Development of a nomogram for severe costal cartilage calcification: a cohort study
Introduction
Due to its abundance and robust strength, costal cartilage has been widely used in plastic and reconstructive surgery in recent years. Compared with other prosthesis materials, it is nonabsorbable, better biocompatibility, and easy to carve, especially in rhinoplasty and microtia reconstruction (1-3). In general, the sixth, seventh or eighth rib’s cartilage is most often harvested because of its length. Though it has many merits, limitations are inevitable such as calcification and the associated stiffness of cartilage, which lead to difficult carving and may have unsatisfactory results (4). For patients with severe cartilage calcification, we only choose other materials and surgical methods.
The published article showed that the calcification rate was close to 50%, with a female predominant (4,5). Moreover, it is reported that the occurrence of calcified costal cartilage was associated with age and sex (4,6). A few studies have provided information on the characteristics of calcified costal cartilage. But there is few research on the causes or factors of costal cartilage calcification. Thus, our study explored the potential factors influencing costal cartilage calcification using the nomogram methods. Especially, for patients who need to use costal cartilage for rhinoplasty or ear reconstruction, this study could guide them to prevent costal cartilage calcification in the long time. We present the following article in accordance with the TRIPOD reporting checklist (available at https://apm.amegroups.com/article/view/10.21037/apm-21-3114/rc).
Methods
Data collection
The cohort study included the patients who went in our outpatient and underwent computed tomographic (CT) scan of the chest with three-dimensional reconstructions were included in our study. The study was conducted in accordance with the Declaration of Helsinki (as revised in 2013). The study was approved by Institutional Research Ethics Committee Board of Plastic Surgery Hospital, Chinese Academy of Medical Sciences and Peking Union Medical College (No. 2021122) and informed consent was taken from all the patients. The data were collected through a questionnaire that patients consecutively completed voluntarily between June 2020 and October 2021.
A standardized questionnaire was administered by trained doctors, including sociodemographic characteristics and lifestyle behaviors. The sociodemographic characteristics included age, sex, weight, height, body mass index (BMI), nation, and residence (north or south city divided by the Qinling Mountains-Huaihe River boundary). Life behavior included smoking, drinking, bed-time, exercise, self-pressure, and the average food consumption frequencies, including milk, yogurt, carbonated drink, meat, fish, seafood, fruits. The frequencies of food consumption were recorded as no intake, mild intake (once or twice per week of food >100 g or 250 mL/cup), and high intake (more than three times per week of food >100 g or 250 mL/cup). The staying up time was divided into <11 pm, 11 pm to 1 am, and >1 am. The frequencies of exercise were recorded as no exercising, occasionally exercising (once or twice per week of the exercising time >40 min), and often exercising (more than three times per week of the exercising time >40 min). The degree of self-pressure was divided into no pressure, occasionally (one or two days) and often (more than two days). In addition, we also collected whether patients had hypertension, diabetes, thyroid or parathyroid disease, osteoarthritis, and other immune or endocrine diseases. The calcification of costal cartilage was divided into 5 degrees: 0 (0%), 1 (1–25%), 2 (26–50%), 3 (51–75%), 4 (76–100%) (4,5) (Figure 1). Level of 3 and 4 were defined as severe calcification. The calcification rate was calculated with M-view software of Marotec Medical System. The proportion of calcification was measured with this software by outlining the calcified area.
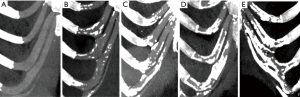
Overall, 21 factors were collected in our study, including age, sex, BMI, nation, residence, smoking, drinking, bedtime, exercise, self-pressure, the frequencies of milk, yogurt, carbonated drink, meat, fish, sea food, fruits intake, hypertension, diabetes, thyroid or parathyroid disease and osteoarthritis.
Statistical analysis
SPSS software 23.0 (IBM, Armonk, NY, USA) was used for statistical analysis. Continuous variables were given as t-tests, and categorical variables were given as χ2 tests or Fisher’s exact test. Univariate and multivariate logistic regression analyses were carried out to identify independent predictors of calcification. Nomogram analysis was carried out with R software (version 4.1.0; https://cran.r-project.org/). A P value <0.05 was considered to be statistically significant.
