Derivation and validation of a simple nomogram prediction model for all-cause mortality among middle-aged and elderly general population
Introduction
Multiple studies have shown that living environment (1), economic status (2,3) and anthropometric indicators (4) were all closely related to mortality. Studies have also demonstrated that obesity (5), elevated blood pressure (6), and diabetes (7) were significantly associated with the risk of all-cause mortality. In addition, meta-analyses suggested that some simple demographic information, such as marital status and education levels were both important factors to predict all-cause mortality (8,9). Meanwhile, many high-quality studies and meta-analyses also indicated that smoking (10) and alcohol consumption (11) were strong risk factors for all-cause mortality.
The aforementioned variables have received attention for being the key components of risk stratification, because these variables were easily measurable, accessible and can be routinely collected. At the moment, there are limited clinical models that use those simple and accessible indicators to predict all-cause mortality among general population.
We present the following article in accordance with the TRIPOD reporting checklist (available at http://dx.doi.org/10.21037/apm-20-580).
Methods
Study design and study population
The study conforms to the transparent reporting of a multivariable prediction model for individual prognosis or diagnosis (TRIPOD): the TRIPOD statement. All participants were recruited from the 1999–2006 National Health and Nutrition Examination Surveys (NHANES). The NHANES is a nationally representative survey which was designed by the National Center for Health Statistics, Centers for Disease Control and Prevention (NCHS, CDC) to assess the health and nutritional status of non-institutionalized, community-dwelling adults and children in the United States (12). There were 41,474 subjects included in the 1999–2006 NHANES. In the present study, we excluded people aged <40 years (n=28,448). Our final analytical cohort included 13,026 adults as shown in Figure 1. The survey protocol was approved by the Institutional Review Board of the Centers for Disease Control and Prevention (Protocol #98-12, Protocol #2005-06, Continuation of Protocol #2005-06). The study conformed to the provisions of the Declaration of Helsinki (as revised in 2013). All participants gave written informed consent.
Derivation and validation cohorts
The derivation and Validation models were developed using data from the NHANES cohort. Participants come from the 1999–2002 surveys were designed as the derivation cohort, while participants from 2003–2006 surveys were used as the validation cohort. Finally, a total of 6,671 and 6,355 subjects were included in the derivation and validation cohort, respectively.
Candidate predictor variables
Demographic characteristics of participants were acquired via self-reported questionnaire, including age, gender (female and male), race (non-Hispanic White, Mexican American, Black, other Hispanic, and other), smoking status (current, former, never), alcohol intake in gram (assessed by a 1-day food record), marriage status (married, single, never married), income, education(less than high school, high school diploma and more than high school) and previous diseases (such as diabetes, hypertension). In addition, anthropometric and blood pressure (BP) measurement was performed by standardized procedures. Weight was determined with an electronic digital scale (kilograms), and height (meters) was determined by a stadiometer after deep inhalation. Body Mass Index (BMI) was calculated as weight (kilograms) divided by height squared (meters squared). Poverty to income ratio (PIR) was calculated by dividing family income by the poverty guidelines, specific to family size, as well as the appropriate year and state. Diabetes was defined as fasting blood glucose ≥126 mg/dL or self-report) (13) and hypertension was defined as BP ≥140/90 mmHg or self-report) (14).
Mortality
All-cause mortality data were abstracted from the 1999–2006 NHANES public-use linked mortality files, which captured the vital status and cause of death of survey participants from survey participation (1999–2006) to 31 December 2015. We examined all-cause mortality by using the International Classification of Diseases-10th Revision codes. Detailed mortality variables for participants can be found on the website (https://www.cdc.gov/nchs/data-linkage/mortality-public.htm).
