Analysis of immune-related genes in idiopathic pulmonary fibrosis based on bioinformatics and experimental verification
Introduction
Idiopathic pulmonary fibrosis (IPF) is an age-related, chronic, and progressive lung disease, and is the most common type of interstitial pneumonia, with an annual incidence of about 1.2–76.4/100,000, globally (1). The main pathological manifestations of IPF are fibroblast proliferation and extracellular matrix (ECM) deposition (2). Diagnostic criteria for IPF were intended to comply with the following two requirements. First, exclusion of other known causes of interstitial lung disease (ILD) (e.g., domestic and occupational environmental exposures, connective tissue disease, and drug toxicity). Second, the presence of a UIP pattern on high-resolution computed tomography (HRCT) in patients not subjected to surgical lung biopsy (3). The clinical manifestations are unexplained persistent and progressive dyspnea, and often accompanied by coughing and Velcro rales at the base of both lungs (4). At the early stage, IPF progresses slowly, and it will gradually cause diffuse fibrosis of the lungs, eventually leading to respiratory failure and death (5). Due to the lack of in-depth understanding of the pathogenesis of IPF and early intervention, IPF has become a serious life-threatening disease. Studies have reported that the median survival time of patients after the diagnosis of IPF is about 2.5–3.5 years (6). The mortality rate of IPF is even higher than that of many malignant tumors, such as colorectal cancer, bladder cancer, and nasopharyngeal cancer (7,8). At present, the diagnosis of IPF is usually based on clinical manifestations, imaging features, and lung biopsy. However, it is difficult to make an accurate early diagnosis with simple methods (9). Due to the lack of accurate diagnosis and effective treatment, IPF is progressive in most patients and is associated with a poor prognosis. Therefore, exploring new biomarkers for the diagnosis of IPF is very important to improve the prognosis of IPF patients.
The pathogenesis of IPF is very complicated, and not yet fully understood. The majority of studies believe that the abnormal repair of alveolar epithelial cells after injury is the main mechanism of IPF (10). Many environmental and genetic risk factors, such as smoking, inhaled suspended particles, viral infections, cell aging, and genetic mutations, all cause repeated damage and abnormal repair of alveolar epithelium (11). Studies have shown that the onset of IPF is related to the recruitment of fibroblasts in an injury site of the lung and the epithelial-mesenchymal transformation (EMT) of cells. After the transformation of epithelial cells into mesenchymal phenotype, they lose contact adhesion and tip-basal polarity, gain the ability to invade and migrate, and have the interstitial characteristics of producing ECM (12). ECM deposition results from increased synthesis as well as decreased degradation that due to decrease ECM removing activity of matrix metalloproteinases (MMPs) (13). In this EMT process, transforming growth factor-β1 (TGF-β1) is considered a key factor leading to the occurrence of IPF (14). Type II alveolar epithelial cell dysfunction can also increase the susceptibility to IPF (15). Currently, with new research methods such as proteomics and transcriptomics analysis, researchers have been able to partially reveal some genes and pathways related to the pathogenesis of IPF, including TGF-β pathway, Wnt pathway, autophagy-related pathway, and immune-related pathway (16,17). Recent studies have shown that immunology plays an important role in the occurrence and development of IPF (18). For example, Toll-like receptor 4 (TLR4) is involved in the regeneration and renewal of type II alveolar epithelial cells, affecting the repair of lung injury and the formation of fibrosis (19,20). Macrophage infiltration and high levels of reactive oxygen species (ROS) have been shown to be the key characteristics of lung fibrosis (21). Immune cells can affect the host’s defense function, regulate the stability of the local microenvironment and the production and degradation of ECM, thereby affecting the process of pulmonary fibrosis (22). Another study investigated the interaction between immune T cells and lung myofibroblasts. The result indicated that myofibroblasts possess Fas/FasL-pathway-dependent characteristics that enable them to avoid immune surveillance and leading to pulmonary fibrosis (23). Cell-type Identification by Estimating Relative Subsets of RNA Transcripts (CIBERSORT) is a biological information analysis tool that evaluates the proportion of immune cells based on RNA-seq and obtains different immune cell ratios from samples (24). It has been widely used to analyze the infiltration of immune cells in a variety of human immune-related diseases, such as tumors, osteoarthritis, and lupus nephritis (25-27). Li et al. (28) also developed a hypoxia-immune-related prediction model for the prognosis of IPF by using CIBERSORT. Different from previous studies, we analyzed the immune-related genes which can be utilized as diagnostic markers for IPF by machine learning. Moreover, we investigated the association between hub immune-related genes and immune cell infiltration in IPF.
Firstly, we downloaded the IPF gene chip data set from the Gene Expression Omnibus (GEO) database, analyzed the differential genes, and further screened immune-related genes (IRGs) by using machine learning-based methods, which might be used as biomarkers of IPF diagnosis. We used the GSE150910 cohort which is different from Li et al. Subsequently, we used CIBERSORT to analyze the differences in immune infiltration between IPF tissues and normal tissues in 22 immune cell subgroups. In addition, we also studied the relationship between IRGs and infiltrating immune cells, and conducted clinical verification to better understand the molecular immune mechanism in the development of IPF. We present the following article in accordance with the MDAR reporting checklist (available at https://dx.doi.org/10.21037/apm-21-2676).
Methods
Data collection in GEO database
The chip data of the IPF samples and the lung tissue samples of the control group were downloaded from the GEO database (https://www.ncbi.nlm.nih.gov/geo/). All participants were diagnosed with IPF by pathological biopsy. The GEO patients with IPF included in this study all met the IPF diagnostic criteria stated by the consensus statement of the American Thoracic Association and the European Respiratory Association. The lung tissue samples of the normal control group were from patients without IPF, including paracancerous tissues of lung cancer patients and lung tissues of lung transplant patients. The included data sets were GSE150910 (n=206) (29), GSE10667 (n=38) (30), and GSE32537 (n=169) (31). The clinical characteristics of patients in the 3 independent data sets are summarized in Table 1. The gene set of IRGs came from the ImmPort database (https://immport.niaid.nih.gov/home).
