A clinical and laboratory-based nomogram for predicting nonalcoholic fatty liver disease in non-diabetic adults: a cross-sectional study
Introduction
Nonalcoholic fatty liver disease (NAFLD) is a kind of liver disease induced by metabolic stress which is closely related to insulin resistance and genetic susceptibility (1). It includes a range of disorders, such as simple steatosis, nonalcoholic steatohepatitis (NASH), liver cirrhosis, and hepatocellular carcinoma (2). As a metabolic disease, NAFLD has many extra-hepatic manifestations, including type 2 diabetes mellitus, cardiovascular disease, obstructive sleep apnea, chronic kidney disease, and osteoporosis (3). The global prevalence of NAFLD is estimated at approximately 25%, and is associated with severe comorbidities and financial burden (4). Considering all of these factors, the early detection of this disease is of great significance to enable the provision of an early intervention, thus avoiding the progression and exacerbation of NAFLD.
There is a close relationship between insulin resistance (IR) and NAFLD, with a high prevalence of NAFLD among diabetic patients (5). IR is defined clinically as the inability of a known quantity of exogenous or endogenous insulin to increase glucose uptake and utilization in an individual as much as it does in a normal population. Even if there are still insufficiently known data about elucidating the immunopathogenic mechanism behind NAFLD and its connections with diabetes, IR seems to be one of the key events that appear in both disorders (6). Epidemiological studies noted that there is a five-fold higher prevalence of NAFLD in patients with diabetes compared to that in patients without diabetes. However, this is not a reason to focus only on the incidence of NAFLD in people with diabetes because people who are suffering from insulin resistance are not necessarily diagnosed with diabetes, which results in the overlook of NAFLD in non-diabetic population. As the symptoms are not typical, non-diabetic population may not take the time or initiative to check themselves. Actually, we are obligated to pay attention to those non-diabetic population for early detection and intervention of NAFLD. There is a lack of knowledge and little research regarding the prediction of NAFLD among adults without diabetes, which means there is a lack of a convenient tool for predicting NAFLD in non-diabetic adults.
Although liver biopsy is still the gold standard for identifying NAFLD, it is regarded as impractical because of its invasiveness, poor acceptability, cost, and sampling variability (7). In recent years, significant progress has been made in the noninvasive assessment of NAFLD, including the use of imaging tests, blood chemical examination, and physical examination (8). Anthropometric indicators, such as body mass index (BMI), waist circumference (WC), and waist-to-height ratio (WHtR), can be used to screen for NAFLD, and they are simple, low-cost, and non-invasive. Nevertheless, the best indicator for predicting NAFLD has yet to be identified, and the cut-off points may differ between racial and ethnic groups; hence, more studies are needed to identify factors useful for predicting NAFLD (9). While imaging-derived proton density fat fraction (MRI-PDFF) and transient elastography can quantitatively assess liver fat (10-13), the cost of these methods limits their use to mostly clinical research. Blood biochemical indexes have been shown to be associated with disease (14-16), but there is lack of a convenient predictive model to combine these indexes together. To get the accurate diagnosis of NAFLD, detailed present history, imaging tests, blood biochemical indexes, and even liver biopsy are all needed. A personalized and novel model could help physicians make a better decision, whether a non-diabetic person should be asked about a deatiled history of drinking and medicine, to get blood tests for viral hepatitis, immune system disease and liver enzymes. In this study, we developed and validated a model to predict the risk of NAFLD among adults without diabetes. This novel model, which takes many associated risk factors into consideration, provides an accurate tool for predicting the diagnosis of the disease, and the simple nomogram has the potential to save more medical resources and make less missed diagnosis. We present the following article in accordance with the STROBE reporting checklist (available at https://apm.amegroups.com/article/view/10.21037/apm-21-2988/rc).
Methods
Study design and participants
All data used in this study were from a medical examination program, which aimed to promote public health and conduct a thorough inquiry of chronic diseases and their risk factors (17). Research data for this program were uploaded to the Dryad Digital Repository website (http://www.datadryad.org), an international, open-access repository of raw research data publicly available for users to download according to the terms of service of the Dryad database. The study was conducted in accordance with the Declaration of Helsinki (as revised in 2013).