Statistical analysis
All the continuous variables were presented as mean ± standard deviation, and categorical variables were presented in frequency or as a percentage. The Kruskal-Wallis Rank Sum Test, Fisher test, Student’s t-test and chi-square tests were performed to detect subgroup differences by baseline characteristics. We used univariate and multivariate Cox proportional hazards regression to estimate the risks of all-cause mortality. Hazard ratios (HRs) and 95% CIs were presented as effect estimates. Subgroup analysis was performed according to age (<50, 50–60, 60–70, >70 years), PIR (<1, 1–3, >3) and BMI (<25, ≥25 kg/m2). To develop a clinical prediction model, the candidate variables were: age, gender (0= male, 1= female), BMI, SBP, smoking (0= non-smoker, 1= ex-smoker, 2= current smoker), alcohol intake, marriage status (1= married, 2= single, 3= never married), education (0= less than high school, 1= high school diploma, 2= more than high school), PIR, diabetes (0= no, 1= yes) and hypertension (0= no, 1= yes). This study used three methods to establish a predictive model and to verify internal validation. First, a full model including age, gender, BMI, SBP, smoking, alcohol intake, diabetes, hypertension, marriage, education and PIR were fitted in the derivation model. Second, we established a simplified model by stepwise regression analysis of screening variables. Step Akaike information criterion was used to screen variables into the simplified model. Prediction nomogram was built in the derivation cohort based on multivariate regression analysis. Third, for further checking, a least absolute shrinkage and selection operator (LASSO) binomial regression model was applied to determine the ideal coefficient for each variables and estimate the likelihood deviance. For assessing the discriminative performance of the nomogram, the area under the curve (AUC) in receiver operating characteristic (ROC) analysis was measured to evaluate predictive accuracy. The performance of the model in terms of establishing, discrimination and calibration were evaluated in the validation cohort by using the same methods described above. All analysis was conducted by SPSS 22.0 (SPSS Inc., Chicago, IL, USA) and the R software version 3.3.2 (R Foundation for Statistical Computing, Vienna, Austria). Two tailed P<0.05 indicated statistical significance.
Results
Baseline characteristics of the cohorts
The final study sample included 13,026 participants (6,414 men, mean age was 61.59±13.80 years). There were 6,671 and 6,355 participants in the derivation cohort and validation cohort, respectively. During the mean follow-up period of 129.23±9.62 months, 4,321 participants have died. As shown in Table 1, there were significant differences in SBP, DBP, alcohol use, PIR, marriage, education, race and hypertension between the derivation cohort and validation cohort.
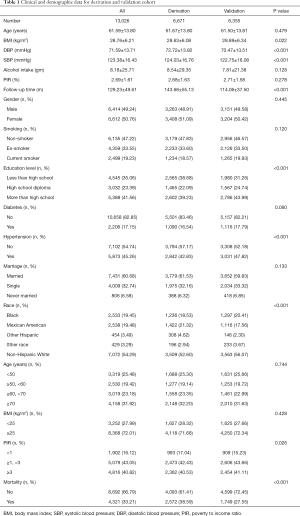
Full table
The association of candidate predictor variables with all-cause mortality
As shown in Table 2, univariate Cox proportional hazards regression demonstrated that female (HR: 0.79, 95% CI: 0.75–0.84, P<0.001), SBP (HR: 1.01, 95% CI: 1.01–1.01, P<0.001), DBP (HR: 0.97, 95% CI: 0.97–0.98, P<0.001), alcohol intake (HR: 1.00, 95% CI: 1.00–1.00, P<0.001), diabetes (HR: 1.83, 95% CI: 1.71–1.97, P<0.001) and hypertension (HR: 1.73, 95% CI: 1.63–1.84, P<0.001) were associated with all-cause mortality. In addition, current smoker, people who had less than high school education, with age ≥70 years and PIR <1% had the highest risk for all-cause mortality. Further multivariate Cox proportional hazards regression revealed that current smoker (HR: 1.89, 95% CI: 1.63–2.19, P<0.001), people who never married (HR: 1.57, 95% CI: 1.25–1.97, P<0.001), people aged ≥70 years (HR: 9.95, 95% CI: 8.18–12.11, P<0.001) have a higher risk for all-cause mortality. However, BMI, PIR, DBP and education were inversely associated with all-cause mortality (Table 2).

