Prediction of postpartum pelvic floor dysfunction with a nomogram model based on big data collected during pregnancy
Introduction
The incidence of pelvic floor dysfunction (PFD) in the adult female population is 10–58%, and pregnancy and childbirth are the main risk factors for the reduction and damage of pelvic floor function (1,2). Regardless of the mode of delivery, pelvic floor function is impaired to some extent, leading to varying degrees of PFD. PFD easily leads to pelvic organ prolapse, stress urinary incontinence, and chronic pelvic pain. At present, the pelvic floor muscle tension measured at 42 days postpartum is often used clinically to assess the presence of PFD so that rehabilitation can be performed as early as possible to restore pelvic floor function (3). The recovery of pelvic floor function may be easy or difficult depending on the severity of PFD. Therefore, the prediction of PFD and the use of targeted measures to prevent its occurrence are more beneficial than postpartum rehabilitation after PFD occurs. Relevant information has shown that age, parity, history of vaginal birth, body mass index (BMI), constipation, smoking, fetal head circumference, and overweight of the fetus are associated with the occurrence of PFD (4-7). The effects of these factors on PFD occurrence have been elucidated. However, few studies have reported the prediction of postpartum PFD in women. The discriminations of the nomograms established by Jelovsek et al. to predict postpartum PFD were lower due to the lack of big data support for prenatal ultrasound. Artificial intelligence and big data research have undergone a boom in the medical field in recent years, and their high accuracy and efficiency have shown tremendous advantages in medical diagnosis. Currently, the use of artificial intelligence and big data in the diagnosis of cancers such as breast cancer, lung cancer, and thyroid cancer has gradually been recognized, and its accuracy in identifying benign and malignant nodules in lung cancer screening has reached 74.3% (8-10). A nomogram PFD prediction model based on the large amount of accumulated prenatal check-up data was established in this study, with the aim of identifying women at high risk for postpartum PFD through the model and formulating personalized prevention strategies as early as possible to effectively reduce the incidence of PFD. We present the following article in accordance with the TRIPOD reporting checklist (available at http://dx.doi.org/10.21037/apm-21-166).
Methods
Study subjects
All procedures performed in this study involving human participants were in accordance with the Declaration of Helsinki (as revised in 2013). This study was approved by the Ethics Committee of the Zhuji Maternal and Child Health Hospital. Individual consent for this retrospective analysis was waived. The complete data of 1,500 pregnant women who underwent regular prenatal check-ups and examinations for PFD at 42 days postpartum at the Zhuji Maternal and Child Health Hospital between May 2015 and May 2020 were collected. The inclusion criteria were as follows: Women who (I) delivered healthy full-term singletons at the age of 20–40 years and (II) had no abnormalities of liver, kidney, heart, and cognitive functions and no history of pelvic surgery. Data from the following groups were excluded: (I) women with abnormal amniotic fluid volume; (II) women with fetal growth restriction; (III) women with fetal weight >4,000 g; (IV) women with placental retention; and (V) women with prolonged labor or dystocia. All data from the included pregnant women were recorded, including height, weight, smoking history, history of chronic cough, history of diabetes mellitus, history of childbirth, history of constipation, and urinary incontinence during pregnancy.
Fetal growth- and development-related ultrasound examination
A GE Voluson E8 color Doppler ultrasound machine was operated by a sonographer with more than 5 years of work experience. The sonographer measured and recorded the fetal biparietal diameter (BPD), head circumference, femoral diaphysis length (FL) and abdominal circumference (AC) (see Figure 1) at 32 weeks of gestation and estimated the fetal weight using BPD, AC, and FL (weight=1.07*BPD3+0.3*AC2*FL).

Examination of pelvic floor function at 42 days postpartum
Electromyography (EMG) of the pelvic floor was performed at 42 days postpartum using an MCD B2T standard EMG biofeedback device (Nanjing Medlander Medical Technology Co., Ltd., Nanjing, China). Each participant was placed on an examination table in the lithotomy position, and vaginal electrodes were placed in the vagina and on the sacrococcygeal region. According to the voice prompts of the software, the participant performed 60 s of relaxation (pre-baseline), 5 rapid contractions (flick), 5 sustained contractions and relaxations (tonic), 60 s of contraction (endurance), and 60 s of relaxation after exercise (post-baseline). The muscle activity in each phase was measured with EMG and by using a pressure curve, and the relevant EMG parameters were automatically recorded, including the mean value and variability of myoelectric potential and other parameters in the pre-baseline, flick, tonic, endurance, and post-baseline phases. Scores were provided by the EMG biofeedback device for each phase (see Figure S1); scores below 80 points did not meet qualifications, and scores of 80 points or more were considered qualified.
