Investigation of COVID-19-related symptoms based on factor analysis
Introduction
The novel coronavirus pneumonia, also known as coronavirus disease 2019 (COVID-19), is caused by a betacoronavirus strain, severe acute respiratory syndrome coronavirus 2 (SARS-CoV-2), which is highly contagious and highly pathogenic (1). The general population is susceptible to SARS-CoV-2. The main clinical symptoms are fever, dry cough, and fatigue, and shortness of breath and difficulty breathing gradually develop as the disease progresses. An article in Lancet reported that fever (98%), cough (76%), and myalgia or fatigue (44%) were the main symptoms of this disease (2). The Guidelines for the Diagnosis and Treatment of COVID-19 (Trial Version 7) (3) and front-line experts fighting against COVID-19 in China (4,5) have comprehensively summarized the clinical characteristics of COVID-19, including symptoms, physical signs, and imaging findings. The Guidelines for the Diagnosis and Treatment of COVID-19 pointed out that fever, dry cough, and fatigue are the major symptoms of COVID-19, and a few patients have nasal congestion, rhinorrhea, sore throat, myalgia, and diarrhea. Respiratory symptoms such as fever and cough are clinically significant in early recognition and in clinical treatment and management. However, some patients start with digestive symptoms, nervous system symptoms, or cardiovascular symptoms (Shi et al.) (6), suggesting that in clinical diagnosis and management, the identification of relevant correlations between symptoms is important to correctly identify, treat, and manage COVID-19. In this study, symptom-related factors were submitted to principal component analysis, factor analysis, and correlation analysis and comparison to search for any correlations between symptom-related factors during disease progression.
We present the following article in accordance with the STROBE reporting checklist (available at http://dx.doi.org/10.21037/apm-20-1113).
Methods
Clinical data
General information
The data used in the present study were obtained from Jingzhou Hospital of Traditional Chinese Medicine and the Second People’s Hospital of Longgang District in Shenzhen. All patients were outpatients and inpatients of these two hospitals between January 27, 2020 and February 11, 2020. A total of 60 patients who met the inclusion criteria were collected. There were 32 male patients and 28 female patients aged 20–86 years, with an average age of 43.56±2.70 years for males and 50.04±1.75 years for females. There were nine male patients and 12 female patients with abnormal lung computed tomography (CT) findings. There was no significant difference in sex or age between the normal and abnormal lung CT groups (P>0.05). This study is in line with the Nuremberg Code and the Declaration of Helsinki (as revised in 2013) and was approved by the Ethics Review Committee of Jingzhou Hospital of Traditional Chinese Medicine (No. 202003). (Informed consent was taken from all the patients.)
Inclusion criteria
According to the Guidelines for the Diagnosis and Treatment of COVID-19 (Trial Version 4), patients who met the following criteria were included: having any one epidemiological history characteristic and any two relevant clinical symptoms and having available pathological evidence (positive nucleic acid test result by real-time fluorescence reverse transcription-polymerase chain reaction detection of SARS-CoV-2 in respiratory specimens or blood specimens).
Exclusion criteria
- Patients doesn’t meet the inclusion criteria.
- Patients who were unable to accurately describe their symptoms or were unconscious were excluded.
- Patients with other viral infection of lung diseases at the same time.
Research methods
Clinical information collection method
Using the integrated clinical and research technology platform, qualified clinical researchers filled out the registration forms of COVID-19 patients and entered the corresponding information into the clinical information collection system. Based on the Guidelines for the Diagnosis and Treatment of COVID-19 (Trial Version 5), the training of personnel in the research group was strengthened to ensure the quality of the clinical research data.
Data preprocessing
Each patient was assigned a unique code. A human-computer coupled data-preprocessing system was used to standardize the symptoms to ensure the correct symptom names were used and had relatively uniform granularity. The unrelated data and the easily identifiable noise data were removed, the blank data were deleted, and some missing data and inconsistent data were re-entered based on the actual conditions of the patients.
