Re-analysis of symptom clusters in advanced cancer patients attending a palliative outpatient radiotherapy clinic
Introduction
A diagnosis of incurable metastatic cancer may lead to significant psychological distress for patients as well as a high symptom burden, with patients experiencing an average of 11–13 concurrent symptoms (1,2). Proper palliative care is necessary to manage presenting symptoms and improve the quality of life (QOL) of patients (3). Symptoms can result from treatment, the disease itself, or other comorbidities (4,5). Multiple symptoms also have the ability to independently predict changes in functional status and overall patient outcomes (6,7). Historically, symptom management has focused on treating one symptom at a time, which has led to increased knowledge of individual symptoms, but may not reflect the entirety of the patient experience as symptoms rarely present alone (1,2,5). This rationalizes the need for the treatment of multiple symptoms at once. Identifying symptoms that often cluster together endeavors to better characterize the symptom experience of advanced cancer patients and increase the effectiveness of palliative care.
In the literature, there is currently no universal definition of a “symptom cluster” used in research or clinical practice, although it is commonly defined as two or more inter-related and concurrent symptoms that are reproducible and relatively independent of other clusters (8). Symptoms may or may not share a common etiology (8). The basis for treating symptoms in a cluster depends upon the hypothesis that single interventions may affect multiple symptoms and concurrent symptoms may share a common etiology and affect each other negatively, indicating that addressing one symptom may prevent the incidence and exacerbation of another (9). Increased awareness of the relationship between concurrent symptoms is necessary to improve overall QOL and to develop a formal recommendation for the treatment of symptom clusters in clinical oncology practice.
In 2017, our group published a preliminary analysis of symptom clusters based on a smaller sample size of 182 patients (10). The present study sought to re-analyze the previous data with additional patient information. This study will present the results of our analysis and compare findings to previous studies from our institution as well as recent literature to identify areas of heterogeneity and consensus.
Methods
A retrospective database was reviewed and analyzed. The present study was approved by the Sunnybrook Health Sciences Centre Research Ethics Board (REB PIN: 391-2016).
Patient population
Patients receiving treatment from the Rapid Response Radiotherapy Program (RRRP) at the Sunnybrook Odette Cancer Centre from February 2016 to April 2017 were eligible for inclusion in this study. The RRRP is a palliative outpatient radiotherapy clinic that delivers timely radiation treatment to advanced cancer patients with the aim of alleviating symptoms from painful bony metastases to improve QOL. Patients in this condition usually have several concurrent symptoms experienced at once, indicating the suitability of this patient population for the analysis of symptom clusters that tend to present together.
Data collection
Patients attending the RRRP were approached to complete the Edmonton Symptom Assessment System (ESAS) at their appointment before seeing the radiation oncologist. All patients were approached by a clinical research assistant to complete a paper version of the ESAS as a part of our standard of care. The patients were given the option to complete the ESAS on their own, with the help of a family member or friend present at the appointment, or with the help of the clinical research assistant. As such, some patients declined to complete the ESAS, however this represents a small minority of our patients. Some patients who did not speak English or have a family member to translate for them also declined to complete the ESAS. The ESAS symptom assessment tool asks patients to rate the severity of 8 common symptoms and overall well-being on a scale of 0–10, with 0 indicating an absence of the symptom and 10 indicating the worst possible manifestation of the symptom (11). Patients were given the option of adding and scoring an additional symptom that they were experiencing at the time of ESAS completion, with constipation reported often as an additional symptom. Patients also completed the Patient-Reported Functional Status (PRFS) questionnaire which rates activity level over the past month by choosing one of five options that describe varying degrees of functional activity (12). These assessments have been frequently utilized and validated within the cancer population (11,12). Patient demographics such as age, gender, functional status, and disease characteristics were also collected.
Statistical analyses
Patient characteristics were summarized as median, interquartile and range for age and KPS and proportions for categorical variables. A descriptive analysis was also performed to calculate the median, interquartile and range for 9 ESAS items with the addition of constipation as a frequently reported symptom. Spearman correlations among these 10 ESAS items were conducted.
