Network pharmacology-based analysis of the role of traditional Chinese herbal medicines in the treatment of COVID-19
Introduction
As 2019 drew to a close, several cases of pneumonia of unknown etiology were reported in Wuhan, the capital of Hubei province in China (1). Up to now, the outbreak of COVID-19, caused by a new coronavirus, Genes related to severe acute respiratory syndromes (SARS) and Middle East respiratory syndrome (MERS) were queried in the GeneCards database (2). As of February 20, 2020, based on data released by China CDC (http://2019ncov.chinacdc.cn/nCoV/), the total number of confirmed cases was 74,680, with 2,122 of these cases resulting in death. At present, no drug exists to effectively treat or prevent COVID-19 (3). In addition, immune response to the new coronavirus differs according to the individual, leading to a variety of clinical symptoms, disease course, and treatment response to drugs and vaccines (4). Based on the National Health Commission of China Diagnosis and Treatment Protocol for COVID-19 Infection (Sixth Edition) as well as the experience of managing the virus in Hubei province (especially in Wuhan), the Hubei Province Diagnosis and Treatment Protocol for COVID-19 has been developed and released. In our current study, we analyzed data relevant to exploring the effectiveness and possible action mechanism of traditional Chinese herbal medicine (CHM) formulas for the prevention and treatment of the novel coronavirus.
Methods
Collection of active ingredients
Two CHM formulas were obtained from the Hubei Province Diagnosis and Treatment Protocol for COVID-19: Formula A: Rhizoma Atractylodis, Flos Lonicerae, Pericarpium Citri Reticulatae, Rhizoma Phragmitis, Folium Mori, and Radix Astragali seu Hedysari; and Formula B: Radix Astragali seu Hedysari, Rhizoma Atractylodis Macrocephalae, Radix Saposhnikoviae, Cyrtomium fortunei J. Sm., Flos Lonicerae, Eupatorium fortunei Turcz., and Pericarpium Citri Reticulatae.
Screening for active ingredients and target genes
Oral bioavailability (OB) reflects the rate of absorption of an orally administered drug that enters the circulation via the liver after absorption into the gastrointestinal tract. Drug-likeness (DL) refers to the structural similarity of herbal ingredients to a known drug. There is a positive correlation between these two descriptors. With the help of the TCMSP data platform (5) (http://tcmspw.com/tcmsp.php), the chemical constituents in the compounds in Formulas A and B that had OB ≥30% and DL ≥0.18 were retrieved as the active ingredients in the study. A high OB value often reflects better DL (6).
Screening of disease targets
No data on COVID-19-related genes were available in the GeneCards (https://www.genecards.org/) database at the time of study. Since the new coronavirus is highly similar to SARS-CoV and MERS-CoV (7,8), especially the bat SARS-like coronavirus (Genbank accession number MG772933), “Severe Acute Respiratory Syndromes” (SARS) and “Middle East Respiratory Syndrome” (MERS) were used in our study to search for genes that may be associated with the new coronavirus.
Screening of COVID-19-related genes acted by the active CHM ingredients
A Venn diagram was created to visualize the amount of overlap between the genes related to the active CHM ingredients and COVID-19-related genes.
Construction of protein-protein interaction (PPI) networks and screening of its core network
The protein-protein interaction core network (PPICN) refers to the correlation between compounds and disease-related protein molecules, taking into account biochemistry, signal transduction, and genetic networks. The obtained intersection genes were uploaded onto STRING11.0 (http://string-db.org/cgi/input.pl) to obtain the relationships of PPIs.
Comparison and analysis of target pathways
We analyzed the core genes screened out from the two formulas, as well as their roles in signaling pathways, to explore their functions. To achieve this, a Gene Ontology (GO)-based functional enrichment and annotation tool and the Kyoto Encyclopedia of Genes and Genomes (KEGG) were used. Data were obtained from the website (http://bioconductor.org/biocLite.R) and the results were visualized with Rstudio.
Results
Active compounds
Some of the compounds (i.e., those with the 10 highest OB values) in these two formulas were retrieved through the Traditional Chinese Medicine Systems Pharmacology Database and Analysis Platform (TCMSP). OB ≥30% and DL ≥0.18 served as the criteria (Tables 1,2).