In the original study, all participants underwent a health examination at the Murakami Memorial Hospital between 2004 and 2015. The cohort study by Okamura et al. initially enrolled 20,944 patients and then excluded those with alcoholic fatty liver disease (18), known medication usage, viral hepatitis, known liver disease, missing covariant data, age <18 years, and impaired fasting blood glucose, leaving 15,464 adults participating in the baseline analysis. In our study, in order to reach an accurate diagnosis of NAFLD, we added the following exclusion criteria: male alcohol consumption ≥210 g/week and female alcohol consumption ≥140 g/week (19). Finally, we constructed a cross-sectional study including 14,251 participants. To improve the accuracy and reliability of our analysis, 14,251 NAFLD patients were randomly divided in a ratio of 3:1 into a training dataset with 10,689 participants and a validation dataset with 3,562 participants, using the classification and regression training (caret) package in R software v. 4.0.3 (R Foundation for Statistical Computing, Vienna, Austria; https://www.R-project.org).
Data collection
All participants were encouraged to complete blood collection, abdominal ultrasonography, and a standardized, self-administered questionnaire, which included questions regarding age, gender, height, weight, WC, systolic blood pressure (SBP), diastolic blood pressure (DBP), alcohol consumption, and exercise habits. We defined regular exercisers as participants who regularly played any type of sports >1×/week (20). Taking the exclusion criteria into consideration, alcohol consumption was classified into 3 grades: non-drinking or minimal drinking (<40 g/week), light drinking (40–139 g/week), and moderate drinking (140–209 g/week). After at least 8 h of overnight fasting, blood collections were completed, and included gamma-glutamyl transferase (GGT), alanine transaminase (ALT), high-density lipoprotein cholesterol (HDL-C), aspartate aminotransferase (AST), total cholesterol (TC), hemoglobin A1c (HBA1c), total triglycerides (TG), and fasting blood glucose (FBG). Abdominal ultrasonography was performed by trained technicians and read by experienced gastroenterologists without access to any other personal data of the participants. The final diagnosis of NAFLD was made by the evaluation of 4 known criteria: vascular blurring, liver brightness, hepatorenal echo contrast, and deep attenuation.
Statistical analysis
For continuous variables, the descriptive analysis was determined according to whether the data conformed to normal distribution. Values were expressed as means ± standard deviations (SDs) or medians (interquartile ranges, IQRs) for continuous variables and numbers (percentages, %) for categorical variables. Participant characteristics were compared using the Student’s t-test or Mann-Whitney U test for continuous variables and the chi-square test for categorical variables. Univariate and multivariate logistic regression models were constructed to explore the independent factors strongly associated with NAFLD, and based on this, we constructed a prediction model. Additionally, the least absolute shrinkage and selection operator (LASSO) method was selected as a suitable tool for the reduction of high-dimensional data. Features with nonzero coefficients in the LASSO regression model were selected (Figure 1A,1B). Thus, variables for prediction were selected by multivariable logistic regression analysis, the LASSO method, and clinical experience. A nomogram is a graphical presentation which can predict the probability of a certain type of event. To evaluate the predictive ability of the model, an area under the receiver operator characteristic curve (AUROC) was performed. Sensitivity and specificity analysis were used to demonstrate the ability of prediction in the model. A calibration curve was used to correct the prediction model. Decision curve analysis (DCA) was used to evaluate the net benefit and the clinical practicability of the nomogram. A total of 1,000 bootstrap resamples were applied to the AUROC value and calibration curve. A data analysis software package, R v. 4.0.3 (R Foundation for Statistical Computing, Vienna, Austria; https://www.R-project.org), was used to carry out all statistical tests. A 2-sided P value <0.05 was considered statistically significant.
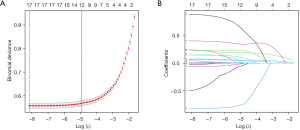
Results
Participant characteristics
In the present study, 10,689 participants aged 18–79 years old from the training dataset were included in the cross-sectional analysis. Table 1 shows that there was no significant difference between the training dataset (n=10,689) and the validation dataset (n=3,562). In the training dataset, the average age of participants was 43.6 years, 52.3% (n=5,594) of the participants were male, and 17.6% (n=1,881) of them had a diagnosis of NAFLD. In the validation dataset, the average age of participants was 43.4 years, 51% (n=1,817) of the participants were males, and 17.5% (n=623) of them had a diagnosis of NAFLD.