Full table
Prediction of all-cause mortality in the derivation and validation cohort
There were 2,572 participants (38.59%) and 1,749 (27.55%) died in derivation and validation cohort, respectively. First, eleven independent predictors for all-cause mortality were enrolled in this full model. Each of these variables was assigned a score on the point scale. After calculating the total score and locating it on the total point scale, we drew a vertical line down to get the predicted probability of all-cause mortality. The higher score of total points reflected a higher probability of all-cause mortality (Figure 2A). Second, in order to optimize the model, step Akaike information criterion was used to screen the optimal variables into the simplified model. As shown in Figure 2B, a simple nomogram model, including age, gender, smoking, alcohol intake, diabetes, hypertension, marriage status, education and PIR could predict all-cause mortality among middle-aged and elderly general population. The same analytical method was used for the full and simplified model for making the nomogram, ROC curve and calibration plots. The area under the ROC curve of the full and simplified model was both 0.842 (Figure 3A). The analysis method of full model, optimized and simplified model, LAASO model in the validation cohort were all similar to derivation cohort. In order to validate the training optimized and simplified model, the C statistic of this models were both 0.849 (Figure 3B), and nearly the same as the full model in the derivation cohort. The decision curve analysis for the nomogram was demonstrated in Figure 4, using the nomogram in the present study to predict all-cause mortality successfully. The decision curve analysis for the nomogram of derivation (Figure 4A) and validation (Figure 4B) cohort indicated that these models could predict all-cause mortality successfully with good sensitivity and specificity (Table 3). What is more, as shown in Figure 5A, the optimism-corrected AUC values at different follow-up time, the model had all AUC values more than 0.80. It demonstrated that this model was very stable, and the predictive value of the model has no significant relationship with the follow-up time. Similarly, the model had all AUC values greater than 0.80 at different follow-up time, suggesting no significant change in area under the ROC curve with the change of follow-up time for predicting all-cause mortality in the validation cohort (Figure 5B).
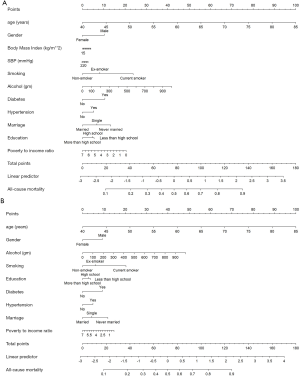
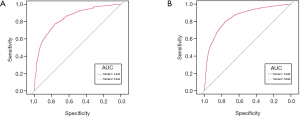
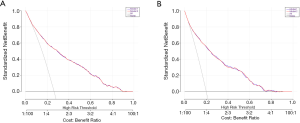
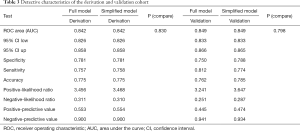
Full table
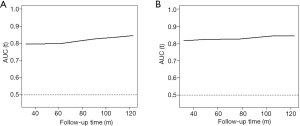
In addition, to confirm the predictors of the simplified model, LASSO binomial regression was also performed with λ of 0.0143 and nine predictors were selected into this model. Tuning parameter (lambda) selection in the LASSO model used 10-fold cross-validation. As shown in Figure 6A,B, a cross-validated error plot of the LASSO regression model and a coefficient profile plot were produced, respectively. To consolidate and verify the stability of the simplified model, a LASSO cox regression model was performed by using the same variables of the simplified model, and with λ of 0.0108. The path of the coefficients included in this model, with varying log-transformed lambda values, 9 potential predictors, including age, gender, smoking, alcohol intake, diabetes, hypertension, marriage, education and PIR were shown in Figure 6C,D.
