Prevalence and risk factors of diabetes mellitus: a community-based sectional survey
Introduction
The prevalence of diabetes is increasing globally and alarmingly so in Asia. According to the latest global diabetes distribution map released by the International Diabetes Federation (IDF) in 2019 (1), there were 463 million diabetic patients worldwide, including 114 million cases in China (2,3); an increase of 11.57% in the global figure compared to 2015. On average, 1 in 11 adults between the ages of 20 and 79 suffers from the disease. The prevalence of adult diabetes and prediabetes (PD) in China has been increasing year by year since the first epidemiological survey of 300,000 people was performed in 1980 and indicated a prevalence of only 0.67% (4). Epidemiological studies have documented an incidence rate of 4.5% in the urban population over 18 years, which was an increase of 15 times compared with 1980 compared with the 2002 National Diabetes Survey (5,6), and by 2013, the incidence of adult diabetes was as high as 10.9%. The increasing prevalence of diabetes mellitus (DM) has become a global public health concern in the 21st century. Over the past few decades, China’s urbanization and rising living standards have led to reduced physical activity and unhealthy diets which, in combination with an already ageing population, are factors behind the soaring prevalence of the disease. There were many reports on the prevalence and the risk factors related to different diabetes complications from the different areas and different cities in China. Shanghai as the high urbanization and developed city, the prevalent trend of DM among the residents and the management are very important and representative.
Among patients with diabetes, 76.4% reported at least one kind of complication which was proven to be the cause of death (7). More than half of individuals with diabetes remain undiagnosed in China, making it the country with the largest number of diabetic patients in the world (8,9). It was reported that 60.7% of adults with diabetes had not previously been diagnosed and 66.7% of diabetes patients had not received regular examination as recommended by clinical guidelines (1,8). With the accelerated of population aging, the older the age, the higher the risk factors of metabolic disease. Besides the aging factor, other major factors include overweight, hypertension, hyperlipidemia, and inheritance. Consequently, how to detect and monitor these elder individuals earlier and guide self-management in the community is very important. This study was designed to explore the prevalence of diabetes and other metabolic parameters in healthy aged 45 and above community inhabitants in Shanghai.
We present the following article in accordance with the SURGE reporting checklist (available at https://dx.doi.org/10.21037/apm-21-3251).
Methods
Subjects and data collection
A total of 6,183 inhabitants aged over 45-years and living in the Shanghai Songjiang district participated in the survey from 2011 to 2013. All inhabitants who met the age requirements received notice in advance, understood the purpose of the research, and agreed to participate in the flow of information and sample collection. Participants were requested to complete a general information survey form which sought their name, gender, age, and contact information, and their clinical history of alcoholism, smoking, hypertension, diabetes, thyroid, liver, renal disease, family history of cardiovascular disease, history of drug treatment. The comprehensive survey was administered by trained research staff. Subjects were also evaluated for fasting glucose and an oral glucose tolerance test (OGTT), and those who failed to complete the test were excluded. All procedures performed in this study involving human participants were in accordance with the Declaration of Helsinki (as revised in 2013). This study was validated and approved by the Ethics Committee of Shanghai General Hospital (No. 2009KY037), and informed consent was taken from all the subjects.
Physical examination
All subjects underwent a physical examination in which their weight, height, waist circumference, hip circumference, systolic blood pressure (SBP), diastolic blood pressure (DBP), and heart rate were recorded. Hip circumference (HC) was measured in standing subjects, and the body mass index (BMI) was calculated as body weight/heights2 in kg/m2. The waist-hip ratio (WHR) was calculated as weight circumference/hip circumference.
Biochemical measurement
Peripheral blood samples were collected from all subjects in the morning after overnight fasting for over 10 hours. All subjects underwent a 75-g OGTT, and biochemical parameters, including plasma glucose concentrations, serum lipid metabolic related, total cholesterol (TC), high density lipoprotein cholesterol (HDL-C), low density lipoprotein cholesterol (LDL-C), and triglycerides (TG) were measured by an automatic biochemistry analyzer. The fasting insulin concentration, serum level of thyroid-stimulation hormone (TSH), and free triiodothyronine (FT3)/free tetraiodothyronine concentration (FT4) were monitored by electrochemiluminescence analyzer. Urea nitrogen (Ur) and creatinine (Cr), alanine aminotransferase levels were also detected.
