Inter-rater reliability in performance status assessment among health care professionals: a systematic review
Introduction
Oncologists are often required to estimate the survival of patients with incurable malignancies to recommend treatment options and hospice enrolment. In the United States, Canada, and many European countries, hospice referrals require a physician-predicted prognosis of 6 months or less (1). The correct survival prediction during end-of-life discussions can also help to avoid aggressive medical care that is associated with lower quality of life, greater medical care costs and worse caregiver bereavement outcomes (2-4).
There are multiple factors that are important in determining the prognosis of cancer patients, including tumor size, stage, grade and genetics, but none seem to play a significant role in predicting prognosis in end- of-life care (5,6). Many studies have reported that performance status (PS) is a good prognostic indicator in patients with advanced cancer (7-14). In a retrospective study published in 1985, Evans et al. showed a moderate correlation between Karnofsky performance status (KPS) and survival (15). In addition, in a study that observed greater magnitudes of decreases in the palliative performance scale (PPS) associated with worse prognosis, Chan et al. reported that changes in PS are also indicative of prognosis (16).
A literature review by Krishnan et al. showed that 10 of 13 examined models for predicting prognosis (7,9-11,17-24) incorporated PS scores, whether it was KPS, PPS, or Eastern Cooperative Oncology Group Performance Status (ECOG PS) (1). PS has also been used in the enrolment of patients into clinical trials and as a potential stratification factor in the trial analysis. However, despite the clear significance of PS in predicting prognosis, different health care professionals (HCPs) may grade PS differently, thus giving rise to the important question of which PS assessment should be relied upon to guide treatment and trial decisions. Some studies report differences (25,26) while others report similarities (27-29) among different HCPs. The purpose of this systematic review is to investigate the PS scores evaluated by different HCPs, and to assess the inter-rater variability in the assessment scores.
Methods
Search strategy
A literature search was conducted in Ovid MEDLINE and OLDMEDLINE from 1946 to Present (July 5, 2015), Embase Classic and Embase from 1947 to 2015 week 26, and Cochrane Central Register of Controlled Trials up to May 2015. Terms and phrases such as “Karnofsky Performance Status”, “Eastern Cooperative Oncology Group Performance Status”, “Palliative Performance Status”, “physician or doctor or nurse or research assistant or clinician or practitioner or specialist”, and “prognostic tool or prognostic instrument” were included in the search. The complete search strategy is displayed in Figures 1-3. Titles and abstracts were then reviewed to identify references that were relevant for full-text screening.
Selection criteria for full-text screening
Articles were selected for full-text screening if the title or abstract mentioned PS in addition to the involvement of at least two HCPs. Articles that compared PS of HCPs to patients were excluded. Duplicates of articles found in each database were also omitted.
Data extraction
The primary information of interest was whether there was a difference of PS assessment among HCPs. Secondary information of interest included the breakdown of which HCPs gave the higher or lower PS scores. Other statistical information given to assess variation in ratings, such as Cohen’s kappa coefficient, Krippendorff’s alpha coefficient, Spearman Rank Coefficient and Kendall’s correlation was extracted.
Statistical measures
Kappa values vary between −1 and +1; +1 means full agreement, 0 indicates that agreement can be explained solely by chance, and <0 is found when the observed agreement is less than expected by chance (30,31). Kappa values greater than 0.40 indicate moderate agreement, and values above 0.75 represent excellent agreement (32). The Spearman Rank Correlation ranges between −1 and +1, where −1 indicates perfect negative agreement, 0 means no correlation and +1 indicates perfect positive agreement. Values of 0–0.3 show low positive correlation, 0.3–0.6 illustrate moderate correlation and 0.6–0.10 indicate strong correlation (33).