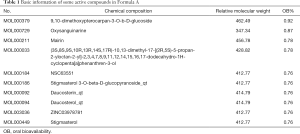
Full table
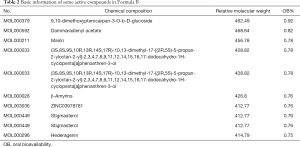
Full table
Core action genes in the CHM formulas
Aided by the TCMSP database, the genes linked to the effects of the active ingredients in these two CHM formulas were obtained. Genes related to COVID-19 were retrieved from the GeneCard database using the keywords “SARS” and “MERS”. Gene intersections were generated based on the intersection results from these two groups (Figures 1,2).
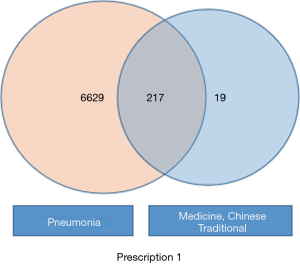
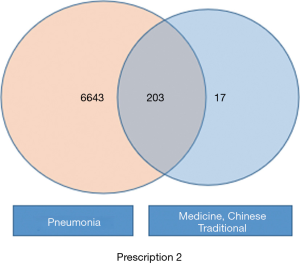
Construction and screening of PPICN between CHM formulas and COVID-19
The 217 and 203 intersected genes were analyzed using the STRING software, with a coefficient of 0.990 indicating correlation. The PPICN (PPI correlation network) of Formula A contained 217 nodes and 99 connections, with the average degree of nodes being 0.912. Formula B contained 203 nodes and 98 connections, with the average degree of nodes being 0.314 (Figures 3,4).
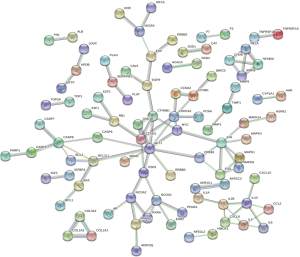
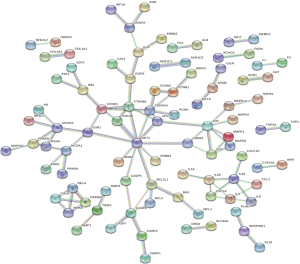
Target pathways
GO-based functional enrichment and annotation of Formula A yielded 192 GO entries (P<0.05). The 20 pathways with the highest GO enrichment are shown in Figures 5 and 6.
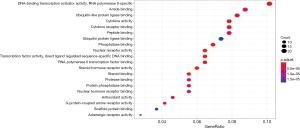
GO-based functional enrichment and annotation of Formula A yielded 192 GO entries (P<0.05). Twenty pathways with highest GO enrichment are shown in Figures 7 and 8.
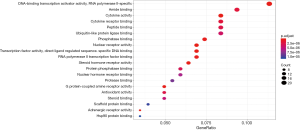
The KEGG pathways of Formulas A and B were enriched and screened to obtain the 20 most enriched signal pathways (P<0.05), including the hepatitis B pathway, Kaposi sarcoma-associated herpesvirus (KSHV) infection-related pathways, and human cytomegalovirus infection-related pathways (Figures 9-12).
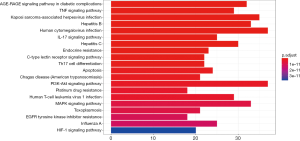
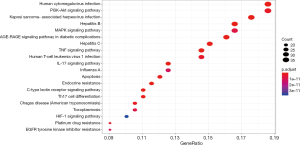
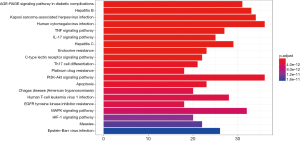
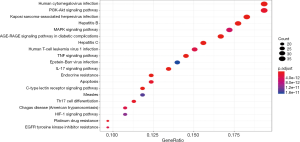
Discussion
In the absence of drugs to effectively treat COVID-19 infection, CHM offers unique antiviral benefits. For instance, astragalus polysaccharide at a non-cytotoxic concentration (30 µg/mL) significantly suppresses the expressions of two early viral proteins (Zta and Rta) in the Epstein-Barr virus lytic cycle to exert an antiviral effect (9). CHM can directly inhibit respiratory pathogens or coordinate immune system activity to prevent or alleviate respiratory infections (10). A combination of traditional Chinese and Western medicine was capable of promoting the absorption of pulmonary infiltrates in SARS patients (11). In addition to their antiviral benefits, CHMs can also be applied at the rehabilitative stage (12). Therefore, CHM may be effective for the treatment of COVID-19 (13).