Table 1
GSE No. | Sample number | Ages | IPF patients (male/female) | Sample type | Chip platform | Authors |
---|---|---|---|---|---|---|
GSE32537 | IPF sample [119] + NL [50] | 62.55±8.75 | 77/42 | Lung tissue | GPL622 | Yang et al. |
GSE110147 | IPF sample [22] + NL [11] | 62±6 | 17//5 | Lung tissue | GPL622 | Cecchini et al. |
GSE150910 | IPF sample [103] + NL [103] | 60.3±8.3 | 57/46 | Lung tissue | GPL24676 | Furusawa et al. |
IPF, idiopathic pulmonary fibrosis; GEO, Gene Expression Omnibus; NL, normal lung tissue.
Screening of differentially expressed IRGs in IPF
The “DEseq2” program package in R software (version 3.6.1, https://www.r-project.org/) was used to analyze the differential genes of the original data of GSE150910, and the unpaired Student’s t-test was used to calculate the P value. Differentially expressed genes (DEGs) were selected with |log2FC| >1 and corrected P value [false discovery rate (FDR)] <0.05 as the threshold. A volcano map was drawn of the expressed genes, the Venn diagram method was used to take the intersection of the obtained genes and the IRGs in the ImmPort database, and a heat map was drawn of the intersection genes.
Enrichment analysis of biological functions of IRGs
Kyoto encyclopedia of genes and genomes (KEGG) pathway enrichment analysis and Gene Ontology (GO) functional analysis were used to annotate differentially expressed IRGs from biological process (BP), molecular function (MF), and cellular components (CCs). Employing KEGG pathway enrichment analysis can provide high-level pathway functions and biological information through large-scale molecular data sets. The above analysis used the Database for Annotation, Visualization, and Integrated Discovery (DAVID) to analyze the biological function of the differential gene online, the threshold was set at the corrected P value (FDR value) <0.05, and the result was visualized using the “ggplot” program package in the R software.
Screening and verification of diagnostic markers
The diagnostic markers of IPF were screened using least absolute shrinkage and selection operator (LASSO) regression, random forest algorithm, and support vector machine-recursive feature elimination (SVM-RFE). Due to the large sample size of IPF and the same chip annotation platform in the GSE32537 and GSE10667 data sets, the two data sets were merged into an independent data set after batch correction using the “SVA” program package in the R software. The combined data set was used as a verification set to analyze the diagnostic efficacy of the biomarkers obtained from GSE150910. The LASSO regression algorithm was conducted with the “glmnet” package, the random forest algorithm with the “randomForest” package, and the SVM-RFE with the “e1071” package. The same IRGs screened from the three algorithms were verified with the collected clinical samples and TGF-β-treated A549 cells and HBE cells.
Tissue and cell lines
A total of 18 patients with IPF admitted to the First Affiliated Hospital of Chengdu Medical College from January 2018 to December 2020 were recruited. All patients were diagnosed in accordance with the IPF diagnostic criteria of the 2018 American Thoracic Association and European Respiratory Association consensus statement (32). A total of 18 IPF tissues were taken from patients via lung biopsy. Normal lung tissue (5 cm away from tumor tissue) from 21 patients with lung cancer resection was collected as the control group. All tissues were collected at the First Affiliated Hospital of Chengdu Medical College. This study was approved by the Ethics Committee of the First Affiliated Hospital of Chengdu Medical College (2020CYFYIRB-BA-101) in compliance with the Declaration of Helsinki (as revised in 2013). Informed consent was taken from all the patients.
Human adenocarcinoma cell lines (A549) and human bronchial epithelial cells (HBE) were purchased from the Cell Bank of Type Culture Collection of Chinese Academy of Sciences (Shanghai, China). Cells were cultured in Roswell Park Memorial Institute-1640 (RPMI-1640) medium containing 10% fetal bovine serum (FBS), 100 U/mL penicillin and 100 mg/mL streptomycin (Gibco, Amarillo, TX, USA) at 37 °C with 5% CO2. The A549 cells or HBE cells were treated with recombinant human TGF-β1 (PeproTech, East Windsor, NJ, USA) at a concentration of 10 ng/mL for 48 h before further analysis.
Real-time quantitative polymerase chain reaction
Total RNA was extracted from lung tissues or A549 cells or HBE cells with TRIzol reagent (Invitrogen, Carlsbad, CA, USA). The RNA concentration was measured using a NanoDrop 2000 spectrophotometer (Thermo Fisher Scientific, Waltham, MA, USA). Then, 1,000 ng of total RNA was reverse transcribed using PrimeScript RT kit (TaKaRa, Dalian, China), and then complementary DNA (cDNA) was amplified with SYBR PreMix Ex Taq (TaKaRa, Dalian, China). Glyceraldehyde 3-phosphate dehydrogenase (GAPDH) was selected as the internal reference, and 2-∆∆Ct values were used for relative quantitative analysis of expression. The primers used in this study were listed in Table 2.
Table 2
Gene | Primer sequences (5'-3') |
---|---|
PLXNA4 | F: TCTCAGTACAACGTGCTG |
R: TAGCACTGGATCTGATTGC | |
SLIT2 | F: TGCCCATCAATGCGTTCTCCTAC |
R: TTCGTACAGCCGCACTTCACCACT | |
FOXP3 | F: GTCGATGTCCATGAATACAACCT |
R: CAAGGCTAATGACGGCAAAC | |
GAPDH | F: CATCACCATCTTCCAGGAGCG |
R: TGACCTTGCCCACAGCCTTG |
Western blotting
After they had been treated for 48 h, cells were collected and washed with pre-cooled phosphate-buffered saline (PBS), and then cell lysis buffer was added to extract total protein. Total protein concentration was determined using protein quinolinic acid method [bicinchoninic (BCA) method]. Then, protein samples were loaded and run through 10% sodium dodecyl sulfate-polyacrylamide gel electrophoresis (SDS-PAGE), followed by transferring to polyvinylidene fluoride (PVDF) membrane. After blocking in 5% skim milk for 2 h, the membrane was incubated with primary antibody overnight at 4 °C. The next day, the membrane was washed with tris-buffered saline with Tween 20 (TBST) for 3 times, and incubated with secondary antibody at room temperature for 2 h. Images were obtained with the Bio-Rad imaging system after washing (Bio-Rad Laboratories, Hercules, CA, USA). The signal was normalized using Image J software, and each group of experiments was repeated 3 times. In this experiment, the following antibodies were used: anti-PLXNA4 (1:1,000; Abcam, Shanghai, China), anti-SLIT2 (1:2,000; Abcam), and anti-GAPDH (1:5,000; Abcam).