Table 1
Characteristics | Training dataset | Validation dataset | P value |
---|---|---|---|
Participants (n) | 10,689 | 3,562 | |
Gender, male (%) | 5,594 (52.3) | 1,817 (51.0) | 0.171 |
Age (years) | 43.6±8.9 | 43.4±8.8 | 0.168 |
BMI (kg/m2) | 22.1±3.1 | 22.1±3.2 | 0.885 |
Body weight (kg) | 60.3±11.6 | 60.1±11.7 | 0.489 |
WC (cm) | 76.2±9.1 | 76.0±9.1 | 0.297 |
ALT (IU/L) | 19.8±15.2 | 19.6±12.1 | 0.372 |
AST (IU/L) | 18.2±9.2 | 18.2±6.7 | 0.978 |
GGT (IU/L) | 19.2±16.6 | 18.9±14.8 | 0.310 |
HDL-C (mmol/L) | 1.5±0.4 | 1.5±0.4 | 0.131 |
TG (mmol/L) | 0.9±0.6 | 0.9±0.6 | 0.264 |
TC (mmol/L) | 5.1±0.9 | 5.1±0.9 | 0.138 |
HbA1c (%) | 5.2±0.3 | 5.2±0.3 | 0.811 |
FBG (mmol/L) | 5.2±0.4 | 5.1±0.4 | 0.751 |
Regular exerciser (%) | 1,852 (17.3) | 618 (17.3) | 0.974 |
SBP (mmHg) | 113.9±14.9 | 113.9±14.7 | 0.997 |
DBP (mmHg) | 71.1±10.4 | 71.1±10.2 | 0.919 |
Drinking status (%) | 0.550 | ||
Non or small (<40 g/w) | 8,077 (75.6) | 2,705 (76.0) | |
Light (40–140 g/w) | 2,089 (19.5) | 692 (19.4) | |
Moderate (140–209 g/w) | 523 (4.9) | 165 (4.6) | |
NAFLD (%) | 1,883 (17.6) | 624 (17.5) | 0.894 |
Values are expressed as means ± SDs or for continuous variables and numbers (percentages, %) for categorical variables. BMI, body mass index; ALT, alanine transaminase; AST, aspartate aminotransferase; HbA1c, hemoglobin A1c; FBG, fasting blood glucose; TG, total triglycerides; TC, total cholesterol; HDL-C, high-density lipoprotein cholesterol; WC, waist circumference; GGT, γ-glutamyl transpeptidase; SBP, systolic blood pressure; DBP, diastolic blood pressure; NAFLD, nonalcoholic fatty liver disease.
Compared with normal (non-NAFLD) participants, those with NAFLD were more likely to have the attributes of male gender, older age, higher blood pressure, and higher values of BMI, WC, ALT, AST, GGT, TC, TG, FBG, and Hba1c (all P<0.001), as shown in Table 2. The chi-square test showed no significant linear association between drinking status and risk of NAFLD (P=0.296). Higher HDL-C levels and more regular exercise patterns were observed in normal (non-NAFLD) participants than among NAFLD participants.
Table 2
Characteristics | Without NAFLD | With NAFLD | P value |
---|---|---|---|
Participants (n) | 8,806 | 1,883 | |
Gender, male (%) | 4,064 (46.2) | 1,530 (81.3) | <0.001 |
Age (years) | 43.3±9.0 | 45.0±8.4 | <0.001 |
BMI (kg/m2) | 21.3±2.6 | 25.5±3.1 | <0.001 |
Body weight (kg) | 57.8±10.0 | 72.1±11.3 | <0.001 |
WC (cm) | 74.2±7.9 | 85.9±7.8 | <0.001 |
ALT (IU/L) | 17.1±12.5 | 32.4±19.7 | <0.001 |
AST (IU/L) | 17.3±8.8 | 22.4±10.1 | <0.001 |
GGT (IU/L) | 17.2±14.2 | 28.4±22.5 | <0.001 |
HDL-C (mmol/L) | 1.5±0.4 | 1.2±0.3 | <0.001 |
TG (mmol/L) | 0.8±0.5 | 1.4±0.8 | <0.001 |
TC (mmol/L) | 5.0±0.9 | 5.4±0.9 | <0.001 |
HbA1c (%) | 5.2±0.3 | 5.3±0.3 | <0.001 |
FBG (mmol/L) | 5.1±0.4 | 5.4±0.4 | <0.001 |
Regular exerciser (%) | 1,570 (17.8) | 282 (15.0) | 0.003 |
SBP (mmHg) | 111.9±14.0 | 123.4±15.1 | <0.001 |
DBP (mmHg) | 69.7±9.9 | 77.8±10.4 | <0.001 |
Drinking status (%) | 0.296 | ||
Non or small (<40 g/w) | 6,668 (75.7) | 1,409 (74.8) | |
Light (40–140 g/w) | 1,716 (19.5) | 373 (19.8) | |
Moderate (140–209 g/w) | 422 (4.8) | 101 (5.4) |
Values are expressed as means ± SDs or for continuous variables and numbers (percentages, %) for categorical variables. NAFLD, nonalcoholic fatty liver disease; BMI, body mass index; ALT, alanine transaminase; AST, aspartate aminotransferase; HbA1c, hemoglobin A1c; FBG, fasting blood glucose; TG, total triglycerides; TC, total cholesterol; HDL-C, high-density lipoprotein cholesterol; WC, waist circumference; GGT, γ-glutamyl transpeptidase; SBP, systolic blood pressure; DBP, diastolic blood pressure.
