Construction and validation of risk prediction model for deep vein thrombosis in acute exacerbations of chronic obstructive pulmonary disease based on serum angiopoietin 2 levels
Introduction
Chronic obstructive pulmonary disease (COPD) is a common disease of the respiratory system mainly characterized by a continuous airflow limitation due to airways and/or alveolar abnormalities usually caused by significant exposure to noxious particles or gases. COPD is associated with recurrent episodes, high morbidity, disability, and mortality (1). Most patients with COPD have persistent systemic inflammation, chronic hypoxia, and impaired pulmonary function over a long time. Therefore, COPD patients show decreased exercise tolerance and lower limb movement, often accompanied by lower limb vascular endothelial injury, thickened blood, and systemic congestion (2,3), which are all risk factors for thrombosis. An AECOPD is defined by a sustained worsening of symptoms, beyond normal day-to-day variation, which requires medical treatment (4). It is reported that the risk of DVT in AECOPD is 2.5 times that of the normal population, and the risk of pulmonary embolism is also doubled (5). Chen et al. (6) indicated that the overall incidence rate of DVT was 18.78 per 10,000 person-years in patients with COPD. A systematic review and meta-analysis showed that the incidence of deep vein thrombosis (DVT) in patients with AECOPD was 12.4% (7). Pulmonary embolism is one of the most serious complications of AECOPD, creating a substantial economic and social burden for families and society (8). An in-depth analysis of the risk factors for DVT in AECOPD patients will help to identify high-risk AECOPD patients at an early stage, thereby reducing the risk of DVT. However, there is currently no clear understanding of the relevant risk factors for DVT in AECOPD in clinical settings. Angiopoietin 2 (Ang-2) is a newly discovered endothelial growth factor enriched in the Weibel-Palade bodies of vascular endothelial cells. Ang-2 is a 57-kDa glycoprotein that shares homology with a number of other angiopoietins and angiopoietin-like molecules. The gene encoding Ang-2 protein is located on chromosomes 8p23.111. Ang-2, a 496 amino acid-long protein, shares ~60% amino acid homology with Ang1 (9,10). It is released outside the cell when vascular endothelial cells are damaged. Ang-2 participates in the occurrence and development of vascular endothelial cell injury by binding to the Tie-2 receptor, and DVT in the lower limbs is closely related to venous vascular endothelial cell injury (11). Alqudah et al. showed that compared with healthy people, serum Ang-2 levels are significantly increased in patients with venous thromboembolism of the lower limbs, a finding which helps diagnose DVT at an early stage (12). Ang-2 is also one of the inflammatory mediators. Increased levels of Ang-2 could destabilize the alveolar vasculature, which damages endothelial cells and leads to leakage, thereby resulting in pulmonary congestion and injury to the lung (13).
A disease risk prediction model is based on multiple causes of a disease. Through the establishment of a statistical model, it can predict the future probability of a disease in a population with specific characteristics (14). Constructing a risk prediction model for lower extremity DVT in patients with AECOPD can effectively aid medical staff in the early identification of people at risk of thrombosis and the implementation of individualized prevention strategies to reduce the occurrence of deep vein complications in the lower extremities. Hippisley-Cox et al. (15) constructed a prediction model for the occurrence of venous thrombosis in the overall population using a multicenter prospective research design. Their model quantified the absolute risk of venous thrombosis at 1 and 5 years to help identify patients with a high risk of venous thrombosis and perform prevention, but the patients included in their model did not specifically include those with AECOPD, and the predictive power of AECOPD with comorbid DVT in the lower extremities was not strong. Matsuo et al. (16) used D-dimer as an important diagnostic indicator for DVT in the lower extremities, but the specificity of D-dimer was only 13.3%. Cho et al. (13) also reported that serum Ang-2 levels were significantly higher in patients with acute exacerbations of COPD than in those with stable COPD or controls. However, the study just enrolled forty-nine patients with AECOPD, and did not investigate the association between serum Ang-2 levels and DVT.