Krippendorff’s alpha range from 0 to 1, where 0 indicates the absence of reliability and 1 indicates perfect reliability (34). An alpha of 0.80 or higher is considered a good correlation (35). The Pearson correlation coefficient ranges from −1 to +1. A value of +1 shows perfect agreement, while −1 denotes perfect disagreement; 0 indicates that the agreement can be solely explained by chance. A strong correlation coefficient is greater than 0.8, and a weak correlation is less than 0.5 (36). On the Kendall’s correlation test, a correlation of greater than 0.7 is considered very reliable (37).
Results
The literature search yielded 744 articles, with 475 from Embase, 234 from Medline, and 35 from Cochrane (Figure 4). Of those, 18 articles were identified for full-text review as specified by the inclusion criteria; 3 of the 18 articles were rejected after full-text review because of the set exclusion criteria (Table 1) (38,43,44). Of the fifteen remaining articles, eleven compared PS between HCPs of different disciplines (25-29,36,39,41,42,48,49), one between the attending and resident physician (40), two between similarly specialized physicians (45,46), and one between two unspecified-specialty physicians (47). Studies assessed inter-reliability of ECOG PS in four studies (25,27,41,45), KPS in four studies (33,40,48,49), and PPS in one study (29). Four studies (39,42,46,47) examined inter-reliability of both ECOG PS and KPS, while one study (28) compared inter-reliability of ECOG PS, KPS and PPS. One other study (26) examined the inter-reliability of an unspecified PS assessment.
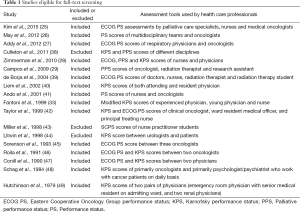
Full table
Studies examining the assessment among HCPs
Three studies (25-27) reported significant differences in the rated PS scores. Kim et al. reported a lack of agreement in ECOG PS assessments between palliative care specialists and medical oncologists (kappa =0.26), as well as between nurses and medical oncologists (kappa =0.23) (25). The nurses and palliative care specialists gave significantly higher, less healthy scores than oncologists (P<0.0001) (25). Meanwhile, palliative care nurses and specialists had moderate correlation (kappa =0.61) (25). In a separate study, May et al. found differences of PS ratings between multidisciplinary teams and oncologists (kappa =0.19) (26). Addy et al. also reported differences in ECOG PS between oncologists and respiratory physicians (Krippendorff’s alpha =0.61 and 0.63, respectively) (Table 2) (27).
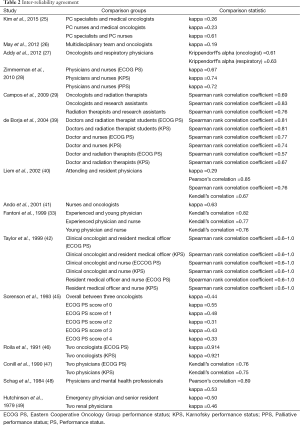
Full table
Another four studies (28,41,45,49) reported moderate inter-rater reliability. Zimmermann et al. examined inter-rater reliability for KPS, ECOG PS, and PPS between physicians and nurses; there was moderate reliability for all three tools (kappa =0.74, 0.72, and 0.67, respectively) (28). They reported a healthier assessment by physicians over nurses for the ECOG PS (P<0.0001) and PPS (P<0.0001), but not for KPS (P<0.5) (28). A study by Ando et al. showed moderate agreement between nurses and oncologists in the ECOG PS score (kappa =0.63), with oncologists reporting healthier assessments (41). In Sorenson et al.’s study, inter-rater reliability between oncologists was moderate for ECOG scores of 0, 1, 3 and overall (kappa =0.55, 0.48, 0.43 and 0.44, respectively) (45). ECOG scores of 2 and 4 were just below moderate agreement, with kappa scores of 0.31 and 0.33, respectively (45). Hutchinson et al. reported moderate agreement between an emergency physician and a senior resident’s KPS assessments (kappa =0.50) as well as KPS assessments between two renal physicians (kappa =0.46) (Table 2) (49).