Logistic regression analysis in the training dataset
Table 3 shows the results of univariate and multivariate regression analyses for the prediction of NAFLD. A multivariable-adjusted logistic regression model was applied to examine the association of variables with the risk of NAFLD. We logically sifted out 6 independent factors strongly associated with NAFLD to establish the model, based on which we created the nomogram. These factors included BMI, AST, HDL-C, TG, HbA1c, and DBP, all of which are easily obtained. We identified 5 of them as risk factors for NAFLD, while HDL-C was a protective factor. In this nomogram (Figure 2), a straight edge can be used to connect known values on 2 lines to determine the number of points the covariates can reach. After summing up each point, the value can be read at the line of total points; thus, we can estimate the probability of NAFLD.
Table 3
Variables | Univariate | Multivariate | |||||
---|---|---|---|---|---|---|---|
OR | 95% CI | P value | OR | 95% CI | P value | ||
Gender (male/female) | 5.057 | 4.472–5.720 | <0.001 | ||||
Age (years) | 1.021 | 1.015–1.026 | <0.001 | ||||
BMI (kg/m2) | 1.646 | 1.607–1.685 | <0.001 | 1.442 | 1.405–1.479 | <0.001 | |
Body weight (kg) | 1.129 | 1.122–1.136 | <0.001 | ||||
WC (cm) | 1.200 | 1.190–1.211 | <0.001 | ||||
ALT (IU/L) | 1.100 | 1.094–1.106 | <0.001 | ||||
AST (IU/L) | 1.087 | 1.079–1.095 | <0.001 | 1.036 | 1.028–1.045 | <0.001 | |
GGT (IU/L) | 1.038 | 1.035–1.042 | <0.001 | ||||
HDL-C (mmol/L) | 0.054 | 0.045–0.065 | <0.001 | 0.304 | 0.243–0.379 | <0.001 | |
TG (mmol/L) | 4.620 | 4.222–5.056 | <0.001 | 1.829 | 1.650–2.027 | <0.001 | |
TC (mmol/L) | 1.649 | 1.557–1.746 | <0.001 | ||||
HbA1c (%) | 4.099 | 3.491–4.813 | <0.001 | 2.422 | 1.991–2.946 | <0.001 | |
FBG (mmol/L) | 6.305 | 5.500–7.227 | <0.001 | ||||
Regular exerciser (%) | 0.812 | 0.707–0.932 | 0.003 | ||||
SBP (mmHg) | 1.053 | 1.049–1.057 | <0.001 | ||||
DBP (mmHg) | 1.078 | 1.072–1.084 | <0.001 | 1.021 | 1.014–1.028 | <0.001 | |
Drinking status | |||||||
Non or small (<40 g/w) | Ref. | ||||||
Light (40–140 g/w) | 1.029 | 0.907–1.167 | 0.660 | ||||
Moderate (140–209 g/w) | 1.133 | 0.905–1.418 | 0.277 |
Univariate and multivariate analysis for the prediction of NAFLD. NAFLD, nonalcoholic fatty liver disease; BMI, body mass index; ALT, alanine transaminase; AST, aspartate aminotransferase; HbA1c, hemoglobin A1c; FBG, fasting blood glucose; TG, total triglycerides; TC, total cholesterol; HDL-C, high-density lipoprotein cholesterol; WC, waist circumference; GGT, γ-glutamyl transpeptidase; SBP, systolic blood pressure; DBP, diastolic blood pressure; OR, odds ratio; CI, confidence interval.