We used the least absolute shrinkage and selection operator (LASSO) Cox model to identify the optimal predictive features from 21 factors in order to prevent overfitting. Then the optimal predictive features, with odds ratio (OR) with 95% confidence interval (CI) and P value, were selected to build a predicting model by multivariable logistic regression analysis. A prognostic nomogram model was built based on the prediction model (7). The predictive of this model was assessed by Harrell’s concordance index (C-index) and calibration with 1,000 bootstrap samples to decrease the overfit bias (8,9). The calibration curves were drawn to evaluate the calibration of the nomogram. We also used the receiver operating characteristic (ROC) curve analysis to calculate the optimal cutoff values which were determined by maximizing the Youden index (10). Moreover, we used the decision curve analysis (DCA) to estimate the clinical usefulness of the nomogram (11). The minimum value of acceptable threshold the area under ROC (AUC) and C-index were 0.70. Finally, we adopted internal validation of enhanced bootstrap adjustment for optimism to predict the C-index of the model.
Results
A total of 882 patients completed the questionnaire with a mean age of (39.95±11.23) from June 2020 to October 2021, of which 798 (90.48%) were female. According to the degree of costal cartilage calcification, calcification (the degree of 1–4) and severe calcification (the degree of 3–4) occurred in 488 (55.32%) and 170 (19.27%) patients, respectively.
We used a LASSO Cox regression model to build a prediction model, in which 11 potential factors were selected from 21 factors (Figure 2). These factors were “Sex”, “Age”, “BMI”, “Residence”, “Milk”, “Yogurt”, “Sea food”, “Fruit”, “Bed time”, “Exercise” and “Self-pressure”. Univariate analyses of these selected characteristics were presented in Table 1. The results showed that the other factors were independent risk factors except for the “Age” and “Residence”. Then, a multivariate logistic regression analysis was performed using the above 11 factors, which were shown in Table 2. Except for “Residence”, “Milk”, “Yogurt”, “Sea food”, the P values of other factors were less than 0.05.
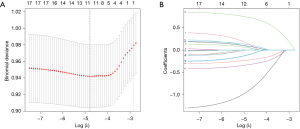
Table 1
Characteristics | Severe calcification | Non-severe calcification | P value |
---|---|---|---|
Number of patients | 170 | 712 | |
Age, years | 0.324 | ||
≥40 | 20 | 66 | |
<40 | 150 | 646 | |
The average age, years | 29.27±7.84 | 28.54±7.77 | 0.273 |
Sex | 0.003 | ||
Female | 164 | 634 | |
Male | 6 | 78 | |
BMI, kg/m2 | <0.001 | ||
<18.5 | 38 | 170 | |
18.5–23.9 | 28 | 460 | |
>23.9 | 104 | 82 | |
Residence | 0.242 | ||
North city | 60 | 286 | |
South city | 110 | 426 | |
Milk | <0.001 | ||
No intake | 20 | 416 | |
Mild intake | 42 | 200 | |
High intake | 108 | 96 | |
Yogurt | 0.002 | ||
No intake | 46 | 170 | |
Mild intake | 52 | 322 | |
High intake | 72 | 220 | |
Sea food | <0.001 | ||
No intake | 38 | 248 | |
Mild intake | 62 | 340 | |
High intake | 70 | 124 | |
Fruit | <0.001 | ||
No intake | 52 | 27 | |
Mild intake | 103 | 389 | |
High intake | 15 | 296 | |
Bed time | <0.001 | ||
<11 pm | 69 | 355 | |
11 pm–1 am | 95 | 263 | |
>1 am | 6 | 94 | |
Exercise | <0.001 | ||
No | 22 | 249 | |
Occasionally | 74 | 299 | |
Often | 74 | 164 | |
Self-pressure | <0.001 | ||
No | 83 | 452 | |
Occasionally | 77 | 173 | |
Often | 10 | 87 |
BMI, body mass index.