Definitions
- We defined the different groups as (i) normal glucose regulation (NGR), in which fasting glucose <6.1 mmol/L and 2 h postprandial glucose <7.8 mmol/L; (ii) impaired fasting glucose (IFG), in which fasting glucose ≥6.1 and <7.0 mmol/L and 2 h postprandial glucose <7.8 mmol/L (10); (iii) impaired glucose tolerance (IGT), in which fasting glucose <6.1 mmol/L and 2 h postprandial glucose ≥7.8 and <11.1 mmol/L (11); (iv) combined glucose impaired (CGI), in which fasting glucose ≥6.1 and <7.0 mmol/L, and 2 h postprandial glucose ≥7.8 and <11.1 mmol/L (11); and (v) NDDM, in which fasting glucose ≥7.0 mmol/L and/ or 2 h postprandial glucose ≥11.1, and there was no diabetes history.
- According to the China standard (WS/T428-2013) (11), the overweight standard was BMI ≥24 kg/m2.
- According to the WHO and China standard for central obesity, the WHR ≥0.9 for males, ≥0.85 for females.
- HOMA-IR = fasting insulin (FINS) × fasting glucose (FPG)/22.5.
- HOMA-β =20× fasting insulin/fasting glucose −3.5.
Exclusion criteria
- History of DM;
- Thyroid dysfunction;
- Chronic renal failure;
- Liver disease;
- History of cancer;
- Diagnosed lipid metabolic dysfunction;
- Failure to finish the examination and test;
- Taking any oral drugs.
Statistical analysis
SPSS 22.0 software was used for statistical analysis of all data. The data were presented as the means ± standard deviation for the ratio and interval data following the normal distribution. For data not following the normal distribution, it is median and interquartile range, For count data, the number of subjects and the composition ratio with the percentages within parentheses. The χ2-test was used to compare the proportion of the subjects between different groups divided by the dichotomized groups of metabolic parameters and glucose status. One-way analysis of variance and Kruskal-Wallis tests were used to analyze the statistical differences of the mean and median values of various parameters among different groups divided by the glucose status. All the reported P values were two-tailed, and those less than 0.05 were considered statistically significant.
Results
General information and glucose metabolic status
Of the 6,813 inhabitants initially screened, 1,213 met the inclusion criteria. Of these, 49.8% (604/1,213) had NGR and 13.7% (166/1,213) were confirmed as NDDM. The ratio of PD, including IFG), IGT, and combined IFG/IGT (CGI) was 36.5%, as shown as in Table 1. Interestingly, a feature of male PD subjects was the high ratio of fasting glucose (12.7%) (Table 1, Figure 1A, Table S1), whereas IGT was higher in female PD subjects (19.62%), as shown as in Table 1, Figure 1B, Table S1. The parameters linked to glucose metabolism are presented by median (interquartile range) as seen in Table 1, which shows there were significant differences between the NGR groups and each hyper-glucose group (P<0.001), except the HOMA-β between the NGR group and IGT group without significant difference.