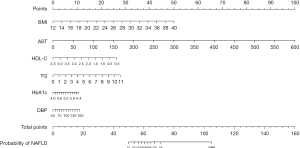
Performance of the nomogram
In the training dataset, the AUROC value of the prediction model was 0.891 (95% CI: 0.884–0.899; P<0.001). The sensitivity and specificity of the nomogram were 84.8% and 77.9%, respectively, and the cut-off was 0.627 (Figure 3A). In the validation dataset, the AUROC value was 0.902 [95% confidence interval (CI): 0.890 to 0.914; P<0.001]. The sensitivity and specificity of the nomogram were 88.5% and 77.1%, respectively, and the cut-off was 0.656 (Figure 3B). The sensitivity and specificity presented above demonstrate the good predictive ability of this model. In predictive models, AUROC can also be called the C index. C index between 0.5 and 0.7 is considered as low differentiation; 0.7–0.9 was moderate; a value greater than 0.9 is considered high. Tests were two-sided and 0.05 was set as the P value for statistical significance. As shown in Figure 4A,4B, the calibration curve used to correct the prediction model demonstrated good agreement between the training dataset and the validation dataset. In another words, this model has a high prediction accuracy because the calibration curve is close to the diagonal line. Results of the DCA of the nomogram in the training dataset and the validation dataset are shown in Figure 4C,4D. This model has good clinical utility, and it is feasible for making useful judgments because the decision curve is far from the 2 extreme curves.
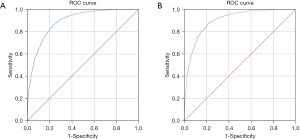
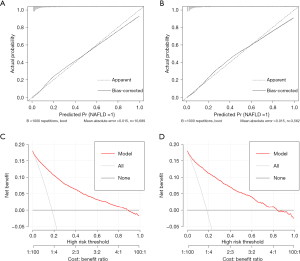
Discussion
The condition of NAFLD, which includes nonalcoholic fatty liver (NAFL) and nonalcoholic steatohepatitis (NASH), is a current public health problem (21). Compared to NAFL, NASH is more likely to cause cirrhosis and hepatocellular carcinoma (HCC). Activation of caspases, Bcl-2 family proteins, and c-Jun N-terminal kinase-induced hepatocyte apoptosis is of great significance in the activation of NAFLD/NASH (22). Large, multicenter, prospective cohort studies are still needed to assess the presence of NASH (23). In our study, the prevalence of NAFLD was 17.59%, which was lower than the global prevalence of 25% (4). Presumably, this is because 17.3% of participants in this study were regular exercisers, and exercise can prevent or delay the progression of NAFLD (24-27). There is an association between NAFLD and higher risk of all-cause mortality, and it is closely related to cardiovascular diseases (CVDs), extrahepatic malignancies, and liver-related complications. In view of the current epidemic and heavy economic burden of NAFLD, early diagnosis and screening are critical.
