The utility of the adjusted-OPTIMIZE-HF risk model for predicting in-hospital length of stay in the Chinese population
Introduction
Heart failure (HF) is the end status of many cardiovascular diseases. Because of its high rate of hospitalization and poor prognosis, its association with in-hospital mortality is relatively high, especially in some developed countries (1). For this reason, many models have been developed to predict in-hospital mortality in patients with HF in recent years (2-6), including the Organized Program to Initiate Lifesaving Treatment in Hospitalized Patients with Heart Failure (OPTIMIZE-HF) risk model. The OPTIMIZE-HF risk model was first proposed by Abraham et al. in 2008 (7). It was developed within a clinical population of American HF patients. It consisted of seven indicators including blood creatinine levels, systolic blood pressure, age, heart rate, sodium, primary cause of hospital admission (HF vs. other), and left ventricular ejection fraction (LVEF, <40% vs. ≥40%). In 2019, Yap et al. used the same variables to optimize the model for an Asian population (8). The validity of the adjusted-OPTIMIZE-HF risk model (whose indicators now included age, heart rate, systolic blood pressure, sodium, blood creatinine levels, LVEF <40% vs. >40%) was verified by the study.
Although this adjusted-OPTIMIZE-HF risk model was very effective in predicting the in-hospital mortality of Asian patients with HF, its ability to predict this population’s length of in-hospital stay (LOS) is unknown. As one of the indicators closely related to HF, LOS is an important outcome for both doctors and patients. The ability to predict patients’ LOS will not only help stratify patients, but also assist with resource allocation. Therefore, this study aimed to explore the association between the adjusted-OPTIMIZE-HF risk model and LOS in Asian patients with acute decompensated heart failure (ADHF). We present the following article in accordance with the STROBE reporting checklist (available at http://dx.doi.org/10.21037/apm-20-1209).
Methods
Study design and population
This was a single-center (Sir Run Run Shaw Hospital, Hangzhou, Zhejiang Province, China), retrospective study of 4,481 consecutive patients with ADHF admitted to the study hospital between 2010 and 2019. We included patients with a confirmed diagnosis of ADHF (classified in accordance with the criteria put forth by the European Society of Cardiology, ESC) that was available in the hospital electronic system. According to the ESC criteria, an ADHF diagnosis should be based upon a thorough medical history that includes assessment of symptoms, prior cardiovascular history, and potential cardiac and non-cardiac precipitants, as well as a physical examination that evaluates signs/symptoms of congestion and/or hypo-perfusion. Symptoms should be further confirmed by appropriate additional investigations such as an electrocardiogram (ECG), chest X-ray, laboratory assessment (of specific biomarkers) and echocardiography. We excluded patients with recurrent admissions for ADHF. Study protocols were in accordance with the Declaration of Helsinki (as revised in 2013) and all participants provided written informed consent. This study was approved by the Institutional Ethics Research Committee of Sir Run Run Shaw Hospital (No. 20200803-34).
Outcomes
The primary outcome was the LOS. It was calculated by subtracting the discharge date from the admission date. This inpatient time-period excluded individuals that died in-hospital (from any cause).
Statistical methods
We described categorical variables using percentages and used the chi-square test to test for differences between groups. We described continuous variables using medians (first quartile, third quartile) due to their non-normal distribution (as assessed by the Kolmogorov-Smirnov test) (Figure S1). In addition, we performed univariate and multivariate logistic regression analyses to determine the relations between our variables of interest and LOS as well as in subgroup analysis. Variables were included in the multivariate logistic regression if P<0.1 in univariate logistic regressions. The optimal cut-off levels and the area under the curve (AUC) were calculated by receiver operator characteristic (ROC) curve analyses in the overall study population as well as subgroups defined by gender, history of smoking, history of drinking, presence of hypertension, and diabetes. We considered P<0.05 to be statistically significant and conducted analyses using Statistical Package for the Social Sciences (SPSS) for Windows (version 26, SPSS Inc., Chicago, IL, USA).
