A noninvasive diagnostic model for significant liver fibrosis in patients with chronic hepatitis B based on CHI3L1 and routine clinical indicators
Introduction
Chronic hepatitis B (CHB) is one of the most common chronic liver diseases worldwide (1). Liver fibrosis is a serious consequence of CHB, and interrupting the development of liver fibrosis is an important goal of antiviral therapy for CHB patients. Timely diagnosis and dynamic assessment of liver fibrosis can effectively prevent the progression of CHB into cirrhosis or hepatocellular carcinoma (HCC) (2,3). Although liver biopsy remains the gold standard for assessing the degree of liver fibrosis, its clinical application is still limited due to its invasiveness, inconvenience, risk of complications, and sampling errors. In addition, it is not suitable for the dynamic monitoring of liver fibrosis changes (4). Therefore, a series of noninvasive indicators (e.g., serological markers or imaging findings) have been developed, but to date there is still no single indicator that can sensitively and accurately reflect the degree of liver fibrosis. In recent years, many noninvasive liver fibrosis models have been developed to partially replace liver biopsy. Among these, the aspartate transaminase to platelet ratio index (APRI) (5) and the fibrosis 4 score (FIB-4) (6) are two widely applied indicators. Both were recommended as indicators for the noninvasive diagnosis of liver fibrosis in the 2015 World Health Organization (WHO) guidelines for the prevention and treatment of hepatitis B (7) and in the Chinese guidelines for the prevention and treatment of CHB (3). However, these 2 indicators were originally established based on data from patients with chronic hepatitis C virus (HCV) infection, and their applicability to the assessment of liver fibrosis in CHB patients remains to be further validated in clinical studies (3,8,9). Serum chitinase 3-like 1 (CHI3L1) is a potential marker of liver fibrosis that has been identified in recent years. Several previous studies have shown that CHI3L1 level in CHB patients is significantly correlated with the stage of fibrosis (10,11). CHI3L1 was included in the Guidelines for the Treatment of Cirrhotic Hepatic Encephalopathy (12) and the Guidelines for the Prevention and Treatment of Chronic Hepatitis B (3) in 2018 and 2019, respectively, as a useful marker for evaluating the degree of cirrhosis and liver fibrosis. However, few studies have explored the roles of the combinations of CHI3L1 with other routine clinical indicators in the diagnosis of liver fibrosis in patients with CHB. Therefore, this study analyzed the independent predictors associated with liver fibrosis based on serum CHI3L1 levels and other routine clinical indicators in patients with CHB, developed and validated a noninvasive diagnostic model to indirectly determine the degree of liver fibrosis.
We present the following article in accordance with the TRIPOD reporting checklist (available at http://dx.doi.org/10.21037/apm-21-957).
Methods
Subjects
A total of 337 CHB patients, including 239 males and 98 females with ages ranging from 19 to 61 years (mean 36.3±7.9 years) who were treated at Xiamen Hospital of Traditional Chinese Medicine from December 1, 2019 to September 30, 2020, were finally included in this cross-sectional study. The diagnosis of CHB was in accordance with the diagnostic criteria in the Chinese Medical Association’s Guidelines for the Prevention and Treatment of Chronic Hepatitis B (2019 edition) (3). The exclusion criteria were as following: (I) with coinfection of hepatitis C/D virus or HIV, and/or chronic liver disease caused by other causes (including autoimmune liver disease, inherited metabolic disease of the liver, and drug-induced liver damage); (II) with decompensated cirrhosis or hepatocellular carcinoma; (III) with severe cardiac, cerebral, renal diseases or hematological disorders; (IV) excessive alcohol use (>20 g/d for women and >30 g/d for men); (V) undergoing antiviral therapy; and (VI) with unclear serological indicators.
The whole cohort was randomly divided into a training cohort (n=270) and a validation cohort (n=67) by using a random number table at a ratio of 4:1. The training cohort was further divided into a non-significant liver fibrosis (NSLF) group (stages S0–S1; n=189; used as the control group) and an SLF group (stage S2–S4; n=81; used as the outcome group) based on the Scheuer scoring system. The study was approved by the ethics committee of Xiamen Hospital of Traditional Chinese Medicine (No. 2020-K041-01). All procedures performed in this study involving human participants were in accordance with the Declaration of Helsinki (as revised in 2013). Individual consent for this retrospective analysis was waived.
