Systematic analysis reveals a lncRNA-miRNA-mRNA network associated with dasatinib resistance in chronic myeloid leukemia
Introduction
Chronic myelogenous leukemia (CML) is a malignant tumor formed by the clonal proliferation of bone marrow hematopoietic stem cells. There are 1–2 cases per 100,000 people, which accounts for about 15% of newly diagnosed leukemia cases in adults, and the incidence of CML increases annually (1). In the past 20 years, with the advent of imatinib [the first generation of tyrosine kinase inhibitors (TKIs)], TKIs have turned CML from fatal diseases into manageable chronic diseases (2). The majority of patients with CML who received BCR-ABL1 TKIs responded well (3). However, imatinib is far from perfect, with only approximately 60% of CML patients remaining on the standard daily dose of 400 mg after six years, either due to lack of drug tolerance or drug resistance. Among second-generation drugs, both dasatinib and nilotinib are more effective than imatinib, and they have been licensed in the United States. The emergence of TKIs in the treatment of CML opened a new era of precision medicine for various malignancies. In this era, relatively non-specific and toxic drugs were gradually replaced by safer and more tolerated drugs (4). Dasatinib is a second-generation TKI, with a longer-lasting intact hematological and cytogenetic response, as well as resistance to imatinib-resistant or intolerant CML. It is more potent and also shows advantages in newly diagnosed CML compared to imatinib (5). Although TKI has completely changed the CML patients’ treatment patterns and greatly improved its treatment effect, drug resistance remains a significant problem to be solved (6). Therefore, studying the resistance mechanisms of CML and finding new targets for prevention and treatment are still hot topics in the field of cancer research.
Long non-coding RNA (lncRNA) is a non-protein transcript that ranges in length from 200 nucleotides (nt) to about 100 kilobases (kb) (7). While lncRNAs are post-transcriptionally modified in a way similar to mRNA, they are not translated into protein (8), but served key regulatory roles, such as mediating activity or localization of proteins, providing organizational scaffolds for subcellular structures, modulating transcriptional programs, and regulating miRNA expressions instead (9,10). Importantly, a large number of misregulated lncRNAs are involved in the occurrence and development of human cancers. These lncRNAs are involved in the regulation of cancer cell growth, metastasis, and chemotherapy drug resistance through a variety of mechanisms (11). An increasing number of studies report that dysregulation of lncRNA induces drug resistance in cancer cells (12). For example, NF-κB/HOTAIR promotes chemical resistance in ovarian cancer (13). Silencing linc-ROR can attenuate the expression of CD133+ cells in tumor-initiating cells, leading to the development of resistance to sorafenib in liver cancer cells (14). LncRNAs facilitate resistance to Ara-C treatment in AML (15). However, the potential role of lncRNAs in dasatinib resistance in chronic myeloid leukemia cells is unknown. It has been reported that the lncRNA MALAT1/miR-328 axis promotes CML cells’ proliferation and imatinib resistance (16).
In our study, we followed the diagram in Figure 1 and determined the expression profile of dasatinib resistance in CML cells through bioinformatics analysis, and aims to construct a lncRNA-miRNA-mRNA network for dasatinib resistance in CML cells. The key lncRNAs involved in drug resistance were revealed, which may pave the way for further research to identify clinical treatment targets or potential biomarkers of dasatinib resistance in chronic myeloid leukemia cells. We present the following article in accordance with the MDAR reporting checklist (available at http://dx.doi.org/10.21037/apm-20-343).
Methods
Chip data
Gene expression data for both dasatinib-sensitive and dasatinib-resistant samples GSE33290 were downloaded from GEO (http://www.ncbi.nlm.nih.gov/geo/) (17). All raw data are standardized and log2 transformed. The differentially expressed genes were compared by GEO2R (https://ncbi.nlm.nih.gov/geo/geo2r/), comparing two or more samples in the GEO series. Differentiation expressed genes were identified according to |log2 (fold change)| >2 and P<0.05.
Protein-protein interaction (PPI) network construction & hub-genes identification
A PPI network was set up by the STRING (v10.5) (https://string-db.org/) (18) and represented via Cytoscape 3.6.1. Then, we used 3 algorithms (Betweenness, Degree, Closeness) to analyze the overlapping genes with CytoHubba, which was employed to recognize highly interacted hub-genes (19).