Principal component analysis (PCA)
To determine interrelationships between the 9 ESAS items, a PCA with varimax rotation was performed on the symptoms reported at the patient’s first clinic visit. The PCA transforms several observed variables into a smaller number of variables called principal components (13). Most of the variability in the data is accounted for within the first principal component. An eigenvalue higher than 0.8 was used to select the number of significant principal components, each explaining more than 10% of the total variance. The highest factor loading score predicted the assignment of individual symptoms to an independent factor. Cronbach’s alpha was used to assess the internal consistency and reliability of the acquired clusters. An orthogonal varimax rotation, used extensively in the social sciences, was performed amongst three components to maximize the variance of a given column of the factor pattern matrix. A biplot graphic was used to show robust correlations between the symptoms. Arrows that were longer and closer together were interpreted as exhibiting a greater correlation between symptoms. The final communality was also reported, which refers to the percent of variance in an observed variable that is accounted for by the retained components.
Exploratory factor analysis (EFA)
The EFA is commonly used in cancer research to identify unknown groupings from a range of symptoms (8,14,15). This method identifies correlations between symptoms by finding the commonality that connects 2 or more symptoms within a common concept (8,14,15). A set of latent factors that causes covariance among a group of symptoms was predicted using factor analysis. EFA was conducted for all 9 ESAS items. The maximum likelihood method and the varimax orthogonal rotation was applied for approximately normal, multivariate data. Factors were selected by an eigenvalue greater than 0.8, indicating that approximately 10% of variance within the symptom is shared with the latent factor after controlling for the correlation between factors. Cronbach’s alpha was again used to assess the internal consistency and reliability of the calculated clusters.
Hierarchical cluster analysis
HCA is an exploratory technique that is used to discover underlying groups of individuals who have similar symptom experiences or profiles (13). This method focuses on classifying and grouping similar entities together into a cluster and subsequently separating each cluster from the other clusters found (13). 1–R2 ratio compared the correlative value within an individual cluster and the value from the next closest cluster. The occurrence of low ratios indicated well-separated clusters.
All statistical analyses were conducted using Statistical Analysis Software (SAS version 9.4 for Windows, Cary, NC) and R package (version 3.4.2). PROC FACTOR procedure was applied for PCA and EFA; PROC VARCLUS and PROC TREE graphical hierarchy procedures were applied for HCA. Constipation was not included in the cluster analyses due to the lack of patients that reported experiencing this symptom.
Results
Table 1 summarizes demographics and disease characteristics of 252 patients included in the present study. The study population consisted of patients with a median age of 71 years, most of whom were male (n=155, 61.5%) with a primary cancer site of prostate (n=60, 23.8%) or lung (n=56, 22.2%). The median and inter-quartile range of the 9 ESAS items and constipation can be found in Table 2. Spearman correlation coefficients showed constipation had a significant correlation with pain, tiredness, appetite loss, and well-being. The other 9 ESAS items had significant correlations (P<0.05) with each other.
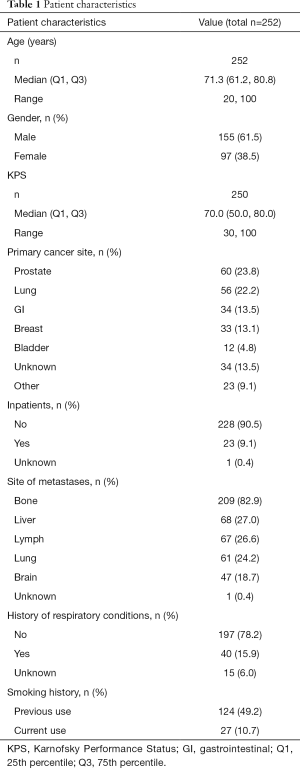
Full table
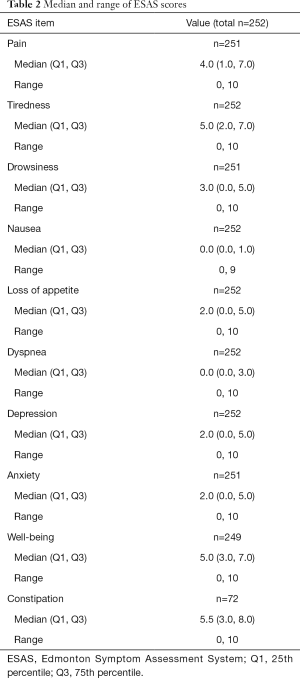
Full table
PCA
Three components with eigenvalues greater than 0.8 were derived, accounting for 65% of the total variance, each explaining more than 10%. Components 1, 2 and 3 accounted for 44%, 11% and 11% of the total variance, respectively. A summary of these results can be found in Table 3. Component 1 contained the items depression and anxiety. Component 2 included nausea, loss of appetite, and dyspnea. Component 3 included pain, well-being, tiredness, and drowsiness. The final communality determined that all components were well accounted for within the 3 clusters, with final estimates ranging from 0.48 for dyspnea to 0.80 for depression (Table 4). The internal reliabilities of the three clusters were 0.83, 0.58, and 0.76, respectively. The biplot graphics among 3 components is displayed in Figure 1.