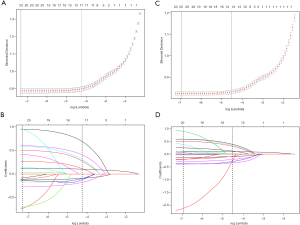
The calibration plots fit a prediction and observation data well in the derivation cohort (Figure S1A). The calibration of predictions from the full model demonstrated an excellent correlation between observed and predicted all-cause mortality (Figure S1B). As shown in Figure S2A, the area under the ROC curve for the derivation sample model was 0.865, suggesting that the simplified clinical tool has a high predictive power to all-cause mortality. The LASSO model showed a great prediction of prognostic capacity for all-cause mortality in middle-aged and elderly general population with an area under the curve (AUC) of 0.854 in the validation cohort (Figure S2B). Compared with the full model, simplified model and LASSO model between derivation and validation cohort, we found that these three models have almost the same area under the curve, indicating that the simplified model has a good predictive performance and stability for all-cause mortality among middle-aged and elderly general population.
Discussion
In the present study, we developed and validated a simple and accurate nomogram model to predict all-cause mortality in the nationally representative cohort. The nomogram model included nine items: age, gender, smoking, alcohol intake, diabetes, hypertension, marriage, education and PIR. To the best of our knowledge, this is the first study to develop a predictive model to predict all-cause mortality among middle-aged and elderly general population. The nomogram model demonstrated good accuracy and discrimination through different model analytical methods.
In the multivariate Cox regression analysis, we found that SBP (15), smoking (16), diabetes (17), hypertension, age and marriage status (18) were significantly associated with all-cause mortality. Our results agreed with previous studies. However, we also showed that DBP, education level, BMI and PIR were inversely associated with all-cause mortality. The J-curve phenomenon of DBP with adverse cardiovascular events has been reported, especially in post-hoc analysis and observational studies. A previous study demonstrated that DBP of less than 70 mm Hg was associated with adverse cardiovascular outcomes, including mortality, supporting the existence of a J-curve relationship (19). The magnitude of relationship between BMI and all-cause mortality is still controversial. A meta-analysis of 239 prospective studies in four continents demonstrated that the associations of both overweight and obesity with higher all-cause mortality were broadly consistent in four continents (20). However, a dose-response meta-analysis of prospective cohort studies found a U-shaped association between BMI and all-cause mortality (21).
Therefore, we established a full model including age, gender, smoking, alcohol intake, diabetes, hypertension, marriage status, education levels, SBP, BMI and PIR. Although the full model has good predictive value, the nomogram revealed that the range of SBP and BMI scores vary narrowly, suggesting the utility of SBP and BMI were affected in this full model. In order to optimize the model, step Akaike information criterion was used to screen the optimal variables into the simplified model. Interestingly, the simplified model has a same AUC in the derivation and validation cohort, but with higher accuracy and specificity compared to the full model in the validation cohort.
Further, LASSO model including the same items of the simplified model were performed by validating the predictive value for all-cause mortality. LASSO model discriminated all-cause mortality best with an AUC of 0.865 and 0.854 in the derivation and validation cohort, suggesting were similar to simplified model with an AUC of 0.842 and 0.849, respectively. In addition, decision curve analysis for the prediction model and time-dependent AUC values of the model in the derivation and validation cohort showed that the simplified model has good stability and accuracy. These results indicated that the model could be easily implemented into clinical practice with convenient, practical and accurate.
In a recent previous study demonstrated that regression models that used age, sex, and indicator variables for the Johns Hopkins’ Aggregated Diagnosis Groups (ADGs) categories have accurately predicted one-year all-cause mortality in population-based cohorts of subjects (22). Moreover, there was also research indicated that the Mortality Risk Score (MRS) collapses age, sex, and the ADGs to a single summary score could predict the annual risk of all-cause death in adults very well (23). The ADGs including 32 diagnosis clusters based on the International Classification of Disease (ICD) codes (-9 version, -9-CM version, or -10 version) (23). Although MRS and ADGs accurately predicted one-year mortality in a general population cohort, these two models included too many variables, which might be difficult to promote in clinical practice. In the present study, our model included fewer variables and has similar discriminating power for all-cause mortality in general adults. It means that our model may be useful for risk assessment and warning in primary care health services.