Bioinformatic analysis
To explore the functional annotation and pathway enrichment, the Gene Ontology and Kyoto Encyclopedia of Genes and Genomes database analyses were conducted using a Database for Annotation, Visualization and Integrated Discovery (DAVID: available online: http://david.abcc.ncifcrf.gov/) (20,21), FunRich (http://www.funrich.org/) (22) and STRING (https://string-db.org/).
Establishment of lncRNA-miRNA-mRNA network
Due to the complexity of mRNA-miRNA interactions, a single mRNA may predict a large number of miRNAs. Web-based tools mirDIP, miRDB, and miRanda were used to identify and predict miRNA functions, and plot the three sets of Wayne diagrams (http://bioinformatics.psb.ugent.be/webtools/Venn/). The obtained miRNAs were used to predict lncRNA in STARBASE and LncBase Predicted v.2. The network was visualized by Cytoscape3.6.1 software.
Survival analysis
Gene expression profiling interactive analysis (GEPIA) and OncoLnc (http://www.oncolnc.org/) are visualization webs server for analyzing the RNA sequencing expression data based on the TCGA (23). Overall survival was evaluated with the Kaplan-Meier method.
Statistical analysis
Data were expressed as the mean ± SD from at least three independent experiments for each group. All statistical analyses were performed with SPSS 19.0 (SPSS Inc., Chicago, IL, USA) and P less than 0.05 was considered statistically significant.
Results
Expression profiles of mRNAs in chronic myeloid leukemia
The microarray dataset (GSE33290) that comprises mRNA expression profile in CML were analyzed. Thirty-nine mRNAs were significantly differentially expressed in dasatinib-resistant chronic myeloid leukemia patients compared with the dasatinib-sensitive CML patients in the GEO dataset. As a result, only 6 mRNAs were upregulated, whereas 33 mRNAs were downregulated on the criteria that |logFC| >2 and P<0.05. Sorted by |logFC| size, some high- and low-expressed gene names and corresponding information are shown in Table 1.
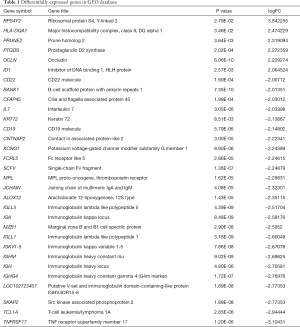
Full table
Recognition of hub genes from PPI network with CytoHubba
After introducing DEGenes into STRING, 28 isolated and non-interacting genes were removed, and the interactions among the overlapping genes was displayed in a PPI network in Figure 2A. The remaining 12 interacting genes were introduced into CYTOSCAPE for network visualization. The subnetwork was showed in Figure 2B, including 12 nodes and 25 edges were screened with CytoHubba, which revealed the vital roles of the twelve hub genes (HLA-DQA1, MPL, BANK1, TCL1A, TNFRSF17, MZB1, IL7, CD19, IGJ, IGLL1, IGLL5, IGHV4-38-2) in dasatinib resistance.
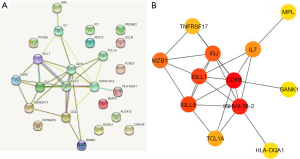
Functional enrichment of the twelve hub genes
Gene Ontology (GO) analysis in FunRich found that DEGenes focused on molecular function (MF) in receptor activity and receptor signaling complex scaffold activity in Figure 3A. Figure 3B demonstrated that these functions are closely related to immune response, signal transduction, and cell communication in biological processes (BP). The matching proteins and FDR were devoted to revealing the value of functional enrichment analysis. The enriched gene oncology terms and pathways were relevant with an adjusted FDR <0.05.
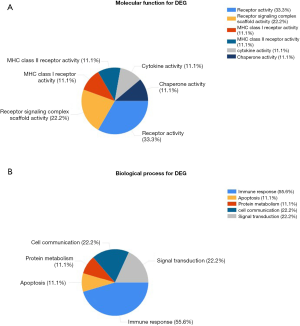
KEGG analysis of DEGenes in STRING demonstrated that these genes mainly interact with PI3K-Akt signaling pathway, Jak-STAT signaling pathway, and cytokine-cytokine receptor. According to the FDR <0.05 standard, the related enrichment pathways and their corresponding gene names were shown in Table 2.