Study methods
The Transparent Reporting of a multivariable prediction model for Individual Prognosis Or Diagnosis (TRIPOD) statement (13) was used as the reporting guide for our study. Data collected included (I) general information, (II) liver biopsy data, (III) hematological markers, and (IV) live stiffness measurements.
- General information entailed patients’ clinical data, including gender, age, past histories, family history of cirrhosis or HCC, and history of medications that might have damaged the liver were collected.
- All patients underwent liver biopsy, and the pathologic diagnoses of liver tissue inflammation and fibrosis were based on the Scheuer scoring system. Liver tissue specimens were >1.0 cm in length, and the number of confluent areas was >5. The tissue specimens were fixed in 10% buffered neutral formalin and processed for paraffin sectioning before reticulin staining and Masson trichrome staining. Staging of liver fibrosis (S0–S4) was carried out according to the Scheuer classification by one pathologist blinded to the clinical characteristics of all study subjects and confirmed by another pathologist independently. S0 and S1 referred to no significant liver fibrosis (NSLF); ≥S2 was considered to significant liver fibrosis (SLF), with the presence of septa or bridging fibrosis; and S4 indicated cirrhosis.
- Eleven hematological markers were used based on hepatitis B virus (HBV) infection status, liver function, and liver fibrosis-related indicators reported in CHB patients, including hepatitis B surface antigen (HBsAg), hepatitis B e antigen (HBeAg), HBV deoxyribonucleic acid (HBV DNA), alpha-fetoprotein (AFP), CHI3L1, international normalized ratio (INR), platelets (PLT), albumin (ALB), alanine amino transferase (ALT), aspartate amino transferase (AST), and total bilirubin (TBIL). Among them, AFP, HBsAg, and HBeAg were detected using Abbott i2000 fully automated chemiluminescent immunoassay analyzer and accompanying reagents (Abbott Ireland Diagnostic Division, Finisklin Business Park, Sligo, Ireland). HBV DNA was determined using a Roche COBAS Ampli Prep/COBAS TaqMan system (Roche Molecular Systems, Inc., Branchburg, NJ, USA). CHI3L1 was measured by ELISA kit (Hangzhou Proprium Biotech Company Limited, Hangzhou, China), and its optical density value was measured using a BIO-RAD iMark microplate absorbance reader (Bio-Rad Laboratories Inc, Benicia, CA, USA). Serum liver function indicators including ALB, ALT, AST, and TBIL were measured using an Abbott c16000 fully automated biochemical analyzer (Abbott GmbH&Co.KG, Wiesbaden, Germany). PLT was measured using the XN-1000 Sysmex hematology analyzer (Sysmex Corporation, Kobe, Japan). INR was measured using the STR-R Evolution fully automated hemagglutination analyzer (Diagnostica Stago, Taverny, France). Blood samples were collected within 2 weeks before and after liver biopsy, and all the hematological markers were measured in our clinical laboratory.
- Liver stiffness measurement (LSM) was performed with vibration-controlled transient elastography (VCTE) using FibroScan (Echosens, Paris, France). The examinations were performed in the areas between the seventh, eighth, and ninth rib from the right anterior axillary line to the midaxillary line. After 10 successful examinations were performed, the median was used as the final examination result, which was expressed as elasticity (kPa). The success rate was required to be no lower than 60%, with a deviation value greater than one-third of the median.