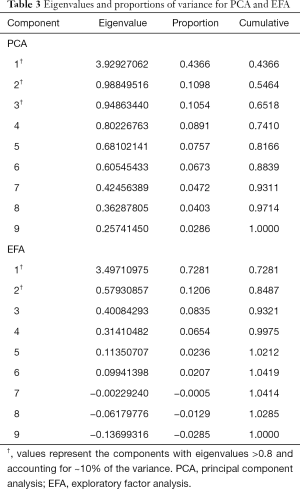
Full table
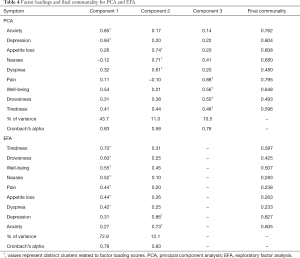
Full table
EFA
From eigenvalues and proportions of variance, two factors were retained (eigenvalue >0.8; proportion >10%), the first and second showing a variance of 72.8% and 12.1%, respectively, with a cumulative variance of 84.9% (Table 3). The first cluster included tiredness, drowsiness, well-being, pain, nausea, loss of appetite, and dyspnea. The second cluster consisted of depression and anxiety. The final communality determined final estimates ranging from 0.23 for dyspnea to 0.83 for depression (Table 4). The Cronbach’s alpha value indicating internal consistency was 0.78 for the first cluster and 0.83 for the second cluster.
HCA
The centroid cluster algorithm split the variables into two clusters explaining 60% and 48% of the total variation, respectively. The second cluster was then split, resulting in the final three clusters (Table 5). The first cluster consisted of pain, depression, anxiety and well-being. The second cluster included tiredness, drowsiness and dyspnea and the third cluster consisted of nausea and loss of appetite. Cumulatively, the three centroid components accounted for 63% of the variability in the 9 items. Additionally, the smallest correlation between the items and their respective cluster component was 0.44 for pain in cluster 1. The intercluster correlation between cluster 1 and cluster 2 was 0.59, between cluster 1 and cluster 3 was 0.44, and between cluster 2 and cluster 3 was 0.47. The cluster hierarchy is displayed in Figure 2, showing three distinct clusters.
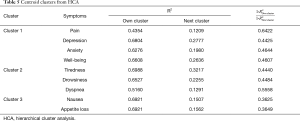
Full table
Discussion
From the PCA, EFA and HCA analyses, we derived various clusters of symptoms using the 9 ESAS items. The following items were always in the same cluster: depression and anxiety; nausea and appetite loss; well-being and pain; tiredness and drowsiness. All three statistical methods are exploratory and descriptive, examining the underlying structure of a group of symptoms (EFA), the clustering of individuals with similar symptom patterns (HCA), or reducing the original items into a fewer number of components (PCA) (13). Cluster analysis could be useful in a clinical setting to identify subgroups of individuals who have distinctive profiles of symptoms, allowing clinicians to target specific interventions to each subgroup. However, PCA does not account for the underlying structure or causality in a group (13). A review of multivariate methods in cancer symptom cluster research revealed factor and cluster analysis to produce the most conceptually accurate methods of cancer cluster analysis and did not recommend the use of the PCA, perhaps limiting the validity of our PCA results in this context (13).
From the previous analysis published by Ganesh et al. in 2017 (n=182), the clusters obtained from the PCA analysis were the same with the exception of well-being grouped with depression and anxiety in the previous analysis whereas well-being was grouped with pain, tiredness and drowsiness in the present study (10). The results from the EFA analysis were identical, although the HCA results displayed several differences: loss of appetite was grouped with nausea in the present study and was differentially grouped with depression, anxiety and well-being in the Ganesh et al. study and pain was grouped with depression, anxiety and well-being in the present study as opposed to tiredness and drowsiness in the Ganesh et al. study. Since the analyses performed were identical and the patient characteristics were largely similar, the heterogeneity of results may have been introduced through differing patient characteristics and the difference in sample size. For example, the Ganesh et al. study reported 25.3% of participants as inpatients whereas only 9.1% of participants in the present study were inpatients. A study by Chen and Tseng found that patients with stage 3 or 4 disease and lower functional status had greater associations with a “sickness cluster” consisting of pain, fatigue, sleep disturbance, loss of appetite and drowsiness than lower stages of disease and greater functional status scores (16). Although this analysis used the EFA technique, this may provide explanation for the difference in the HCA grouping of pain with tiredness and drowsiness in the Ganesh et al. study as the study population featured an increased number of inpatients who may exhibit greater stages of disease and lower functional status resulting in greater associations with a similar “sickness cluster”.