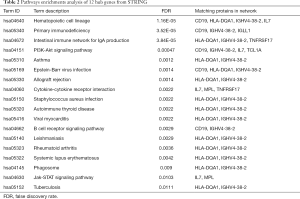
Full table
LncRNA-miRNA-mRNA network construction
The miRDB database was used to predict a total of 1,666 corresponding miRNAs. The mirDIP database was used to predict a total of 76 corresponding miRNAs. In addition, 172 corresponding miRNAs were predicted by using miRanda. Then, we focused on delineating the dasatinib resistance-specific mRNA-miRNA correlation relationship. The dasatinib resistance-specific mRNA-miRNA correlation network contained 12 mRNAs, 172 miRNAs predicted in miRanda and 135 mRNA-miRNA correlation edges in Figure 4A. Based on the above results, the network diagram constructed in the CYTOSCAPE for the predicted lncRNA, miRNAs and mRNAs. The network contains 15 nodes (7 lncRNAs, 3 miRNAs, 5 mRNAs) and 14 relationship pairs. Figure 4B summarized the network including seven lncRNAs (MALAT1, OIP5-AS1, LINC00665, LINC00657, ERVK3-1, NEAT1, CTC-444N24.11), three miRNAs (hsa-miR-28-5p, hsa-miR-129-5p, hsa-miR-543) and five mRNAs (MPL, IL7, IGJ, HLA-DQA1, BANK1) which were generated by Cytoscape 3.6.1.
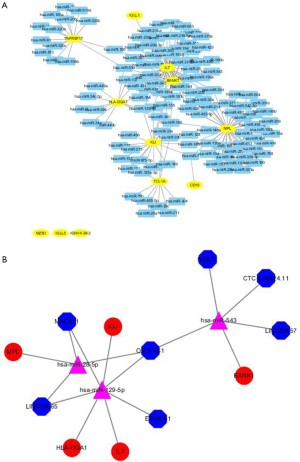
Three overlapping miRNAs that might play critical roles in dasatinib-resistance were selected by intersecting the predicted miRNAs (Figure 5). These 3 miRNAs were used to predict the upstream lncRNA in STARBASE and LncBase Predicted v.2. According to the screening criteria, 7 lncRNAs were predicted.
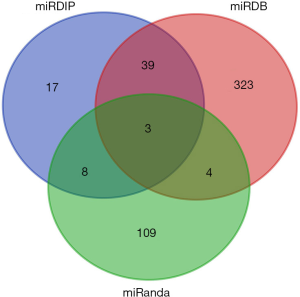
Key RNAs and their associated clinical features
In order to identify the specific RNAs with prognostic characteristics, RNAs were chosen according to the bioinformatics analysis and the LncRNA-miRNA-mRNA network were analyzed the effects significant for survival (P<0.05) through analysis of the association between key RNAs and CML patients’ survival periods.
Among the differentially expressed RNAs, one mRNA (IL7), one miRNA (hsa-miR-28-5p), and one lncRNA (MALAT1) were found to be associated with the overall survival of patients with CML by prognosis analysis. Kaplan-Meier survival curves indicated that the lncRNA MALAT1 (Figure 6A) positively correlated with overall survival, whereas IL7 (Figure 6B) and hsa-miR-28-5p (Figure 6C) were negatively associated with overall survival.
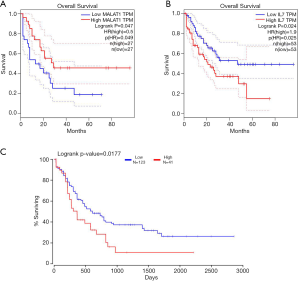
Characteristics of MALAT1 in CML
According to GO analysis, anomalous expression of MALAT1 is related to aberrant regulation of gene function groups such as regulation of alternative mRNA splicing, protein binding, and protein complex binding in Figure 7A.