Statistical analysis
All statistical analyses were performed using MedCalc 19.0.7 software (MedCalc, Mariakerke, Belgium). Normally distributed data are expressed as mean ± standard deviation; independent samples t-test was used for comparisons between 2 groups, and analysis of variance (ANOVA) was used for comparisons of means among multiple groups. Data that were nonnormally distributed or that had uneven variances are shown as median [quartile spacing; M (P25, P75)]. The Mann-Whitney U test was used for comparisons between the 2 groups, the Kruskal-Wallis rank-sum test was used for comparisons among multiple groups, and Dunn’s test for multiple comparisons among multiple groups. Categorical variables were expressed as numbers and percentages, and were compared using the chi-square test. The Jonckheere-Terpstra trend test was used to analyze trends of the new indicator with the change of Scheuer scores. Correlation analysis was performed using the Spearman correlation method. With the NSLF cases as the control group, 10 previously reported or widely recognized liver fibrosis-associated indicators including age, family history of cirrhosis or HCC, CHI3L1, AFP, ALB, ALT, AST, TBIL, PLT, and INR were subjected to univariate logistic regression analysis, and those variables with a P value <0.1 were included in the multivariate logistic regression analysis to screen out independent predictors of SLF in CHB patients and establish a diagnostic model. The diagnostic performance of the model was evaluated in terms of area under the receiver operating characteristic curve (AUROC), cutoff value, sensitivity, and specificity, and the differences in diagnostic performance between the new diagnostic model and the 4 noninvasive diagnostic indicators (including APRI, FIB-4, CHI3L1, and FibroScan LSM values) of liver fibrosis recommended by the guidelines (3) were compared. The DeLong test (14) was used to compared the difference of AUROC. A value of P<0.05 was considered statistically significant.
Results
General information
During December 2019 to September 2020, a total of 537 CHB patients who had received a liver biopsy were enrolled in this study. Overall, 173 patients were excluded base on exclusion criteria, 27 patients were excluded due to insufficient data; 337 patients were included as the eventual participants (Figure 1). Clinical characteristics of age, gender, family history of cirrhosis or HCC, histological features of the liver, HBeAg status, and relevant markers of patients in both training and validation cohorts are shown in Table 1. Except that AFP was significantly higher in the training cohort than in the validation cohort (P<0.05), there were no statistically significant differences in all other variables between the two cohorts (P>0.05).
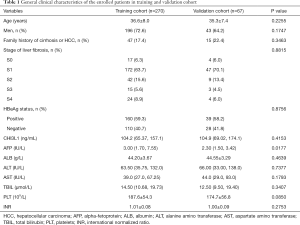
Full table
Establishment of a new model for noninvasive diagnosis of liver fibrosis
The cases enrolled in the training cohort were divided into two groups based on the Scheuer scoring system: a NSLF group (S0–S1, n=189) and an SLF group (S2–S4, n=81). Eight variables that were statistically significant for SLF were identified by univariate logistic regression analysis, which included age, CHI3L1, AFP, ALB, AST, TBIL, PLT, and INR. To exclude confounding variables, the above variables were further subjected to multivariate binary logistic regression analysis (selection criteria: P<0.1), and CHI3L1, AFP, and PLT were found to be independent predictors of SLF (all P<0.05; Table 2). These 3 indicators were included in the following equation to finally establish a new index for diagnosing SLF denoted by CAP, assembled by the first letter of each variable:
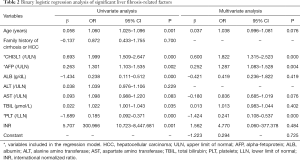
Full table
CHI3L1/AFP/PLT (CAP) = 0.600 × CHI3L1 [upper limit of normal (ULN)] + 0.252 × AFP (/ULN) – 1.424 × PLT [lower limit of normal (LLN)] – 1.223
Diagnostic efficacy of the novel index CAP for SLF
The AUROC of the new index CAP for the diagnosis of SLF in the training cohort and the validation cohort was 0.805 and 0.819, respectively (Figure 2, Table 3), showing no statistically significant difference (P=0.843). The sensitivity, specificity, and Youden index of CAP in the training and validation cohorts for the diagnosis of SLF are shown in Table 3, according to the diagnostic cutoff value of –1.90 at the maximum Youden index in the training cohort.
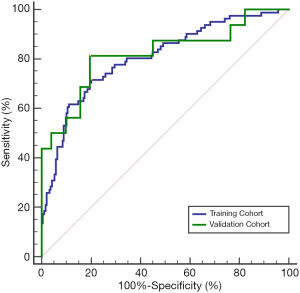

Full table
CAP in different liver fibrosis stages
CAP was further applied to the staging of liver fibrosis. The CAP values in different liver fibrosis stages (S0, S1, S2, S3, and S4) in the whole cohort are shown in Table 4 and Figure 3. The Jonckheere-Terpstra trend test revealed that the CAP value tended to increase gradually with the severity of liver fibrosis (Ptrend<0.001) and had a good correlation (Spearman correlation coefficient: 0.486). Dunn’s test for paired comparisons showed CAP had good performance in distinguishing S2–S4 from S0–S1; however, the difference in CAP among S2, S3, and S4 was not statistically significant (P>0.05) (Table 4, Figure 3).