Considering that AECOPD patients are at a high risk of developing DVT in the lower extremities and that Ang-2 has not been included in the established models at home and abroad, the relationship between the levels of circulating Ang-2 and AECOPD with comorbid DVT in the lower extremities remains to be clarified. Therefore, this study adopted a prospective and observational research design by selecting 650 patients with AECOPD for a case-control study based on serum Ang-2 as a risk factor for DVT. In addition, we examined the association between serum Ang-2 levels and DVT in AECOPD patents. Furthermore, based on the TRIPOD statement (17), this study was targeted specifically at AECOPD patients to establish a serum Ang-2-based risk prediction model of DVT to assist clinicians in the early identification of individuals at risk of lower extremity DVT and provide a theoretical and decision-making basis for early prevention of thrombosis. We present the following article in accordance with the TRIPOD reporting checklist (available at https://dx.doi.org/10.21037/apm-21-1448).
Methods
Research design
This study was a single-center, prospective, observational study.
Research subjects and inclusion and exclusion criteria
The convenience sampling method was used to recruit 650 AECOPD patients admitted to the Department of Pulmonary and Critical Care Medicine of the First Affiliated Hospital of Chengdu Medical College from January 2019 to January 2021. The random number table method was used to divide the enrolled patients into a modeling group (n=455) and a verification group (n=195) according to a 7:3 ratio. The diagnostic criteria of the 2019 GOLD guidelines (18) were used to confirm the diagnosis of chronic obstructive pulmonary disease; the diagnostic criteria of the 2017 edition of the Chinese Medical Association Surgery Branch “Deep Vein Thrombosis Diagnosis and Treatment Guidelines (Third Edition)” (19) were used to establish the diagnosis of lower extremity DVT, which was confirmed by Doppler ultrasound of the lower extremity blood vessels. The current gold standard for the clinical diagnosis of DVT is lower extremity venography. Nevertheless, venography is an invasive procedure, and Doppler ultrasound of the lower extremity blood vessels is a non-invasive procedure which effectively visualizes the thrombosis in veins. Non-invasive procedure is more acceptable to patients. Thus, we chose Doppler ultrasound of the lower extremity blood vessels as a means of detecting DVT. Exclusion criteria were as follows: (I) patients with a history of tumors; (II) patients who refused to participate in this study; (III) patients with neuropsychiatric diseases; and (IV) patients who could not complete the relevant examinations as requested by their treating doctor. Inclusion criteria were as follows: patients who were diagnosed, or patients suspected of being diagnosed, with pulmonary embolism in line with the “2019 ESC/ERS Acute Pulmonary Embolism Diagnosis and Management Guidelines” (20). This study was approved by the Ethics Committee of the First Affiliated Hospital of Chengdu Medical College (2020CYFYIRB-BA-131). All procedures involving human participants complied with the Declaration of Helsinki (revised in 2013), and informed consent was obtained from all patients.
Variable selection
Risk factors were established following a literature review, small-scale expert consultation, and our previous experience in constructing a risk prediction model for DVT of the lower extremities and were screened as follows:
- Basic information included gender, age, past history, smoking history, drinking history, and long-term antiplatelet data;
- Clinical features consisted of initial mean arterial pressure, arrhythmia, lower extremity edema, living ability score, respiratory failure, and length of stay in hospital;
- Detection of the general biological blood indicators consisted of initial results of white blood cell count; hemoglobin, hematocrit, and platelet levels; blood creatinine content; D-dimer; international normalized ratio (INR); and blood gas analysis following admission;
- Detection of Ang-2: an enzyme-linked immunosorbent assay was used to detect the blood Ang-2 levels of patients (Human Ang-2 ELISA kit, R&D System, Inc., MN, USA), according to the manufacturer’s instructions. Signals were quantified by measuring the absorbance at 450 nm using a BioTek plate reader;
- Auxiliary examination was conducted with a color Doppler ultrasound of the blood vessels of the lower extremities.
Outcome indicators
Patients in the modeling group were further subdivided into a thrombus group and a non-thrombus group according to the results of a lower extremity deep vein color Doppler ultrasonography after admission.
Statistical analysis
Assessment of the sample size
According to small-scale expert consultation, a literature review (mainly a systematic review of the risk factors for COPD with comorbid DVT), and the experience with a DVT analysis and prediction model constructed by our predecessors, 21 candidate factors were identified for DVT of the lower extremities. The names and values of the candidate factors are shown in Table 1. The sample size was calculated depending on number of independent variables in prediction model. The sample size was at least 10 times more than the number of independent variables (21). Assuming that each factor requires at least 10 cases for verification and the follow-up loss rate is 10%, the expected sample size should be at least 233 cases, with the formula: 21×10÷0.9=233. To increase the reliability of the predicted model, 650 cases were finally included in the study.