Results
A total of 4,481 patients with ADHF [61.6% male, median age 71 years (range, 62–78 years)] were included in our study. We divided them into two groups according to median LOS—the short LOS group (n=2,177, LOS <6 days) and the long LOS group (n=2,304, LOS ≥6 days). The median OPTIMIZE-HF risk score in the overall population was 1.20 (range, 0.75–1.92). Table 1 presents participants’ demographic data.
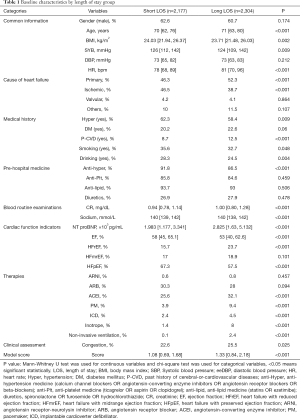
Full table
We divided the demographic variables in Table 1 into seven categories: common information, cause of HF, medical history, pre-hospital medication, routine blood examinations, cardiac function index, clinical assessment, therapies, and OPTIMIZE-HF. Comparisons between the short LOS and the long LOS groups showed statistical differences across all variables with the exception of gender, diastolic pressure, HF caused by valvular heart diseases, other causes of HF, antilipidemic drugs, antiplatelet drugs, and diuretics.
Univariate and multivariate logistic regressions further assessed the relations between these demographic variables and LOS. Table 2 presents the results of these logistic regression analyses. In univariate logistic regression analyses, all but eight variables (i.e., gender, diastolic blood pressure, HF caused by valvular heart diseases, other causes of HF, diabetes, antilipidemic drugs, antiplatelet drugs, and diuretics) were significantly associated with LOS. Similarly, no significant differences were present between the short LOS and long LOS groups across these eight variables at baseline. Variables with a P<0.1 in univariate regression analyses were included in the multivariate logistic regression analysis. The results showed that heart rate, creatinine, sodium, NT-proBNP, EF, inotrope and non-invasive ventilation were independently associated with LOS (P<0.05).
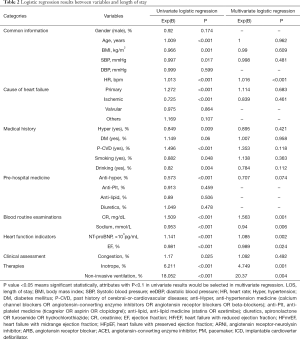
Full table
Figure 1 presents a direct comparison of the risk scores of the short LOS group (median score 1.08) and long LOS group (median score 1.33). This difference in risk scores was statistically significant (P<0.001). In order to further explore the relation between the risk score and LOS, variables with a P<0.1 (including the risk score) were selected for multivariate regression. Since the risk score is calculated using six variables (i.e., age, heart rate, systolic blood pressure, sodium, creatinine and LVEF <40%), we excluded these variables from the multivariate regression even if they were significant at P<0.01 in univariate regressions. The results are presented in Table 3 (OR 1.248, 95% CI: 1.094–1.424, P=0.001).
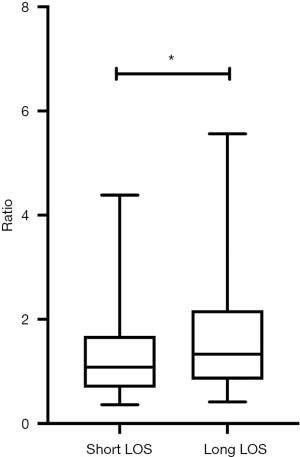
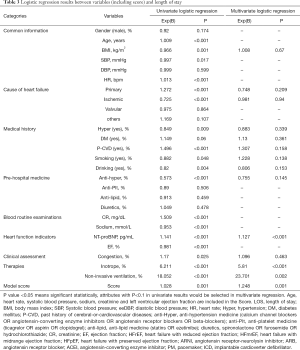
Full table
We performed a ROC curve analysis to assess the predictive ability of risk scores. We calculated the AUC (AUC 0.583, 95% CI: 0.567–0.600, P<0.001) and used Youden’s index to determine the optimal cut-off value (cut-off value 1.32, sensitivity 0.507, specificity 0.626). Figure 2 displays these results. Table 4 presents the AUC results which showed that the risk score in all subgroups performed well in predicting LOS. Table 5 displays the relations between the risk score and LOS in specific subgroups (i.e., gender, smoking history, drinking history, presence of diabetes, and presence of hypertension).
