Development and validation of predictive models for Crohn’s disease patients with prothrombotic state: a 6-year clinical analysis
Introduction
Crohn’s disease (CD) is a chronic, idiopathic inflammatory disease characterized by intestinal disruption and numerous complications (1-3). Thrombus events (TE) are often overlooked as complications, but can increase the mortality rate of CD patients (4). Bargen et al. first reported the relationship between TE and CD in 1936, and subsequent studies have established that patients with CD have a 2–3 fold higher risk of developing TE compared to healthy individuals (5-7). Although the cause of thrombosis remains unclear, previous studies have shown that several inherited and acquired risk factors for coagulation can increase the susceptibility of CD patients to a prothrombotic state (PTS). For instance, a platelet count, endothelial disruption, vitamin deficiencies, high D-dimer levels and inflammation are all potential risk contributors of PTS (7). Elevated D-dimer, a fibrin degradation product, is a predictor of thrombosis in inflammatory diseases like rheumatoid arthritis and idiopathic pulmonary fibrosis (8,9). In addition, D-dimer has also been identified as a sensitive and significant indicator for PTS in CD patients (10,11). Nevertheless, CD patients with PTS remain poorly characterized. Since PTS reflects a predisposition to thrombosis, predicting this condition can identify the CD patients at higher risk of developing the same. The aim of our study was to classify CD on the basis of D-dimer levels, and construct a prediction model for PTS using the relevant clinical and laboratory variables through machine learning. We present the following article in accordance with the STROBE reporting checklist (available at http://dx.doi.org/10.21037/apm-20-875).
Methods
Population and study design
This single-center retrospective study was conducted in accordance with the Declaration of Helsinki (as revised in 2013). The study protocol was approved by the ethics committee of the Xiangya Hospital of Central South University (No. 201911027) and individual consent for this retrospective analysis was waived. Data of CD patients admitted to the Department of Xiangya Hospital Central South University from January 2013 to December 2018 were collected. The inclusion criteria were: (I) availability of complete demographic and clinical data, (II) patients had complete laboratory indicators including D-dimer at the time of admission. Patients with incomplete medical records, and those with a history of thrombosis, cancer, organ transplantation or chronic kidney disease that can confound D-dimer levels were excluded. According to the consensus on prevention and treatment of venous thromboembolism in hospitalized patients with inflammatory bowel disease in China (11), PTS CD was defined as D-dimer (fibrinogen equivalent units, FEU) levels ≥500 µg/L, and non-PTS (nPTS) CD as D-dimer (FEU) levels <500 µg/L (Figure S1).
Data collection
Demographic data (age, gender, and body weight), history of smoking, disease phenotype (disease location and behavior), perianal lesions, CD activity index (CDAI) score, other manifestations (joint pain, fever, mouth ulcer, and abdominal mass), surgical history, and laboratory indicators including leukocyte, lymphocyte, monocyte and platelet counts, hematocrit, thrombocytocrit, mean corpuscular volume (MCV), mean platelet volume (MPV), red blood cell volume distribution width (RDW), erythrocyte sedimentation rate (ESR), C-reactive protein (CRP), prothrombin time (PT), activated partial thromboplastin time (APTT), thrombin time (TT), fibrinogen, hemoglobin, albumin and globulin were collected by chart review. All laboratory test data were collected at the time of admission, and the patient did not receive any drug or surgical intervention prior to these tests.
Definitions
Disease location and behavior were determined by endoscopic, histologic, radiographic and operative reports. Disease location was characterized by Montreal consensus as L1 (terminal ileum), L2 (colon), L3 (ileocolonic) and L4 (upper gastrointestinal). Upper gastrointestinal involvement was defined as endoscopic or histological evidence of inflammation involving the esophagus, stomach, duodenum, jejunum or proximal ileum. Disease behavior was characterized as inflammatory (non-stricturing and non-penetrating), stricturing and penetrating. Perianal lesions included fistula abscess and fissure. Patients with CDAI score ≥150 were considered to be in disease activity. Abdominal surgery was defined as CD diagnosis by surgery (excluding fistulization).
Statistical analysis
Univariate analysis
Univariate analysis was performed to identify the clinical and laboratory variables that were significantly different between the PTS and nPTS groups. Clinical variables were analyzed using the chi-square test for categorical variables and the Student’s t-test was used for continuous variables. Laboratory variables were analyzed using the Mann-Whitney U test. P values <0.05 were considered statistically significant.
Variable importance ranking
The significant factors identified by the univariate analysis were used for calculating the importance ranking. Logistic regression analysis was performed using the “Logic regression” module in the python scikit-learn package in python to obtain the adjusted odds ratio (OR) for each variable. In the random forest analysis, the “feature_importances” function in the python scikit-learn package was used to obtain the metric mean decrease GINI (MDG) in order to quantify the major factors contributing to classification accuracy. Higher MDG indicated that the degree of impurity arising from a category could be reduced farthest by one variable, which pointed to a significant predictive factor.