In our study, we developed a quantifiable nomogram, based on BMI, AST, HDL-C, TG, HbA1c, and DBP, to predict the risk of NAFLD in a Japanese population without diabetes. One of the significant factors in our prediction model was BMI, which is commonly used to measure obesity. Obesity can lead to an excessive accumulation of triglycerides and cholesterol in hepatocytes, inducing hepatic steatosis and inflammation (28). Therefore, obesity may support the prediction of a less favorable long-term prognosis (29). Some studies have shown that the alleviation of NAFLD is accompanied by a decrease in BMI (30-33). The DBP is another physically examinable feature, which reflects blood pressure level. In a recent study, NAFLD prevalence was shown to be higher in patients with hypertension compared with healthy participants (34). Liver regeneration has close links with angiocrine signals, implying a relationship between hypertension and the development of liver disease (35). The HbA1c level reflects the average blood glucose levels in the previous 2–3 months, and is a more convenient measurement than FBG as it does not require fasting (36). As a hepatic manifestation of metabolic syndrome, NAFLD usually emerges concurrently with symptoms of diabetes (37). A study suggested that a high level of HbA1c may contribute to the progression of NAFLD by mechanisms which include stimulus of the receptor for advanced glycation end products, promotion of hypoxia, and suppression of the release of nitrous oxide (NO) (38). In our study, participants with diabetes were excluded, yet HbA1c was still shown to be associated with NAFLD. Accordingly, this association was relatively more credible. The ratio of TG/HDL-C is independently related to NAFLD and could potentially be used as a predictor of NAFLD (39). The state of IR not only impacts the metabolism of TG and HDL-C (40), but also suppresses the lipolysis of adipose tissue, resulting in increased delivery of free fatty acids to the liver. In summary, IR may provide a connection between TG/HDL-C and the development of NAFLD. Lipotoxicity, oxidative stress, and metabolic inflammation in NAFLD can induce the injury of hepatocytes (41), and AST is a marker of hepatocellular injury. Therefore, AST is another significant factor in our prediction model. This study has shown that our model not only has good predictive ability, but also provides a high level of accuracy and clinical utility. Physical examinations and blood tests are easily available, and it is recommended that people with a high possibility of NAFLD undergo further examinations, such as ultrasound.
The strengths of this study are the population-based design and visualization of the predictive model. However, our study had several potential limitations. First, abdominal ultrasonography underestimates hepatic steatosis, and liver biopsy is still the gold standard of NAFLD, regardless of poor acceptability. Second, the training dataset and validation dataset are both from the Murakami Memorial Hospital in Japan. It would have been advantageous to incorporate participants from different regions for further external validation. Third, data on dietary habits and physical activity were scarce, so the predictive ability of the model is limited.
In conclusion, our nomogram offers a convenient and economical method for screening NAFLD in non-diabetic Japanese people. It is suggested that individuals at high risk of NAFLD should undergo further examinations to confirm the diagnosis of NAFLD. Our predictive model is promising for the early diagnosis and intervention of NAFLD, which are important for reducing the global burden of the disease.
Acknowledgments
We thank the Dryad Digital Repository website, Takuro Okamura and his colleagues.
Funding: This work was supported by a grant from the National Natural Science Foundation of China (No. 81873567).
Footnote
Reporting Checklist: The authors have completed the STROBE reporting checklist. Available at https://apm.amegroups.com/article/view/10.21037/apm-21-2988/rc
Peer Review File: Available at https://apm.amegroups.com/article/view/10.21037/apm-21-2988/prf
Conflicts of Interest: All authors have completed the ICMJE uniform disclosure form (available at https://apm.amegroups.com/article/view/10.21037/apm-21-2988/coif). The authors have no conflicts of interest to declare.
Ethical
Open Access Statement: This is an Open Access article distributed in accordance with the Creative Commons Attribution-NonCommercial-NoDerivs 4.0 International License (CC BY-NC-ND 4.0), which permits the non-commercial replication and distribution of the article with the strict proviso that no changes or edits are made and the original work is properly cited (including links to both the formal publication through the relevant DOI and the license). See: https://creativecommons.org/licenses/by-nc-nd/4.0/.