Evaluation of immune cell infiltration
Samples with P<0.05 were screened after uploading the gene expression matrix data in GSE150910 to CIBERSORT, and the immune cell infiltration matrix was obtained. We used the “ggplot2” program package to draw violin plots of immune cell infiltration in IPF tissue and normal lung tissue.
Correlation analysis between diagnostic markers and infiltrating immune cells
The “ggstatsplot” software package was used to perform Spearman correlation analysis on the selected diagnostic markers and infiltrating immune cells, and the results were visualized. Immune cells with the largest absolute value of the correlation coefficient were selected for experimental verification.
Statistical analysis
The software GraphPad 8.0 (
Results
Data processing and screening of differential genes
A total of 1,692 differential genes were selected from the gene expression matrix of the GSE150910 data set using R software, of which 566 were up-regulated genes and 1,126 were down-regulated genes (Figure 1). After intersection with 1,811 immune gene sets, 171 candidates different IRGs were obtained, of which 74 were down-regulated genes and 97 were up-regulated (Figure 2A,2B).
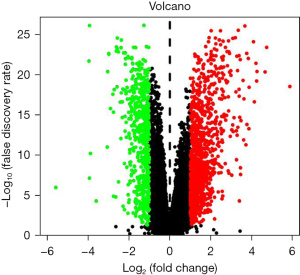
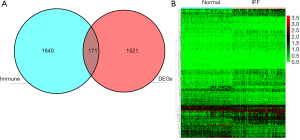
Functional enrichment
The GO analysis results showed that DEGs were mainly related to BPs such as positive regulation of secretion, leukocyte migration, second-messenger-mediated signaling, and involved in external side of plasma membrane, cytoplasmic vesicle lumen, and vesicle lumen, as well as other CCs and receptor ligand activity, cytokine activity, cytokine receptor binding, and other molecular roles. The KEGG pathway enrichment analysis results showed that 171 differentially expressed IRGs were mainly enriched in cytokine-cytokine receptor interaction, neuroactive ligand-receptor interaction, viral protein interaction with cytokine, and cytokine receptor and other pathways (Figure 3).
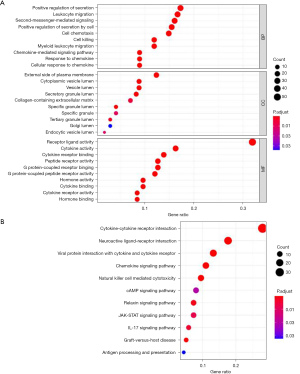
Screening and verification of diagnostic markers
A total of 31 genes were identified from the differential IRGs as diagnostic markers of IPF using the LASSO regression algorithm (Figure 4A). Among the differential IRGs, 26 were identified as diagnostic markers of IPF after performing the random forest algorithm (condition setting Mean Decrease Gini >1) (Figure 4B). The SVM-RFE algorithm was used to identify 19 genes from differential IRGs as diagnostic markers of IPF (Figure 4C). We then took the intersection of the genes obtained by the 3 algorithms, and finally obtained 2 diagnostic markers (PLXNA4, SLIT2) (Figure 4D). In the GSE150910 data set, PLXNA4 was significantly high expressed in IPF tissues (P<0.05), and SLIT2 was significantly low expressed in IPF tissues (P<0.05) (Figure 4E,4F). We merged the GSE32537 and GSE10667 into 1 data set as the validation set. Principal component analysis (PCA) showed that the samples were randomly distributed after batch correction (Figure 5A,5B). In the GSE150910 data set, when PLXNA4 and SLIT2 were used for combined diagnosis, AUC =0.966 (95% CI: 0.931 to 0.986) (Figure 6A). In the validation set, when PLXNA4 and SLIT2 were used for combined diagnosis, AUC =0.911 (95% CI: 0.857 to 0.949) (Figure 6B), indicating that PLXNA4 and SLIT2 have high diagnostic value. In order to further confirm the differential expression of PLXNA4 and SLIT2 in IPF, we performed real-time quantitative polymerase chain reaction (RT-qPCR) detection with clinical samples (18 cases of IPF and 21 cases of control group). The detailed information about IPF patients is listed in Table S1. The results showed that the combined diagnosis of PLXNA4 and SLIT2 still had high potency, with AUC =0.899 (95% CI: 0.760 to 0.972) (Figure 6C). Besides, the difference of messenger RNA (mRNA) levels of PLXNA4 and SLIT2 in IPF tissues and normal lung tissues were consistent with the results of bioinformatics analysis (Figure 6D,6E, P<0.05). In addition, we also tested the expression of PLXNA4 and SLIT2 after the treatment of TGF-β1 in A549 cells and HBE cells. As shown in Figure 7A and Figure 7B, in A549 cells stimulated by TGF-β1, the mRNA level of PLXNA4 was significantly increased, and the mRNA level of SLIT2 was significantly decreased (P<0.05). We also used western blotting to analyze the expression of PLXNA4 and SLIT2 protein in A549 cells after TGF-β1 treatment. The results showed that PLXNA4 protein was upregulated and SLIT2 protein was downregulated (P<0.05). The difference was statistically significant, as shown in Figure 7C-7E. Similar results were also seen in HBE cells (Figure S1).
