAP (30). However, these diagnostic tools were not included as risk factors in the multivariate model.
In our study, multivariate logistic regression analysis showed that the risk of death among patients with COPD was higher than that among patients without COPD. Recent studies have also shown that in adults with CAP, especially elderly individuals, COPD is a risk factor of mortality (31-34). In addition, a lower CD3+CD4+ T cell count was associated with a poor outcome for elderly patients with SCAP. Currently, flow cytometry is the most rapid, accurate, and reliable method for the detection of surface markers. Therefore, T cell count, T cell subgroup classification, and T cell activation degree can be easily detected using flow cytometry. Through these T cell assays, immune status can be understood quickly and the results can provide a guide for clinical immunotherapy to regulate immune function. CD3+CD4+ T lymphocytes, also known as helper T cells, are believed to play an important role in both specific and non-specific types of immunity in the body and have an important regulatory role in both cellular and humoral immunity. Remarkably, it has been demonstrated that an inverse association between immune function and age may contribute to the poor prognosis in elderly patients with CAP (5,8,35). A recent study has also shown that sepsis-related immunosuppression is associated with the loss of CD4 effector T cells due to proliferation or apoptosis (36).
In our study, gender and age were adjusted when evaluating the risk of elderly patients with SCAP through multivariate logistic regression, and a prognostic model was established based on COPD and CD3+CD4+ T cells. The data suggested that the AUROC of the predictive model was greater than that of each constituent variable. The optimal cut-off value for the predictive model was determined to be 0.539 (P value) using the Youden index, which resulted in a sensitivity of 91.53%, a specificity of 86.89%, and a predictive accuracy of 78.41% for predicting in-hospital mortality. Subsequently, the model was applied to a cohort of 22 individuals from an independent validation set, resulting in a slightly lower sensitivity and higher specificity, with an accuracy of 73.50%. These data suggested that the model has a moderate level of performance for predicting mortality in elderly patients with SCAP. Although it was validated in our center, further studies need to be performed to validate this new scoring system in patients from other districts.
Although this study has several interesting findings, some limitations should be noted. First, it was a single-center study with a relatively small population. Second, the mechanisms for some findings, such as the lower isolation rate for bacterial culture, remain unclear. Third, we didn’t analyze the correlation between inflammatory factors and mortality of SCAP, in our future work, we will focus on more inflammatory indicators, such as IL-2, IL-6, IL-10, IFN-γ, TNF-α, etc. Fourth, although several scoring systems, namely APACHE II, SOFA, PSI, and CURB-65, were assessed and their predictive values were determined, a large-scale, multi-center study is required to define new prognostic variables (such as COPD and immune function) and the appropriate thresholds for elderly individuals. In addition, a mortality-risk scoring system for treatment strategies is useful and needed. This is because antibiotic resistance, new antibiotics, new microbiological methods, antibacterial regimens, and optimal treatment times would have a significant impact on the management of elderly patients with SCAP.
In conclusion, our findings indicate that the coexistence of COPD and a low CD3+CD4+ T cell count are independent prognostic factors of mortality in elderly patients with SCAP, and the constructed model was moderately accurate in the prediction of mortality among this population. The decline of immune function associated with the aged makes the worse prognosis with SCAP. Therefore, the evaluation of immune status and appropriate immune support treatment may improve the prognosis of elderly patients with SCAP and may be an important component of disease control.
Acknowledgments
We gratefully acknowledge Dr. Wu Jian’s help in English language editing.
Funding: This work was supported by grants from the Science and Technology Project in Zhuhai, Guangdong Province, China (20171009E030045).
Footnote
Reporting Checklist: The authors have completed the STARD reporting checklist. Available at https://dx.doi.org/10.21037/apm-21-2537
Data Sharing Statement: Available at https://dx.doi.org/10.21037/apm-21-2537
Conflicts of Interest: All authors have completed the ICMJE uniform disclosure form (available at https://dx.doi.org/10.21037/apm-21-2537). The authors have no conflicts of interest to declare.