Two other studies (40,48) reported a mix of low, moderate, and strong inter-rater reliability. Liem et al. reported low inter-rater agreement of KPS between the attending and resident physician using the kappa statistical tool (kappa =0.29), moderate inter-rater agreement using Kendall’s correlation (Kendall’s correlation =0.67), but strong agreement when using two other tools (Pearson’s correlation =0.85; Spearman’s rank correlation =0.76) (40). In a study by Schag et al., the reported Pearson’s correlation of KPS between physicians and mental health professionals indicated strong inter-rater reliability (Pearson’s correlation =0.89) while the Kappa statistic only showed moderate reliability (kappa =0.53). The oncologists typically reported higher ratings than mental health professionals (48).
The remaining six studies all found strong inter-rater reliability (29,33,39,42,46,47). Campos et al. examined inter-rater reliability of the PPS, and found a strong correlation between the oncologist and research assistant (Spearman’s rank correlation =0.83), the oncologist and radiation therapist (Spearman’s rank correlation =0.69), and the research assistant and radiation therapist (Spearman’s rank correlation =0.76) (29). The oncologists reported lower, less healthy scores than the radiation therapists and research assistants (29). A separate study by Fantoni et al. examined the inter-rater reliability of a modified KPS scale for HIV-infected individuals between a young physician (practicing less than 5 years with less than 2 years of experience around HIV/AIDS individuals), an experienced physician (practicing more than 10 years and with more than 5 years of experience around HIV/AIDS individuals), and nurses; there was strong agreement between the young and experienced physician (Kendall’s correlation =0.82), experienced physician and nurse (Kendall’s correlation =0.77), and young physician and nurse (Kendall’s correlation =0.76) (33). The nurses averaged healthier scores than both the young and experienced physician (33). de Borja et al. studied the correlation between doctors and nurses, as well as between radiation therapists and radiation therapist students using both ECOG PS and KPS (39). Strong correlation was reported for ECOG PS and KPS scores between doctors and nurses (Spearman rank correlation =0.77 and 0.74 respectively), and doctors and radiation therapist students (Spearman rank correlation =0.81 for both scoring systems) (39). ECOG PS and KPS scores between doctors and radiation therapists had strong and moderate correlation, respectively (Spearman rank correlation =0.67 and 0.57, respectively) (39). Strong correlation was also reported for ECOG PS and KPS scores in a study by Taylor et al. between oncologists and resident medical officers (Spearman rank correlation =0.6–1.0), oncologists and nurses (Spearman rank correlation =0.6–1.0), and nurses and the resident medical officer (Spearman rank correlation =0.6–1.0) (42). Roila et al. reported strong correlation between two oncologists in the ECOG PS (kappa =0.914) and KPS (kappa =0.921) scoring (46). Conill et al. also reported strong correlation between two physicians in the ECOG PS and KPS (Kendall correlation =0.75 and 0.76 respectively) (47).
Studies examining the assessment in patients with better vs. worse performance status (PS)
Four studies (29,40,45,46) discovered that inter-rater reliability varied with the nature of the PS; for example, inter-rater reliability would differ between patients with higher and lower PS. Campos et al. reported that doctors and radiation therapists had better agreement at lower ratings, while radiation therapists and research assistants had better agreement at higher ratings (29). In a study by Liem et al. that investigated reliability between the attending and resident physician, stronger agreement was found for KPS scores below 70 (Spearman rank correlation =0.69; Kendall’s correlation =0.61) than for scores greater or equal to 70 (Spearman rank correlation =0.48; Kendall’s correlation =0.43) (40). On the ECOG PS scale, Sorenson et al. reported that ECOG scores of 0, 1, or 2 had a higher chance of agreement (probability =0.92) than ECOG scores of 3 or 4 (probability =0.82) (45). Roila et al. examined both ECOG PS and KPS scales, and observed that the chance of agreement was higher for KPS scores between 50–100 and ECOG PS scores of 0–2 (probability =0.992 and 0.989, respectively) than for KPS scores and ECOG PS scores greater than 50 and 3, respectively (probability =0.882 and 0.909, respectively) (46).