Table 1
Characteristics | NGR (n=604) | IFG (n=118) | IGT (n=207) | CGI (n=118) | NDDM (n=166) | P value | P<0.05 |
---|---|---|---|---|---|---|---|
Age (years) | 57.0 (12.0) | 59.0 (13.0) | 59.0 (12.0) | 59.5 (12.0) | 61.0 (10.0) | ||
Male, n (%) (n=525) | 271 (51.6) | 67 (12.7) | 72 (13.7) | 43 (8.19) | 72 (13.71) | ||
Female, n (%) (n=688) | 333 (48.4) | 51 (7.42) | 135 (19.62) | 75 (10.9) | 94 (13.66) | ||
FPG | 5.4 (0.5) | 6.3 (0.3) | 5.7 (0.5) | 6.4 (0.4) | 7.2 (1.2) | <0.001 | a,b,c,d, |
PPG | 6.2 (1.4) | 6.6 (1.3) | 8.7 (1.5) | 8.8 (1.6) | 12.6 (4.5) | <0.001 | a,b,c,d, |
HbA1C | 5.4 (0.4) | 5.6 (0.4) | 5.6 (0.5) | 5.8 (0.4) | 6.1 (1.1) | <0.001 | a,b,c,d, |
FINS | 6.0 (4.5) | 7.3 (6.0) | 7.7 (5.1) | 9.6 (5.9) | 9.7 (7.6) | <0.001 | a,b,c,d, |
HOMA-IR | 1.5 (1.1) | 2.1 (1.7) | 1.9 (1.3) | 2.8 (1.7) | 3.1 (2.5) | <0.001 | a,b,c,d, |
HOMA-β | 65.7 (43.7) | 50.1 (48.1) | 69.6 (47.6) | 65.7 (42.3) | 61.3 (49.4) | <0.001 | a,c,d |
Statistical indicators: a: NGR group vs. IFG group; b: NGR group vs. IGT group; c: NGF group vs. CGI group; d: NGR group vs. NDDM group. NGR, normal glucose regulation; IFG, impaired fasting glucose; IGT, impaired glucose tolerance; CGI, combined IFG/IGT; NDDM, new diagnosis diabetes mellitus; FPG, fasting plasma glucose; PPG, postprandial plasma glucose; HbA1c, hemoglobin A1c; FINS, fasting insulin; HOMA-IR, HOMA insulin resistance; HOMA-β, HOMA of beta cell function.
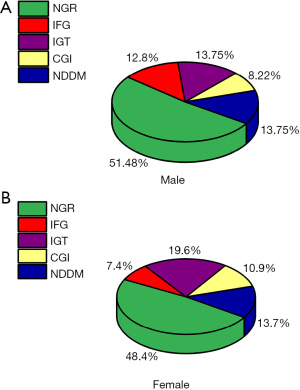
Age and sex constituent ratio at different glucose status
Among the 1,213 subjects, 525 were male (43%) and 688 were female (67%). Based on the statistical analysis, we substantiated that age was a risk factor for hyper-glucose and metabolic syndrome. As shown as in Figure 2A,2B, the percentage of PD and NDDM increased with age. Further, the ratio of NDDM was higher in 61–65-year-old subjects and the ratio of PD was higher in 56–60-year-old subjects. To explore the incidence trends with different sex and different ages, we compared the age constituent ratios at different glucose status among male and female subjects. As can be seen in Figure 2C,2D, the 61–65-year-old age group had the highest ratio in male hyper-glucose subjects, while the 56–60-year-old age group was the highest in female hyper-glucose subjects. We also found the 51–55-year-old male subjects had a higher ratio to be diagnosed as DM, compared to the similar age range in female subjects.
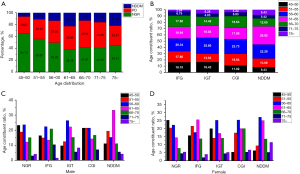
BMI and WHR related to glucose status
BMI and WHR are important indexes for obesity and are also reported to be related to glucose metabolism. Here, we substantiated that the two indexes were significantly different between subjects with normal glucose status and those with hyper-glucose status (Table 2). We also analyzed the relationship between the two indexes and glucose status at different ages and between genders. According to the China Standard, BMI ≥24 is defined as overweight, and Figure 3A,3B and Table S2 show the number of male overweight subjects was 325, accounting for 26.5% (325/1,213) of total subjects and 61.9% (325/525) of all male subjects. Among these, the 56–60-year-old male group showed a higher ratio of PD, while the 61–65-year-old male group showed a higher ratio of NDDM. There were 387 female overweight subjects accounting for 31.9% (387/1,213) of total subjects and 56.3% (387/688) of all female subjects. Among them, 56–60-year-old females showed a higher ratio with PD and NDDM.
Table 2
Indexes | NGR (n=604) | IFG (n=118) | IGT (n=207) | CGI (n=118) | NDDM (n=166) | P value | P<0.05 |
---|---|---|---|---|---|---|---|
BMI | 24.0±3.4 | 24.6±2.9 | 25.4±3.4 | 25.6±3.4 | 26.1±3.3 | <0.001 | a,b,c,d |
WHR | 0.90 (0.09) | 0.913 (0.07) | 0.910 (0.08) | 0.901 (0.07) | 0.92 (0.07) | <0.001 | a,b,c,d |
Statistical indicators: a: NGR group vs. IFG group; b: NGR group vs. IGT group; c: NGF group vs. CGI group; d: NGR group vs. NDDM group. NGR, normal glucose regulation; IFG, impaired fasting glucose; IGT, impaired glucose tolerance; CGI, combined IFG/IGT; NDDM, new diagnosis diabetes mellitus; BMI, body mass index; WHR, waist-hip ratio.