However, our study has some limitations. On the one hand, our current study did not include blood biomarkers, and we did not adjust some confounding factors of mortality. On the other hand, the simplified model is established in the American population and might not be directly applied to other population.
In conclusion, the present study developed and validated a prediction nomogram that can be conveniently used to predict all-cause mortality among middle-aged and elderly general population. Our model included easy-to-use parameters with good accuracy, which might have important implications for clinical practice.
Acknowledgments
Funding: This work was supported by the Science and Technology Program of Guangzhou (No. 201604020143, No. 201604020018, No. 201604020186, and No. 201803040012), the National Key Research and Development Program of China (No. 2017YFC1307603, No. 2016YFC1301305) and the Key Area R&D Program of Guangdong Province (No. 2019B020227005).
Footnote
Reporting Checklist: Available at http://dx.doi.org/10.21037/apm-20-580
Data Sharing Statement: Available at http://dx.doi.org/10.21037/apm-20-580
Conflicts of Interest: All authors have completed the ICMJE uniform disclosure form (available at http://dx.doi.org/10.21037/apm-20-580). The authors have no conflicts of interest to declare.
Ethical Statement: The authors are accountable for all aspects of the work in ensuring that questions related to the accuracy or integrity of any part of the work are appropriately investigated and resolved. The survey protocol was approved by the Institutional Review Board of the Centers for Disease Control and Prevention (Protocol #98-12, Protocol #2005-06, Continuation of Protocol #2005-06). The study conformed to the provisions of the Declaration of Helsinki (as revised in 2013). All participants gave written informed consent.
Open Access Statement: This is an Open Access article distributed in accordance with the Creative Commons Attribution-NonCommercial-NoDerivs 4.0 International License (CC BY-NC-ND 4.0), which permits the non-commercial replication and distribution of the article with the strict proviso that no changes or edits are made and the original work is properly cited (including links to both the formal publication through the relevant DOI and the license). See: https://creativecommons.org/licenses/by-nc-nd/4.0/.
References
- Rantakokko M, Tormakangas T, Rantanen T, et al. Environmental barriers, person-environment fit and mortality among community-dwelling very old people. Bmc Public Health 2013;13:783. [Crossref] [PubMed]
- Stringhini S, Zaninotto P, Kumari M, et al. Socio-economic trajectories and cardiovascular disease mortality in older people: the English Longitudinal Study of Ageing. Int J Epidemiol 2018;47:36-46. [Crossref] [PubMed]
- Kalediene R, Prochorskas R, Sauliune S. Socio-economic mortality inequalities in Lithuania during 2001-2009: the record linkage study. Public Health 2015;129:1645-51. [Crossref] [PubMed]
- Zhu S, Heo M, Plankey M, et al. Associations of body mass index and anthropometric indicators of fat mass and fat free mass with all-cause mortality among women in the first and second National Health and Nutrition Examination Surveys follow-up studies. Ann Epidemiol 2003;13:286-93. [Crossref] [PubMed]
- Flegal KM, Kit BK, Orpana H, et al. Association of all-cause mortality with overweight and obesity using standard body mass index categories: a systematic review and meta-analysis. JAMA 2013;309:71-82. [Crossref] [PubMed]
- Huang Y, Su L, Cai X, et al. Association of all-cause and cardiovascular mortality with prehypertension: a meta-analysis. Am Heart J 2014;167:160-8.e1. [Crossref] [PubMed]