Studies examining the assessment using difference instrument tools
In addition, five studies (28,39,42,46,47) found that the inter-rater reliability changed between assessment tools. Zimmerman et al. reported that KPS performed best for both absolute agreement and non-chance agreement, in comparison to ECOG PS and PPS (28). de Borja et al. reported a better degree of complete agreement and degree of correlation for KPS (55.6% complete agreement, and Spearman rank correlation =0.81 and 0.77 for radiation therapist students and nurses, respectively) as opposed to ECOG PS (44.4% complete agreement, and Spearman rank correlation =0.64 and 0.51 for radiation therapist students and nurses, respectively) (39). Two studies by Roila et al. and Conill et al. reported marginally better inter-rater reliability for KPS over ECOG PS (kappa =0.921 vs. 0.914 and kappa =0.76 vs. 0.75, respectively) (46,47). A study by Taylor et al. found that the level of agreement was much higher for the ECOG scale rather than the KPS scale (42).
Discussion
This is the first systematic review assessing the differences in PS ratings by different HCPs. Its findings suggest that there is disagreement in existing literature about the inter-rater reliability between different HCP assessments of PS. While some studies suggest poor agreement, and others suggest moderate agreement, there are a great number of studies that suggest good agreement.
Several factors that might explain the differences in PS scores given by different HCPs include their different medical backgrounds as well as the different assessment techniques that they may employ; differences are a result of the subjective nature of scoring on the impression of patients. For example, medical oncologists place emphasis on documenting efficacy and toxicity while palliative care specialists focus on symptom distress and daily function. Moreover, medical oncologists may have a more optimistic bias in determining PS because they may think that patients with better PS are eligible to continue chemotherapy while those with very poor PS would need to discontinue treatment. This might bias oncologists to perceive the patient as more active than they really are. This difference in perspectives may have resulted in different understandings of the grading systems. Coupled with different alterations of PS scores when they receive unexpected survival findings, HCPs from different backgrounds may assess PS very differently (25).
Despite the reported differences in inter-rater PS scores, the numerous studies highlighting correlation of PS scores suggest, in contrast, that scores are similarly rated across the HCPs. However, while these studies (28,29,33,39-42,45-49) suggest moderate or good correlation, perfect correlation has never been reported. Even among such studies, there still exist differences in the rating of PS scores among HCPs.
The difference of PS scores, whether reported as common (25-27) or uncommon (28,29,33,39-42,45-49), results in either a more optimistic or pessimistic prognosis. It is important to note that the HCPs who typically bring patients into the examination room will have a great understanding of patients’ disabilities (28). As nurses and research assistants generally interact more with patients, they often exhibit a greater understanding of the functionality and mobility of the patients, as well as any of the additional concerns patients may have (28,29). Oncologists, who may not be as aware of patients’ disabilities, often grade patients as both healthier (25,28) and unhealthier (29,33) than what nurses and research assistants report. However, there is no verification in the existing literature that nurses or research assistants rate the PS scores of patients with greater accuracy.
The variation of inter-rater reliability of PS scores also lacks a clear consensus in the literature. Of the four studies that investigated the reliability, two reported better reliability for healthier PS scores (45,46) while the other two reported better reliability for poorer PS scores (29,40). In contrast, the relative inter-rater reliability of different PS assessment tools is subject to much less dispute in the literature; four studies reported KPS as having better inter-rater reliability as opposed to ECOG PS and PPS (28,39,46,47), while only one study reported the ECOG PS as having slightly better agreement than KPS (42). The majority of studies claim that KPS has better inter-rater reliability, possibly because the KPS scale is more widely used in oncology practice (47).