Table 2
Intercept and variable | β | OR (95% CI) | P value |
---|---|---|---|
Intercept | −1.79 | 0.17 (0.05–0.52) | 0.003 |
Sex | 1.57 | 0.21 (0.08–0.48) | <0.001 |
Age | 0.62 | 1.86 (1.02–3.29) | 0.036 |
BMI | 0.72 | 2.06 (1.18–3.53) | 0.009 |
Residence | 0.19 | 0.83 (0.56–1.22) | 0.348 |
Milk | 0.20 | 1.22 (0.69–2.22) | 0.505 |
Yogurt | 0.46 | 0.63 (0.36–1.10) | 0.107 |
Sea food | 0.92 | 1.62 (0.91–2.89) | 0.102 |
Fruit | 0.33 | 0.53 (0.26–1.14) | 0.010 |
Bed time | 1.13 | 2.63 (1.13–7.20) | 0.013 |
Exercise | 0.83 | 0.89 (0.60–1.34) | 0.004 |
Self-pressure | 1.09 | 2.96 (2.01–4.39) | <0.001 |
OR, odds ratio; CI, confidence interval; BMI, body mass index.
According to the results of the Cox model, nomograms of predictive of calcification were generated (Figure 3). As seen from the nomogram, “Sex” and “Self-pressure” were the most important factors due to the largest proportion. The C-index of the nomogram was 0.805, which indicated the model achieved a high accuracy of prediction. Validation of the calibration curve for the prediction of calcification exhibited good agreement in this cohort (Figure 4). We used internal validation of enhanced bootstrap adjustment for optimism, and the model had a validated C-index of 0.735, showing good model discrimination. Figure 5 presented the DCA for the nomogram. If the threshold probability of a patient is 1–77%, the predicted risk adds will more benefit than the scheme. For clinical use, the optimal cutoff value of the total nomogram scores was determined to be 360. Patients with a score of 360 or more were a high-risk subgroup. The AUC was 0.805, and the sensitivity and specificity were 0.647 and 0.894, respectively.
Discussion
At present, a growing number of plastic and reconstructive surgeries used autologous costal cartilage due to its ample volume and strength, such as microtia reconstruction, nose reconstruction, and rhinoplasty (2,3,5). Thus, the quality of costal cartilage plays a vital role in the therapeutic effect. Calcified costal cartilage aggravates the difficulty and time of carving and affects the shape and the possibility of warping after the operation. A preoperative CT scan of chest evaluation is helpful to understand the width, length, shape and degree of calcification of costal cartilage. However, the factors affecting the calcification of costal cartilage are not apparent. Thus, we explored the potential risk factors.
Our study was the first study that applied the nomogram in predicting the factors affecting the calcification of costal cartilage. Nomogram is widely used in clinical research, helping to understand prognosis to make better clinical decision-making (12). In our prediction model, 21 factors were reduced to 11 potential predictors with the LASSO method to reduce the possibility of the regression coefficients. “Sex”, “Age”, “BMI”, “Residence”, “Milk”, “Yogurt”, “Sea food”, “Fruit”, “Bed time”, “Exercise” and “Self-pressure” may be the key factors in our nomogram. We used these factors to build a relatively accurate prediction model of calcification of autogenous costal cartilage (C-index, 0.805), which was then validated in the internal validation (C-index, 0.735), which demonstrated good discrimination and can be accurately used.
Previously published articles had summarized the characteristics of calcified costal cartilage. Overall, about 41.4–59.78% of cartilage was calcified, which was consistent with our research of 55.32% (4-6); 10.9% of severe calcification was found in patients, even in teenagers (4), which was slighter lower than our study. They also found that the degree of calcification increased with age, and the rate was higher in female than in male patients (5,6,13). In our model, “Sex” and “Age” were also potential risk factors. The scholars thought that though the calcification was increased with age, the calcium volume in the costal cancellous bone decreased with age in contrast (14). Thus, the calcification was not the increase of mineral density but the decrease of bone volume (15). They considered that menopausal women were the leading group of bone loss, so the incidence of calcification was high.
As for the factors of “BMI” and “Exercise”, published articles found they were related to bone mineral density (16). Weight loss might cause the loss of bone mineral density regardless of weight regain during aerobic exercise (16,17). They thought it might be related to hormones, such as leptin, sex hormones, growth hormone, which had adverse effects on bone metabolism during exercise (16). Therefore, when the bone mineral density was decreased and did not supply calcium in time, the calcium content may be lost, and the possibility of calcification will be reduced. But obesity can cause a high prevalence of chronic diseases. The strategies made to lower bone mineral density during exercise need further study.