References
- Watt MJ, Miotto PM, De Nardo W, et al. The Liver as an Endocrine Organ-Linking NAFLD and Insulin Resistance. Endocr Rev 2019;40:1367-93. [Crossref] [PubMed]
- Kupčová V, Fedelešová M, Bulas J, et al. Overview of the Pathogenesis, Genetic, and Non-Invasive Clinical, Biochemical, and Scoring Methods in the Assessment of NAFLD. Int J Environ Res Public Health 2019;16:3570. [Crossref] [PubMed]
- Rosato V, Masarone M, Dallio M, et al. NAFLD and Extra-Hepatic Comorbidities: Current Evidence on a Multi-Organ Metabolic Syndrome. Int J Environ Res Public Health 2019;16:3415. [Crossref] [PubMed]
- Mundi MS, Velapati S, Patel J, et al. Evolution of NAFLD and Its Management. Nutr Clin Pract 2020;35:72-84. [Crossref] [PubMed]
- Khan RS, Bril F, Cusi K, et al. Modulation of Insulin Resistance in Nonalcoholic Fatty Liver Disease. Hepatology 2019;70:711-24. [Crossref] [PubMed]
- Tanase DM, Gosav EM, Costea CF, et al. The Intricate Relationship between Type 2 Diabetes Mellitus (T2DM), Insulin Resistance (IR), and Nonalcoholic Fatty Liver Disease (NAFLD). J Diabetes Res 2020;2020:3920196. [Crossref] [PubMed]
- Castera L, Friedrich-Rust M, Loomba R. Noninvasive Assessment of Liver Disease in Patients With Nonalcoholic Fatty Liver Disease. Gastroenterology 2019;156:1264-1281.e4. [Crossref] [PubMed]
- Piazzolla VA, Mangia A. Noninvasive Diagnosis of NAFLD and NASH. Cells 2020;9:1005. [Crossref] [PubMed]
- Almeida NS, Rocha R, Cotrim HP, et al. Anthropometric indicators of visceral adiposity as predictors of non-alcoholic fatty liver disease: A review. World J Hepatol 2018;10:695-701. [Crossref] [PubMed]
- Dulai PS, Sirlin CB, Loomba R. MRI and MRE for non-invasive quantitative assessment of hepatic steatosis and fibrosis in NAFLD and NASH: Clinical trials to clinical practice. J Hepatol 2016;65:1006-16. [Crossref] [PubMed]
- Caussy C, Reeder SB, Sirlin CB, et al. Noninvasive, Quantitative Assessment of Liver Fat by MRI-PDFF as an Endpoint in NASH Trials. Hepatology 2018;68:763-72. [Crossref] [PubMed]
- Tapper EB, Loomba R. Noninvasive imaging biomarker assessment of liver fibrosis by elastography in NAFLD. Nat Rev Gastroenterol Hepatol 2018;15:274-82. [Crossref] [PubMed]
- Xiao G, Zhu S, Xiao X, et al. Comparison of laboratory tests, ultrasound, or magnetic resonance elastography to detect fibrosis in patients with nonalcoholic fatty liver disease: A meta-analysis. Hepatology 2017;66:1486-501. [Crossref] [PubMed]
- Serper M, Vujkovic M, Kaplan DE, et al. Validating a non-invasive, ALT-based non-alcoholic fatty liver phenotype in the million veteran program. PLoS One 2020;15:e0237430. [Crossref] [PubMed]
- Yang H, Li D, Song X, et al. Joint associations of serum uric acid and ALT with NAFLD in elderly men and women: a Chinese cross-sectional study. J Transl Med 2018;16:285. [Crossref] [PubMed]
- Mato JM, Alonso C, Noureddin M, et al. Biomarkers and subtypes of deranged lipid metabolism in non-alcoholic fatty liver disease. World J Gastroenterol 2019;25:3009-20. [Crossref] [PubMed]
- Okamura T, Hashimoto Y, Hamaguchi M, et al. Ectopic fat obesity presents the greatest risk for incident type 2 diabetes: a population-based longitudinal study. Int J Obes (Lond) 2019;43:139-48. [Crossref] [PubMed]
- Chitturi S, Farrell GC, Hashimoto E, et al. Non-alcoholic fatty liver disease in the Asia-Pacific region: definitions and overview of proposed guidelines. J Gastroenterol Hepatol 2007;22:778-87. [Crossref] [PubMed]
- Chalasani N, Younossi Z, Lavine JE, et al. The diagnosis and management of nonalcoholic fatty liver disease: Practice guidance from the American Association for the Study of Liver Diseases. Hepatology 2018;67:328-57. [Crossref] [PubMed]
- Ryu S, Chang Y, Kim DI, et al. gamma-Glutamyltransferase as a predictor of chronic kidney disease in nonhypertensive and nondiabetic Korean men. Clin Chem 2007;53:71-7. [Crossref] [PubMed]