Study protocol
The medical records of 1,500 women were reviewed and used to establish a database. They were randomly divided into a training cohort (n=1,000) and a validation cohort (n=500). According to whether PFD was diagnosed at 42 days postpartum, the patients in each cohort were divided into a PFD group (with poor pelvic floor function) and a non-PFD group (with normal pelvic floor function). First, univariate and multivariate analyses were conducted for the PFD group and the non-PFD group in the training cohort to obtain independent predictors of postpartum PFD at 32 weeks of gestation, and these predictors were used to establish a prediction model. Second, the training cohort data were used for internal validation of the discrimination and calibration of the model. Finally, the verification cohort was used for external validation to further confirm the predictive value of the model (see Figure 2).
Statistical analysis
SPSS 21.0, MedCalc 19, and R software (Windows version 3.6.2) were used for statistical analysis. Measurement data are expressed as the mean ± standard deviation, and count data are expressed as the number of cases (percentage). The t-test, chi-square test or Fisher’s exact test was used for between-group comparisons. Multivariate logistic regression analysis was performed on the variables with significant differences in the univariate analysis. The performance of the nomogram was internally and externally verified. Discrimination was quantitatively analyzed using area under the receiver operating characteristic (ROC) curve (AUC), and calibration was assessed using the Hosmer–Lemeshow test.
Results
Clinical data used for training and validation
In the training cohort, 389 women who were diagnosed with PFD at 42 days postpartum were included in the PFD group (the incidence rate of PFD was 38.9%), and the remaining 611 women without PFD were included in the non-PFD group. In the validation cohort, 196 women were included in the PFD group (the incidence rate of PFD was 39.2%), and 304 women were included in the non-PFD group. Table 1 shows the clinical data of the women in the training and validation cohorts. The basic clinical data of the training and validation cohorts were not significantly different (all P > 0.05; Table 1).
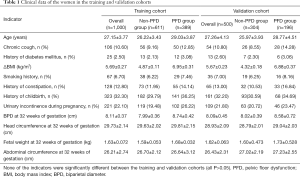
Full table
Univariate and multivariate analysis of factors associated with postpartum PFD
In the training cohort, maternal age, history of chronic cough, diabetes mellitus, history of constipation, history of childbirth, urinary incontinence during pregnancy, fetal BPD at 32 weeks of gestation, and fetal weight at 32 weeks of gestation were greater in the PFD group than in the non-PFD group. Further multivariate analysis showed that age [odds ratio (OR) =1.896, P<0.001], history of childbirth (OR =4.531, P<0.001), history of constipation (OR =3.475, P<0.001), urinary incontinence during pregnancy (OR =6.416, P<0.01), and BPD at 32 weeks of gestation (OR =51.672, P=0.012) were independent predictors of PFD at 42 days postpartum (Table 2).
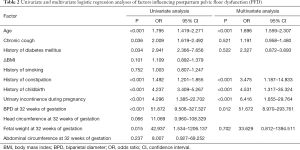
Full table
Establishment of a postpartum PFD risk prediction model
Based on the results in Table 2, 5 variables, including age, history of childbirth, history of constipation, urinary incontinence during pregnancy, and BPD at 32 weeks of gestation were eventually included in the nomogram prediction model. Each predictor variable is assigned a specific number on a scale from 0 to 100 points. By calculating the total score and positioning it on the total score scale, the incidence of PFD at 42 days postpartum can be predicted (Figure 3).
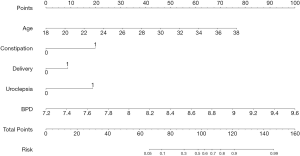
Validation of the postpartum PFD risk prediction model
The AUC of the nomogram based on the training cohort was 0.893 [95% confidence interval (CI): 0.814‒0.991], showing good discrimination (>0.75) (Figure 4A). The calibration test showed no significant deviation (χ2=9.028, P=0.340, Hosmer-Lemeshow test), indicating that the predicted incidence of PFD at 42 days postpartum was well correlated with the actual observation. To validate the effectiveness of the nomogram, an external validation cohort consisting of the data of 500 women, 39.2% of whom had PFD, was used. Analysis of the nomogram predictions showed that the AUC generated by the external validation data was 0.842 (95% CI: 0.793–0.857), which proved that the nomogram also had good discrimination ability in the validation cohort (Figure 4B).