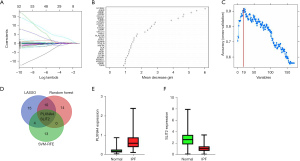
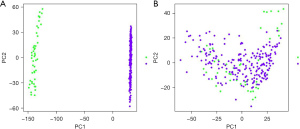
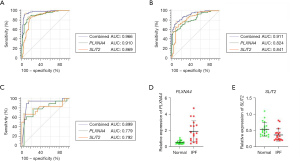
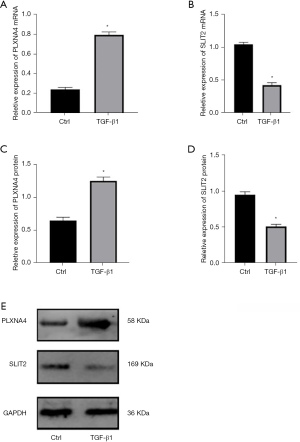
Immune cell infiltration
The violin plot of the difference in infiltration of 22 immune cells showed that compared with the normal control group, there were more infiltration in memory B cells, plasma cells, regulatory T cells (Tregs), follicular helper T cells, mast cells, M0 macrophages, and γT cells, while resting CD4 cells, resting natural killer (NK) cells, monocytes, and eosinophils were less infiltrated, as shown in Figure 8.
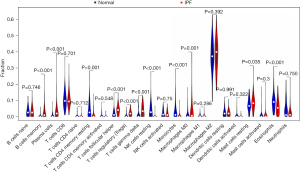
The correlation in PLXNA4, SLIT2, and infiltrating immune cells
Correlation analysis showed that SLIT2 was negatively correlated with Tregs, follicular helper T cells, γT cells, and memory B cells, which was positively correlated with resting NK cells, monocytes, and neutrophils (Figure 9A); However, PLXNA4 was positively correlated with Tregs, follicular helper T cells, and γT cells, while negatively correlated with neutrophils, monocytes, and resting NK cells (Figure 9B). It is worth noting that the absolute value of the correlation coefficient between PLXNA4 and Tregs and SLIT2 and Tregs was the highest. In order to further verify the correlation between PLXNA4, SLIT2, and Tregs, we used RT-qPCR to detect the expression of FOXP3 in clinical samples, which is the function-related molecule of Tregs (33). The results suggested that compared with normal tissues, mRNA level of FOXP3 in IPF tissues was significantly overexpressed (P<0.05, Figure 10A). The mRNA level of FOXP3 was positively correlated with the mRNA level of PLXNA4 (r=0.637, P=0.012), but negatively correlated with the mRNA level of SLIT2 (r=−0.582, P=0.003) (Figure 10B,10C).
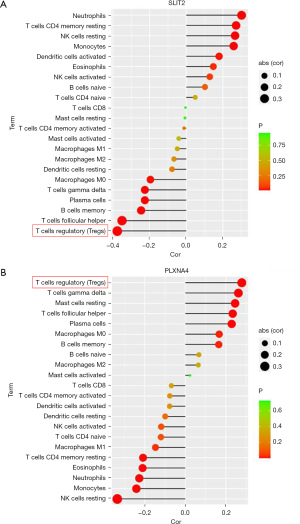
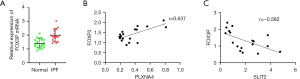
Discussion
A serious life-threatening chronic disease, IPF is characterized by abnormal lung remodeling and ECM deposition. The pathogenesis of IPF is still unclear, and effective treatment methods have not been well developed (34). Therefore, it is very important to study the molecular mechanism of biomarkers for the onset and development of IPF, as well as to find the therapeutic target of IPF. Due to the lack of early diagnostic indicators for IPF, patients often miss their best opportunity for treatment, leading to progressive disease progression. Studies believe that immune cell infiltration can clear aging alveolar epithelial cells and play an important role in the occurrence and development of IPF (35). Therefore, it is of since for the improvement of prognosis to find specific immune-related markers and analyze the types of immune cells infiltrated by IPF. With the rapid development of science and technology, bioinformatics, as an emerging interdisciplinary subject, is widely used in the study of the pathogenesis of diseases. It can identify genes that play important roles in the development of diseases and discover new therapeutic targets for disease treatment. It also provides a powerful strategy for screening molecular markers (36). In this study, we attempted to use bioinformatics methods to determine the diagnostic markers of IPF, and further used the CIBERSORT tool to explore the role of immune cell infiltration in IPF.
The IPF expression profile data set were downloaded from the GEO database and we screened a total of 171 differentially expressed IRGs. The GO enrichment analysis showed that these genes were related to BPs including positive regulation of secretion, leukocyte migration, second-messenger-mediated signaling, and related to CCs such as external side of plasma membrane, cytoplasmic vesicle lumen, vesicle lumen, and effects of receptor ligand activity, cytokine activity, and cytokine receptor binding. The KEGG pathway enrichment analysis suggested that 171 differentially expressed IRGs were mainly enriched in cytokine-cytokine receptor interaction, neuroactive ligand-receptor interaction, viral protein interaction with cytokine and cytokine receptor, and interleukin-17 (IL-17) signaling pathway. The above results suggest that immune response plays an important role in the occurrence of IPF. Yang et al. (37) showed that when macrophages were exposed to the outer membrane vesicles of gram-negative bacteria, they could drive the release of IL-17B through TLR2/4, and then induce alveolar epithelial cells to secrete chemokines and growth factors, leading to the occurrence of pulmonary fibrosis. This report is consistent with the IL-17 pathway predicted in the KEGG enrichment pathway in this study. Wang et al. also suggested that IL-17 could induce EMT in A549 cells via Smad2/3 activation mediated by TGF-β1 (38). Wynn et al. (39) also reported that the development of pulmonary fibrosis was closely related to helper T lymphocytes, involving multiple cytokines produced by helper T lymphocytes and macrophages, such as IL-4 and IL-13. Results of the above research and this study suggest that immune response plays an important role in the occurrence and development of IPF. Susceptible/aging lung tissues are repeatedly damaged and repaired, leading to the abnormality of alveolar epithelial cells, which is currently the recognized pathogenesis of IPF. Most experimental evidence supports that the immune system is involved in damage repair and collagen deposition of IPF. At the frontier of immunity, they sense and respond to dangerous stimuli, ultimately modulating the lung’s response at the level of immune cells, and play a vital role in lung homeostasis, inflammation, and fibrosis mechanisms. Therefore, we chose TGF-β1 to stimulate A549 cells and HBE cells to verify the results of bioinformatics analysis.