The final consideration is if patients themselves should be the ones to report their PS. It would be necessary to produce educational material and check their understanding. Patient reported outcome measures are a very effective way to achieve a “gold standard” for other problems.
This systematic review was not without limitations. Two of the studies included in this systematic review were published only as abstracts, and hence it was difficult to interpret the reasoning behind the inter-rater reliability statistics. Additionally, studies used different PS assessment tools, whether it was ECOG PS, PPS, KPS or another PS assessment, leading to inconsistencies across the literature that made comparison between studies difficult.
In conclusion, the existing literature cites both good and bad inter-rater reliability of PS assessment scales. It is difficult to conclude which HCPs’ PS assessments are more accurate; however, in terms of the relative inter-rater reliability of different PS assessment tools, it has been found that KPS has better agreement over both the ECOG PS and the PPS. Future studies should examine the accuracy of KPS assessments by different HCPs in predicting prognosis. Additionally, training programs may be useful in standardizing performance-scoring assessments, to ultimately increasing inter-rater reliability.
Acknowledgements
We thank the generous support of Bratty Family Fund, Michael and Karyn Goldstein Cancer Research Fund, Joey and Mary Furfari Cancer Research Fund, Pulenzas Cancer Research Fund, Joseph and Silvana Melara Cancer Research Fund, and Ofelia Cancer Research Fund.
Footnote
Conflicts of Interest: The authors have no conflicts of interest to declare.
References
- Krishnan M, Temel JS, Wright AA, et al. Predicting life expectancy in patients with advanced incurable cancer: a review. J Support Oncol 2013;11:68-74. [Crossref] [PubMed]
- Temel JS, Greer JA, Muzikansky A, et al. Early palliative care for patients with metastatic non-small-cell lung cancer. N Engl J Med 2010;363:733-42. [Crossref] [PubMed]
- Wright AA, Zhang B, Ray A, et al. Associations between end-of-life discussions, patient mental health, medical care near death, and caregiver bereavement adjustment. JAMA 2008;300:1665-73. [Crossref] [PubMed]
- Zhang B, Wright AA, Huskamp HA, et al. Health care costs in the last week of life: associations with end-of-life conversations. Arch Intern Med 2009;169:480-8. [Crossref] [PubMed]
- Hauser CA, Stockler MR, Tattersall MH. Prognostic factors in patients with recently diagnosed incurable cancer: a systematic review. Support Care Cancer 2006;14:999-1011. [Crossref] [PubMed]
- Viganò A, Dorgan M, Buckingham J, et al. Survival prediction in terminal cancer patients: a systematic review of the medical literature. Palliat Med 2000;14:363-74. [Crossref] [PubMed]
- Chow E, Abdolell M, Panzarella T, et al. Predictive model for survival in patients with advanced cancer. J Clin Oncol 2008;26:5863-9. [Crossref] [PubMed]
- Maltoni M, Caraceni A, Brunelli C, et al. Prognostic factors in advanced cancer patients: evidence-based clinical recommendations--a study by the Steering Committee of the European Association for Palliative Care. J Clin Oncol 2005;23:6240-8. [Crossref] [PubMed]
- Lingjun Z, Jing C, Jian L, et al. Prediction of survival time in advanced cancer: a prognostic scale for Chinese patients. J Pain Symptom Manage 2009;38:578-86. [Crossref] [PubMed]
- Suh SY, Choi YS, Shim JY, et al. Construction of a new, objective prognostic score for terminally ill cancer patients: a multicenter study. Support Care Cancer 2010;18:151-7. [Crossref] [PubMed]
- Pirovano M, Maltoni M, Nanni O, et al. A new palliative prognostic score: a first step for the staging of terminally ill cancer patients. Italian Multicenter and Study Group on Palliative Care. J Pain Symptom Manage 1999;17:231-9. [Crossref] [PubMed]