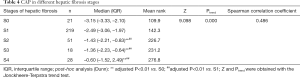
Full table
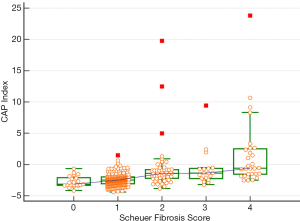
Comparisons of CAP with APRI, FIB-4, CHI3L1, and FibroScan LSM
In the whole cohort, the AUROC, sensitivity, and specificity of CAP for the diagnosis of SLF (S2–S4) were significantly higher than those of the 2 noninvasive indices, APRI and FIB-4, and CHI3L1 for the diagnosis of SLF (all P<0.05, Table 5). However, no significant difference was found when compared with those of FibroScan LSM [E(kPa); P=0.758; Table 5].
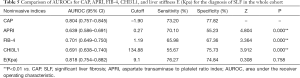
Full table
Diagnostic efficacy of CAP in different subgroups
The efficacy of CAP in diagnosing SLF (S2–S4) was also analyzed in subgroups with different ALT levels and HBeAg statuses. It was found that the AUROCs of CAP in diagnosing SLF were not significantly different among the 3 ALT subgroups [≤ULN, (1–2) × ULN, and >2×ULN] and between the HBeAg-positive and HBeAg-negative subgroups (Tables 6,7).

Full table

Full table
Discussion
Liver fibrosis is caused by the excessive accumulation of extracellular matrix (ECM) due to a variety of reasons (15), with the activation of hepatic stellate cells (HSC) being the central link (16). Assessment of the degree of liver fibrosis in CHB patients is useful for early detection of liver fibrosis and cirrhosis and is valuable for treatment planning and prognosis prediction (17). In our current study, we found that 3 indicators including CHI3L1, AFP, and PLT were independent predictors of SLF in CHB patients. In previous studies, all 3 of these markers were shown to be associated with SLF. CHI3L1, a member of the mammalian chitinase family, has a variety of biological activities; in particular, it is involved in inflammatory responses, cell migration, fibrosis, and tissue remodeling (18). Recent studies have shown that intrahepatic CHI3L1, which is mainly derived from hepatic macrophages and HSC, can activate HSC and promote the differentiation of hematopoietic stem cells into myofibroblasts, which secrete large amounts of ECM proteins (including type I and III collagen), leading to the formation of hepatic fibrosis (10). AFP is an important marker for the diagnosis of primary HCC; however, several recent studies have also found an association between AFP and liver fibrosis (19,20). Feng et al. developed a novel index using AFP and activated partial thromboplastin time (APTT) to predict SLF in CHB patients who were HBeAg-negative and had alanine aminotransferase <2× ULN, with higher diagnostic efficacy than those of several other noninvasive diagnostic indices (21). Thrombocytopenia is a common complication of chronic liver disease. In CHB patients, increased blockage and destruction of PLT by an enlarged spleen, depressed thrombopoietin levels in hepatocytes due to progression of liver fibrosis, and bone marrow suppression result in a reduced rate of PLT production (22).