Dairy products provide calcium as the primary food source, increasing the total body bone mineral content (18). Moreover, the probiotics in the yogurt can also improve intestinal calcium absorption and bone metabolism. According to our model, it could be seen that people with long-term dairy products had a higher rate of severe costal cartilage calcification. Thus, excessive intake of calcium may deposit on costal cartilage. In addition, sea food can provide abundant protein and microelements. The literature has demonstrated that higher protein intakes had no disadvantages on bone loss and could increase bone volume and calcium loss (18,19). Thus, it may also have a higher rate of severe costal cartilage calcification. Besides, the vitamin C rich in fruit could also increase bone mineral density, and vitamin K as an element of osteocalcin synthesis promoted the mineralization process. So high intake of fruit may lead to severe costal cartilage calcification (18,20).
To the factors of “Bed time” and “Self-pressure”, our model showed that staying up late and higher pressure may have a higher rate of severe costal cartilage calcification. Previously published literature has not clarified the relationship between them or bone metabolism. We guess it may be due to changes in hormone levels, including sex hormones, growth hormone, and insulin-like growth factor-1, related to bone and muscle metabolism. The specific mechanism is worthy of further exploration. It was not difficult to understand the influence of “Residence” on the severe calcification through the above analysis. Although it seems incredible to us, it may be caused by various factors that are unknown at present. We are divided into north and south cities according to the boundary of the Qinling Mountains-Huaihe River. Due to their different latitudes and weather conditions, there were also differences in dietary and living habits that caused different degrees of calcification.
We developed a risk prediction model of severe costal cartilage calcification to explore the factors of calcification and preoperative and postoperative prevention simultaneously. However, our study has certain limitations. Firstly, we did not include all potential factors, mainly life factors—lack of objective indicators, such as some hormones value. Then, most patients were female and healthy, lacking data on patients with arthritis, osteoporosis, hip or spinal fractures. Moreover, external validation was also needed using another data in another center. Thus, we need to explore the mechanism of calcification further.
Conclusions
We built a novel nomogram that predicts severe calcified costal cartilage, and 11 features—“Sex”, “Age”, “BMI”, “Residence”, “Milk”, “Yogurt”, “Sea food”, “Fruit”, “Bed time”, “Exercise” and “Self-pressure” may be the key elements of calcification. It could be seen that many factors cause calcification, and the process was more complex. We want to explore the factors of calcified costal cartilage. Through the improvement of living habits, calcification of costal cartilage can be prevented preoperatively and postoperatively.
Acknowledgments
We would like to thank Fan Gu for her help in polishing our paper.
Funding: This study was funded by the Foundation of the Chinese Academy of Medical Sciences-Plastic Surgery Hospital (No. 3060120043).
Footnote
Reporting Checklist: The authors have completed the TRIPOD reporting checklist. Available at https://apm.amegroups.com/article/view/10.21037/apm-21-3114/rc
Data Sharing Statement: Available at https://apm.amegroups.com/article/view/10.21037/apm-21-3114/dss
Conflicts of Interest: All authors have completed the ICMJE uniform disclosure form (available at https://apm.amegroups.com/article/view/10.21037/apm-21-3114/coif). The authors have no conflicts of interest to declare.
Ethical Statement: The authors are accountable for all aspects of the work in ensuring that questions related to the accuracy or integrity of any part of the work are appropriately investigated and resolved. The study was conducted in accordance with the Declaration of Helsinki (as revised in 2013). The study was approved by Institutional Research Ethics Committee Board of Plastic Surgery Hospital, Chinese Academy of Medical Sciences and Peking Union Medical College (No. 2021122) and informed consent was taken from all the patients.
Open Access Statement: This is an Open Access article distributed in accordance with the Creative Commons Attribution-NonCommercial-NoDerivs 4.0 International License (CC BY-NC-ND 4.0), which permits the non-commercial replication and distribution of the article with the strict proviso that no changes or edits are made and the original work is properly cited (including links to both the formal publication through the relevant DOI and the license). See: https://creativecommons.org/licenses/by-nc-nd/4.0/.