Model development
Multivariate models were developed with candidate variables that were significant in the univariate analysis using logistic regression and random forest analysis, and their predictive capabilities for PTS were compared. The logistic regression model is binary whereas the random forest creates multiple training sets for decision trees, wherein each tree is built based on a bootstrap sample drawn randomly from the original dataset using the CART method and the Decrease Gini Impurity as the splitting criterion (12). Furthermore, at each branching, only a given number of randomly selected features were considered as candidates. Accordingly, the data were divided into the training and test sets at the ratio of 7:3, and the training set included 520 cases. The optimum model was then constructed using the 10-fold cross validation; the training data set was divided into 10 parts, of which 9 were used for training and 1 for validation. The best trained models were then validated on the test set of 224 cases on the basis of confusion matrix, which included criteria such as true positive, false negative, false positive and true negative, accuracy, precision, sensitivity and specificity. The area under the receiver-operating characteristic curve (AUC) was calculated to determine the true positive rate (or sensitivity) vs. the false positive rate (or 1-specificity). Python (ver 3.7.5) was used for the above analysis.
Results
Baseline characteristics
The medical records of 747 CD patients were collected, of which 2 were excluded due to the history of thrombus and 1 on account of incomplete information. The mean age of onset among the remaining 744 patients was 31.5 years, and the mean body weight was 52.9 kg (13–97.5 kg). PTS was identified in 116 patients, which was nearly one-sixth of the cohort. In terms of disease location, only 1.8% of the patients had L1 lesions and 59.4% had L4 lesions, and the lesions were confined to the colon in 14.8% of the cases. In addition, more than half (57.5%) of the patients exhibited complicated disease behavior, characterized by stricturing or internal penetration. Perianal diseases were seen in 197 patients, whereas nearly one-tenth (9.4%) of the patients developed fever during the disease, and a quarter (25.3%) underwent abdominal surgery during hospitalization (Table 1).
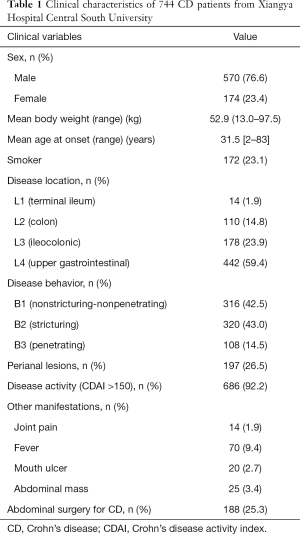
Full table
Significant factors associated with PTS CD
PTS mainly affected the older patients (P=0.002), and compared to the nPTS patients, showed frequent isolated colonic involvement (OR 1.87, P=0.012). The Montreal classification based on the disease location did not reveal any significant differences, except for the patients who had isolated colonic involvement. Furthermore, no significant differences were seen for any colonic or small intestinal diseases (Table 2). PTS was associated with higher incidences of penetrating behavior (OR 2.76, P<0.001), disease activity (OR 3.61, P=0.023), fever (OR 3.05, P<0.001), and abdominal surgery (OR 1.87, P=0.003) at the time of diagnosis (Table 2). In addition, patients with PTS had lower lymphocyte counts and hematocrit levels, and higher leukocyte counts and MCV levels compared to the nPTS patients. The inflammatory factors ESR and CRP, as well as PT and fibrinogen levels, were increased in the PTS vs. nPTS patients. Furthermore, the PTS patients had lower levels of albumin and higher levels of globulin (Table 3). As shown in Figure 1, the variables with highest MDG were CRP, albumin, leukocyte, MCV and age at onset (Figure 1A), and those with highest adjusted OR were fever, penetrating behavior, disease activity, L2 (colon) and abdominal surgery (Figure 1B).