The SVM-RFE is a machine learning method based on support vector machine, which filters the best variables by subtracting the feature vector generated by SVM (40). The LASSO regression is also a machine learning method, which determines variables by finding λ value when the classification error is the smallest (41). The random forest algorithm is an ensemble learning algorithm based on decision trees, which is mainly used to deal with classification and regression problems. The random forest algorithm can use the Bootstrap resampling technology to randomly select multiple samples with the same number of the original data sample set to form a sample subset, and each sample subset was used to build a decision tree, and then merge the prediction results of multiple decision trees to get the best variables (42). In this study, the mean decrease gini of node impurity was used as an index to measure the importance of a variable. The higher the value, the greater the impact of the variable on classification. By combining the above three learning methods, PLXNA4 and SLIT2 were identified as diagnostic markers of IPF. In the GEO data set and the clinical samples, PLXNA4 was high expressed in IPF tissues, and SLIT2 was low expressed in IPF tissues. In the GSE150910 data set and validation set, PLXNA4 combined with SLIT2 showed a high diagnostic efficiency in diagnosing IPF, with AUC values >0.7, and this phenomenon had been verified in clinical samples. These results suggest that PLXNA4 and SLIT2 may play a role in the occurrence and development of IPF and can be used as diagnostic markers of IPF.
The PLXNA4 gene encodes the Plexin-A4 protein, a member of the neuroreceptors, which is the receptor of secreted semaphorin 3 (Sema3A) and semaphore 6 (Sema6A) and mediates the effects of a variety of semaphores (43). It does not have intrinsic kinase activity, but it can trigger the activity of Plexins-related receptor and non-receptor tyrosine kinases through receptor-ligand binding. After Plexin-A4 is activated by Sema3A, it can exert certain biological functions, such as regulating cytoskeleton movement, cell adhesion, migration, and integrin functions, which are closely related to pulmonary fibrosis (44). Wen et al. believed that Plexin-A4 was necessary for the activation of GTPase Rac1 induced by TLRs. Semaphorin 3A, as the ligand of Plexin-A4, could exacerbate the cytokine storm caused by TLR agonists and bacterial sepsis (45). The TLRs are transmembrane recognition receptors widely involved in human immune response and the first line of defense for human innate immunity (46). Studies have shown that TLR4 can mediate the remodeling of lung tissue after injury, recognize specific cytokines, promote the proliferation of fibroblasts, and produce a large amount of ECM (47). Given that Plexin-A4 participates in the body’s immune inflammatory response through interacting with TLRs, we speculate that PLXNA4 may be involved in the immune mechanism of IPF. In this study, the expression of Plexin-A4 in A549 cells and HBE cells treated with TGF-β1 was also significantly increased, verifying that PLXNA4 participates in the process of pulmonary fibrosis mediated by TGF-β1, but the mechanism still needs in-depth studies. The SLIT2 gene belongs to SLIT family and is mainly expressed in the kidney. As a secreted ECM protein, the receptor of SLIT2 is Robo immunoglobulin (48). Studies have shown that the SLIT2/ROBO1 signaling pathway is involved in the angiogenesis of glomerular endothelial cells in the early stage of diabetes, which is also involved in the kidney inflammation and fibrosis induced by TGF-β1. The SLIT2 gene is low expressed in renal fibrotic tissues, and the EMT process of renal tubular epithelial cells can be inhibited by the overexpression of SLIT2 (49). Due to the similarity of pathogenesis of renal fibrosis and lung fibers, the significant decrease of SLIT2 expression in A549 cells and HBE cells suggested that SLIT2 may inhibit the formation of pulmonary fibrosis. Huang et al. (50) also believed that the expression of SLIT2 in human fibrotic lung tissue was lower than that in non-fibrotic lung tissue. The negative regulation of SLIT2 on TGF-β1-induced pulmonary fibrosis may be related to the regulation of miR-424. Previous research has shown that PLXNA4 and SLIT2 were involved in the occurrence and development of IPF, suggesting that PLXNA4 and SLIT2 can be used as diagnostic markers of IPF, which has also been verified in this study with a small sample size. However, it is still necessary to expand the sample size to verify the diagnostic value of PLXNA4 and SLIT2.
In order to further explore the role of immune cell infiltration in IPF, CIBERSORT was used to comprehensively evaluate IPF immune infiltration. We found that memory B cells, plasma cells, follicular helper T cells, Tregs, gamma-delta T cells, M0 macrophages, mast cells, and neutrophils infiltrated more, while CD4 memory T cells, NK cells, monocytes, and eosinophils infiltrated less. Plasma cells, also called effector B cells, are derived from B cells and participate in humoral immunity. It has been reported that B-cell activating factor and CD20+ B cells are abnormal in IPF lung tissues (51,52). Follicular helper T cells have been identified as a new type of helper T cells. Studies have found that follicular helper T cells have obvious proliferation and pathological activation in the peripheral blood of IPF patients (53). Studies have shown that Tregs can promote the release of TGF-β1 and the deposition of collagen during lung injury (54). In the peripheral blood of IPF patients, the number of Tregs is significantly increased, and the ratio of Tregs/Th17 is in an imbalanced state. Gruber et al. (55) performed flow cytometry on the bronchoalveolar lavage fluid (BALF) of IPF patients and found that there was no significant difference in gamma-delta T cells between IPF patients and the control group. This phenomenon is different from the results of this study, which might be due to the difference in samples and detection techniques, and needs to be further verified. Mast cells also play an important role in the development of pulmonary fibrosis. When histamine H1 and angiotensin II from mast cells bind to the corresponding receptors on the surface of fibroblasts, the proliferation of fibroblasts, the secretion of TGF-β1, and the synthesis of collagen are promoted, thereby promoting the occurrence of fibrosis (56). There is no research on the role of M0 macrophages, monocytes, and resting NK cells in IPF.