Several noninvasive diagnostic indicators containing routine serum markers have been used for clinical evaluation of liver fibrosis in patients with chronic liver disease. Among them, APRI and FIB-4 have been widely accepted as indicators of liver fibrosis in CHB patients in guidelines for the prevention and treatment of CHB (3,8). The AUROC of the newly established CAP index in our current study was significantly higher than those of APRI and FIB-4 for diagnosing SLF. A possible explanation is that APRI and FIB-4 were originally developed for assessing the degree of Chronic hepatitis C (CHC)-related liver fibrosis based on data from CHC patients (5,6). HBV and HCV infections have very different effects on liver fibrosis progression and relevant markers (23). Our study included only CHB patients, and the diagnostic model developed for assessing the degree of liver fibrosis was more tailored to CHB patients. We also compared the diagnostic efficacy between the new index CAP and the liver stiffness measured by FibroScan and found CAP had a comparable AUROC with LSMs for diagnosing SLF. FibroScan, which was developed on the basis of transient elastography, has the advantages of being convenient, fast, and noninvasive. In recent years it has been increasingly favored as a useful tool for assessing liver fibrosis by measuring liver stiffness. However, the results of FibroScan tests can be easily affected by many factors, such as obesity, ascites, ALT, AST, and bilirubin. Furthermore, the FibroScan system is too expensive for most of the grassroots hospitals in China. Among the variables included in the newly developed CAP index, CHI3L1 can be obtained by a simple enzyme-linked immunosorbent assay (ELISA) test, and both AFP and PLT are routine laboratory tests for patients with CHB, which are easily available at all levels of medical institutions. Therefore, CAP can be used as a noninvasive diagnostic index in grassroots hospitals as an alternative to FibroScan LSM for the diagnosis of SLF.
By comparing the differences in CAP levels among different liver fibrosis stages, we further found that CAP could better identify SLF and that its level showed a progressive increase with the severity of liver fibrosis. However, no statistical difference in CAP was found among S2, S3, and S4 stages. Therefore, CAP is more suitable for the diagnosis of SLF in CHB patients and has a limited role in distinguishing the specific stages of liver fibrosis in CHB patients who have already developed SLF. However, this may be related to the relatively small number of SLF cases in the present study, and therefore multicenter studies with larger sample sizes are warranted to further validate the diagnostic performance of CAP.
The natural course of CHB is usually divided into 4 different phases: immune tolerance, immune clearance, inactive carrier, and reactivation (3). For HBeAg-positive patients in the immune clearance phase and for HBeAg-negative patients in the reactivation phase, charactered by high viral load (HBV DNA ≥2,000 IU/mL) and persistently abnormal ALT (> ULN), most guidelines recommend that antiviral therapy should be initiated directly, which has been widely recognized both in China and other countries (3,24-27). However, the treatment and management of those patients with low HBV DNA levels (≤2,000 IU/mL) and persistently normal ALT remains highly controversial (25-27). Some of these patients are still in the immune tolerance phase and do not require immediate antiviral therapy. However, another group of those patients has actually entered the immune clearance phase, with significant liver inflammation or fibrosis on liver histology; however, their serum ALT levels can still be normal, making it difficult to distinguish them from those in the immune tolerance phase, which often causes delays in diagnosis and leads to insidious progression to cirrhosis or even liver cancer (28). In the present study, we found no statistically significant differences in the AUROCs of CAP index in diagnosing SLF among CHB patients with different ALT levels and between HBeAg-negative and HBeAg-positive CHB patients, suggesting the diagnostic efficacy of CAP for SLF was not affected by ALT level and HBeAg status. Thus, CAP is applicable to patients in different stages of CHB and helps to determine the timing of antiviral therapy in CHB patients.
Despite these findings, our study had certain limitations. First, the small sample size of patients with SLF among the enrolled cases might have influenced the actual diagnostic efficacy of the model. Second, model validation was performed in another randomly selected group of cases at the same center where the training cohort was enrolled, meaning the study lacked data from multicenter external validation. Third, we developed the model by using clinical indicators obtained from a single time point, and no follow-up data were obtained from the enrolled cases. Therefore, before the clinical application of CAP, we will further follow up the enrolled cases at different time points and further validate the model in multicenter studies with large sample sizes.
In conclusion, we developed a noninvasive model CAP to diagnose liver fibrosis in CHB patients. This newly established index has better diagnostic efficacy for SLF than do other noninvasive indicators, including APRI, FIB-4, and CHI3L1, and is clinically valuable in the noninvasive diagnosis of liver fibrosis in CHB patients. In addition to routine biomarkers, some emerging new candidates including the oxidative stress-mediated biomarkers, epigenetic and genetic markers, exosomes, miRNAs could serve as diagnostic biomarkers for hepatic fibrosis. In the following study, further extensive evaluations are required to circumscribe the predictive value of these biomarkers and to establish a model by combining liver-specific biomarkers for early diagnosis and staging of liver fibrosis with better sensitivity and specificity, and to stratify patients for possible therapeutic interventions (29).