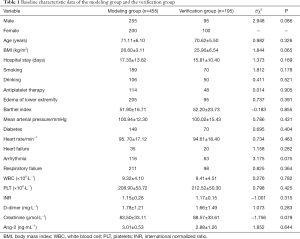
Full table
Processing of missing values
Only patients with complete data were included in the analysis.
Data were analyzed using SPSS 23.0 (SPSS Inc., Chicago, IL, USA) and R 3.6.1software (R Development Core Team, www.r-project.org). Count data are described by the number of cases (rate) and were analyzed by the χ2 test. Normally distributed measurement data are represented by the mean ± SD () and were analyzed using independent t-tests for two-group comparisons. Binary logistic regression analysis was performed on variables reaching statistical significance to determine the independent risk factors for DVT. The independent risk factors were imported into R software for further analysis, and the “rms” program package in R software was used to establish the nomogram and correction diagram of DVT occurrence. Discrimination, calibration, and clinical validity were verified in the modeling group and the verification group. The ROC curve was drawn based on the results of the nomogram model. The evaluation of the predictive power of the model for DVT in patients with AECOPD was performed by calculating the area under the curve (AUC), where 0.5< AUC <0.7 indicates that the model’s distinguishing ability is low; 0.7< AUC <0.9 indicates a moderate ability and AUC>0.9 reflects a higher discrimination ability. The Hosmer-Lemeshow goodness-of-fit test was used to evaluate the calibration of the prediction model: in this test, a P value >0.05 suggests a small difference between the predicted value of the model and the actual observation, indicating the model calibration is good. A decision curve package was used to draw the decision curve, and a clinical impact curve was drawn to evaluate the net clinical benefit and clinical impact of the model. Results with a P value <0.05 indicated a statistically significant difference.
Results
General information
From January 2019 to January 2021, 700 cases of AECOPD were initially considered for the study. Of these, 25 patients with comorbid tumors were excluded, and 25 patients died before the screening was completed. A final 650 patients who met the criteria were included in the study, and the incidence of thrombosis in this sample was 25.8% (168/650). These cases were then divided into a modeling group (455 cases) and a verification group (195 cases), with details shown in Table 1 and Figure 1. Differences in demographic data, clinical characteristics, auxiliary examinations, and other data between the two groups were not statistically significant (P>0.05), indicating the two groups were comparable.
Analysis of individual factors in the modeling group
In the modeling group, there was no statistical difference between the thrombus group and the non-thrombus group in terms of gender composition, age, drinking history, smoking history, hospital stay, long-term antiplatelet therapy, living ability score, heart rate, arrhythmia, or heart failure (P>0.05). In the thrombus group, average arterial pressure was higher than that in the non-thrombus group. The incidence of lower extremity edema, respiratory failure and diabetes was also higher in the thrombus group relative to the non-thrombus group.
Additionally, the BMI index of the thrombus group was significantly higher than the non-thrombus group (P<0.05, Table 2).
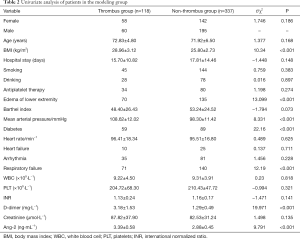
Full table
There was no significant difference in white blood cell count (WBC), platelet count, hematocrit, INR, or blood creatinine content between the two subgroups of patients (P>0.05). The concentration of D-dimer and serum Ang-2 in the thrombus group was significantly higher than that in the non-thrombus group (P<0.05, Figure 2A). When serum Ang-2 concentration alone was used as a diagnostic indicator, the sensitivity was 96.14%, the specificity was 67.99%, the cutoff value was 3.01 ng/mL, AUC =0.782, and the 95% CI: 0.742–0.819 (Figure 2B).