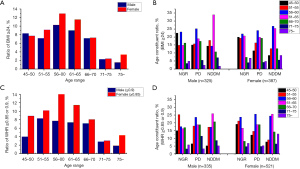
According to the WHO standard, a WHR ≥0.9 (male) or WHR ≥0.85 (female) signifies central obesity. We found 63.8% (335/525) of male subjects and 91.4% (629/688) of female subjects exhibited abnormal WHR, and the 56–65-year age group were higher ratio linked to hyper-glucose, as shown as in Figures 3C,3D and Table S3.
Other metabolic indexes at different glucose status groups
Fat metabolism is highly related to glucose regulation. In our study community, fat rating, fat mass, TC, and TG were increased significantly when IGT and diagnosis diabetes occurred, but not only IFG (P<0.001), as shown in Table 3 and Figure 4A.
Table 3
Indexes | NGR (n=604) | IFG (n=118) | IGT (n=207) | CGI (n=118) | NDDM (n=166) | P value | P<0.05 |
---|---|---|---|---|---|---|---|
Fat rating | 28.8 (11.1) | 28.2 (10.7) | 32.4 (11.7) | 32.6 (11.6) | 32.2 (12.1) | <0.001 | b,c,d |
Fat mass | 17.5 (7.1) | 18.3 (7.8) | 19.8 (8.4) | 19.8 (6.4) | 20.9 (7.6) | <0.001 | b,c,d |
Visceral fat rating | 9.0 (6.0) | 10.0 (7.0) | 9.0 (5.0) | 9.0 (7.0) | 10.3 (6.3) | <0.001 | c |
TC (mmol/L) | 5.1±0.8 | 5.2±0.9 | 5.2±0.9 | 5.4±0.9 | 5.5±1.0 | <0.001 | b,c,d |
HDL-C (mmol/L) | 1.6 (0.5) | 1.6 (0.6) | 1.5 (0.5) | 1.6 (0.6) | 1.5 (0.5) | 0.162 | |
LDL-C (mmol/L) | 2.8 (1.0) | 2.8 (1.0) | 2.9 (1.0) | 3.0 (1.2) | 3.1 (1.0) | <0.001 | c,d |
TG (mmol/L) | 1.2 (0.8) | 1.2 (0.9) | 1.4 (1.0) | 1.8 (1.5) | 1.7 (1.1) | <0.001 | b,c,d |
Statistical indicators: a: NGR group vs. IFG group; b: NGR group vs. IGT group; c: NGF group vs. CGI group; d: NGR group vs. NDDM group. NGR, normal glucose regulation; IFG, impaired fasting glucose; IGT, impaired glucose tolerance; CGI, combined IFG&IGT; NDDM, new diagnosis diabetes mellitus; TC, total cholesterol; HDL-C, high density lipoprotein cholesterol; LDL-C, low density lipoprotein cholesterol; TG, triglycerides.
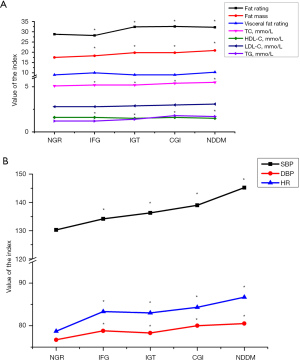
Cardiovascular indicators, such as blood pressure and heart rate, are also key factors coordinated with glucose metabolism, and Table 4 and Figure 4B show the three key indicators, SBP, DBP, and HR were enhanced significantly when glucose level increased.