Through analyzing the correlation between PLXNA4 and SLIT2 and immune cells, we found that the absolute value of the correlation coefficient between Tregs, PLXNA4, and SLIT2 was the largest, Tregs were positively correlated with PLXNA4, and negatively correlated with SLIT2. We then verified the results of biosynthesis by detecting Tregs-specific marker (FOX3P) in clinical samples, and results suggested that Tregs participated in the immune regulation of IPF and played an important role in maintaining the body’s self-tolerance and immune homeostasis. The study found that the number and proportion of Tregs in the peripheral blood of IPF patients increased, as well as the proportion of TGF-β1-expressed Tregs (57). Birjandi et al. (58) found that after pulmonary fibrosis induced by bleomycin in mice, the number of Tregs in the lung tissue increased. Bleomycin may cause changes in the phenotype and function of Tregs, leading to the destruction of immune homeostasis in the lungs, and promote the formation of pulmonary fibrosis. However, the role of Tregs in the process of pulmonary fibrosis is still controversial. Studies have shown that the number of Tregs in the peripheral blood and BALF of IPF patients is reduced and the inhibitory ability of Tregs to Th cells is impaired (59). The difference to this study may be because the samples included in this study were mainly IPF lung tissue specimens and were detected by PCR, and the sample size was small. In addition, the mechanism of Tregs in pulmonary fibrosis is complex, which is related to the stage of pulmonary fibrosis, the immune microenvironment in the lung, and the interaction with other T cell subsets (60). Therefore, further studies are needed to verify the results of this study and clarify the complex interaction between genes and immune cells.
There are also some limitations of this study. The CIBERSORT analysis was based on limited gene expression profile data, and these data do not include the interaction of immune cells and the plasticity of immune cell phenotypes, which may have led to certain biases in the results. Besides, this study was predominantly a second mining and analysis of the data set based on previously published data. Although clinical samples and experimental verification were also carried out, the included sample size was relatively small. Furthermore, only lung tissues were involved, and the sample size still needs to be expanded. Meanwhile, the detection of serum and BALF of patients with IPF should be multi-dimensionally verified.
Conclusions
In summary, PLXNA4 and SLIT2 were shown to be diagnostic markers of IPF. We also found that memory B cells, plasma cells, follicular helper T cells, Tregs, gamma-delta T cells, M0 macrophages, and resting mast cells may be involved in the occurrence and development of IPF. In addition, Tregs are positively correlated with PLXNA4, and negatively correlated with SLIT2. Tregs may play an important role in the occurrence and development of IPF. Further research on Tregs may determine new targets for IPF immunotherapy and help improve the immunomodulatory treatment effect of IPF patients.
Acknowledgments
Funding: None.
Footnote
Reporting Checklist: The authors have completed the MDAR reporting checklist. Available at https://dx.doi.org/10.21037/apm-21-2676
Data Sharing Statement: Available at https://dx.doi.org/10.21037/apm-21-2676
Conflicts of Interest: All authors have completed the ICMJE uniform disclosure form (available at https://dx.doi.org/10.21037/apm-21-2676). The authors have no conflicts of interest to declare.
Ethical Statement: The authors are accountable for all aspects of the work in ensuring that questions related to the accuracy or integrity of any part of the work are appropriately investigated and resolved. This study was approved by the Ethics Committee of the First Affiliated Hospital of Chengdu Medical College (2020CYFYIRB-BA-101) in compliance with the Declaration of Helsinki (as revised in 2013). Informed consent was taken from all the patients.
Open Access Statement: This is an Open Access article distributed in accordance with the Creative Commons Attribution-NonCommercial-NoDerivs 4.0 International License (CC BY-NC-ND 4.0), which permits the non-commercial replication and distribution of the article with the strict proviso that no changes or edits are made and the original work is properly cited (including links to both the formal publication through the relevant DOI and the license). See: https://creativecommons.org/licenses/by-nc-nd/4.0/.
References
- Wakwaya Y, Brown KK. Idiopathic Pulmonary Fibrosis: Epidemiology, Diagnosis andOutcomes. Am J Med Sci 2019;357:359-69. [Crossref] [PubMed]
- Kershaw CD, Batra K, Torrealba JR, et al. Characteristics and evaluation of acute exacerbations in chronic interstitial lung diseases. Respir Med 2021;183:106400 [Crossref] [PubMed]
- Raghu G, Collard HR, Egan JJ, et al. An official ATS/ERS/JRS/ALAT statement: idiopathic pulmonary fibrosis: evidence-based guidelines for diagnosis and management. Am J Respir Crit Care Med 2011;183:788-824. [Crossref] [PubMed]
- Krauss E, Gehrken G, Drakopanagiotakis F, et al. Clinical characteristics of patients with familial idiopathic pulmonary fibrosis (f-IPF). BMC Pulm Med 2019;19:130. [Crossref] [PubMed]