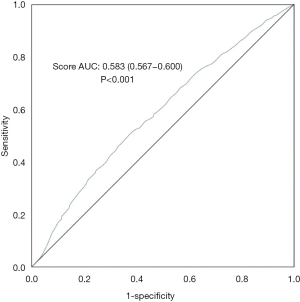

Full table
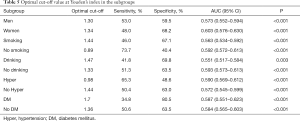
Full table
Discussion
Our study’s main findings were that the adjusted-OPTIMIZE-HF risk model had the potential to predict LOS in patients with ADHF and had stable predictive ability in certain subgroups. In multivariate analyses, we found that heart rate, creatinine, sodium, NT-proBNP, EF, inotrope and non-invasive ventilation were independent predictors of LOS. Some of these results (i.e., sodium, heart rate, NT-proBNP, and creatinine) have also been reported in other studies (9-12). Generally speaking, lower sodium may prolong LOS because of the dilution hyponatremia caused by the decrease of water excretion in the kidney. An elevated heart rate negatively impacts the myocardium, which makes HF worse. Since NT-proBNP levels are used to diagnose the severity of HF, as well as its prognosis, it is not surprising that patients with higher NT-proBNP levels had longer LOS. Creatinine, as a biomarker, has a negative association with renal function. In the one hand, renal dysfunction is at a high risk in ADHF patients due to the hemodynamic, venous congestion, the use of drugs like diuretics and so on (13), in the other hand, the reduced urine output may worsen the decreased cardiac output and cause a longer LOS. Although the use of inotropes can improve the symptoms of patients with critical HF, studies have demonstrated that it has adverse reactions (14). Non-invasive ventilation may be associated with longer LOS because patients who need non-invasive ventilation, generally speaking, are at a critical state in their illness. Thus, although non-invasive ventilation has been shown to reduce LOS (15), these patients may still have a longer LOS than those in earlier stages of the illness. As for EF, to some extent, it is negatively related to the severity of HF and is indirectly related to LOS. In addition to the variables we found to be independently associated with LOS, studies have also found independent relations between LOS and other variables as well. For example, Massari et al. demonstrated the role of congestion in predicting LOS in HF patients (16). This variable was also investigated in our research, though it was not significant in our multivariable regression. Furthermore, Wright et al. found that weight changes, duration of diuretic use, impairment of renal function, social problems, and respiratory complications affected LOS in patients with HF (17) while Sahin et al. (18) found that anemia, severe mitral regurgitation, systolic blood pressure, and urea nitrogen were also independent predictors of LOS. Finally, studies have reported that albumin and troponin levels may also predict LOS in HF patients (19,20). In conclusion, future research should consider including these variables if available. This future work has the potential to contribute to the development of a new predictive model of LOS in HF patients.
Many predictive models have been proposed in the past which are relevant to LOS, including the artificial neural network (ANN) model, intensive care delivery screening checklist (ICDSC) score, and Braden Score (21-23). However, limitations exist within these models. The ANN model was found to have low accuracy and the inability to predict longer LOS (21). For the ICDSC score, the sample size was too small and the relations between the score and LOS still need to be verified (22). Finally, the Braden Score might not be generalizable because it was developed through a single-center-study (23). A predictive risk model that is widely used in the assessment of LOS is still relatively lacking (in terms of, for example, considerations related to accuracy, specificity, and applicability), so it is important to develop a model with improved predictive ability. The OPTIMIZE-HF risk model used in this study was first proposed in 2008 and shown to be able to predict the in-hospital mortality of patients with HF. Later, it was also confirmed to have good predictive ability for other indicators. For instance, Nguyen et al. demonstrated that the model could help determine the best candidate for heart transplantation (24). The model was also shown to have good predictive ability for one-year mortality (with the exception of in-hospital mortality) in patients in a cardiac intensive care unit (25). To our knowledge, ours is the first study to explore the relations between the OPTIMIZE-HF risk model and LOS in patients with ADHF.