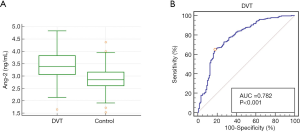
Logistic multivariate regression analysis of the modeling group
Logistic multivariate regression analysis was conducted by taking DVT as the dependent variable and the statistically significant factors in the univariate analysis results (respiratory failure, lower extremity edema, BMI index, diabetes, D-dimer, and serum Ang-2) as the independent variables. The variable assignment table is shown in detail in Table 3. The results suggested that lower extremity edema, BMI index, diabetes, respiratory failure, D-dimer, and serum Ang-2 were independent risk factors for COPD, while mean arterial pressure was not an independent risk factor for COPD complicated with DVT (Figure 3).
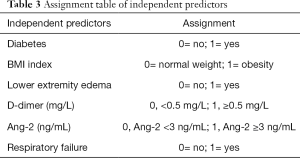
Full table
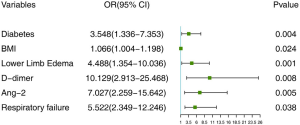
Establishing the risk nomogram model of AECOPD with comorbid DVT
The selected independent risk factors were entered into the R software, and the “lrm” function in the “rms” package was used to build the nomogram prediction model of AECOPD with comorbid DVT (Figure 4). The nomogram was constructed as follows: the score value corresponding to each predictive factor was obtained, and the sum of these score values was recorded as the total score. Finally, the predicted probability corresponding to the total score was the risk of DVT in AECOPD.
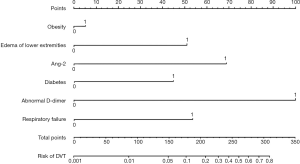
Verification of the prediction model
Distinguishing degree
The ROC curve of the two groups (see Figure 5A) shows that the modeling group had an AUC value of 0.844 (95% CI: 0.808–0.932) and a C index of 0.823. The verification group had an AUC value of 0.755 (95% CI: 0.679–0.861) and a C index of 0.764 (see Figure 5B), indicating that the C index of the prediction model in both groups was greater than 0.75.
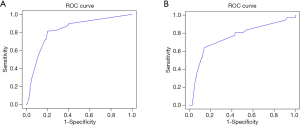
Calibration
Results of the Hosmer-Lemeshow test on the prediction model in the modeling group (P=0.124) and the verification group (P=0.086) showed that the P value in both groups was greater than 0.05, indicating that the difference was not statistically significant. The calibration chart showed that the predicted probability of the nomogram was highly similar to the actual probability of the modeling group and the verification group (Figure 6A,B).
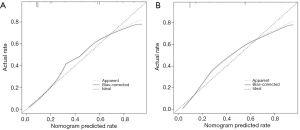
Clinical effectiveness
Decision curve analysis (DCA) was used to evaluate the availability and benefits of the prediction model, and the curve was drawn using the R software “rmda” package. The decision curve for the modeling group and the verification group is shown in Figure 7A,B. The abscissa represents the threshold probability, and the probability of DVT in AECOPD predicted by the model is represented as Pi. When Pi reaches a certain threshold (recorded as Pt), it is defined as positive and indicates that the model will benefit the patient (gain). However, there will also be losses in patients with DVT which are not predicted (loss), and the net benefit (NB) is calculated by gain minus loss (22). The decision curve analysis illustrated in Figure 7A,B showed that the nomogram had highest NB across 10-92% threshold probabilities in the modeling group. Similar results were observed in the verification group. In this study, the “plot clinical impact function” in R software was used to draw the clinical impact curve of the prediction model. The model was set up to predict risk stratification for 1,000 people, displaying the “loss” and “gain” axes and assigning 10 levels (Figure 7C,D). The red curve represents the number of people classified with DVT by the model under each threshold probability; the blue curve is the actual number of people with DVT at each threshold probability. The clinical impact curve suggests that the predicted probability of the model is essentially the same as the probability of DVT in the actual clinical setting.