By using the technology offered by network pharmacology, we analyzed the molecular mechanisms of two CHM formulas for the prevention of COVID-19. Although different herbal drugs were used in these two formulas, the results of the PPI networks, GO-based enrichment analysis, and KEGG enrichment analysis were fairly similar, which is not coincidental.
The internal regulation of the body involves a complex regulatory network rather than a single signaling pathway. Signal transduction exists among different signaling pathways and targets; therefore, the therapeutic effects of drugs are not just a result of direct targeting. Instead, they more commonly directly regulate the target while indirectly regulating other targets. Data on PPIs facilitate our understanding of the regulatory roles of targets. Akt, as the core target of CTHM formulas, plays an important role in containing coronavirus infections. In SARS patients, the phosphorylation level of the cell survival protein Akt is downregulated in cells expressing M protein. Meanwhile, the overexpression of 3-phosphoinositide-dependent protein kinase-1 (PDK1), an upstream kinase for Akt, inhibits M protein-induced apoptosis, indicating that M-protein perturbs the PDK1 and PKB/Akt cell survival signaling pathway (14). Through Akt activation, which inhibits apoptosis, CHM formulas can suppress viral replication (15), reduce apoptosis, and thereby repair damage to the body. Based on PPI networks, we can further investigate the targets via which CHM formulas affect the occurrence and development of COVID-19, map the PPI target networks, and thus pave the way for further network analysis and investigation of their underlying mechanisms.
In the GO-based enrichment analyses, the most enriched biological processes of these two CHM formulas included nuclear receptor activity, transcription factor activity, and direct ligand regulated sequence-specific DNA binding pathways. Nuclear receptors (NRs) are a superfamily of ligand-dependent transcription factors that regulate a variety of biological processes including growth and development, metabolism, and inflammation (16,17). After coronavirus infection, some of the NR-encoded proteins (e.g., nsp1) can inhibit the translation of the host without seriously affecting the expressions of viral genes (18). Fortunately, the targets of CHM formulas are enriched in NR activity, which, to a certain extent, can alleviate the damage caused by coronavirus infection. However, whether viral replication can be suppressed via the activation of coronavirus membrane fusion, which takes place through a receptor-driven ratcheting mechanism, remains unclear and further verification is required (19).
The KEGG analysis showed that a vast majority of the most enriched pathways in these two formulas were associated with viral infections. These pathways included the cytomegalovirus infection, hepatitis B virus infection, and P13K/Akt signaling pathways. Notably, the P13K/Akt pathway serves as a central regulator of many important processes that control translation, metabolism, and apoptosis. Active PI3K/Akt signals can meet the needs of replication for many viruses. When the “proviral” kinase is activated, it is also involved in the host’s response to viral infection and ultimately inhibits viral replication (20).
Limitations and prospects
CHMs have been widely recognized not only for their multiple biologically active ingredients and multiple pharmacological activities, but also their production of other biologically active or inactive metabolites when delivered in vivo. Therefore, it is difficult to determine whether the antiviral activity of a CHM is a consequence of a single and precise action mechanism or a result of a synergistic therapeutic effect. Furthermore, the medicinal properties or toxicities of CHM may be influenced by many other factors, including the methods of processing, combining, and frying involved with some medicines (21,22).
In summary, a network pharmacology method was used in our current study to investigate the effectiveness of CHM for COVID-19. Although the results were promising, more clinical trials are warranted for our findings to be confirmed.
Acknowledgments
Funding: This work was supported by 345 Talent Project of Shenjing Hospital.
Footnote
Conflicts of Interest: The authors have no conflicts of interest to declare.
Ethical Statement: The authors are accountable for all aspects of the work in ensuring that questions related to the accuracy or integrity of any part of the work are appropriately investigated and resolved.
Open Access Statement: This is an Open Access article distributed in accordance with the Creative Commons Attribution-NonCommercial-NoDerivs 4.0 International License (CC BY-NC-ND 4.0), which permits the non-commercial replication and distribution of the article with the strict proviso that no changes or edits are made and the original work is properly cited (including links to both the formal publication through the relevant DOI and the license). See: https://creativecommons.org/licenses/by-nc-nd/4.0/.