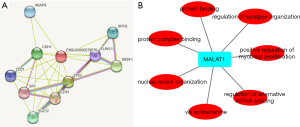
Protein–protein interaction analysis revealed that metastasis associated lung adenocarcinoma transcript 1 (non-protein coding) (MALAT1) can interact with several proteins including A-kinase anchoring protein 9 (AKAP9), splicing factor proline and glutamine rich (SFPQ), serine and arginine rich splicing factor 1 (SRSF1), ELAV like RNA binding protein 1 (ELAVL1), tumor protein p53 (TP53), enhancer of zeste 2 polycomb repressive complex 2 subunit (EZH2), SUZ12 polycomb repressive complex 2 subunit(SUZ12), Sp1 transcription factor (SP1), cadherin 1(CDH1), zinc finger E-box binding homeobox 1 (ZEB1) in Figure 7B. KEGG analysis of MALAT1 in STRING revealed that it mainly interacts with IL-17 signaling pathway, transcriptional misregulation in cancer and endocrine resistance are closely related. According to the FDR<0.05 standard, the related enrichment pathways and their corresponding gene names are shown in Table 3.
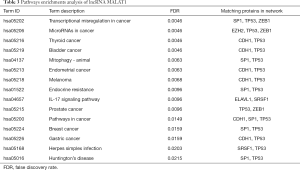
Full table
Discussion
CML is a relatively rare disease (5,980 new cases each year in the United States), mainly affecting elder patients (median age at diagnosis of 67 years), but the prevalence of CML is expected to increase dramatically. The effectiveness of TKI treatment and reduction in CML-related deaths is obvious (24). Therefore, the identification of biomarkers of CML resistance involved therein is essential for treatment and prognosis prediction.
In the GEO database, dasatinib-resistant CML sample information was used to screen 39 differentially expressed genes with high reliability, and 12 core genes were found. In this study, miRDIP, miRDB and miRanda were used to predict the upstream miRNAs of 12 core genes. Three miRNAs (hsa-miR-28-5p, hsa-miR-129-5p, hsa-miR-543) were found through the intersection analysis. Studies have shown that these miRNAs are closely related to leukemia development. For example, the decrease in miR-28-5p endogenous levels by dexamethasone counteract their ability to block NDRG2’s stress response increase to dexamethasone (25); miR-28-5p reinforce the notion that inactivation of targeted genes is linked to malignant progression in cancer (26). These results suggested that the predicted miRNAs might play an important role in promoting leukemia occurrence or progression.
Using STARBASE and LncBase Predicted v.2 software to predict the upstream lncRNAs of these 3 miRNAs, a total of 7 lncRNAs were screened (MALAT1, OIP5-AS1, LINC00665, LINC00657, ERVK3-1, NEAT1, CTC-444N24.11). Studies have shown that some of these lncRNAs are closely related to drug resistance. For example, LncRNA MALAT1 promotes cell proliferation and imatinib resistance by sponging miR-328 in CML. Lots of evidences were confirmed that MALAT1 related to drug resistance (27-29) and cancer development (30). These results indicate that the predicted lncRNAs play an important role in drug resistance in leukemia.
The GO analysis results showed that the 12 hub genes were obtained focused on the molecular functions of the receptor activity and the receptor signaling complex scaffold activity as well as MHC class 1 receptor activity, MHC class 2 receptor activity, cytokine activity, and chaperone activity. These functions are closely related to immune response, signal transduction and cellular communication, as well as protein metabolism and apoptosis. In addition, KEGG analysis showed that these genes are mainly related to the hematopoietic cell lineage, primary immune deficiency, asthma, autoimmune thyroid disease, rheumatoid arthritis, systemic lupus erythematosus, allograft rejection, and also related to PI3K-Akt Signaling pathway, Jak-STAT signaling pathway, B cell receptor signaling pathway, the interaction of cytokines and cytokine receptors, as well as the intestinal immune network that produces IgA, phagosomes; Epstein-Barr virus infection, Staphylococcus aureus infection, Viral myocarditis, leishmaniasis, tuberculosis. These pathways predicted above are related to metabolism, apoptosis, immunity and transcription regulation, which may be related to the potential regulatory functions of CML.
Through systematic analysis, the dasatinib-resistant lncRNA-miRNA-mRNA network of CML were revealed. We also found that the lncRNAs involved in dasatinib resistance primarily regulate metabolic pathways and the key lncRNA MALAT1 was related to the positive prognosis of CML. The expressions of IL7 and hsa-miR-28-5p were negatively correlated with the overall survival of patients. Nevertheless, there are no reports on the association of MALAT1 with dasatinib-resistance in CML. In addition, other lncRNAs that involved in dasatinib resistance of CML cells were deserved detailed analysis in the future.