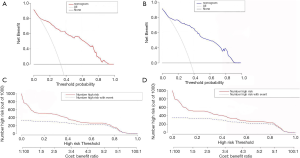
Discussion
The incidence and mortality of COPD are increasing yearly, and it is currently the fourth cause of death worldwide (23). China has the main burden of COPD with a total of over 100 million patients suffering from COPD. Along with hypertension and diabetes, COPD is the most common chronic disease in China and causes substantial social and family economic burdens (24). COPD patients often suffer from chronic hypoxia, poor lung function, decreased activity endurance, and long-term bed rest in some cases, and these factors lead to slower blood flow and hypercoagulable blood that create the pathophysiological conditions for the formation of DVT. Studies have shown that more than 90% of pulmonary embolisms arise from DVT embolisms, the incidence of COPD with pulmonary embolism is approximately 15%, and the incidence of AECOPD with DVT is 19–29% (25,26). Yue et al. (27) have shown that the incidence of pulmonary embolism secondary to AECOPD is 23% higher than that of non-AECOPD patients, and the incidence of AECOPD with comorbid DVT was 19.61%. DVT has become a serious common comorbidity of AECOPD. The incidence of AECOPD with comorbid DVT in this study was 25.8%, which is consistent with the results of previous studies.
In this study, a univariate analysis of factors including gender, age, smoking and drinking history, length of hospitalization, antiplatelet therapy, living ability score, heart rate, and arrhythmia in the thrombus and non-thrombus subgroups of the modeling group showed no significant differences between the two groups, whereas lower limb edema, respiratory failure, and BMI index were significantly different between the two groups. Multivariate logistic regression analysis was subsequently performed on the significant factors identified by the univariate analysis. Results indicated that lower limb edema and BMI index were independent risk factors for lower limb thrombosis, whereas mean arterial pressure was not an independent risk factor. Lung function injury of AECOPD, especially in the elderly, can substantially reduce lower limb exercise, leading to slowing the blood flow in the lower extremities and lower extremity edema. Slowing of blood may contribute to thrombosis development, which can result in swelling in lower extremities. Lower limb thrombosis and lower limb edema influence and promote each other. Determining exactly how these factors are causally related, therefore, warrants further study. At the same time, the nomogram also suggested that obese people have an increased risk of DVT. BMI is an important indicator for evaluating nutritional status, and it has been reported that people with a BMI greater than 30 kg/m2 have a risk of DVT that is three times higher than that of normal-weight people (28). Because obesity is a state of chronic low-grade inflammation (29), it is more likely to induce vascular endothelial dysfunction and increase coagulation factor levels, both of which are pathophysiological factors associated with DVT of the lower extremities. It is worth noting that the predictive model of this study indicated that diabetes and respiratory failure are also independent risk factors for AECOPD with comorbid DVT. This may be due to the fact that long-term hyperglycemia can cause an increase in oxidative stress and damage the function and structural integrity of vascular endothelial cells, further increasing the incidence of thrombotic diseases. Studies have reported that DVT incidence in diabetic patients can be as high as 15–48% (30). In addition, hypoxia or carbon dioxide retention caused by respiratory failure can not only further damage the function of the vascular endothelium but may also lead to an increase in secondary red blood cells causing increased blood viscosity and blood stasis to form DVT in the lower extremities (31). The prediction model in this study was able to predict the risk of DVT in AECOPD with comorbid diabetes and respiratory failure in the clinical setting and thus allowed a timely investigation and intervention for DVT.