Ethical Statement: The authors are accountable for all aspects of the work in ensuring that questions related to the accuracy or integrity of any part of the work are appropriately investigated and resolved. This study conformed to the Declaration of Helsinki (as revised in 2013) and was approved by the ethics committee of the Fifth Affiliated Hospital, Sun Yat-sen University (2016, No. K15-1). Written informed consent was obtained from the patients or their guardians before inclusion in the study.
Open Access Statement: This is an Open Access article distributed in accordance with the Creative Commons Attribution-NonCommercial-NoDerivs 4.0 International License (CC BY-NC-ND 4.0), which permits the non-commercial replication and distribution of the article with the strict proviso that no changes or edits are made and the original work is properly cited (including links to both the formal publication through the relevant DOI and the license). See: https://creativecommons.org/licenses/by-nc-nd/4.0/.
References
- Ashton C, Bajekal M, Raine R. Quantifying the contribution of leading causes of death to mortality decline among older people in England, 1991-2005. Health Stat Q 2010;100-27. [Crossref] [PubMed]
- Millett ER, Quint JK, Smeeth L, et al. Incidence of community-acquired lower respiratory tract infections and pneumonia among older adults in the United Kingdom: a population-based study. PLoS One 2013;8:e75131 [Crossref] [PubMed]
- Jain S, Self WH, Wunderink RG, et al. Community-acquired pneumonia requiring hospitalization among U.S. adults. N Engl J Med 2015;373:415-27. [Crossref] [PubMed]
- Cillóniz C, Polverino E, Ewig S, et al. Impact of age and comorbidity on cause and outcome in community-acquired pneumonia. Chest 2013;144:999-1007. [Crossref] [PubMed]
- Bian LQ, Bi Y, Zhou SW, et al. T cell responses in senior patients with community-acquired pneumonia related to disease severity. Exp Cell Res 2017;361:56-62. [Crossref] [PubMed]
- Garg SK, Delaney C, Toubai T, et al. Aging is associated with increased regulatory T-cell function. Aging Cell 2014;13:441-8. [Crossref] [PubMed]
- Zhao B, Chen YY, Tan MQ. Value of lymphocyte count in assessing cellular immune function in patients with community-acquired pneumonia. Nan Fang Yi Ke Da Xue Xue Bao 2016;36:273-6. [PubMed]
- Cilloniz C, Ceccato A, San Jose A, et al. Clinical management of community acquired pneumonia in the elderly patient. Expert Rev Respir Med 2016;10:1211-20. [Crossref] [PubMed]
- Waterer GW, Rello J, Wunderink RG. Management of community-acquired pneumonia in adults. Am J Respir Crit Care Med 2011;183:157-64. [Crossref] [PubMed]
- Woodhead M, Welch CA, Harrison DA, et al. Community-acquired pneumonia on the intensive care unit: secondary analysis of 17,869 cases in the ICNARC Case Mix Programme Database. Crit Care 2006;10:S1. [Crossref] [PubMed]
- Baldo V, Cocchio S, Gallo T, et al. Pneumococcal conjugated vaccine reduces the high mortality for community-acquired pneumonia in the elderly: an Italian regional experience. PLoS One 2016;11:e0166637 [Crossref] [PubMed]
- Lim WS, Macfarlane JT. Defining prognostic factors in the elderly with community acquired pneumonia: a case controlled study of patients aged > or = 75 yrs. Eur Respir J 2001;17:200-5. [Crossref] [PubMed]
- Pieralli F, Vannucchi V, De Marzi G, et al. Performance status and in-hospital mortality of elderly patients with community acquired pneumonia. Intern Emerg Med 2018;13:501-7. [Crossref] [PubMed]
- Cao B, Huang Y, She DY, et al. Diagnosis and treatment of community-acquired pneumonia in adults: 2016 clinical practice guidelines by the Chinese Thoracic Society, Chinese Medical Association. Clin Respir J 2018;12:1320-60. [Crossref] [PubMed]
- Gonçalves-Pereira J, Conceição C, Póvoa P. Community-acquired pneumonia: identification and evaluation of nonresponders. Ther Adv Infect Dis 2013;1:5-17. [Crossref] [PubMed]