References
- Zhou P, Yang XL, Wang XG, et al. A pneumonia outbreak associated with a new coronavirus of probable bat origin. Nature 2020;579:270-3. [Crossref] [PubMed]
- Zhao S, Lin Q, Ran J, et al. Preliminary estimation of the basic reproduction number of novel coronavirus (2019-nCoV) in China, from 2019 to 2020: A data-driven analysis in the early phase of the outbreak. Int J Infect Dis 2020;92:214-7. [Crossref] [PubMed]
- Huang C, Wang Y, Li X, et al. Clinical features of patients infected with 2019 novel coronavirus in Wuhan, China. Lancet 2020;395:497-506. [Crossref] [PubMed]
- Castrucci MR. Factors affecting immune responses to the influenza vaccine. Hum Vaccin Immunother 2018;14:637-46. [Crossref] [PubMed]
- Ru J, Li P, Wang J, et al. TCMSP: a database of systems pharmacology for drug discovery from herbal medicines. J Cheminform 2014;6:13. [Crossref] [PubMed]
- Ahmed SS, Ramakrishnan V. Systems biological approach of molecular descriptors connectivity: optimal descriptors for oral bioavailability prediction. PLoS One 2012;7:e40654. [Crossref] [PubMed]
- Lu R, Zhao X, Li J, et al. Genomic characterisation and epidemiology of 2019 novel coronavirus: implications for virus origins and receptor binding. Lancet 2020;395:565-74. [Crossref] [PubMed]
- Ren LL, Wang YM, Wu ZQ, et al. Identification of a novel coronavirus causing severe pneumonia in human: a descriptive study. Chin Med J (Engl) 2020. [Epub ahead of print].
- Guo Q, Sun X, Zhang Z, et al. The effect of Astragalus polysaccharide on the Epstein-Barr virus lytic cycle. Acta Virol 2014;58:76-80. [Crossref] [PubMed]
- Wang X, Liu Z. Prevention and treatment of viral respiratory infections by traditional Chinese herbs. Chin Med J (Engl) 2014;127:1344-50. [PubMed]
- Zhang MM, Liu XM, He L. Effect of integrated traditional Chinese and Western medicine on SARS: a review of clinical evidence. World J Gastroenterol 2004;10:3500-5. [Crossref] [PubMed]
- Luo Y, Wang CZ, Hesse-Fong J, et al. Application of Chinese Medicine in Acute and Critical Medical Conditions. Am J Chin Med 2019;47:1223-35. [Crossref] [PubMed]
- Luo H, Tang QL, Shang YX, et al. Can Chinese Medicine Be Used for Prevention of Corona Virus Disease 2019 (COVID-19)? A Review of Historical Classics, Research Evidence and Current Prevention Programs. Chin J Integr Med 2020. [Epub ahead of print]. [Crossref] [PubMed]
- Tsoi H, Li L, Chen ZS, et al. The SARS-coronavirus membrane protein induces apoptosis via interfering with PDK1-PKB/Akt signalling. Biochem J 2014;464:439-47. [Crossref] [PubMed]
- Mizutani T, Fukushi S, Saijo M, et al. Importance of Akt signaling pathway for apoptosis in SARS-CoV-infected Vero E6 cells. Virology 2004;327:169-74. [Crossref] [PubMed]
- Gustafsson JA. Historical overview of nuclear receptors. J Steroid Biochem Mol Biol 2016;157:3-6. [Crossref] [PubMed]
- Kininis M, Kraus WL. A global view of transcriptional regulation by nuclear receptors: gene expression, factor localization, and DNA sequence analysis. Nucl Recept Signal 2008;6:e005. [Crossref] [PubMed]
- Nakagawa K, Lokugamage KG, Makino S. Viral and Cellular mRNA Translation in Coronavirus-Infected Cells. Adv Virus Res 2016;96:165-92. [Crossref] [PubMed]
- Walls AC, Xiong X, Park YJ, et al. Unexpected Receptor Functional Mimicry Elucidates Activation of Coronavirus Fusion. Cell 2019;176:1026-39.e15. [Crossref] [PubMed]
- Dunn EF, Connor JH. HijAkt: The PI3K/Akt pathway in virus replication and pathogenesis. Prog Mol Biol Transl Sci 2012;106:223-50. [Crossref] [PubMed]
- Izzo AA. Interactions between herbs and conventional drugs: overview of the clinical data. Med Princ Pract 2012;21:404-28. [Crossref] [PubMed]
- Milić N, Milosević N, Golocorbin Kon S, et al. Warfarin interactions with medicinal herbs. Nat Prod Commun 2014;9:1211-6. [Crossref] [PubMed]