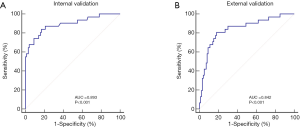
Discussion
Big data collected at prenatal check-ups were analyzed in this study. The results show that age, history of childbirth, history of constipation, urinary incontinence during pregnancy, and BPD at 32 weeks of gestation were independent factors influencing postpartum PFD. A postpartum PFD prediction model based on these factors can accurately predict the incidence of PFD at 42 days postpartum, allowing individualized preventive measures to be formulated for women at high risk for postpartum PFD to prevent or reduce the severity of PFD.
PFD can adversely affect women’s daily life, sexual life, and quality of life. The pathogenesis of PFD remains unclear. Risk factors for PFD include pregnancy, childbirth, age, and estrogen levels, among which pregnancy and childbirth are the most important factors (11-15). Rehabilitation after the occurrence of PFD often does not achieve the most satisfactory effect, and appropriate guidance and close follow-up are indispensable for achieving effective therapy (16,17). Therefore, it is necessary to identify pregnant women with a high risk of PFD in advance and formulate personalized preventive measures to prevent PFD. Studies have shown that pelvic floor muscle training before 32 weeks of gestation can effectively prevent the occurrence of PFD (18), and the 32nd gestational week is one of the time points for routine prenatal check-ups in China. Therefore, we chose to measure and collect various indicators at 32 weeks of pregnancy.
Age, history of childbirth, history of constipation, urinary incontinence during pregnancy, and BPD at 32 weeks of gestation are common factors that influence postpartum PFD. Our study showed that the older a pregnant woman is, the greater her probability of developing postpartum PFD. This finding is similar to that of Chen et al. (19) and may be explained by the decrease in the ratio of muscle fiber to connective tissue and in muscle strength with increasing age. A history of childbirth is a recognized risk factor for PFD (20). This is because childbirth inevitably compromises pelvic floor function, regardless of the mode of delivery, due to the continuous pulling of the pelvic floor tissue by the gravity of the uterus during pregnancy, the effects of various hormones on connective tissue metabolism, and the backward posture of pregnant women while standing. A second childbirth is more likely to cause some degree of PFD. Constipation is another recognized factor that easily leads to postpartum PFD. Long-term constipation can lead to dry and hard stools, and abdominal force during defecation generates repeated and persistent pressure on the pelvic floor muscles, resulting in an increased risk of PFD (21). Urinary incontinence during pregnancy is the biggest factor affecting PFD at 42 days postpartum compared with the history of childbirth and constipation. The risk of developing PFD at 42 days postpartum is 4.416 times higher among women with urinary incontinence during pregnancy than among women without urinary incontinence during pregnancy. Bø et al. and Barbosa et al. reported that the pelvic floor muscle strength of pregnant women with urinary incontinence during pregnancy was higher than that of pregnant women with urinary incontinence during pregnancy, suggesting that pelvic floor muscle strength is associated with the mechanism of urinary continence, which is similar to the conclusion of this study (22,23). Fetal BPD is clearly an important factor influencing PFD. The greater the fetal BPD, the greater the pressure on the pelvic floor muscles and the more significant the decrease in muscle tension. When the fetal BPD is too large, it can damage or even destroy the pelvic floor muscles or ligaments and reduce the blood supply to the pelvic floor muscles, thereby leading to PFD (24). It is worth noting that the multivariate analysis in this study showed that fetal weight at 32 weeks of gestation was not an independent factor influencing the occurrence of PFD. The possible reason is that besides BPD, the estimation of fetal weight is affected by HL and AC as well, leading to unobvious changes.