- Enomoto N, Naoi H, Aono Y, et al. Acute exacerbation of unclassifiable idiopathic interstitial pneumonia: comparison with idiopathic pulmonary fibrosis. Ther Adv Respir Dis 2020;14:1753466620935774 [Crossref] [PubMed]
- Biondini D, Balestro E, Sverzellati N, et al. Acute exacerbations of idiopathic pulmonary fibrosis (AE-IPF): an overview of current and future therapeutic strategies. Expert Rev Respir Med 2020;14:405-14. [Crossref] [PubMed]
- Caminati A, Lonati C, Cassandro R, et al. Comorbidities in idiopathic pulmonary fibrosis: an underestimated issue. Eur Respir Rev 2019;28:190044 [Crossref] [PubMed]
- Liu P, Zhao L, Gu Y, et al. LncRNA SNHG16 promotes pulmonary fibrosis by targeting miR-455-3p to regulate the Notch2 pathway. Respir Res 2021;22:44. [Crossref] [PubMed]
- Lynch DA, Sverzellati N, Travis WD, et al. Diagnostic criteria for idiopathic pulmonary fibrosis: a Fleischner Society White Paper. Lancet Respir Med 2018;6:138-53. [Crossref] [PubMed]
- Krempaska K, Barnowski S, Gavini J, et al. Azithromycin has enhanced effects on lung fibroblasts from idiopathic pulmonary fibrosis (IPF) patients compared to controls corrected. Respir Res 2020;21:25. [Crossref] [PubMed]
- Yao MY, Zhang WH, Ma WT, et al. microRNA-328 in exosomes derived from M2 macrophages exerts a promotive effect on the progression of pulmonary fibrosis via FAM13A in a rat model. Exp Mol Med 2019;51:1-16. [Crossref] [PubMed]
- Beisang DJ, Smith K, Yang L, et al. Single-cell RNA sequencing reveals that lung mesenchymal progenitor cells in IPF exhibit pathological features early in their differentiation trajectory. Sci Rep 2020;10:11162. [Crossref] [PubMed]
- Deng Z, Fear MW, Suk Choi Y, et al. The extracellular matrix and mechanotransduction in pulmonary fibrosis. Int J Biochem Cell Biol 2020;126:105802 [Crossref] [PubMed]
- Qian W, Cai X, Qian Q, et al. lncRNA ZEB1-AS1 promotes pulmonary fibrosis through ZEB1-mediated epithelial-mesenchymal transition by competitively binding miR-141-3p. Cell Death Dis 2019;10:129. [Crossref] [PubMed]
- Schuliga M, Pechkovsky DV, Read J, et al. Mitochondrial dysfunction contributes to the senescent phenotype of IPF lung fibroblasts. J Cell Mol Med 2018;22:5847-61. [Crossref] [PubMed]
- Todd JL, Neely ML, Overton R, et al. Peripheral blood proteomic profiling of idiopathic pulmonary fibrosis biomarkers in the multicentre IPF-PRO Registry. Respir Res 2019;20:227. [Crossref] [PubMed]
- Chanda D, Otoupalova E, Smith SR, et al. Developmental pathways in the pathogenesis of lung fibrosis. Mol Aspects Med 2019;65:56-69. [Crossref] [PubMed]
- Wang J, Sun L, Nie Y, et al. Protein Kinase C δ (PKCδ) Attenuates Bleomycin Induced Pulmonary Fibrosis via Inhibiting NF-κB Signaling Pathway. Front Physiol 2020;11:367. [Crossref] [PubMed]
- Wang Y, Zhang X, Feng X, et al. Expression of Toll-like receptor 4 in lungs of immune-suppressed rat with Acinetobacter baumannii infection. Exp Ther Med 2016;12:2599-605. [Crossref] [PubMed]
- Armstrong L, Medford AR, Uppington KM, et al. Expression of functional toll-like receptor-2 and -4 on alveolar epithelial cells. Am J Respir Cell Mol Biol 2004;31:241-5. [Crossref] [PubMed]
- He C, Larson-Casey JL, Davis D, et al. NOX4 modulates macrophage phenotype and mitochondrial biogenesis in asbestosis. JCI Insight 2019;4:126551 [Crossref] [PubMed]
- Liu M, Zeng X, Wang J, et al. Immunomodulation by mesenchymal stem cells in treating human autoimmune disease-associated lung fibrosis. Stem Cell Res Ther 2016;7:63. [Crossref] [PubMed]
- Wallach-Dayan SB, Elkayam L, Golan-Gerstl R, et al. Cutting edge: FasL(+) immune cells promote resolution of fibrosis. J Autoimmun 2015;59:67-76. [Crossref] [PubMed]
- Newman AM, Liu CL, Green MR, et al. Robust enumeration of cell subsets from tissue expression profiles. Nat Methods 2015;12:453-7. [Crossref] [PubMed]
- Chen B, Khodadoust MS, Liu CL, et al. Profiling Tumor Infiltrating Immune Cells with CIBERSORT. Methods Mol Biol 2018;1711:243-59. [Crossref] [PubMed]
- Deng YJ, Ren EH, Yuan WH, et al. GRB10 and E2F3 as Diagnostic Markers of Osteoarthritis and Their Correlation with Immune Infiltration. Diagnostics (Basel) 2020;10:171. [Crossref] [PubMed]
- Cao Y, Tang W, Tang W. Immune cell infiltration characteristics and related core genes in lupus nephritis: results from bioinformatic analysis. BMC Immunol 2019;20:37. [Crossref] [PubMed]
- Li X, Cai H, Cai Y, et al. Investigation of a Hypoxia-Immune-Related Microenvironment Gene Signature and Prediction Model for Idiopathic Pulmonary Fibrosis. Front Immunol 2021;12:629854 [Crossref] [PubMed]
- Furusawa H, Cardwell JH, Okamoto T, et al. Chronic Hypersensitivity Pneumonitis, an Interstitial Lung Disease with Distinct Molecular Signatures. Am J Respir Crit Care Med 2020;202:1430-44. [Crossref] [PubMed]
- Konishi K, Gibson KF, Lindell KO, et al. Gene expression profiles of acute exacerbations of idiopathic pulmonary fibrosis. Am J Respir Crit Care Med 2009;180:167-75. [Crossref] [PubMed]
- Yang IV, Coldren CD, Leach SM, et al. Expression of cilium-associated genes defines novel molecular subtypes of idiopathic pulmonary fibrosis. Thorax 2013;68:1114-21. [Crossref] [PubMed]
- Raghu G, Remy-Jardin M, Myers JL, et al. Diagnosis of Idiopathic Pulmonary Fibrosis. An Official ATS/ERS/JRS/ALAT Clinical Practice Guideline. Am J Respir Crit Care Med 2018;198:e44-68. [Crossref] [PubMed]