Table 4
Parameters | NGR (n=604) | IFG (n=118) | IGT (n=207) | CGI (n=118) | NDDM (n=166) | P value | P<0.05 |
---|---|---|---|---|---|---|---|
SBP | 130.3 (19.6) | 134.2 (22.4) | 136.3 (19.7) | 139.0 (20.8) | 145.2 (21.2) | <0.001 | b,c,d |
DBP | 76.7 (12.3) | 78.8 (11.6) | 78.3 (12.3) | 80.7 (16.5) | 80.5 (11.1) | <0.001 | b,c,d |
HR | 78.7 (14.0) | 83.3 (18.3) | 83.0 (15.3) | 84.3 (18.8) | 86.7 (19.3) | <0.001 | a,b,c,d |
Statistical indicators: a: NGR group vs. IFG group; b: NGR group vs. IGT group; c: NGF group vs. CGI group; d: NGR group vs. NDDM group. SBP, systolic blood pressure; DBP, diastolic blood pressure; HR, heart rate; NGR, normal glucose regulation; IFG, impaired fasting glucose; IGT, impaired glucose tolerance; CGI, combined IFG&IGT; NDDM, new diagnosis diabetes mellitus.
We also observed the value change of ALT, Ur, Cr, FT3, FT4, and TSH at different glucose status in the community, as shown in Table 5, and there was a significant difference in ALT between the NGR and IGT groups or between NGR and NDDM groups. The Cr level in the IFG group was significant compared to the normal glucose group, and only FT3 showed a significant difference between the NGR group and the IFG group of thyroid indicators.
Table 5
Parameters | NGR (n=604) | IFG (n=118) | IGT (n=207) | CGI (n=118) | NDDM (n=166) | P value | P<0.05 |
---|---|---|---|---|---|---|---|
ALT | 18.0 (11.0) | 18.0 (11.0) | 19.0 (13.0) | 18.0 (11.0) | 23.0 (15.0) | <0.001 | b,d |
Ur | 5.3 (1.7) | 5.2 (1.9) | 5.2 (1.5) | 5.6 (1.8) | 5.4 (1.7) | 0.956 | |
Cr | 63.0 (18.0) | 67.0 (17.0) | 58.0 (21.0) | 59.0 (19.0) | 62.5 (20.0) | <0.001 | b |
TSH | 2.3 (2.0) | 2.3 (2.0) | 2.2 (2.0) | 2.5 (2.0) | 2.3 (1.0) | 0.599 | |
FT3 | 5.1 (0.9) | 5.4 (0.9) | 5.0 (0.8) | 5.2 (0.9) | 5.0 (0.9) | 0.002 | a |
FT4 | 15.6 (2.7) | 15.5 (2.8) | 15.5 (3.1) | 15.5 (3.9) | 15.5 (2.9) | 0.482 |
Statistical indicators: a: NGR group vs. IFG group; b: NGR group vs. IGT group; c: NGF group vs. CGI group; d: NGR group vs. NDDM group. NGR, normal glucose regulation; IFG, impaired fasting glucose; IGT, impaired glucose tolerance; CGI, CGI, combined IFG/IGT; NDDM, new diagnosis diabetes mellitus. ALT, alanine aminotransferase; Ur, urea nitrogen; Cr, creatinine. TSH, Thyroid Stimulating Hormone; FT3, free triiodothyronine, FT4, free tetraiodothyronine.
Discussion
There are approximately 425 million DM patients worldwide, of whom 114 million are in China (12,13). In the past 30 years, with the rapid economic growth and increasing population, the prevalence of type 2 diabetes in China has substantially increased (14,15). However, there are enormous variations in diabetes prevalence among urban and rural areas and wealthy and poorer regions. A 2010 Chinese survey found the prevalence of diabetes ranged from 14.3% in urban and 10.3% in rural areas (16). Higher-income urban counties also had a higher average prevalence rate (13.1%), than rural low-income counties (8.7%) (17), while in a 2013 survey these figures were 12.6% and 9.5%, respectively (18). However, many patients with diabetes go undiagnosed, and while 13.7% of subjects in the present study had been diagnosed with diabetes newly and 36.5% with prediabetes, more than half of cases were undiagnosed before this study. Differences in regional economic development and living habits have different effects on the incidence of diabetes. A recent epidemiological survey (7) enrolled a total of 75,880 volunteers from 31 provinces and administrative regions in mainland China between 2015 to 2017 and revealed the prevalence of diabetes in Chinese adults to be 12.8%. In Shanghai area, a study run in 1998 resulted that age-standardized prevalence was not statistically different between males and females: respectively 2.54% and 2.14% for DM and 1.42% and 1.31% for IGT (19). Another study was run among Shanghai residents aged 35 and above in 2013 and found that the prevalent of DM were 19.3% in men and 15.8% in women as well as 19.1%, 15.4%, and 16.1% in urban, suburban, and rural residents, respectively (20). In the current survey, we investigated inhabitants of the Shanghai Songjiang district to explore their glucose status and management. Songjiang district is in southwest of Shanghai, suburban area. The subjects recruited are the residents of Shanghainese and the survey from the subjects has the important reference value for the prevalent of DM in the specific area of Shanghai. Our community-based survey showed the prevalence of NDDM was 13.7% and the ratio of PD reached as high as 36.5%. Importantly, the results also showed over 50% of inhabitants were unaware of the importance of blood glucose levels and high risk of metabolic syndrome. Considering aged 45 and above residents without DM history, we didn’t subgroup to Type 1 or Type 2 DM for further discussion.