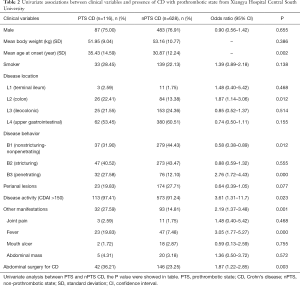
Full table
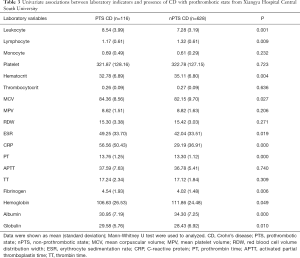
Full table
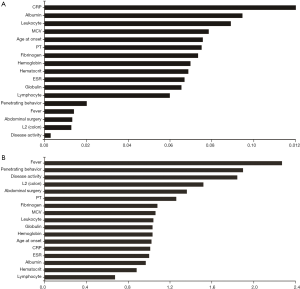
Random forest and logistic regression analysis and model development
Two predictive models were constructed based on the aforementioned variables, and the confusion matrix is shown in Table 4. The true positive, false negative, false positive and true negative values of the random forest vs. logistic regression models were 8 vs. 7, 20 vs. 21, 1 vs. 2 and 195 vs. 194 respectively. In addition, the random forest model showed that the accuracy, precision, sensitivity and specificity of the prediction model were 90.63%, 88.89%, 28.57% and 99.49% respectively. The predicted AUC of this model was 0.84 (Figure 2A). Logistic regression model showed that the accuracy, precision, sensitivity and specificity of the prediction model were 89.73%, 77.78%, 25.00% and 98.98% respectively, and the predicted AUC was 0.76 (Figure 2B).
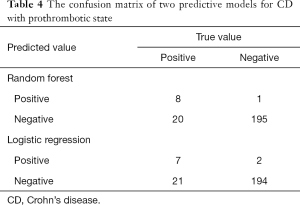
Full table
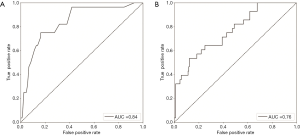
Discussion
In this study, we constructed two predictive models for PTS—defined as D-dimer (FEU) levels ≥500 µg/L (11)—in CD patients. The logistic regression model identified fever, MCV, CRP levels and hematocrit as the significant predictive variable for PTS, of which fever was statistically most prominent in terms of both P value and adjusted OR (Table 5). Fever is a common symptom associated with thrombosis (13), while CRP is an indicator of disease activity, remission and recurrence in CD patients, and a risk factor of venous thromboembolism as well (14,15). The aberrant MCV levels in CD patients can be attributed to the increased blood stasis due to inflammation, which alters vascular flow by increasing viscosity and deforming microcytic red blood cells (16-18). CRP levels and MCV were also identified by the random forest model.
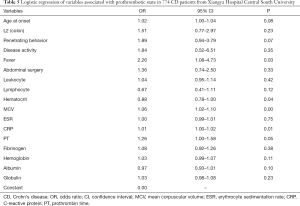
Full table
Both models showed good predictive performance for PTS in CD. The respective accuracy and AUC of the random forest vs. logistic regression models were 90.63% vs. 89.73% and 0.84 vs. 0.76 for AUC, indicating better performance of the former. This is likely due to the robust performance and strong generalization power of the random forest model, which can analyze large datasets, high-order interactions and multicollinearity, and make a prediction using bootstrap aggregation. In our study, the random forest model consisted of 50 decision trees, which increased the reliability of the results (19,20).
Our study not only confirmed the thrombus-related risk factors reported in previous studies using a large study population and machine learning, but also established their correlation to PTS in CD patients. Furthermore, we used importance ranking to explore the interaction between those factors. Finally, two predictive models were constructed and validated, which increased the reliability of the PTS-related factors. However, there are some limitations in our study that ought to be addressed. Although several variables were analyzed, the retrospective design of the study may have introduced some bias. Furthermore, both models need to be validated using external data in order to improve their adaptability. Finally, the prospective clinical use of this model can only be verified by a randomized controlled trial.
In conclusion, two predictive models based on clinical and laboratory variables were constructed to screen for CD patients with PTS. Our findings provide novel insights into PTS in CD patients, and can enable early diagnosis of coagulation as well as individual therapeutic approaches.
Acknowledgments
Funding: This work was supported by the National Natural Science Foundation of China under Grant (81770584).
Footnote
Reporting Checklist: The authors have completed the STROBE reporting checklist. Available at http://dx.doi.org/10.21037/apm-20-875
Data Sharing Statement: Available at http://dx.doi.org/10.21037/apm-20-875
Peer Review File: Available at http://dx.doi.org/10.21037/apm-20-875
Conflicts of Interest: All authors have completed the ICMJE uniform disclosure form (available at http://dx.doi.org/10.21037/apm-20-875). The authors have no conflicts of interest to declare.
Ethical Statement: The authors are accountable for all aspects of the work in ensuring that questions related to the accuracy or integrity of any part of the work are appropriately investigated and resolved. This single-center retrospective study was conducted in accordance with the Declaration of Helsinki (as revised in 2013). The study protocol was approved by the ethics committee of the Xiangya Hospital of Central South University (No. 201911027) and individual consent for this retrospective analysis was waived.
Open Access Statement: This is an Open Access article distributed in accordance with the Creative Commons Attribution-NonCommercial-NoDerivs 4.0 International License (CC BY-NC-ND 4.0), which permits the non-commercial replication and distribution of the article with the strict proviso that no changes or edits are made and the original work is properly cited (including links to both the formal publication through the relevant DOI and the license). See: https://creativecommons.org/licenses/by-nc-nd/4.0/.