Our study established the potential of the adjusted-OPTIMIZE-HF risk model to predict LOS in patients with ADHF. Yap et al.’s study found that the adjusted-OPTIMIZE-HF risk model performed well in predicting in-hospital mortality in 15,219 Singaporeans. Their adjusted-OPTIMIZE-HF model had a C-statistic of 0.741. Since the initial purpose of their model was to predict the in-hospital mortality of patients with HF, and the in-hospital mortality reflected the severity of HF, we expected there to be relations between the model and one predicting LOS since patients with more advanced stages of HF usually have longer LOS. This was also indicated by the six variables in the model, which were all closely related to the severity of HF. Therefore, it was reasonable to predict LOS through their use. Any deviations in predictive ability may be due to the fact that some patients with a higher severity of HF died earlier during hospitalization, which meant their LOS was shorter and undermined the strength of the relation between severity of HF and LOS.
ROC curve analyses determined that our model’s predictive accuracy was unaffected by subgroup analyses, which reflects the stability of the predictive model and its applicability to the general HF population as well as specific subgroups within it. A possible explanation for its stability across groups is that the model was established and/or adjusted within a wide range of populations.
Studies by Formiga et al. (26) and Zaprutko et al. (27) confirmed that the New York Heart Association (NYHA) classification of patients with HF was independently related to LOS, and it was included in many risk models used to predict patient mortality. This variable was not included in our study because it was unavailable in our dataset. Thus, further research could explore the influence of this variable in an adjusted-OPTIMIZE-HF model. Secondly, although the adjusted-OPTIMIZE-HF model used in this study was recalibrated for use in the Asian population, the cohort in Yap et al.’s study was primarily composed of Singaporeans. No validation studies have been performed in a Chinese cohort. Thus, subsequent studies should consider readjusting the model based on Chinese data and then investigating whether the adjusted model’s ability to predict the LOS in HF patients remains the same or even improves. Finally, the results of the ROC curve analysis show that there is still room for improvement in the model’s predictive abilities.
Several limitations exist within our study that warrant mentioning. First, our study was a single-center, retrospective observational study. Although we used multivariate logistic regression to adjust for variables, we could not avoid all sources of bias and confounding due to our study’s retrospective nature. Secondly, we were unable to include the NYHA classification as a variable in our study. Previous studies reported a positive association between this variable and the LOS of patients with HF so there is a strong possibility that this relation exists. Thirdly, as mentioned previously, the adjusted-OPTIMIZE-HF risk model used in this study was developed in a cohort primarily comprised of Singaporeans. Whether this adjusted-OPTIMIZE-HF risk model is representative of the Chinese population warrants further investigation. Finally, although the predictive ability of the adjusted-OPTIMIZE-HF risk model was confirmed, a more accurate predictive model or formula is worth exploring in the future.
Conclusions
In conclusion, the adjusted-OPTIMIZE-HF risk model performed well in predicting LOS greater than 6 days in the Chinese patients with ADHF. This predictive ability was stable in subgroups defined by gender, history of smoking, history of drinking, presence of hypertension, and presence of diabetes.
Acknowledgments
The authors would like to express their gratitude to EditSprings (https://www.editsprings.com/) for the expert linguistic services provided.
Funding: This work was supported by the National Natural Science Foundation of China (81500212 and 81800212) and Zhejiang Natural Science Foundation (LY18H020007 and LQ16H020001).