Acknowledgments
Funding: Supported by Youth Project of Fujian Provincial Health Commission (2018-2-68).
Footnote
Reporting Checklist: The authors have completed the TRIPOD reporting checklist Available at http://dx.doi.org/10.21037/apm-21-957
Data Sharing Statement: Available at http://dx.doi.org/10.21037/apm-21-957
Conflicts of Interest: All authors have completed the ICMJE uniform disclosure form (available at http://dx.doi.org/10.21037/apm-21-957). The authors have no conflicts of interest to declare.
Ethical Statement: The authors are accountable for all aspects of the work in ensuring that questions related to the accuracy or integrity of any part of the work are appropriately investigated and resolved. The study was approved by the ethics committee of Xiamen Hospital of Traditional Chinese Medicine (No. 2020-K041-01). All procedures performed in this study involving human participants were in accordance with the Declaration of Helsinki (as revised in 2013). Individual consent for this retrospective analysis was waived.
Open Access Statement: This is an Open Access article distributed in accordance with the Creative Commons Attribution-NonCommercial-NoDerivs 4.0 International License (CC BY-NC-ND 4.0), which permits the non-commercial replication and distribution of the article with the strict proviso that no changes or edits are made and the original work is properly cited (including links to both the formal publication through the relevant DOI and the license). See: https://creativecommons.org/licenses/by-nc-nd/4.0/.
References
- Trepo C, Chan HL, Lok A. Hepatitis B virus infection. Lancet 2014;384:2053-63. [Crossref] [PubMed]
- Abo El-Khair SM, El-Alfy HA, Elsamanoudy AZ, et al. Development of a novel glycated protein-based fibrosis prediction score for determination of significant liver fibrosis in HCV-infected patients, a preliminary study. J Med Virol 2020; Epub ahead of print. [Crossref] [PubMed]
- Chinese Society of Infectious Diseases, Chinese Medical Association. Chinese Society of Hepatology, Chinese Medical Association. The guidelines of prevention and treatment for chronic hepatitis B (2019 version). Zhonghua Gan Zang Bing Za Zhi 2019;27:938-61.
- Yang F, Liu Y, Zeng B, et al. Noninvasive assessment of liver fibrosis for predicting acute-on-chronic liver failure in patients with chronic hepatitis B. Hepatology International 2021; Epub ahead of print. [Crossref] [PubMed]
- Wai CT. A simple noninvasive index can predict both significant fibrosis and cirrhosis in patients with chronic hepatitis C. Hepatology 2003;38:518-26. [Crossref] [PubMed]
- Sterling RK, Lissen E, Clumeck N, et al. Development of a simple noninvasive index to predict significant fibrosis in patients with HIV/HCV coinfection. Hepatology 2006;43:1317-25. [Crossref] [PubMed]
- WHO Guidelines Approved by the Guidelines Review Committee. Guidelines for the Prevention, Care and Treatment of Persons with Chronic Hepatitis B Infection. 2015.