The occurrence and development of thrombotic diseases are not only related to abnormal blood coagulation and fibrinolysis processes but also to intravascular inflammation. Chung et al. (32) showed that thrombosis is also an inflammatory reaction process because there is a close relationship between inflammatory reaction and thrombosis. The massive release of inflammatory mediators and the damage to the vascular endothelium during infection leads to platelet adhesion and aggregation. The Ang-2 gene is located in the 8q23.1 chromosome and expressed in vascular endothelial cells. In the resting state, vascular endothelial cells express only a small amount of Ang-2, while the expression of Ang-2 increases significantly after being activated by external stimuli (33). In this study, the comparison of serum Ang-2 between the thrombus and non-thrombus groups showed that serum Ang-2 in the thrombus group was significantly higher than that in the non-thrombus group, and Ang-2 also had a higher sensitivity in diagnosing AECOPD complicated with DVT of the lower extremities. Gurnik et al. (34) reported that the main function of activated Ang-2 is to change the binding force of the Tie-2 receptor and depolymerize the Tie-2/Tie-1 receptor dimer. After depolymerization, Tie-2 can activate intracellular tyrosine kinase activity to activate downstream signaling pathways and realize related functions: by releasing the anchor chain effect on the intracellular skeletal protein, the structure of endothelial cells becomes relatively loose; and the interaction of Tie-2/Tie-1 between endothelial cells is changed, thereby increasing the gap between capillaries, and promoting the migration of peripheral white blood cells to the tissues. These changes will affect the flow of tissue fluid, aggravate the inflammatory response, and lead to edema, suggesting that lower extremity edema may also be related to increased Ang-2 expression and could be the reason why lower extremity edema and Ang-2 are independent risk factors for DVT. Sahni et al. (35) also reported that the serum level of Ang-2 is positively correlated with inflammatory mediators such as TNF-α and interleukin 6, and these two inflammatory factors have been proved to be risk factors for DVT in many studies. As a pro-inflammatory factor, Ang-2 plays an important role in the physiological activities of promoting inflammation and vascular leakage (32). D-dimer is not only the smallest fibrin degradation product but also a specific degradation product of the fibrous matrix of venous thrombosis. D-dimer will be abnormally elevated in the case of blood hypercoagulability or secondary hyperfibrinolysis and is a highly sensitive indicator of thrombosis and dissolution (36). This study also found that D-dimer concentration was significantly higher in patients with AECOPD complicated with DVT than those without thrombosis. However, most studies believe that D-dimer has a lower specificity in diagnosing DVT. Therefore, this study combined D-dimer and other clinical features and indicators to establish a nomogram model, which combines the factors of lower extremity edema, BMI, diabetes, respiratory failure, D-dimer, and serum Ang-2. When the calculated score increases in the nomogram model, the corresponding risk of DVT also increases significantly. In this study, the Hosmer-Lemeshow test and ROC curve analysis were performed to verify the predictive performance of the nomogram, which indicated that the model fits well and has good predictive value in estimating the probability of DVT occurrence in AECOPD. Furthermore, by using the DCA and clinical impact curve to evaluate the availability and benefit of the model, it was found that the model can benefit 0.37–0.92 of patients, and the predicted probability of the model is consistent with the actual clinical probability of DVT. These results indicate that the model has an important impact on clinical benefit and helps clinicians with timely recognition and prevention of DVT in patients with AECOPD.
However, this study has some limitations. There have been many reports in the literature that bed rest or immobilization for more than 3 days are independent risk factors for DVT in patients with AECOPD and can lead to a significant increase in DVT incidence (37,38). But, in this study, none of the AECOPD patients were ICU patients, and their bed rest was no more than three days, so bed rest was not included as a risk factor. Secondly, there was no dynamic monitoring of serum Ang-2 levels undertaken in this study, so it was not possible to explore the correlation between the fluctuation of serum Ang-2 levels and the prognosis of AECOPD complicated with DVT; Finally, Due to the limited experimental conditions and other resources, this study is a single-center study, and the model has not been externally verified by other centers. Therefore, in future studies, the sample size should be expanded, and the predictive value of the nomogram model should be verified by a multi-center study.
Conclusions
In summary, our nomogram model for predicting the risk of DVT in AECOPD based on serum Ang-2 levels has good calibration, discrimination, and clinical impact. The model is a good fit and has a high predictive value. Due to the limitations of this study, multi-center follow-up studies with larger samples will be needed to verify and add to the current findings.
Acknowledgments
Funding: None.
Footnote
Reporting Checklist: The authors have completed the TRIPOD reporting checklist. Available at https://dx.doi.org/10.21037/apm-21-1448
Data Sharing Statement: Available at https://dx.doi.org/10.21037/apm-21-1448
Conflicts of Interest: All authors have completed the ICMJE uniform disclosure form (available at https://dx.doi.org/10.21037/apm-21-1448). The authors have no competing interests to declare
Ethical Statement: The authors are accountable for all aspects of the work in ensuring that questions related to the accuracy or integrity of any part of the work are appropriately investigated and resolved. This study was approved by the Ethics Committee of The First Affiliated Hospital of Chengdu Medical College (No. 2020CYFYIRB-BA-131). All procedures performed in this study involving human participants were in accordance with the Declaration of Helsinki (as revised in 2013). Informed consent was obtained from all patients.