Footnote
Reporting Checklist: The authors have completed the STROBE reporting checklist. Available at http://dx.doi.org/10.21037/apm-20-1209
Data Sharing Statement: Available at http://dx.doi.org/10.21037/apm-20-1209
Conflicts of Interest: All authors have completed the ICMJE uniform disclosure form (available at http://dx.doi.org/10.21037/apm-20-1209). The authors have no conflicts of interest to declare.
Ethical Statement: The authors are accountable for all aspects of the work in ensuring that questions related to the accuracy or integrity of any part of the work are appropriately investigated and resolved. The study was designed and implemented in accordance with the ethical principles of the Declaration of Helsinki (as revised in 2013). All patients gave a written consent to participate in the study. This study was approved by the Institutional Ethics Research Committee of Sir Run Run Shaw Hospital (No. 20200803-34).
Open Access Statement: This is an Open Access article distributed in accordance with the Creative Commons Attribution-NonCommercial-NoDerivs 4.0 International License (CC BY-NC-ND 4.0), which permits the non-commercial replication and distribution of the article with the strict proviso that no changes or edits are made and the original work is properly cited (including links to both the formal publication through the relevant DOI and the license). See: https://creativecommons.org/licenses/by-nc-nd/4.0/.
References
- Bytyçi I, Bajraktari G. Mortality in heart failure patients. Anatol J Cardiol 2015;15:63-8. [Crossref] [PubMed]
- Cameli M, Pastore MC, De Carli G, et al. ACUTE HF score, a multiparametric prognostic tool for acute heart failure: A real-life study. Int J Cardiol 2019;296:103-8. [Crossref] [PubMed]
- Fonarow GC, Adams KF Jr, Abraham WT, et al. Risk stratification for in-hospital mortality in acutely decompensated heart failure: classification and regression tree analysis. JAMA 2005;293:572-80. [Crossref] [PubMed]
- Lupón J, de Antonio M, Vila J, et al. Development of a novel heart failure risk tool: the barcelona bio-heart failure risk calculator (BCN bio-HF calculator). PLoS One 2014;9:e85466. [Crossref] [PubMed]
- Salah K, Kok WE, Eurlings LW, et al. A novel discharge risk model for patients hospitalised for acute decompensated heart failure incorporating N-terminal pro-B-type natriuretic peptide levels: a European coLlaboration on Acute decompeNsated Heart Failure: ELAN-HF Score. Heart 2014;100:115-25. [Crossref] [PubMed]
- Senni M, Parrella P, De Maria R, et al. Predicting heart failure outcome from cardiac and comorbid conditions: the 3C-HF score. Int J Cardiol 2013;163:206-11. [Crossref] [PubMed]
- Abraham WT, Fonarow GC, Albert NM, et al. Predictors of in-hospital mortality in patients hospitalized for heart failure: insights from the Organized Program to Initiate Lifesaving Treatment in Hospitalized Patients with Heart Failure (OPTIMIZE-HF). J Am Coll Cardiol 2008;52:347-56. [Crossref] [PubMed]
- Yap J, Lim FY, Chia SY, et al. Prediction of Survival in Asian Patients Hospitalized With Heart Failure: Validation of the OPTIMIZE-HF Risk Score. J Card Fail 2019;25:571-5. [Crossref] [PubMed]
- Omar HR, Guglin M. Longer-than-average length of stay in acute heart failure: Determinants and outcomes. Herz 2018;43:131-9. [Crossref] [PubMed]
- Davison BA, Metra M, Senger S, et al. Patient journey after admission for acute heart failure: length of stay, 30-day readmission and 90-day mortality. Eur J Heart Fail 2016;18:1041-50. [Crossref] [PubMed]