Statistical methods
Excel 2019 was used for data entry and management, and frequency analysis was used to analyze the occurrence frequency of individual symptoms. SPSS 26.0 was used for principal component analysis and factor analysis of symptoms of COVID-19, and the correlations between factors were further analyzed. The specific steps included (I) extracting data by principal component analysis; (II) testing whether the data collected in this study were suitable for factor analysis by using the Kaiser-Meyer-Olkin (KMO) test and Bartlett’s test of sphericity; (III) determining whether the data reflected the contents of all components by determining the number of factors, the factor eigenvalues, and the cumulative percentage of variance; (IV) rotating the component matrix using varimax with Kaiser normalization to find the best analytical result and, when the rotation converged after a certain number of iterations, acquiring the symptom-related factors and their factor loadings; and (V) analyzing common factors by their clinical character.
Results
Statistics of symptom frequency
Frequency statistics on the symptoms of COVID-19 showed that among the 14 symptoms included in the statistics, fever was the most common, accounting for 83.33% of the total samples, followed by cough (68.33%), poor appetite (41.67%), and fatigue (40.00%). Detailed data are shown in Figure 1.
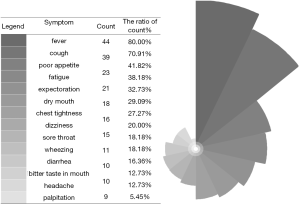
Analysis of symptoms
The KMO test value was 0.511, and Bartlett’s test of sphericity output P<0.01. Hence principal component analysis and factor analysis is valuable for further analysis. Principal component analysis yielded five factors with eigenvalues greater than 1 (Table 1, Figure 2). In Table 1, those symptoms were classified into 5 components. Each component includes eigenvalues of each symptom. Number in 5 components shows different load coefficient score in different symptoms. Their cumulative percentage of variance was 59.88% (Table 2), which shows those factors could well represent the whole data. The varimax rotation was done five times to obtain the factor loading matrix (Table 3). Finally we obtained a total of five symptom-related factors. Each symptom-related factor included a number of variables with a loading factor greater than 0.3 (Table 4, Figure 3). Through factor analysis, these five common factors were classified as respiratory-digestive-related, nervous system-related, cough-related, upper respiratory tract-related, and digestive-related factors. Those system-related symptoms could summarize COVID-19 patients symptoms characteristic and classify with disease systems.
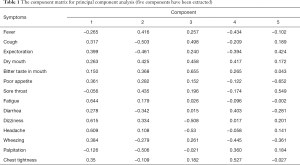
Full table
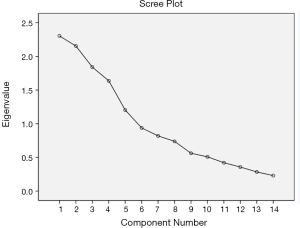
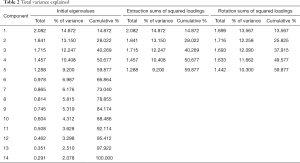
Full table
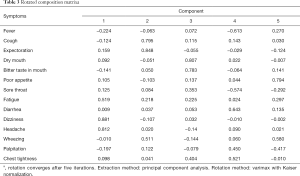
Full table
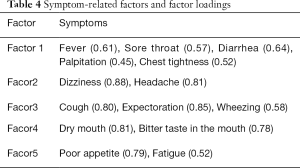
Full table
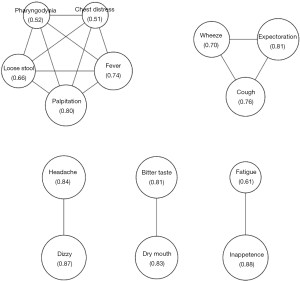
Correlation analysis of symptoms
Pairwise correlation analysis of the 14 symptoms revealed correlations between eight pairs of symptoms (P<0.05), including fever-palpitation, cough-expectoration, expectoration-wheezing, dry mouth-bitter taste in the mouth, poor appetite-fatigue, fatigue-dizziness, diarrhea-palpitation, and dizziness-headache. Interestingly, although some symptoms could be seen together in clinical practice, like fever-palpitation and cough-expectoration, some combined symptoms haven’t been reported, like example, diarrhea and palpitation.