- Kim WR, Berg T, Asselah T, et al. Evaluation of APRI and FIB-4 scoring systems for non-invasive assessment of hepatic fibrosis in chronic hepatitis B patients. J Hepatol 2016;64:773-80. [Crossref] [PubMed]
- Dong XQ, Wu Z, Zhao H, et al. Evaluation and comparison of thirty noninvasive models for diagnosing liver fibrosis in chinese hepatitis B patients. J Viral Hepat 2019;26:297-307. [Crossref] [PubMed]
- Yan L, Deng Y, Zhou J, et al. Serum YKL-40 as a biomarker for liver fibrosis in chronic hepatitis B patients with normal and mildly elevated ALT. Infection 2018;46:385-93. [Crossref] [PubMed]
- Wang L, Liu T, Zhou J, et al. Changes in serum chitinase 3-like 1 levels correlate with changes in liver fibrosis measured by two established quantitative methods in chronic hepatitis B patients following antiviral therapy. Hepatol Res 2018;48:E283-90. [Crossref] [PubMed]
- Chinese Society of Hepatology, Chinese Medical Association. Guidelines for the diagnosis and management of hepatic encephalopathy in cirrhosis. Zhonghua Gan Zang Bing Za Zhi 2018;26:721-36. [PubMed]
- Collins GS, Reitsma JB, Altman DG, et al. Transparent reporting of a multivariable prediction model for individual prognosis or diagnosis (TRIPOD): the TRIPOD statement. BMJ 2015;350:g7594. [Crossref] [PubMed]
- DeLong ER, DeLong DM, Clarke-Pearson DL. Comparing the areas under two or more correlated receiver operating characteristic curves: a nonparametric approach. Biometrics 1988;44:837-45. [Crossref] [PubMed]
- Schuppan D, Ruehl M, Somasundaram R, et al. Matrix as a modulator of hepatic fibrogenesis. Semin Liver Dis 2001;21:351-72. [Crossref] [PubMed]
- Tsuchida T, Friedman SL. Mechanisms of hepatic stellate cell activation. Nat Rev Gastroenterol Hepatol 2017;14:397-411. [Crossref] [PubMed]
- Mak LY, Seto WK, Hui RW, et al. Fibrosis evolution in chronic hepatitis B e antigen-negative patients across a 10-year interval. J Viral Hepat 2019;26:818-27. [Crossref] [PubMed]
- Kumagai E, Mano Y, Yoshio S, et al. Serum YKL-40 as a marker of liver fibrosis in patients with non-alcoholic fatty liver disease. Sci Rep 2016;6:35282. [Crossref] [PubMed]
- Gamil M, Alboraie M, El-Sayed M, et al. Novel scores combining AFP with non-invasive markers for prediction of liver fibrosis in chronic hepatitis C patients. J Med Virol 2018;90:1080-6. [Crossref] [PubMed]
- Liu YR, Lin BB, Zeng DW, et al. Alpha-fetoprotein level as a biomarker of liver fibrosis status: a cross-sectional study of 619 consecutive patients with chronic hepatitis B. BMC Gastroenterol 2014;14:145. [Crossref] [PubMed]
- Feng L, Sun K, Zhang J, et al. A novel non-invasive index using AFP and APTT is associated with liver fibrosis in patients with chronic hepatitis B infection: a retrospective cohort study. BMJ Open 2015;5:e008032 [Crossref] [PubMed]
- Witters P, Freson K, Verslype C, et al. Review article: blood platelet number and function in chronic liver disease and cirrhosis. Aliment Pharmacol Ther 2008;27:1017-29. [Crossref] [PubMed]
- Wang Y, Xu MY, Zheng RD, et al. Prediction of significant fibrosis and cirrhosis in hepatitis B e-antigen negative patients with chronic hepatitis B using routine parameters. Hepatol Res 2013;43:441-51. [Crossref] [PubMed]
- Terrault NA, Bzowej NH, Chang KM, et al. AASLD guidelines for treatment of chronic hepatitis B. Hepatology 2016;63:261-83. [Crossref] [PubMed]
- Sarin SK, Kumar M, Lau GK, et al. Asian-Pacific clinical practice guidelines on the management of hepatitis B: a 2015 update. Hepatol Int 2016;10:1-98. [Crossref] [PubMed]
- Korean Association for the Study of the Liver. KASL clinical practice guidelines: management of chronic hepatitis B. Clin Mol Hepatol 2016;22:18-75. [Crossref] [PubMed]
- European Association for the Study of the Liver. EASL 2017 Clinical Practice Guidelines on the management of hepatitis B virus infection. J Hepatol 2017;67:370-98. [Crossref] [PubMed]
- Gao S, Li XY, Fan YC, et al. A noninvasive model to predict liver histology in HBeAg-positive chronic hepatitis B with alanine aminotransferase ≤ 2upper limit of normal. J Gastroenterol Hepatol 2017;32:215-20. [Crossref] [PubMed]
- Zehra M, Curry JC, Pillai SS, et al. Elucidating Potential Profibrotic Mechanisms of Emerging Biomarkers for Early Prognosis of Hepatic Fibrosis. Int J Mol Sci 2020;21:4737. [Crossref] [PubMed]
(English Language Editor: J. Gray)