Conclusions
All in all, we predict that MALAT1 as a lncRNA may be a tumor suppressor for CML patients. According to our data, MALAT1 may have potential as a molecular biomarker for the occurrence and development of CML resistance. Whether it can be effective therapeutic targets for changing the prognosis of tolerating dasatinib in CML patients is worth further exploration.
Acknowledgments
Funding: This work was funded by the National Nature Science Foundation of China (No. 81401391), China Postdoctoral Science Foundation Grant (No. 2015M570696) and High-level University Construction Research and Teaching Academic Upgrading project of Guangzhou Medical University.
Footnote
Reporting Checklist: The authors have completed the MDAR reporting checklist. Available at http://dx.doi.org/10.21037/apm-20-343
Conflicts of Interest: All authors have completed the ICMJE uniform disclosure form (available at http://dx.doi.org/10.21037/apm-20-343). The authors have no conflicts of interest to declare.
Ethical Statement: The authors are accountable for all aspects of the work in ensuring that questions related to the accuracy or integrity of any part of the work are appropriately investigated and resolved.
Open Access Statement: This is an Open Access article distributed in accordance with the Creative Commons Attribution-NonCommercial-NoDerivs 4.0 International License (CC BY-NC-ND 4.0), which permits the non-commercial replication and distribution of the article with the strict proviso that no changes or edits are made and the original work is properly cited (including links to both the formal publication through the relevant DOI and the license). See: https://creativecommons.org/licenses/by-nc-nd/4.0/.
References
- Jabbour E, Kantarjian H. Chronic myeloid leukemia: 2018 update on diagnosis, therapy and monitoring. Am J Hematol 2018;93:442-59. [Crossref] [PubMed]
- Moslehi JJ, Deininger M. Tyrosine Kinase Inhibitor-Associated Cardiovascular Toxicity in Chronic Myeloid Leukemia. J Clin Oncol 2015;33:4210-8. [Crossref] [PubMed]
- Chopade P, Akard L. Improving Outcomes in Chronic Myeloid Leukemia Over Time in the Era of Tyrosine Kinase Inhibitors. Clin Lymphoma Myeloma Leuk 2018;18:710-23. [Crossref] [PubMed]
- Mughal TI, Radich P, Deininger W, et al. Chronic myeloid leukemia: reminiscences and dreams. Haematologica 2016;101:541-58. [Crossref] [PubMed]
- Sato T, Goyama S, Kataoka K, et al. Evi1 defines leukemia-initiating capacity and tyrosine kinase inhibitor resistance in chronic myeloid leukemia. Oncogene 2014;33:5028-38. [Crossref] [PubMed]
- Patel AB, O'Hare T, Deininger W. Mechanisms of Resistance to ABL Kinase Inhibition in Chronic Myeloid Leukemia and the Development of Next Generation ABL Kinase Inhibitors. Hematol Oncol Clin North Am 2017;31:589-612. [Crossref] [PubMed]
- Leti F, Legendre C, Still D, et al. Altered expression of MALAT1 lncRNA in nonalcoholic steatohepatitis fibrosis regulates CXCL5 in hepatic stellate cells. Transl Res 2017;190:25-39.e21. [Crossref] [PubMed]
- Yan B, Wang Z. Long noncoding RNA: its physiological and pathological roles. DNA Cell Biol 2012;31 Suppl 1:S34-41. [Crossref] [PubMed]
- Li Y, Egranov D, Yang L, et al. Molecular mechanisms of long noncoding RNAs-mediated cancer metastasis. Genes Chromosomes Cancer 2019;58:200-7. [Crossref] [PubMed]
- Moran VA, Perera J, Khalil M, et al. Emerging functional and mechanistic paradigms of mammalian long non-coding RNAs. Nucleic Acids Res 2012;40:6391-400. [Crossref] [PubMed]
- Bartonicek N, Maag L, Dinger E, et al. Long noncoding RNAs in cancer: mechanisms of action and technological advancements. Mol Cancer 2016;15:43. [Crossref] [PubMed]