Discussion
COVID-19 is highly infectious and highly pathogenic (7). At present, due to the unknown pathogenesis of the SARS-CoV-2 infection, there are no effective treatment or preventive measures, although vaccines are being actively developed in China and other countries. It is mainly treated with antiviral Western medicines and traditional Chinese medicine decoctions. The general population is susceptible to SARS-CoV-2, and the infection in the elderly is more likely to progress to severe conditions. The main routes of transmission are respiratory droplets and close contact. Under special circumstances, the possibility of aerosol transmission and fecal–oral transmission cannot be ruled out (1). COVID-19 spreads rapidly, posing a high risk to human health. Although its overall mortality rate is low (7), the mortality rate in severe cases is high (8). Currently, there is no specific treatment. Therefore, prevention and control of COVID-19 and its progression have become a top priority in fighting against the pandemic.
Similar to the human coronaviruses severe acute respiratory syndrome coronavirus (SARS-CoV) and Middle East respiratory syndrome coronavirus (MERS-CoV), which can cause respiratory infections, SARS-CoV-2 can cause severe respiratory symptoms (9), including fever, dry cough (10), etc. Instead of the clinical symptoms of respiratory tract infections, some patients start with digestive symptoms, such as poor appetite, fatigue, nausea, vomiting, and diarrhea. As a distinct feature of zoonoses, diarrhea also occurs in 20% to 25% of MERS-CoV- or SARS-CoV-infected individuals (11). Other symptoms include nervous system symptoms such as headache and cardiovascular symptoms such as palpitation and chest tightness (6). The above symptoms and clinical symptoms were observed in the patients included in this study. Because these symptoms are easily confused with symptoms of other chronic diseases, it is somewhat difficult to diagnose COVID-19. Therefore, the analysis and mining of correlations between the symptoms may help with the identification of COVID-19 and provide some ideas for the diagnosis and treatment of COVID-19 and the management of patients of various types.
Although the clinical characteristics of COVID-19 have been widely reported, the correlation between the symptoms has not been clarified. Therefore, to better diagnose, treat, and manage COVID-19, this study explored the correlations between different symptoms of COVID-19 by analyzing the clinical symptoms of 60 patients with COVID-19 from two medical centers, Jingzhou Hospital of Traditional Chinese Medicine and the Second People’s Hospital of Longgang District in Shenzhen, using principal component analysis, factor analysis, and correlation analysis. The results provide a basis for further investigation of its pathogenesis.
Currently, studies on the symptoms of COVID-19 generally only use descriptive and frequency statistics. However, due to the large number of symptom types and the unclear epidemiological significance of most clinical symptoms, most studies fail to obtain instructive results. Principal component analysis and factor analysis are widely used statistical methods for dimensionality reduction. The basic principle is to use a few variable factors to comprehensively reflect the primary information of the original variables to effectively solve a problem. These methods reduce dimensionality and thus the difficulty of data processing (12). In this study, various types of symptoms were subjected to dimensionality reduction, and the various symptoms were distilled into five main factors: respiratory–digestive-related, nervous system-related, cough-related, upper respiratory tract-related, and digestive-related factors. On the one hand, the characteristics of COVID-19 were mainly reflected in respiratory and digestive symptoms, which is consistent with previous studies. In addition, a correlation between these two types of symptoms was found, which provides some ideas for further study of the pathogenesis of this disease. One the other hand, this suggests that for cases of COVID-19, we need to pay attention to the influence of psychiatric disease-related factors. The method presented here can be used in future studies analyzing the symptoms of COVID-19. In this study, the KMO test value was greater than 0.5, and the results of the Bartlett’s test of sphericity rejected the null hypothesis that the correlation matrix was a unit matrix, indicating that the data collected in this study were suitable for factor analysis. Principal component analysis yielded five factors with eigenvalues greater than 1, and their cumulative percentage of variance was 59.88%. According to the principles of statistics, it is generally believed that a component with an eigenvalue greater than 1 can basically reflect the content of all components (Figure 2). Therefore, factor analysis was used to reflect the 14 symptoms of COVID-19 included in this study. Each of the five main factors had some variables with a loading factor greater than 0.3, which were used for analysis and summarizing.
Through factor analysis, this study found that the clinical symptoms of COVID-19 patients could be classified into five types: respiratory–digestive-related, nervous system-related, cough-related, upper respiratory tract-related, and digestive-related. Based on this classification, we conducted validation analysis, which provided new ideas for the comprehensive analysis of clinical symptoms of COVID-19. Therefore, this study could serve as a useful reference for studying the clinical symptoms of COVID-19 in this pandemic.