- Zhang G, Zhang W, Li B, et al. MicroRNA-200c and microRNA- 141 are regulated by a FOXP3-KAT2B axis and associated with tumor metastasis in breast cancer. Breast Cancer Res 2017;19:73. [Crossref] [PubMed]
- Li X, Yu X, Xie Y, et al. Critical appraisal of the quality of clinical practice guidelines for idiopathic pulmonary fibrosis. Ann Transl Med 2020;8:1405. [Crossref] [PubMed]
- Waters DW, Blokland KEC, Pathinayake PS, et al. Fibroblast senescence in the pathology of idiopathic pulmonary fibrosis. Am J Physiol Lung Cell Mol Physiol 2018;315:L162-72. [Crossref] [PubMed]
- Gauthier J, Vincent AT, Charette SJ, et al. A brief history of bioinformatics. Brief Bioinform 2019;20:1981-96. [Crossref] [PubMed]
- Yang D, Chen X, Wang J, et al. Dysregulated Lung Commensal Bacteria Drive Interleukin-17B Production to Promote Pulmonary Fibrosis through Their Outer Membrane Vesicles. Immunity 2019;50:692-706.e7. [Crossref] [PubMed]
- Wang T, Liu Y, Zou JF, et al. Interleukin-17 induces human alveolar epithelial to mesenchymal cell transition via the TGF-β1 mediated Smad2/3 and ERK1/2 activation. PLoS One 2017;12:e0183972 [Crossref] [PubMed]
- Wynn TA. Fibrotic disease and the T(H)1/T(H)2 paradigm. Nat Rev Immunol 2004;4:583-94. [Crossref] [PubMed]
- Sanz H, Valim C, Vegas E, et al. SVM-RFE: selection and visualization of the most relevant features through non-linear kernels. BMC Bioinformatics 2018;19:432. [Crossref] [PubMed]
- Cheung-Lee WL, Link AJ. Genome mining for lasso peptides: past, present, and future. J Ind Microbiol Biotechnol 2019;46:1371-9. [Crossref] [PubMed]
- Yang L, Wu H, Jin X, et al. Study of cardiovascular disease prediction model based on random forest in eastern China. Sci Rep 2020;10:5245. [Crossref] [PubMed]
- Kang SS, Kurti A, Wojtas A, et al. Identification of plexin A4 as a novel clusterin receptor links two Alzheimer's disease risk genes. Hum Mol Genet 2016;25:3467-75. [Crossref] [PubMed]
- Yamashita N, Usui H, Nakamura F, et al. Plexin-A4-dependent retrograde semaphorin 3A signalling regulates the dendritic localization of GluA2-containing AMPA receptors. Nat Commun 2014;5:3424. [Crossref] [PubMed]
- Wen H, Lei Y, Eun SY, et al. Plexin-A4-semaphorin 3A signaling is required for Toll-like receptor- and sepsis-induced cytokine storm. J Exp Med 2010;207:2943-57. [Crossref] [PubMed]
- Takeda K, Akira S. Toll Receptors. Curr Protoc Immunol 2002;52:14.12.1-14.12.11.
- Ebener S, Barnowski S, Wotzkow C, et al. Toll-like receptor 4 activation attenuates profibrotic response in control lung fibroblasts but not in fibroblasts from patients with IPF. Am J Physiol Lung Cell Mol Physiol 2017;312:L42-55. [Crossref] [PubMed]
- Chaturvedi S, Robinson LA. Slit2-Robo signaling in inflammation and kidney injury. Pediatr Nephrol 2015;30:561-6. [Crossref] [PubMed]
- Yuen DA, Huang YW, Liu GY, et al. Recombinant N-Terminal Slit2 Inhibits TGF-β-Induced Fibroblast Activation and Renal Fibrosis. J Am Soc Nephrol 2016;27:2609-15. [Crossref] [PubMed]
- Huang Y, Xie Y, Abel PW, et al. TGF-β1-induced miR-424 promotes pulmonary myofibroblast differentiation by targeting Slit2 protein expression. Biochem Pharmacol 2020;180:114172 [Crossref] [PubMed]
- François A, Gombault A, Villeret B, et al. B cell activating factor is central to bleomycin- and IL-17-mediated experimental pulmonary fibrosis. J Autoimmun 2015;56:1-11. [Crossref] [PubMed]
- Todd NW, Scheraga RG, Galvin JR, et al. Lymphocyte aggregates persist and accumulate in the lungs of patients with idiopathic pulmonary fibrosis. J Inflamm Res 2013;6:63-70. [Crossref] [PubMed]
- Asai Y, Chiba H, Nishikiori H, et al. Aberrant populations of circulating T follicular helper cells and regulatory B cells underlying idiopathic pulmonary fibrosis. Respir Res 2019;20:244. [Crossref] [PubMed]
- Boveda-Ruiz D, D'Alessandro-Gabazza CN, Toda M, et al. Differential role of regulatory T cells in early and late stages of pulmonary fibrosis. Immunobiology 2013;218:245-54. [Crossref] [PubMed]
- Gruber R, Pforte A, Beer B, et al. Determination of gamma/delta and other T-lymphocyte subsets in bronchoalveolar lavage fluid and peripheral blood from patients with sarcoidosis and idiopathic fibrosis of the lung. APMIS 1996;104:199-205. [Crossref] [PubMed]
- Wygrecka M, Dahal BK, Kosanovic D, et al. Mast cells and fibroblasts work in concert to aggravate pulmonary fibrosis: role of transmembrane SCF and the PAR-2/PKC-α/Raf-1/p44/42 signaling pathway. Am J Pathol 2013;182:2094-108. [Crossref] [PubMed]
- Galati D, De Martino M, Trotta A, et al. Peripheral depletion of NK cells and imbalance of the Treg/Th17 axis in idiopathic pulmonary fibrosis patients. Cytokine 2014;66:119-26. [Crossref] [PubMed]
- Birjandi SZ, Palchevskiy V, Xue YY, et al. CD4(+)CD25(hi)Foxp3(+) Cells Exacerbate Bleomycin-Induced Pulmonary Fibrosis. Am J Pathol 2016;186:2008-20. [Crossref] [PubMed]
- Kotsianidis I, Nakou E, Bouchliou I, et al. Global impairment of CD4+CD25+FOXP3+ regulatory T cells in idiopathic pulmonary fibrosis. Am J Respir Crit Care Med 2009;179:1121-30. [Crossref] [PubMed]
- Hou Z, Ye Q, Qiu M, et al. Increased activated regulatory T cells proportion correlate with the severity of idiopathic pulmonary fibrosis. Respir Res 2017;18:170. [Crossref] [PubMed]