Open Access Statement: This is an Open Access article distributed in accordance with the Creative Commons Attribution-NonCommercial-NoDerivs 4.0 International License (CC BY-NC-ND 4.0), which permits the non-commercial replication and distribution of the article with the strict proviso that no changes or edits are made and the original work is properly cited (including links to both the formal publication through the relevant DOI and the license). See: https://creativecommons.org/licenses/by-nc-nd/4.0/.
References
- Polverino F, Kheradmand F. COVID-19, COPD, and AECOPD: Immunological, Epidemiological, and Clinical Aspects. Front Med (Lausanne) 2021;7:627278 [Crossref] [PubMed]
- Vanfleteren LE, Spruit MA, Groenen M, et al. Clusters of comorbidities based on validated objective measurements and systemic inflammation in patients with chronic obstructive pulmonary disease. Am J Respir Crit Care Med 2013;187:728-35. [Crossref] [PubMed]
- Bakeer M, Funk GC, Valipour A. Chronic obstructive pulmonary disease phenotypes: imprint on pharmacological and non-pharmacological therapy. Ann Transl Med 2020;8:1472. [Crossref] [PubMed]
- Harrison SL, Goldstein R, Desveaux L, et al. Optimizing nonpharmacological management following an acute exacerbation of chronic obstructive pulmonary disease. Int J Chron Obstruct Pulmon Dis 2014;9:1197-205. [Crossref] [PubMed]
- Dutt TS, Udwadia ZF. Prevalence of venous thromboembolism in acute exacerbations of chronic obstructive pulmonary disease: an Indian perspective. Indian J Chest Dis Allied Sci 2011;53:207-10. [PubMed]
- Chen CY, Liao KM. The Incidence of Deep Vein Thrombosis in Asian Patients With Chronic Obstructive Pulmonary Disease. Medicine (Baltimore) 2015;94:e1741 [Crossref] [PubMed]
- Rizkallah J, Man SFP, Sin DD. Prevalence of pulmonary embolism in acute exacerbations of COPD: a systematic review and metaanalysis. Chest 2009;135:786-93. [Crossref] [PubMed]
- Peng L, Han L, Li XN, et al. The Predictive Value of microRNA-134 and microRNA-1233 for the Early Diagnosis of Acute Exacerbation of Chronic Obstructive Pulmonary Disease with Acute Pulmonary Embolism. Int J Chron Obstruct Pulmon Dis 2020;15:2495-503. [Crossref] [PubMed]
- Wang JM, Xu WD, Yuan ZC, et al. Serum levels and gene polymorphisms of angiopoietin 2 in systemic lupus erythematosus patients. Sci Rep 2021;11:10. [Crossref] [PubMed]
- Akwii RG, Sajib MS, Zahra FT, et al. Role of Angiopoietin-2 in Vascular Physiology and Pathophysiology. Cells 2019;8:471. [Crossref] [PubMed]
- Joussen AM, Ricci F, Paris LP, et al. Angiopoietin/Tie2 signalling and its role in retinal and choroidal vascular diseases: a review of preclinical data. Eye (Lond) 2021;35:1305-16. [Crossref] [PubMed]
- Alqudah M, Qandeel H, Al-Zoubi N, et al. Changes of serum growth factors profiles in patients with venous thromboembolism. Scand J Clin Lab Invest 2017;77:595-600. [Crossref] [PubMed]
- Cho YJ, Ma JE, Yun EY, et al. Serum angiopoietin-2 levels are elevated during acute exacerbations of COPD. Respirology 2011;16:284-90. [Crossref] [PubMed]
- Stark GF, Hart GR, Nartowt BJ, et al. Predicting breast cancer risk using personal health data and machine learning models. PLoS One 2019;14:e0226765 [Crossref] [PubMed]
- Hippisley-Cox J, Coupland C. Development and validation of risk prediction algorithm (QThrombosis) to estimate future risk of venous thromboembolism: prospective cohort study. BMJ 2011;343:d4656. [Crossref] [PubMed]
- Matsuo H, Nakajima Y, Ogawa T, et al. Evaluation of D-Dimer in Screening Deep Vein Thrombosis in Hospitalized Japanese Patients with Acute Medical Diseases/Episodes. Ann Vasc Dis 2016;9:193-200. [Crossref] [PubMed]
- Collins GS, Reitsma JB, Altman DG, et al. Transparent reporting of a multivariable prediction model for individual prognosis or diagnosis (TRIPOD): the TRIPOD statement. BMJ 2015;350:g7594. [Crossref] [PubMed]
- Singh D, Agusti A, Anzueto A, et al. Global Strategy for the Diagnosis, Management, and Prevention of Chronic Obstructive Lung Disease: the GOLD science committee report 2019. Eur Respir J 2019;53:1900164 [Crossref] [PubMed]
- Li XQ, Zhang FX. Guidelines for the diagnosis and treatment of deep vein thrombosis (3rd Ed.). Chinese Journal of Vascular Surgery (Electronic Edition) 2017;9:250-7.