- Ray-Coquard I, Ghesquière H, Bachelot T, et al. Identification of patients at risk for early death after conventional chemotherapy in solid tumours and lymphomas. Br J Cancer 2001;85:816-22. [Crossref] [PubMed]
- van der Linden YM, Dijkstra SP, Vonk EJ, et al. Dutch Bone Metastasis Group. Prediction of survival in patients with metastases in the spinal column: results based on a randomized trial of radiotherapy. Cancer 2005;103:320-8. [Crossref] [PubMed]
- Bachelot T, Ray-Coquard I, Catimel G, et al. Multivariable analysis of prognostic factors for toxicity and survival for patients enrolled in phase I clinical trials. Ann Oncol 2000;11:151-6. [Crossref] [PubMed]
- Evans C, McCarthy M. Prognostic uncertainty in terminal care: can the Karnofsky index help? Lancet 1985;1:1204-6. [Crossref] [PubMed]
- Chan EY, Wu HY, Chan YH. Revisiting the Palliative Performance Scale: Change in scores during disease trajectory predicts survival. Palliat Med 2013;27:367-74. [Crossref] [PubMed]
- Morita T, Tsunoda J, Inoue S, et al. The Palliative Prognostic Index: a scoring system for survival prediction of terminally ill cancer patients. Support Care Cancer 1999;7:128-33. [Crossref] [PubMed]
- Chiang JK, Cheng YH, Koo M, et al. A computer-assisted model for predicting probability of dying within 7 days of hospice admission in patients with terminal cancer. Jpn J Clin Oncol 2010;40:449-55. [Crossref] [PubMed]
- Hyodo I, Morita T, Adachi I, et al. Development of a predicting tool for survival of terminally ill cancer patients. Jpn J Clin Oncol 2010;40:442-8. [Crossref] [PubMed]
- Martin L, Watanabe S, Fainsinger R, et al. Prognostic factors in patients with advanced cancer: use of the patient-generated subjective global assessment in survival prediction. J Clin Oncol 2010;28:4376-83. [Crossref] [PubMed]
- Ohde S, Hayashi A, Takahasi O, et al. A 2-week prediction model for terminal cancer patients in a palliative care unit at a Japanese general hospital. Palliat Med 2011;25:170-6. [Crossref] [PubMed]
- Feliu J, Jiménez-Gordo AM, Madero R, et al. Development and validation of a prognostic nomogram for terminally ill cancer patients. J Natl Cancer Inst 2011;103:1613-20. [Crossref] [PubMed]
- Trédan O, Ray-Coquard I, Chvetzoff G, et al. Validation of prognostic scores for survival in cancer patients beyond first-line therapy. BMC Cancer 2011;11:95. [Crossref] [PubMed]
- Gwilliam B, Keeley V, Todd C, et al. Development of prognosis in palliative care study (PiPS) predictor models to improve prognostication in advanced cancer: prospective cohort study. BMJ 2011;343:d4920. [Crossref] [PubMed]
- Kim YJ, Hui D, Zhang Y, et al. Difference in performance status assessments between palliative care specialists, nurses and oncologists. J Pain Symptom Manage 2015;49:1050-8.e2.
- May CH, Lester JF, Lee S. PS score discordance and why it matters. Lung Cancer 2012;75:S18. [Crossref]
- Addy C, Sephton M, Suntharalingam J, et al. Assessment of performance status in lung cancer: Do oncologists and respiratory physicians agree? Lung Cancer 2012;75:S17-8. [Crossref]
- Zimmermann C, Burman D, Bandukwala S, et al. Nurse and physician inter-rate agreement of three performance status measures in palliative care outpatients. Support Care Cancer 2010;18:609-16. [Crossref] [PubMed]
- Campos S, Zhang L, Sinclair E, et al. The palliative performance scale: Examining its inter-rater reliability in an outpatient palliative radiation oncology clinic. Support Care Cancer 2009;17:685-90. [Crossref] [PubMed]
- Cohen J. A coefficient of agreement for nominal scales. Educ Psychol Meas 1960;20:37-46. [Crossref]
- Fleiss JL, Nee JC, Landis JR. Large sample variance of kappa in the case of different sets of rates. Psychological Bulletin 1979;86:974-7. [Crossref]
- Fleiss JL, Levin B, Paik MC, editors. Statistical methods for rates and proportions, 3rd ed. Hoboken: John Wiley & Sons, Inc., 2003.