- Sura L, Madhavan A, Carnaby G, et al. Dysphagia in the elderly: management and nutritional considerations. Clin Interv Aging 2012;7:287-98. [PubMed]
- Meyer KC. The role of immunity and inflammation in lung senescence and susceptibility to infection in the elderly. Semin Respir Crit Care Med 2010;31:561-74. [Crossref] [PubMed]
- Schöll N, Rohde GGU. Community-acquired pneumonia in the elderly. Pneumologie 2019;73:e3 [PubMed]
- Palmu AA, Saukkoriipi A, Snellman M, et al. Incidence and etiology of community-acquired pneumonia in the elderly in a prospective population-based study. Scand J Infect Dis 2014;46:250-9. [Crossref] [PubMed]
- Petrosillo N, Cataldo MA, Pea F. Treatment options for community-acquired pneumonia in the elderly people. Expert Rev Anti Infect Ther 2015;13:473-85. [PubMed]
- Restrepo MI, Faverio P, Anzueto A. Long-term prognosis in community-acquired pneumonia. Curr Opin Infect Dis 2013;26:151-8. [Crossref] [PubMed]
- Simonetti AF, Viasus D, Garcia-Vidal C, et al. Management of community-acquired pneumonia in older adults. Ther Adv Infect Dis 2014;2:3-16. [Crossref] [PubMed]
- Calle A, Márquez MA, Arellano M, et al. Geriatric assessment and prognostic factors of mortality in very elderly patients with community-acquired pneumonia. Arch Bronconeumol 2014;50:429-34. [Crossref] [PubMed]
- González Del Castillo J, Martín-Sánchez FJ, Llinares P, et al. Consensus guidelines for the management of community acquired pneumonia in the elderly patient. Rev Esp Geriatr Gerontol 2014;49:279-91. [PubMed]
- Torres A, Peetermans WE, Viegi G, et al. Risk factors for community-acquired pneumonia in adults in Europe: a literature review. Thorax 2013;68:1057-65. [Crossref] [PubMed]
- Montull B, Menéndez R, Torres A, et al. Predictors of severe sepsis among patients hospitalized for community-acquired pneumonia. PLoS One 2016;11:e0145929 [Crossref] [PubMed]
- Feldman C, Anderson R. Community-acquired pneumonia: pathogenesis of acute cardiac events and potential adjunctive therapies. Chest 2015;148:523-32. [Crossref] [PubMed]
- Lanspa MJ, Jones BE, Brown SM, et al. Mortality, morbidity, and disease severity of patients with aspiration pneumonia. J Hosp Med 2013;8:83-90. [Crossref] [PubMed]
- Kim MA, Park JS, Lee CW, et al. Pneumonia severity index in viral community acquired pneumonia in adults. PLoS One 2019;14:e0210102 [Crossref] [PubMed]
- Ranzani OT, Prina E, Menéndez R, et al. New sepsis definition (sepsis-3) and community-acquired pneumonia mortality. a validation and clinical decision-making study. Am J Respir Crit Care Med 2017;196:1287-97. [Crossref] [PubMed]
- Almirall J, Serra-Prat M, Bolíbar I, et al. Risk factors for community-acquired pneumonia in adults: a systematic review of observational studies. Respiration 2017;94:299-311. [Crossref] [PubMed]
- Pasquale CB, Vietri J, Choate R, et al. Patient-reported consequences of community-acquired pneumonia in patients with chronic obstructive pulmonary disease. Chronic Obstr Pulm Dis 2019;6:132-44. [Crossref] [PubMed]
- Shin B, Kim SH, Yong SJ, et al. Early readmission and mortality in acute exacerbation of chronic obstructive pulmonary disease with community-acquired pneumonia. Chron Respir Dis 2019;16:1479972318809480 [Crossref] [PubMed]
- Bordon J, Slomka M, Gupta R, et al. Hospitalization due to community-acquired pneumonia in patients with chronic obstructive pulmonary disease: incidence, epidemiology and outcomes. Clin Microbiol Infect 2020;26:220-6. [Crossref] [PubMed]
- Faverio P, Aliberti S, Bellelli G, et al. The management of community-acquired pneumonia in the elderly. Eur J Intern Med 2014;25:312-9. [Crossref] [PubMed]
- Misra AK, Levy MM, Ward NS. Biomarkers of immunosuppression. Crit Care Clin 2020;36:167-76. [Crossref] [PubMed]