References
- Wang H, Wu L, Xu Y, et al. Bilateral Fan-Shaped Septal Extension Struts in East Asian Augmentation Rhinoplasty. Aesthetic Plast Surg 2021;45:652-60. [Crossref] [PubMed]
- Qian J, Li Z, Liu T, et al. Auricular Reconstruction in Hemifacial Microsomia with an Expanded Two-Flap Method. Plast Reconstr Surg 2017;139:1200-9. [Crossref] [PubMed]
- Park JH, Jin HR. Use of autologous costal cartilage in Asian rhinoplasty. Plast Reconstr Surg 2012;130:1338-48. [Crossref] [PubMed]
- Sunwoo WS, Choi HG, Kim DW, et al. Characteristics of rib cartilage calcification in Asian patients. JAMA Facial Plast Surg 2014;16:102-6. [Crossref] [PubMed]
- Guo F, Yu X, Chen W, et al. Preliminary Analysis on Characteristics of Rib Cartilage Calcification in Patients With Congenital Microtia. J Craniofac Surg 2019;30:e28-32. [Crossref] [PubMed]
- Elkeles A. Sex differences in the calcification of the costal cartilages. J Am Geriatr Soc 1966;14:456-62. [Crossref] [PubMed]
- Balachandran VP, Gonen M, Smith JJ, et al. Nomograms in oncology: more than meets the eye. Lancet Oncol 2015;16:e173-80. [Crossref] [PubMed]
- Harrell FE Jr, Lee KL, Mark DB. Multivariable prognostic models: issues in developing models, evaluating assumptions and adequacy, and measuring and reducing errors. Stat Med 1996;15:361-87. [Crossref] [PubMed]
- Pencina MJ, D'Agostino RB. Overall C as a measure of discrimination in survival analysis: model specific population value and confidence interval estimation. Stat Med 2004;23:2109-23. [Crossref] [PubMed]
- Demler OV, Pencina MJ, D'Agostino RB Sr. Misuse of DeLong test to compare AUCs for nested models. Stat Med 2012;31:2577-87. [Crossref] [PubMed]
- Vickers AJ, Cronin AM, Elkin EB, et al. Extensions to decision curve analysis, a novel method for evaluating diagnostic tests, prediction models and molecular markers. BMC Med Inform Decis Mak 2008;8:53. [Crossref] [PubMed]
- Wei L, Champman S, Li X, et al. Beliefs about medicines and non-adherence in patients with stroke, diabetes mellitus and rheumatoid arthritis: a cross-sectional study in China. BMJ Open 2017;7:e017293. [Crossref] [PubMed]
- Hawellek T, Beil FT, Hischke S, et al. Costal Cartilage Calcification: Prevalence, Amount, and Structural Pattern in the General Population and Its Association with Age: A Cadaveric Study. Facial Plast Surg Aesthet Med 2021. [Epub ahead of print]. doi:
10.1089/fpsam.2021.0063 .10.1089/fpsam.2021.0063 - Zhang S, Zhen J, Li H, et al. Characteristics of Chinese Costal Cartilage and Costa Calcification Using Dual-Energy Computed Tomography Imaging. Sci Rep 2017;7:2923. [Crossref] [PubMed]
- Lau AG, Kindig MW, Kent RW. Morphology, distribution, mineral density and volume fraction of human calcified costal cartilage. Acta Biomater 2011;7:1202-9. [Crossref] [PubMed]
- Serra MC, Ryan AS. Bone Mineral Density Changes during Weight Regain following Weight Loss with and without Exercise. Nutrients 2021;13:2848. [Crossref] [PubMed]
- Han CS, Kim HK, Kim S. Effects of Adolescents' Lifestyle Habits and Body Composition on Bone Mineral Density. Int J Environ Res Public Health 2021;18:6170. [Crossref] [PubMed]
- Muñoz-Garach A, García-Fontana B, Muñoz-Torres M. Nutrients and Dietary Patterns Related to Osteoporosis. Nutrients 2020;12:1986. [Crossref] [PubMed]
- Groenendijk I, den Boeft L, van Loon LJC, et al. High Versus low Dietary Protein Intake and Bone Health in Older Adults: a Systematic Review and Meta-Analysis. Comput Struct Biotechnol J 2019;17:1101-12. [Crossref] [PubMed]
- Malmir H, Shab-Bidar S, Djafarian K. Vitamin C intake in relation to bone mineral density and risk of hip fracture and osteoporosis: a systematic review and meta-analysis of observational studies. Br J Nutr 2018;119:847-58. [Crossref] [PubMed]