Acknowledgments
Funding: This research was supported by the research of Corona Virus Disease 2019 TCM symptoms Distribution in Jingzhou.
Footnote
Reporting Checklist: The authors have completed the STROBE reporting checklist. Available at http://dx.doi.org/10.21037/apm-20-1113
Data Sharing Statement: Available at http://dx.doi.org/10.21037/apm-20-1113
Conflicts of Interest: All authors have completed the ICMJE uniform disclosure form (available at http://dx.doi.org/10.21037/apm-20-1113). The authors have no conflicts of interest to declare.
Ethical Statement: The authors are accountable for all aspects of the work in ensuring that questions related to the accuracy or integrity of any part of the work are appropriately investigated and resolved. This study is in line with the Nuremberg Code and the Declaration of Helsinki (as revised in 2013) and was approved by the Ethics Review Committee of Jingzhou Hospital of Traditional Chinese Medicine (No. 202003). (Informed consent was taken from all the patients).
Open Access Statement: This is an Open Access article distributed in accordance with the Creative Commons Attribution-NonCommercial-NoDerivs 4.0 International License (CC BY-NC-ND 4.0), which permits the non-commercial replication and distribution of the article with the strict proviso that no changes or edits are made and the original work is properly cited (including links to both the formal publication through the relevant DOI and the license). See: https://creativecommons.org/licenses/by-nc-nd/4.0/.
References
- Yu P, Zhu J, Zhang Z, et al. A familial cluster of infection associated with the 2019 novel coronavirus indicating potential person-to-person transmission during the incubation period. J Infect Dis 2020. [Epub ahead of print]. [Crossref]
- Huang C, Wang Y, Li X, et al. Clinical features of patients infected with 2019 novel coronavirus in Wuhan, China. Lancet 2020;395:497-506. [Crossref] [PubMed]
- Bureau of Medical Administration. Guidelines for the Diagnosis and Treatment of COVID-19 (Trial Version 7). Available online: http://www.nhc.gov.cn/yzygj/s7653p/202003/46c9294a7dfe4cef80dc7f5912eb1989.shtml. 2020.
- Zhou S, Wang C, Zhang W, et al. Clinical characteristics and treatment effect of 537 cases of novel coronavirus pneumonia in Shandong Province. Journal of Shangdong University (Health Sciences) 2020:1-18.
- Yuan J, Sun Y, Zuo Y, et al. Clinical characteristics of 223 patients with COVID-19 in Chongqing. Journal of Southwest University (Natural Science Edition) 2020:1-07.
- Shi H, Han X, Fan Y, et al. Clinical characteristics and imaging findings of pneumonia caused by 2019-nCoV infection. Journal of Clinical Radiology 2020:1-08.
- Li S, Shan Y. Latest research advances on novel coronavirus pneumonia. Journal of Shangdong University (Health Sciences) 2020:1-07.
- Yang X, Yu Y, Xu J, et al. Clinical course and outcomes of critically ill patients with SARS-CoV-2 pneumonia in Wuhan, China: a single-centered, retrospective, observational study. Lancet Respir Med 2020;8:475-81. [Crossref] [PubMed]
- Lee N, Hui D, Wu A, et al. A major outbreak of severe acute respiratory syndrome in Hong Kong. N Engl J Med 2003;348:1986-94. [Crossref] [PubMed]
- Wang D, Hu B, Hu C, et al. Clinical Characteristics of 138 Hospitalized Patients With 2019 Novel Coronavirus-Infected Pneumonia in Wuhan, China. JAMA 2020;323:1061-9. [Crossref] [PubMed]
- Assiri A, Al-Tawfiq JA, Al-Rabeeah AA, et al. Epidemiological, demographic, and clinical characteristics of 47 cases of Middle East respiratory syndrome coronavirus disease from Saudi Arabia: a descriptive study. Lancet Infect Dis 2013;13:752-61. [Crossref] [PubMed]
- Xie S. Application of Principal Component Analysis and Factor Analysis Based on Mathematical Models: Shandong University of Technology; 2016.