In a meta-analysis of 35 reports on type 2 DM in China from 1997 to 2017 (21), Zhou et al. found a greater number of males were affected than females. In our research, the incidence of abnormal glucose metabolism in women (56.6%) was higher than in men (42.4%), especially for those aged 56–60-year-old. Studies have reported that compared with men, postmenopausal women have a significantly higher incidence of metabolic diseases such as obesity, type 2 diabetes, and coronary heart disease. Aekplakorn et al. evaluated six groups; a normal diet group (NOR), normal diet & ovariectomized group (NOR + OVX), normal diet & ovariectomized & estrogen replacement group (NOR + OVX + E2), high-fat diet group (HF), high-fat diet & ovariectomized group (HF + OVX), and high-fat diet group & ovariectomized & estrogen replacement group (HF + OVX + E2), and after 20 weeks, the blood glucose level and related indicators were monitored (22). The results showed that estrogen therapy could improve insulin resistance by reducing weight, and by acting on adipose tissue directly, affecting the level of adipokines to reduce visceral fat mass and improve insulin sensitivity. In addition, Guo investigated a new pathway for estrogen combining with FOXO1 to reduce insulin resistance (23). These two studies indicate 56–60-year-old females face the challenge of abnormal glucose regulation induced by lower estrogen levels, and estrogen therapy could not only alleviate menopause syndrome, but also control their glucose regulation.
To better understand the risk factors associated with diabetes, we ran single factor and multiple factor regression analysis (raw data: Table S4), and found age, BMI, WHR, TG, fat mass, fat rating, SBP, and HR were related risk factors. Abnormal lipid and abnormal glucose metabolism were mutual inducements, and both were high-risk factors for metabolic syndrome. BMI and WHR were increased significantly in the PD and NDDM groups, and insulin resistance was significantly higher than that in the normal group, while the function of β-cells in the pancreatic islets was significantly reduced. These results are consistent with the fact that obesity can cause insulin resistance (24). What surprised us was that 91.4% of female subjects from this community suffered higher WHR with central obesity, and the ratio of 56–60-year-old was higher than other age range groups.
A recent study examined physical examination parameters and the results of a 75-g OGTT, fasting blood glucose, fasting insulin, serum uric acid, and other biochemical indicators in 2,082 cases of healthy individuals. The correlation between BMI and pancreatic β-cell function was also investigated (25), and the results showed a positive relationship between BMI and the level of HOMA-β and HOMA-IR, and a negative relationship between BMI and insulin level. The researchers proposed that obesity induces the compensatory increase of islet cell function, but insulin secretion was decreased significantly. The mechanisms and the relationship of BMI and islet function, as well as BMI and blood glucose metabolism require further study. As a risk factor, blood pressure was also associated with type 2 diabetes, which was substantiated in our results. Aronow proposed that it is important to control arterial hypertension in adults with DM, and clinicians should target blood pressure at 120–130/80 mmHg to reduce the risk of stroke, with close monitoring for all drug-related adverse events (26). Thyroid hormones are critically important for regulating energy balance and the metabolism of glucose and lipids, and there are many reports on the relationship between thyroid hormone levels and insulin sensitivity. Wang reported that low free T3 (FT3) was significantly associated with decreased HOMA-IR (27). Insulin resistance is also associated with low thyroid hormone levels in non-diabetic individuals, and, paradoxically, it has been known for decades that hyperthyroidism is associated with IR. However, hypothyroidism has recently been reported to be associated with IR. Despite the exclusion of patients with thyroid dysfunction in our study and the regression analysis showing negative results, we also found the level of FT3 was significantly corelated with impaired fasting glucose. The mechanisms underlying the relationship between thyroid function and glucose regulation are intriguing and require further study.