In this study, the nomogram prediction model established using these factors maintained good discrimination in the training cohort and the external validation cohort. As a statistical graphic prediction tool, the nomogram can transform the complex regression equation into a visual graph, making the results of the prediction model more readable; additionally, the nomogram is intuitive and easy to understand. It has higher accuracy than traditional staging criteria in the prediction of disease incidence and survival prognosis (25). The nomogram scores the impact of each influencing factor based on the contribution of each influencing factor to the dependent variable in the regression model and then calculates a total score that provides an individualized prediction. Since all predictive indicators can be obtained during prenatal check-ups, the method proposed in this study is highly applicable in the clinical environment. In the future, clinicians can input the relevant indicators at 32 weeks of gestation into a prediction model to assess the risk of postpartum PFD, and the results can be used as a reference to determine whether preventive measures should be taken in advance or to assess the effectiveness of pelvic floor muscle training during pregnancy.
The model parameters in this study are easy to obtain and use in clinical practice. The established prediction model has high accuracy and goodness of fit and can theoretically be used to provide clinical guidance. However, this study has certain limitations. First, this study is a single-center, retrospective study. Inherent selection bias cannot be avoided. For example, certain indicators, such as the history of constipation, may lose their predictive value due to recall bias or unclear definitions. Second, some variables that might affect the occurrence of PFD were not included in the prediction model. Therefore, it is necessary to further optimize the prediction model through multicenter and large-sample data in future studies.
In summary, the risk of PFD was quantified in this study using a nomogram prediction model based on the big data of prenatal ultrasound at 32 weeks of pregnancy. It permits preventive and early treatment for pregnant women at high risk for postpartum PFD, which is more beneficial than postpartum rehabilitation after PFD occurs.
Acknowledgments
Funding: None.
Footnote
Reporting Checklist: The authors have completed the TRIPOD reporting checklist. Available at http://dx.doi.org/10.21037/apm-21-166
Data Sharing Statement: Available at http://dx.doi.org/10.21037/apm-21-166
Conflicts of Interest: All authors have completed the ICMJE uniform disclosure form (available at http://dx.doi.org/10.21037/apm-21-166). The authors have no conflicts of interest to declare.
Ethical Statement: The authors are accountable for all aspects of the work in ensuring that questions related to the accuracy or integrity of any part of the work are appropriately investigated and resolved. All procedures performed in this study involving human participants were in accordance with the Declaration of Helsinki (as revised in 2013). This study was approved by the Ethics Committee of the Zhuji Maternal and Child Health Hospital. Individual consent for this retrospective analysis was waived.
Open Access Statement: This is an Open Access article distributed in accordance with the Creative Commons Attribution-NonCommercial-NoDerivs 4.0 International License (CC BY-NC-ND 4.0), which permits the non-commercial replication and distribution of the article with the strict proviso that no changes or edits are made and the original work is properly cited (including links to both the formal publication through the relevant DOI and the license). See: https://creativecommons.org/licenses/by-nc-nd/4.0/.
References
- Smith CA, Witherow RO. The assessment of female pelvic floor dysfunction. BJU Int 2000;85:579-87. [Crossref] [PubMed]
- Bozkurt M, Yumru AEE, Sahin L. Pelvic floor dysfunction, and effects of pregnancy and mode of delivery on pelvic floor. Taiwan J Obstet Gynecol 2014;53:452-8. [Crossref] [PubMed]
- Wang QJ, Zhao YJ, Huang LX, et al. Evaluation of the effect of electrical stimulation combined with biofeedback therapy for postpartum pelvic organ prolapse: a static and dynamic magnetic resonance imaging study. Zhonghua Yi Xue Za Zhi 2019;99:375-9. [PubMed]