- Yang JJ, Yu D, Wen W, et al. Association of Diabetes With All-Cause and Cause-Specific Mortality in Asia: A Pooled Analysis of More Than 1 Million Participants. JAMA Netw Open 2019;2:e192696. [Crossref] [PubMed]
- Manzoli L, Villari P, M, Pirone G, et al. Marital status and mortality in the elderly: a systematic review and meta-analysis. Soc Sci Med 2007;64:77-94. [Crossref] [PubMed]
- Khaing W, Vallibhakara SA, Attia J, et al. Effects of education and income on cardiovascular outcomes: A systematic review and meta-analysis. Eur J Prev Cardiol 2017;24:1032-42. [Crossref] [PubMed]
- Mons U, Muezzinler A, Gellert C, et al. Impact of smoking and smoking cessation on cardiovascular events and mortality among older adults: meta-analysis of individual participant data from prospective cohort studies of the CHANCES consortium. BMJ 2015;350:h1551. [Crossref] [PubMed]
- Ronksley PE, Brien SE, Turner BJ, et al. Association of alcohol consumption with selected cardiovascular disease outcomes: a systematic review and meta-analysis. BMJ 2011;342:d671. [Crossref] [PubMed]
- Curtin LR, Mohadjer LK, Dohrmann SM, et al. National Health and Nutrition Examination Survey: sample design, 2007-2010. Vital Health Stat 2 2013.1-23. [PubMed]
- Standards of medical care for patients with diabetes mellitus. American Diabetes Association. Diabetes Care 1994;17:616-23. [Crossref] [PubMed]
- Chobanian AV, Bakris GL, Black HR, et al. Seventh report of the Joint National Committee on Prevention, Detection, Evaluation, and Treatment of High Blood Pressure. Hypertension 2003;42:1206-52. [Crossref] [PubMed]
- Brunström M, Carlberg B. Association of Blood Pressure Lowering With Mortality and Cardiovascular Disease Across Blood Pressure Levels: A Systematic Review and Meta-analysis. Jama Intern Med 2018;178:28-36. [Crossref] [PubMed]
- Pan A, Wang Y, Talaei M, et al. Relation of Smoking With Total Mortality and Cardiovascular Events Among Patients With Diabetes Mellitus: A Meta-Analysis and Systematic Review. Circulation 2015;132:1795-804. [Crossref] [PubMed]
- Vrsalovic M, Vucur K, Vrsalovic PA, et al. Impact of diabetes on mortality in peripheral artery disease: a meta-analysis. Clin Cardiol 2017;40:287-91. [Crossref] [PubMed]
- Manfredini R, De Giorgi A, Tiseo R, et al. Marital Status, Cardiovascular Diseases, and Cardiovascular Risk Factors: A Review of the Evidence. J Womens Health (Larchmt) 2017;26:624-32. [Crossref] [PubMed]
- Vidal-Petiot E, Ford I, Greenlaw N, et al. Cardiovascular event rates and mortality according to achieved systolic and diastolic blood pressure in patients with stable coronary artery disease: an international cohort study. Lancet 2016;388:2142-52. [Crossref] [PubMed]
- Global BMI Mortality Collaboration, Di Angelantonio E, Bhupathiraju ShN, et al. Body-mass index and all-cause mortality: individual-participant-data meta-analysis of 239 prospective studies in four continents. Lancet 2016;388:776-86. [Crossref] [PubMed]
- Qin W, Liu F, Wan C. A U-shaped association of body mass index and all-cause mortality in heart failure patients: A dose-response meta-analysis of prospective cohort studies. Cardiovasc Ther 2017. [Crossref] [PubMed]
- Austin PC, van Walraven C, Wodchis WP, et al. Using the Johns Hopkins Aggregated Diagnosis Groups (ADGs) to predict mortality in a general adult population cohort in Ontario, Canada. Med Care 2011;49:932-9. [Crossref] [PubMed]
- Austin PC, Walraven C. The mortality risk score and the ADG score: two points-based scoring systems for the Johns Hopkins aggregated diagnosis groups to predict mortality in a general adult population cohort in Ontario, Canada. Med Care 2011;49:940-7. [Crossref] [PubMed]