An urban lifestyle with less exercise, increased stress, and sweet food are the key factors causing obesity. On the other hand, obesity induces abnormal glucose regulation. Our study in the community of Shanghai Songjiang district explored the prevalence of PD and DM. The simple survey focuses on the community-based residents in suburban area of Shanghai and hint the potential high risk of the regional economic development and living habits to prevalent of diabetes. The results highlight the importance of managing the health status of individuals with sub-clinical disease to control the incidence of diabetes and other metabolic syndromes. The strategy of community-based education and supervision should be done to urge weight control, more exercise and the bio-parameters regular screening, especially for aging residents.
Acknowledgments
The authors thank all the study participants and all the research staff for their participation and contribution.
Funding: The study was supported by the National Natural Science Foundation of China (No. NSFC81870596 and No. NSFC81870594), Clinical research plan of SHDC (No. SHDC2020CR1016B), the third round cooperation project of Songjiang district municipal Health Commission (No. 0702N18003) and the Shanghai Hongkou District Health Commission for Medical Scientific Research project (No. SHDHC1901-03).
Footnote
Reporting Checklist: The authors have completed the SURGE reporting checklist. Available at https://dx.doi.org/10.21037/apm-21-3251
Data Sharing Statement: Available at https://dx.doi.org/10.21037/apm-21-3251
Conflicts of Interest: All authors have completed the ICMJE uniform disclosure form (available at https://dx.doi.org/10.21037/apm-21-3251). The authors have no conflicts of interest to declare.
Ethical Statement: The authors are accountable for all aspects of the work in ensuring that questions related to the accuracy or integrity of any part of the work are appropriately investigated and resolved. All procedures performed in this study involving human participants were in accordance with the Declaration of Helsinki (as revised in 2013). This study was validated and approved by the Ethics Committee of Shanghai General Hospital (No. 2009KY037), and informed consent was taken from all the subjects.
Open Access Statement: This is an Open Access article distributed in accordance with the Creative Commons Attribution-NonCommercial-NoDerivs 4.0 International License (CC BY-NC-ND 4.0), which permits the non-commercial replication and distribution of the article with the strict proviso that no changes or edits are made and the original work is properly cited (including links to both the formal publication through the relevant DOI and the license). See: https://creativecommons.org/licenses/by-nc-nd/4.0/.
References
- Borgnakke WS. IDF Diabetes Atlas: Diabetes and oral health - A two-way relationship of clinical importance. Diabetes Res Clin Pract 2019;157:107839 [Crossref] [PubMed]
- Benjamin EJ, Muntner P, Alonso A, et al. Heart Disease and Stroke Statistics-2019 Update: A Report From the American Heart Association. Circulation 2019;139:e56-e528. [Crossref] [PubMed]
- Guo LX. The epidemic situation and intervention strategies of diabetes mellitus. Chinese Journal of Clinical Healthcare 2020;23:433-6.
- Pan XR, Yang WY, Li GW, et al. Prevalence of diabetes and its risk factors in China, 1994. National Diabetes Prevention and Control Cooperative Group. Diabetes Care 1997;20:1664-9. [Crossref] [PubMed]
- Kharroubi AT, Darwish HM. Diabetes mellitus: The epidemic of the century. World J Diabetes 2015;6:850-67. [Crossref] [PubMed]
- Wang Z, Zou Z, Wang H, et al. Prevalence and risk factors of impaired fasting glucose and diabetes among Chinese children and adolescents: a national observational study. Br J Nutr 2018;120:813-9. [Crossref] [PubMed]
- Mao W, Yip CW, Chen W. Complications of diabetes in China: health system and economic implications. BMC Public Health 2019;19:269-80. [Crossref] [PubMed]
- American Diabetes Association. 2. Classification and Diagnosis of Diabetes: Standards of Medical Care in Diabetes-2019. Diabetes Care 2019;42:S13-28. [Crossref] [PubMed]
- Global activities of the National Center for Chronic Disease Prevention and Health Promotion (NCCDPHP), Centers for Disease Control and Prevention, USA. Promot Educ 2002;9:155-165, 175-6. [PubMed]
- World Health Organization. (1999). Definition, diagnosis and classification of diabetes mellitus and its complications: report of a WHO consultation. Part 1, Diagnosis and classification of diabetes mellitus. World Health Organization.