- Fantoni M, Izzi I, Del Borgo C, et al. Inter-rater reliability of a modified Karnofsky scale of performance status for HIV-infected individuals. AIDS Patient Care STDS 1999;13:23-8. [Crossref] [PubMed]
- Krippendorff K. Computing Krippendorff’s Alpha Reliability. 2011. Available online: http://repository.upenn.edu/asc_papers/43. Accessed 20 July 2015.
- De Swert K. Calculating inter-coder reliability in media content analysis using Krippendorff’s Alpha. 2002. Available online: http://www.polcomm.org/wp-content/uploads/ICR01022012.pdf. Accessed 20 July 2015.
- Daniela S, Jäntschi L. Pearson versus Spearman, Kendall’s Tau correlation analysis on structure-activity relationships of biological active compounds. Available online: http://ljs.academicdirect.org/A09/179-200.htm. Accessed 20 July 2015.
- Nunnally JC, editor. Psychometric Theory. New York: McGraw-Hill, 1978.
- Culleton S, Dennis K, Koo K, et al. Correlation of the palliative performance scale with the karnofsky performance status in an outpatient palliative radiotherapy clinic. J Pain Manage 2011;4:427-33.
- de Borja MT, Chow E, Bovett G, et al. The correlation among patients and health care professionals in assessing functional status using the karnofsky and eastern cooperative oncology group performance status scales. Support Cancer Ther 2004;2:59-63. [Crossref] [PubMed]
- Liem BJ, Holland JM, Kang MY, et al. Karnofsky Performance Status assessment: Resident versus attending. J Cancer Educ 2002;17:138-41. [PubMed]
- Ando M, Ando Y, Hasegawa Y, et al. Prognostic value of performance status assessed by patients themselves, nurses, and oncologists in advanced non-small cell lung cancer. Br J Cancer 2001;85:1634-9. [Crossref] [PubMed]
- Taylor AE, Olver IN, Sivanthan T, et al. Observer error in grading performance status in cancer patients. Support Care Cancer 1999;7:332-5. [Crossref] [PubMed]
- Miller AM, Wilbur J, Montgomery AC, et al. Standardizing faculty evaluation of nurse practitioner students by using simulated patients. Clin Excell Nurse Pract 1998;2:102-9. [PubMed]
- Litwin MS, Lubeck DP, Henning JM, et al. Difference in urologist and patient assessments of health related quality of life in men with prostate cancer: results of the capsure database. J Urol 1998;159:1988-92. [Crossref] [PubMed]
- Sorenson JB, Klee M, Palshof T, et al. Performance status assessment in cancer patients. An inter-observer variability study. Br J Cancer 1993;67:773-5. [Crossref] [PubMed]
- Roila F, Lupattelli M, Sassi M, et al. Intra- and inter-observer variability in cancer patients’ performance status assessed according to Karnofsky and ECOG scales. Ann Oncol 1991;2:437-9. [PubMed]
- Conill C, Verger E, Salamero M. Performance status assessment in cancer patients. Cancer 1990;65:1864-6. [Crossref] [PubMed]
- Schag CC, Heinrich RL, Ganz PA. Karnofsky performance status revisited: reliability, validity and guidelines. J Clin Oncol 1984;2:187-93. [PubMed]
- Hutchinson TA, Boyd NF, Feinstein AR. Scientific problems in clinical scales, as demonstrated in the Karnofsky index of performance status. J Chronic Dis 1979;32:661-6. [Crossref] [PubMed]