- Sartore A, Pregazzi R, Bortoli P, et al. Assessment of pelvic floor muscle function after vaginal delivery. Clinical value of different tests. J Reprod Med 2003;48:171-4. [PubMed]
- Hilde G, Stær-Jensen J, Siafarikas F, et al. Postpartum pelvic floor muscle training and urinary incontinence: a randomized controlled trial. Obstet Gynecol 2013;122:1231-8. [Crossref] [PubMed]
- Serati M, Salvatore S, Khullar V, et al. Prospective study to assess risk factors for pelvic floor dysfunction after delivery. Acta Obstet Gynecol Scand 2008;87:313-8. [Crossref] [PubMed]
- Durnea CM, Khashan AS, Kenny LC, et al. What is to blame for postnatal pelvic floor dysfunction in primiparous women-Pre-pregnancy or intrapartum risk factors? Eur J Obstet Gynecol Reprod Biol 2017;214:36-43. [Crossref] [PubMed]
- Bi WL, Hosny A, Schabath MB, et al. Artificial intelligence in cancer imaging: Clinical challenges and applications. CA Cancer J Clin 2019;69:127-57. [Crossref] [PubMed]
- Liu X, Zhou H, Hu Z, et al. Clinical Application of Artificial Intelligence Recognition Technology in the Diagnosis of Stage T1 Lung Cancer. Zhongguo Fei Ai Za Zhi 2019;22:319-23. [PubMed]
- Moon JH, Steinhubl SR. Digital Medicine in Thyroidology: A New Era of Managing Thyroid Disease. Endocrinol Metab (Seoul) 2019;34:124-31. [Crossref] [PubMed]
- Kahyaoglu Sut H, Balkanli Kaplan P. Effect of pelvic floor muscle exercise on pelvic floor muscle activity and voiding functions during pregnancy and the postpartum period. Neurourol Urodyn 2016;35:417-22. [Crossref] [PubMed]
- Sobhgol SS, Priddis H, Smith CA, et al. The Effect of Pelvic Floor Muscle Exercise on Female Sexual Function During Pregnancy and Postpartum: A Systematic Review. Sex Med Rev 2019;7:13-28. [Crossref] [PubMed]
- Kolberg Tennfjord M, Hilde G, Staer-Jensen J, et al. Effect of postpartum pelvic floor muscle training on vaginal symptoms and sexual dysfunction-secondary analysis of a randomised trial. BJOG 2016;123:634-42. [Crossref] [PubMed]
- Van Geelen H, Ostergard D, Sand P. A review of the impact of pregnancy and childbirth on pelvic floor function as assessed by objective measurement techniques. Int Urogynecol J 2018;29:327-38. [Crossref] [PubMed]
- Sangsawang B, Sangsawang N. Stress urinary incontinence in pregnant women: a review of prevalence, pathophysiology, and treatment. Int Urogynecol J 2013;24:901-12. [Crossref] [PubMed]
- Alshiek J, Jalalizadeh M, Wei Q, et al. Ultrasongraphic age-related changes of the pelvic floor muscles in nulliparous women and their association with pelvic floor symptoms: A pilot study. Neurourol Urodyn 2019;38:1305-12. [Crossref] [PubMed]
- Mehta N, Pandit A. Concurrence of big data analytics and healthcare: A systematic review. Int J Med Inform 2018;114:57-65. [Crossref] [PubMed]
- Leon-Larios F, Corrales-Gutierrez I, Casado-Mejía R, et al. Influence of a pelvic floor training programme to prevent perineal trauma: A quasi-randomised controlled trial. Midwifery 2017;50:72-7. [Crossref] [PubMed]
- Chen GD. Pelvic floor dysfunction in aging women. Taiwan J Obstet Gynecol 2007;46:374-8. [Crossref] [PubMed]
- Van Geelen H, Ostergard D, Sand P. A review of the impact of pregnancy and childbirth on pelvic floor function as assessed by objective measurement techniques. Int Urogynecol J 2018;29:327-38. [Crossref] [PubMed]
- Amselem C, Puigdollers A, Azpiroz F, et al. Constipation: a potential cause of pelvic floor damage? Neurogastroenterol Motil 2010;22:150-3. [Crossref] [PubMed]
- Bø K. Pelvic floor muscle training in treatment of female stress urinary incontinence, pelvic organ prolapse and sexual dysfunction. World J Urol 2012;30:437-43. [Crossref] [PubMed]
- Barbosa A M, Marini G, Piculo F, et al. Prevalence of urinary incontinence and pelvic floor muscle dysfunction in primiparae two years after cesarean section: cross-sectional study. Sao Paulo Med J 2013;131:95-9. [Crossref] [PubMed]
- Gao L, Ding S, Ding Y, et al. Symptom distribution of female pelvic floor dysfunction patients with constipation as chief complaint. Zhonghua Wei Chang Wai Ke Za Zhi 2018;21:798-802. [PubMed]
- Hu WP, Lhamo T, Liu D, et al. Development of a Nomogram to Predict the Risk of 30-Day Re-Exacerbation for Patients Hospitalized for Acute Exacerbation of Chronic Obstructive Pulmonary Disease. COPD 2019;16:160-7. [Crossref] [PubMed]