In a previous symptom cluster study performed by our group in 2007 (n=1,296), the PCA derived 3 symptom clusters (17). The depression and anxiety clusters were consistent, although our study found dyspnea was grouped with nausea and appetite loss whereas the Fan et al. study grouped nausea and appetite loss with well-being and pain (17). A re-analysis of the same patient population in 2012 using the EFA and HCA derived identical results to our study in the EFA, although the HCA resulted in the grouping of pain and well-being with depression and anxiety instead of nausea and appetite loss (18). These studies confirm the consistent presence of depression and anxiety, nausea and appetite loss, well-being and pain, and tiredness and drowsiness within the same clusters.
A gastrointestinal symptom cluster that includes nausea and vomiting related to appetite loss has been widely studied in the literature, validating our consistent finding of nausea and appetite loss presenting in the same clusters (19-21). In addition, depression and anxiety have been consistently clustered over time and across differing primary cancer sites in analyses of advanced cancer populations (18,20,22,23). Our finding of pain and well-being clustered together was documented in some studies, although it is not as common in the literature as a result of the infrequent inclusion of well-being in cluster analysis with other common symptoms (17,20,24). However, the detrimental effects of pain on overall well-being is known (25). The consistent clustering of tiredness and drowsiness has been well-studied in symptom cluster research, although the term ‘drowsiness’ is often referred to in terms of sleep disturbance or insomnia (6,20,22-24). Although slight differences may exist between these measures, the connection between lack of sleep and drowsiness is inherent. For example, a study by Miaskowski et al. (n=24) collected information from patients attending a palliative bone metastases clinic and found characteristics of sleep disturbance in patients, such as sleep efficiency and total sleep time were significantly (P<0.01) related to morning and evening fatigue (26). The cluster of pain, fatigue and drowsiness and/or disturbed sleep has also been well-documented, verifying the presentation of these symptoms together within cluster 3 of the PCA and cluster 2 of the EFA (20,26).
Due to the heterogeneity in the methodologies of symptom cluster research and the complexities in symptom presentation, there is a need to determine the most clinically relevant clusters to address. A recent study by Henry et al. examined the concept of bridge symptoms that lead to the increased presentation of other symptoms, previously explored in the literature as “sentinel symptoms” (27,28). This empirical study developed a statistical tool that takes into account a network of individual patient symptoms to further elucidate symptom clusters, characteristics of a larger patient population, and bridge symptoms (27). By identifying symptoms that lead to the formation of other symptoms, the detrimental effect on QOL could be minimized with optimal management of these basic symptoms. However, not all symptoms share a common etiology and concurrent interactions can be exceptionally complex and patient dependent. Further research regarding which symptom clusters and associated bridge symptoms are most clinically relevant to address and would provide the most benefit for individual patients is warranted.
Research regarding the treatment of symptom clusters is necessary to ensure that the discovery of symptom clusters is balanced with clinically meaningful improvements in patient outcomes. It has been suggested that treatments that affect more than one symptom at once may reduce toxicity and provide better outcomes as the improvement of one symptom can promote similar improvements in another related symptom (9). A recent review of cancer symptom cluster management has identified five studies that investigated non-pharmacological interventions for the management of elucidated symptom clusters with evidence of efficacy (29). Jarden and colleagues performed a randomized control trial (n=42) investigating the effects of a multimodal relaxation, exercise and psychoeducational intervention on 21 symptoms experienced by inpatients undergoing hematopoietic stem cell transplant, finding significantly lower symptom severity scores over time in the intervention group compared to the control group for four of the five clusters determined in the population (21). A study by Capuron et al. (n=38) also demonstrated that in patients undergoing interferon-α therapy, treatment with the antidepressant paroxetine resulted in benefit for depression, anxiety, pain and cognitive dysfunction (30). These symptoms were likely as a result of the cytokine treatment and therefore may have shared a common etiology (30). It is unknown whether this treatment would perform similarly for comparable symptoms experienced in a different clinical context.