- Kitagawa T, Oda N, Mizukawa M, et al. Hospitalization and medical cost of patients with elevated serum N-terminal pro-brain natriuretic peptide levels. PLoS One 2018;13:e0190979. [Crossref] [PubMed]
- Palmer JB, Friedman HS, Waltman Johnson K, et al. Association of persistent and transient worsening renal function with mortality risk, readmissions risk, length of stay, and costs in patients hospitalized with acute heart failure. Clinicoecon Outcomes Res 2015;7:357-67. [PubMed]
- Sriperumbuduri S, Clark E, Hiremath S. New Insights Into Mechanisms of Acute Kidney Injury in Heart Disease. Can J Cardiol 2019;35:1158-69. [Crossref] [PubMed]
- Kaneko H, Itoh H, Yotsumoto H, et al. Association between the number of hospital admissions and in-hospital outcomes in patients with heart failure. Hypertens Res 2020. [Crossref] [PubMed]
- Wu Q, Xiang G, Song J, et al. Effects of non-invasive ventilation in subjects undergoing cardiac surgery on length of hospital stay and cardiac-pulmonary complications: a systematic review and meta-analysis. J Thorac Dis 2020;12:1507-19. [Crossref] [PubMed]
- Massari F, Scicchitano P, Ciccone MM, et al. Bioimpedance vector analysis predicts hospital length of stay in acute heart failure. Nutrition 2019;61:56-60. [Crossref] [PubMed]
- Wright SP, Verouhis D, Gamble G, et al. Factors influencing the length of hospital stay of patients with heart failure. Eur J Heart Fail 2003;5:201-9. [Crossref] [PubMed]
- Sahin S, Doğan U, Ozdemir K, et al. Evaluation of clinical and demographic characteristics and their association with length of hospital stay in patients admitted to cardiac intensive care unit with the diagnosis of acute heart failure. Anadolu Kardiyol Derg 2012;12:123-31. [Crossref] [PubMed]
- Nishino M, Matsuhiro Y, Nakamura H, et al. Which factors are associated with length of stay in older patients with acute decompensated heart failure with preserved ejection fraction?: AURORA study. Geriatr Gerontol Int 2019;19:1084-7. [Crossref] [PubMed]
- Greenberg BH, Abraham WT, Albert NM, et al. Influence of diabetes on characteristics and outcomes in patients hospitalized with heart failure: a report from the Organized Program to Initiate Lifesaving Treatment in Hospitalized Patients with Heart Failure (OPTIMIZE-HF). Am Heart J 2007;154:277.e1-8. [Crossref] [PubMed]
- Tsai PF, Chen PC, Chen YY, et al. Length of Hospital Stay Prediction at the Admission Stage for Cardiology Patients Using Artificial Neural Network. J Healthc Eng 2016;2016:7035463. [Crossref] [PubMed]
- Sakaguchi T, Watanabe M, Kawasaki C, et al. A novel scoring system to predict delirium and its relationship with the clinical course in patients with acute decompensated heart failure. J Cardiol 2018;71:564-9. [Crossref] [PubMed]
- Bandle B, Ward K, Min SJ, et al. Can Braden Score Predict Outcomes for Hospitalized Heart Failure Patients? J Am Geriatr Soc 2017;65:1328-32. [Crossref] [PubMed]
- Nguyen LS, Coutance G, Ouldamar S, et al. Performance of existing risk scores around heart transplantation: validation study in a 4-year cohort. Transpl Int 2018;31:520-30. [Crossref] [PubMed]
- Lyle M, Wan SH, Murphree D, et al. Predictive Value of the Get With The Guidelines Heart Failure Risk Score in Unselected Cardiac Intensive Care Unit Patients. J Am Heart Assoc 2020;9:e012439. [Crossref] [PubMed]
- Formiga F, Chivite D, Manito N, et al. Admission characteristics predicting longer length of stay among elderly patients hospitalized for decompensated heart failure. Eur J Intern Med 2008;19:198-202. [Crossref] [PubMed]
- Zaprutko J, Michalak M, Nowicka A, et al. Hospitalisation length and prognosis in heart failure patients. Kardiol Pol 2017;75:323-31. [PubMed]