- Targher G, Byrne CD, Tilg H. NAFLD and increased risk of cardiovascular disease: clinical associations, pathophysiological mechanisms and pharmacological implications. Gut 2020;69:1691-705. [Crossref] [PubMed]
- Kanda T, Matsuoka S, Yamazaki M, et al. Apoptosis and non-alcoholic fatty liver diseases. World J Gastroenterol 2018;24:2661-72. [Crossref] [PubMed]
- Balakrishnan M, Loomba R. The Role of Noninvasive Tests for Differentiating NASH From NAFL and Diagnosing Advanced Fibrosis Among Patients With NAFLD. J Clin Gastroenterol 2020;54:107-13. [Crossref] [PubMed]
- Romero-Gómez M, Zelber-Sagi S, Trenell M. Treatment of NAFLD with diet, physical activity and exercise. J Hepatol 2017;67:829-46. [Crossref] [PubMed]
- Farzanegi P, Dana A, Ebrahimpoor Z, et al. Mechanisms of beneficial effects of exercise training on non-alcoholic fatty liver disease (NAFLD): Roles of oxidative stress and inflammation. Eur J Sport Sci 2019;19:994-1003. [Crossref] [PubMed]
- Gao Y, Zhang W, Zeng LQ, et al. Exercise and dietary intervention ameliorate high-fat diet-induced NAFLD and liver aging by inducing lipophagy. Redox Biol 2020;36:101635. [Crossref] [PubMed]
- Hashida R, Kawaguchi T, Bekki M, et al. Aerobic vs. resistance exercise in non-alcoholic fatty liver disease: A systematic review. J Hepatol 2017;66:142-52. [Crossref] [PubMed]
- Esler WP, Bence KK. Metabolic Targets in Nonalcoholic Fatty Liver Disease. Cell Mol Gastroenterol Hepatol 2019;8:247-67. [Crossref] [PubMed]
- Lu FB, Hu ED, Xu LM, et al. The relationship between obesity and the severity of non-alcoholic fatty liver disease: systematic review and meta-analysis. Expert Rev Gastroenterol Hepatol 2018;12:491-502. [Crossref] [PubMed]
- Asgharian A, Askari G, Esmailzade A, et al. The Effect of Symbiotic Supplementation on Liver Enzymes, C-reactive Protein and Ultrasound Findings in Patients with Non-alcoholic Fatty Liver Disease: A Clinical Trial. Int J Prev Med 2016;7:59. [Crossref] [PubMed]
- Noakes TD, Windt J. Evidence that supports the prescription of low-carbohydrate high-fat diets: a narrative review. Br J Sports Med 2017;51:133-9. [Crossref] [PubMed]
- Nogueira MA, Oliveira CP, Ferreira Alves VA, et al. Omega-3 polyunsaturated fatty acids in treating non-alcoholic steatohepatitis: A randomized, double-blind, placebo-controlled trial. Clin Nutr 2016;35:578-86. [Crossref] [PubMed]
- O'Neill BJ. Effect of low-carbohydrate diets on cardiometabolic risk, insulin resistance, and metabolic syndrome. Curr Opin Endocrinol Diabetes Obes 2020;27:301-7. [Crossref] [PubMed]
- Ciardullo S, Monti T, Sala I, et al. Nonalcoholic Fatty Liver Disease and Advanced Fibrosis in US Adults Across Blood Pressure Categories. Hypertension 2020;76:562-8. [Crossref] [PubMed]
- Lorenz L, Axnick J, Buschmann T, et al. Mechanosensing by β1 integrin induces angiocrine signals for liver growth and survival. Nature 2018;562:128-32. [Crossref] [PubMed]
- Sakamoto N, Hu H, Nanri A, et al. Associations of anemia and hemoglobin with hemoglobin A1c among non-diabetic workers in Japan. J Diabetes Investig 2020;11:719-25. [Crossref] [PubMed]
- Abenavoli L, Milic N, Di Renzo L, et al. Metabolic aspects of adult patients with nonalcoholic fatty liver disease. World J Gastroenterol 2016;22:7006-16. [Crossref] [PubMed]
- Chen C, Zhu Z, Mao Y, et al. HbA1c may contribute to the development of non-alcoholic fatty liver disease even at normal-range levels. Biosci Rep 2020;40:BSR20193996. [Crossref] [PubMed]
- Fan N, Peng L, Xia Z, et al. Triglycerides to high-density lipoprotein cholesterol ratio as a surrogate for nonalcoholic fatty liver disease: a cross-sectional study. Lipids Health Dis 2019;18:39. [Crossref] [PubMed]
- Bjornstad P, Eckel RH. Pathogenesis of Lipid Disorders in Insulin Resistance: a Brief Review. Curr Diab Rep 2018;18:127. [Crossref] [PubMed]
- Su Q, Kumar V, Sud N, et al. MicroRNAs in the pathogenesis and treatment of progressive liver injury in NAFLD and liver fibrosis. Adv Drug Deliv Rev 2018;129:54-63. [Crossref] [PubMed]