One limitation of our study was the lack of distinct analyses for primary cancer sites. A systematic review of symptom clusters in cancer patients published in 2006 and other studies have suggested that the experience of symptom clusters may differ across various primary sites (23,31). Due to the nature of the outpatient radiotherapy clinic, often patients only had one visit with information to analyze, resulting in another significant limitation of this study. It has been suggested that the length of time that symptoms occur together is significant for the strength of relationships within the cluster (15,24). Also, the previously mentioned longitudinal symptom cluster study (n=1,296) published by our group noted that from baseline to subsequent follow-ups, regardless of the statistical method, the composition of symptom clusters changed over time (18). An analysis of symptom clusters over time would elucidate the most consistent and practically relevant clusters to address in a clinical context. Also, although the use of the ESAS to characterize patient symptoms produces minimal burden for patients, this 9-item survey does not provide a comprehensive assessment of patient symptoms. Longitudinal studies that use comprehensive symptom assessments and analyze differences in symptom clusters based on several predictors would greatly increase the knowledge of symptom clusters and its complex interactions in advanced cancer patients.
Conclusions
Our study analyzed the presentation of symptom clusters in an advanced cancer population attending an outpatient radiotherapy clinic. Using the PCA, EFA and HCA, two or three clusters were derived for each statistical method. Items that were consistently included in the same cluster were: depression and anxiety; nausea and appetite loss; well-being and pain; tiredness and drowsiness. The consistency of these symptoms within the same cluster despite varying statistical methods suggests a strong relationship that could reflect improvement of one or both symptoms in these clusters with tailored treatment. Further research is needed in symptom clusters to further examine the complex interactions between concurrent symptoms and to elucidate the most clinically meaningful clusters in which treatment would provide the most benefit for QOL in patients.
Acknowledgements
We thank the generous support of Bratty Family Fund, Michael and Karyn Goldstein Cancer Research Fund, Joey and Mary Furfari Cancer Research Fund, Pulenzas Cancer Research Fund, Joseph and Silvana Melara Cancer Research Fund, and Ofelia Cancer Research Fund. We thank Medigas for the use of the pulse oximeter device.
Footnote
Conflicts of Interest: The authors have no conflicts of interest to declare.
Ethical Statement: The present study was approved by the Sunnybrook Health Sciences Centre Research Ethics Board (REB PIN: 391-2016).
References
- Chang VT, Hwang SS, Feuerman M, et al. Symptom and quality of life survey of medical oncology patients at a veterans affairs medical center: A role for symptom assessment. Cancer 2000;88:1175-83. [Crossref] [PubMed]
- Portenoy RK, Thaler HT, Kornblith AB, et al. Symptom prevalence, characteristics and distress in a cancer population. Qual Life Res 1994;3:183-9. [Crossref] [PubMed]
- Shoemaker LK, Estfan B, Induru R, et al. Symptom management: An important part of cancer care. Cleve Clin J Med 2011;78:25-34. [Crossref] [PubMed]
- Given CW, Given B, Azzouz F, et al. Predictors of pain and fatigue in the year following diagnosis among elderly cancer patients. J Pain Symptom Manage 2001;21:456-66. [Crossref] [PubMed]
- Dodd M, Janson S, Facione N, et al. Advancing the science of symptom management. J Adv Nurs 2001;33:668-76. [Crossref] [PubMed]
- Dodd MJ, Miaskowski C, Paul SM. Symptom clusters and their effect on the functional status of patients with cancer. Oncol Nurs Forum 2001;28:465-70. [PubMed]
- Teunissen SC, de Graeff A, de Haes HC, et al. Prognostic significance of symptoms of hospitalised advanced cancer patients. Eur J Cancer 2006;42:2510-6. [Crossref] [PubMed]