- Zhou Bei-FanCooperative Meta-Analysis Group of the Working Group on Obesity in China. Predictive values of body mass index and waist circumference for risk factors of certain related diseases in Chinese adults--study on optimal cut-off points of body mass index and waist circumference in Chinese adults Biomed Environ Sci 2002;15:83-96. [PubMed]
- Patterson CC, Karuranga S, Salpea P, et al. Worldwide estimates of incidence, prevalence and mortality of type 1 diabetes in children and adolescents: Results from the International Diabetes Federation Diabetes Atlas, 9th edition. Diabetes Res Clin Pract 2019;157:107842.
- Luo Z, Fabre G, Rodwin VG. Meeting the Challenge of Diabetes in China. Int J Health Policy Manag 2020;9:47-52. [Crossref] [PubMed]
- Hu D, Sun L, Fu P, et al. Prevalence and risk factors for type 2 diabetes mellitus in the Chinese adult population: the InterASIA Study. Diabetes Res Clin Pract 2009;84:288-95. [Crossref] [PubMed]
- Holmes D. Linong Ji: fighting to turn the tide against diabetes in China. Lancet 2014;383:1961. [Crossref] [PubMed]
- Xu Y, Wang L, He J, et al. Prevalence and control of diabetes in Chinese adults. JAMA 2013;310:948-59. [Crossref] [PubMed]
- Zhou M, Astell-Burt T, Bi Y, et al. Geographical variation in diabetes prevalence and detection in china: multilevel spatial analysis of 98,058 adults. Diabetes Care 2015;38:72-81. [Crossref] [PubMed]
- Wang L, Gao P, Zhang M, et al. Prevalence and Ethnic Pattern of Diabetes and Prediabetes in China in 2013. JAMA 2017;317:2515-23. [Crossref] [PubMed]
- Shi HL, Fang JC, Zhu XX. Prevalence of diabetes mellitus and associated risk factors in an adult urban population in Shanghai. Diabetes & Metabolism 1998;24:539-542. [PubMed]
- Ye R, Qing HY, Ji Y, et al. Epidemiology of Diabetes in Adults Aged 35 and Older from Shanghai, China. Biomedical and Environmental Sciences 2016;29:408-416. [PubMed]
- Zhou T, Liu X, Liu Y, et al. Meta-analytic evaluation for the spatio-temporal patterns of the associations between common risk factors and type 2 diabetes in mainland China. Medicine (Baltimore) 2019;98:e15581 [Crossref] [PubMed]
- Aekplakorn W, Chongsuvivatwong V, Tatsanavivat P, et al. Prevalence of metabolic syndrome defined by the International Diabetes Federation and National Cholesterol Education Program criteria among Thai adults. Asia Pac J Public Health 2011;23:792-800. [Crossref] [PubMed]
- Guo S. Insulin signaling, resistance, and the metabolic syndrome: insights from mouse models into disease mechanisms. J Endocrinol 2014;220:T1-T23. [Crossref] [PubMed]
- Kwon C, Sun JL, Jeong JH, et al. Humanin attenuates palmitate-induced hepatic lipid accumulation and insulin resistance via AMPK-mediated suppression of the mTOR pathway. Biochem Biophys Res Commun 2020;526:539-45. [Crossref] [PubMed]
- Chung JO, Cho DH, Chung DJ, et al. Associations among body mass index, insulin resistance, and pancreatic β-cell function in Korean patients with new-onset type 2 diabetes. Korean J Intern Med 2012;27:66-71. [Crossref] [PubMed]
- Aronow WS, Shamliyan TA. Blood pressure targets for hypertension in patients with type 2 diabetes. Ann Transl Med 2018;6:199. [Crossref] [PubMed]
- Wang CY, Yu TY, Shih SR, et al. Low total and free triiodothyronine levels are associated with insulin resistance in non-diabetic individuals. Sci Rep 2018;8:10685. [Crossref] [PubMed]