- Heery R, Finn S, Cuffe S, et al. Long Non-Coding RNAs: Key Regulators of Epithelial-Mesenchymal Transition, Tumour Drug Resistance and Cancer Stem Cells. Cancers (Basel) 2017;9:38. [Crossref] [PubMed]
- Özeş AR, Miller D, Ozes N, et al. NF-kappaB-HOTAIR axis links DNA damage response, chemoresistance and cellular senescence in ovarian cancer. Oncogene 2016;35:5350-61. [Crossref] [PubMed]
- Takahashi K, Yan I, Kogure T, et al. Extracellular vesicle-mediated transfer of long non-coding RNA ROR modulates chemosensitivity in human hepatocellular cancer. FEBS Open Bio 2014;4:458-67. [Crossref] [PubMed]
- Bester AC, Lee D, Chavez A, et al. An Integrated Genome-wide CRISPRa Approach to Functionalize lncRNAs in Drug Resistance. Cell 2018;173:649-664.e20. [Crossref] [PubMed]
- Wen F, Cao Y, Luo Z, et al. LncRNA MALAT1 promotes cell proliferation and imatinib resistance by sponging miR-328 in chronic myelogenous leukemia. Biochem Biophys Res Commun 2018;507:1-8. [Crossref] [PubMed]
- Silveira RA, Fachel AA, Moreira YB, et al. Protein-coding genes and long noncoding RNAs are differentially expressed in dasatinib-treated chronic myeloid leukemia patients with resistance to imatinib. Hematology 2014;19:31-41. [Crossref] [PubMed]
- Franceschini A, Szklarczyk D, Frankild S, et al. STRING v9.1: protein-protein interaction networks, with increased coverage and integration. Nucleic Acids Res 2013;41:D808-15. [Crossref] [PubMed]
- Chin C, Chen S, Wu H, et al. cytoHubba: identifying hub objects and sub-networks from complex interactome. BMC Syst Biol 8 Suppl 2014;4:S11.
- Huang da W. Sherman BT, Lempicki RA. Systematic and integrative analysis of large gene lists using DAVID bioinformatics resources. Nat Protoc 2009;4:44-57. [Crossref] [PubMed]
- Huang da W. Sherman BT, Lempicki RA. Bioinformatics enrichment tools: paths toward the comprehensive functional analysis of large gene lists. Nucleic Acids Res 2009;37:1-13. [Crossref] [PubMed]
- Benito-Martin A, Peinado H. FunRich proteomics software analysis, let the fun begin! Proteomics 2015;15:2555-6. [Crossref] [PubMed]
- Tang Z, Li C, Kang B, et al. GEPIA: a web server for cancer and normal gene expression profiling and interactive analyses. Nucleic Acids Res 2017;45:W98-W102. [Crossref] [PubMed]
- Khoury HJ, Cortes J, Baccarani M, et al. Omacetaxine mepesuccinate in patients with advanced chronic myeloid leukemia with resistance or intolerance to tyrosine kinase inhibitors. Leuk Lymphoma 2015;56:120-7. [Crossref] [PubMed]
- Mir BA, Islam R, Kalanon M, et al. MicroRNA suppression of stress-responsive NDRG2 during dexamethasone treatment in skeletal muscle cells. BMC Mol Cell Biol 2019;20:12. [Crossref] [PubMed]
- Lim EL, Trinh DL, Scott DW, et al. Comprehensive miRNA sequence analysis reveals survival differences in diffuse large B-cell lymphoma patients. Genome Biol 2015;16:18. [Crossref] [PubMed]
- Fang Z, Chen W, Yuan Z, et al. LncRNA-MALAT1 contributes to the cisplatin-resistance of lung cancer by upregulating MRP1 and MDR1 via STAT3 activation. Biomed Pharmacother 2018;101:536-42. [Crossref] [PubMed]
- Carter S, Miard S, Boivin L, et al. Loss of Malat1 does not modify age- or diet-induced adipose tissue accretion and insulin resistance in mice. PLoS One 2018;13:e0196603. [Crossref] [PubMed]
- Miard S, Girard M, Joubert P, et al. Absence of Malat1 does not prevent DEN-induced hepatocarcinoma in mice. Oncol Rep 2017;37:2153-60. [Crossref] [PubMed]
- Handa H, Kuroda Y, Kimura K, et al. Long non-coding RNA MALAT1 is an inducible stress response gene associated with extramedullary spread and poor prognosis of multiple myeloma. Br J Haematol 2017;179:449-60. [Crossref] [PubMed]