- Kim H-J, McGuire DB, Tulman L, et al. Symptom clusters: concept analysis and clinical implications for cancer nursing. Cancer Nurs 2005;28:270-82. [Crossref] [PubMed]
- Williams LA. Clinical management of symptom clusters. Semin Oncol Nurs 2007;23:113-20. [Crossref] [PubMed]
- Ganesh V, Zhang L, Chan S, et al. An update in symptom clusters using the Edmonton Symptom Assessment System in a palliative radiotherapy clinic. Support Care Cancer 2017;25:3321-7. [Crossref] [PubMed]
- Chang VT, Hwang SS, Feuerman M. Validation of the Edmonton Symptom Assessment Scale. Cancer 2000;88:2164-71. [Crossref] [PubMed]
- Yogananda MN, Muthu V, Prasad KT, et al. Utility of the revised Edmonton Symptom Assessment System (ESAS-r) and the Patient-Reported Functional Status (PRFS) in lung cancer patients. Support Care Cancer 2018;26:767-75. [Crossref] [PubMed]
- Skerman HM, Yates PM, Battistutta D. Multivariate methods to identify cancer-related symptom clusters. Res Nurs Health 2009;32:345-60. [Crossref] [PubMed]
- Kim HJ, Abraham IL. Statistical approaches to modeling symptom clusters in cancer patients. Cancer Nurs 2008;31:E1-10. [Crossref] [PubMed]
- Barsevick AM, Whitmer K, Nail LM, et al. Symptom cluster research: conceptual, design, measurement, and analysis issues. J Pain Symptom Manage 2006;31:85-95. [Crossref] [PubMed]
- Chen ML, Tseng HC. Symptom clusters in cancer patients. Support Care Cancer 2006;14:825-30. [Crossref] [PubMed]
- Fan G, Hadi S, Chow E. Symptom clusters in patients with advanced-stage cancer referred for palliative radiation therapy in an outpatient setting. Support Cancer Ther 2007;4:157-62. [Crossref] [PubMed]
- Chen E, Nguyen J, Khan L, et al. Symptom clusters in patients with advanced cancer: A reanalysis comparing different statistical methods. J Pain Symptom Manage 2012;44:23-32. [Crossref] [PubMed]
- Dong ST, Butow PN, Costa DSJ, et al. Symptom clusters in patients with advanced cancer: A systematic review of observational studies. J Pain Symptom Manage 2014;48:411-50. [Crossref] [PubMed]
- Kirkova J, Aktas A, Walsh D, et al. Cancer symptom clusters: clinical and research methodology. J Palliat Med 2011;14:1149-66. [Crossref] [PubMed]
- Jarden M, Nelausen K, Hovgaard D, et al. The effect of a multimodal intervention on treatment-related symptoms in patients undergoing hematopoietic stem cell transplantation: a randomized controlled trial. J Pain Symptom Manage 2009;38:174-90. [Crossref] [PubMed]
- Olson K, Hayduk L, Cree M, et al. The changing causal foundations of cancer-related symptom clustering during the final month of palliative care: a longitudinal study. BMC Med Res Methodol 2008;8:36. [Crossref] [PubMed]
- Cheung WY, Le LW, Zimmermann C. Symptom clusters in patients with advanced cancers. Support Care Cancer 2009;17:1223-30. [Crossref] [PubMed]
- Hayduk L, Olson K, Quan H, et al. Temporal changes in the causal foundations of palliative care symptoms. Qual Life Res 2010;19:299-306. [Crossref] [PubMed]
- Schug SA, Chandrasena C. Pain management of the cancer patient. Expert Opin Pharmacother 2015;16:5-15. [Crossref] [PubMed]
- Miaskowski C, Lee KA. Pain, fatigue, and sleep disturbances in oncology outpatients receiving radiation therapy for bone metastasis: a pilot study. J Pain Symptom Manage 1999;17:320-32. [Crossref] [PubMed]
- Henry TR, Marshall SA, Avis NE, et al. Concordance networks and application to clustering cancer symptomology. PLoS One 2018;13:e0191981. [Crossref] [PubMed]
- Aktas A. Cancer symptom clusters. Curr Opin Support Palliat Care 2013;7:38-44. [Crossref] [PubMed]
- Kwekkeboom KL, Cherwin CH, Lee JW, et al. Mind-body treatments for the pain-fatigue-sleep disturbance symptom cluster in persons with cancer. J Pain Symptom Manage 2010;39:126-38. [Crossref] [PubMed]
- Capuron L, Gumnick JF, Musselman DL, et al. Neurobehavioral effects of interferon-alpha in cancer patients: phenomenology and paroxetine responsiveness of symptom dimensions. Neuropsychopharmacology 2002;26:643-52. [Crossref] [PubMed]
- Fan G, Filipczak L, Chow E. Symptom clusters in cancer patients: a review of the literature. Curr Oncol 2007;14:173-9. [Crossref] [PubMed]