References
- Gomollón F, Dignass A, Annese V, et al. 3rd European Evidence-based Consensus on the Diagnosis and Management of Crohn's Disease 2016: Part 1: Diagnosis and Medical Management. J Crohns Colitis 2017;11:3-25. [Crossref] [PubMed]
- Torres J, Mehandru S, Colombel J, et al. Crohn's disease. Lancet 2017;389:1741-55. [Crossref] [PubMed]
- Bernstein CN, Fried M, Krabshuis JH, et al. World Gastroenterology Organization Practice Guidelines for the diagnosis and management of IBD in 2010. Inflamm Bowel Dis 2010;16:112-24. [Crossref] [PubMed]
- Murthy SK, Nguyen GC. Venous Thromboembolism in Inflammatory Bowel Disease: An Epidemiological Review. Am J Gastroenterol 2011;106:713-8. [Crossref] [PubMed]
- Zezos P. Inflammatory bowel disease and thromboembolism. World J Gastroenterol 2014;20:13863-78. [Crossref] [PubMed]
- Yuhara H, Steinmaus C, Corley D, et al. Meta-analysis: the risk of venous thromboembolism in patients with inflammatory bowel disease. Aliment Pharmacol Ther 2013;37:953-62. [Crossref] [PubMed]
- Magro F. Venous thrombosis and prothrombotic factors in inflammatory bowel disease. World J Gastroenterol 2014;20:4857-72. [Crossref] [PubMed]
- Navaratnam V, Fogarty AW, McKeever T, et al. Presence of a prothrombotic state in people with idiopathic pulmonary fibrosis: a population-based case–control study. Thorax 2014;69:207-15. [Crossref] [PubMed]
- Bisoendial RJ, Levi M, Tak PP, et al. The Prothrombotic State in Rheumatoid Arthritis: An Additive Risk Factor for Adverse Cardiovascular Events. Semin Thromb Hemost 2010;36:452-7. [Crossref] [PubMed]
- Wang W, Duan K, Ma M, et al. Tranexamic Acid Decreases Visible and Hidden Blood Loss Without Affecting Prethrombotic State Molecular Markers in Transforaminal Thoracic Interbody Fusion for Treatment of Thoracolumbar Fracture-Dislocation. Spine (Phila Pa 1976) 2018;43:E734-E739. [Crossref] [PubMed]
- Chinese Society of Gastroenterology, IBD Working Group. Chinese expert consensus on the prevention and cure of venous thromboembolism of inflammatory bowel disease patients in hospital. Chinese Journal of Inflammatory Bowel Diseases 2018;2:75-82.
- Shkurin A, Vellido A. Using random forests for assistance in the curation of G-protein coupled receptor databases. Biomed Eng Online 2017;16:75. [Crossref] [PubMed]
- Fabio F, Lykoudis P, Gordon P. Thromboembolism in Inflammatory Bowel Disease: An Insidious Association Requiring a High Degree of Vigilance. Semin Thromb Hemost 2011;37:220-5. [Crossref] [PubMed]
- Reinisch W, Wang Y, Oddens BJ, et al. C-reactive protein, an indicator for maintained response or remission to infliximab in patients with Crohn's disease: a post-hoc analysis from ACCENT I. Aliment Pharmacol Ther 2012;35:568-76. [Crossref] [PubMed]
- Olson NC, Cushman M, Lutsey PL, et al. Inflammation markers and incident venous thromboembolism: the REasons for Geographic And Racial Differences in Stroke (REGARDS) cohort. J Thromb Haemost 2014;12:1993-2001. [Crossref] [PubMed]
- Baskurt OK, Meiselman HJ. Blood rheology and hemodynamics. Semin Thromb Hemost 2003;29:435-50. [Crossref] [PubMed]
- Pandey SK, Pandey S, Mishra RM, et al. Prevalence of Factor V Leiden-G1691A and MTHFR-C677T Thrombosis Gene Modifier in Iron Deficiency Anemia: A Pathophysiological Effect in Indian Isolates. Indian J Clin Biochem 2017;32:103-5. [Crossref] [PubMed]
- Conway DSG, Buggins P, Hughes E, et al. Relation of interleukin-6, C-reactive protein, and the prothrombotic state to transesophageal echocardiographic findings in atrial fibrillation. Am J Cardiol 2004;93:1368-73. [Crossref] [PubMed]
- Liaw A, Wiener M. Classification and Regression by RandomForest. R News 2002;2:18-22.
- Cutler DR, Edwards TC, Beard KH, et al. Random Forests for Classification in Ecology. Ecology 2007;88:2783-92. [Crossref] [PubMed]