- Konstantinides SV, Meyer G, Becattini C, et al. 2019 ESC Guidelines for the diagnosis and management of acute pulmonary embolism developed in collaboration with the European Respiratory Society (ERS): The Task Force for the diagnosis and management of acute pulmonary embolism of the European Society of Cardiology (ESC). Eur Respir J 2019;54:1901647 [Crossref] [PubMed]
- Yan YJ, Jiang L, Hu ML, et al. Psychometric Properties of a Simplified Chinese Version of the Secondary Trauma Questionnaire in a Potentially Traumatized Study Sample. Front Psychol 2020;11:767. [Crossref] [PubMed]
- Lv X, Wu Z, Cao J, et al. A nomogram for predicting the risk of lymph node metastasis in T1-2 non-small-cell lung cancer based on PET/CT and clinical characteristics. Transl Lung Cancer Res 2021;10:430-8. [Crossref] [PubMed]
- Borné Y, Ashraf W, Zaigham S, et al. Socioeconomic circumstances and incidence of chronic obstructive pulmonary disease (COPD) in an urban population in Sweden. COPD 2019;16:51-7. [Crossref] [PubMed]
- Liao JP, Wang X, Liu F, et al. Serum surfactant protein D, lung function decline, and incident chronic obstructive pulmonary disease: a longitudinal study in Beijing. J Thorac Dis 2021;13:92-100. [Crossref] [PubMed]
- Rali PM, Criner GJ. Submassive Pulmonary Embolism. Am J Respir Crit Care Med 2018;198:588-98. [Crossref] [PubMed]
- Hassen MF, Tilouche N, Jaoued O, et al. Incidence and Impact of Pulmonary Embolism During Severe COPD Exacerbation. Respir Care 2019;64:1531-6. [Crossref] [PubMed]
- Yue XY, Li FJ, Jiang ZF, et al. Clinical features and risk factors of acute exacerbation of chronic obstructive pulmonary disease complicated with deep venous thrombosis of lower extremity. J Cap Univ Med Sci 2021;42:153-7.
- Sloan M, Sheth N, Lee GC. Is Obesity Associated With Increased Risk of Deep Vein Thrombosis or Pulmonary Embolism After Hip and Knee Arthroplasty? A Large Database Study. Clin Orthop Relat Res 2019;477:523-32. [Crossref] [PubMed]
- Asghar A, Sheikh N. Role of immune cells in obesity induced low grade inflammation and insulin resistance. Cell Immunol 2017;315:18-26. [Crossref] [PubMed]
- Ojemolon PE, Shaka H, Edigin E, et al. Impact of Diabetes Mellitus on Outcomes of Patients With Knee Osteoarthritis Who Underwent Knee Arthroplasty: An Analysis of the Nationwide Inpatient Sample. Cureus 2020;12:e8902 [PubMed]
- Lichota A, Szewczyk EM, Gwozdzinski K. Factors Affecting the Formation and Treatment of Thrombosis by Natural and Synthetic Compounds. Int J Mol Sci 2020;21:7975. [Crossref] [PubMed]
- Chung WS, Lin CL. Association between venous thromboembolism and acute pancreatitis: An analysis from the nationwide inpatient sample. Clin Respir J 2020;14:320-7. [Crossref] [PubMed]
- Chandel S, Sathis A, Dhar M, et al. Hyperinsulinemia promotes endothelial inflammation via increased expression and release of Angiopoietin-2. Atherosclerosis 2020;307:1-10. [Crossref] [PubMed]
- Gurnik S, Devraj K, Macas J, Yamaji M, et al. Angiopoietin-2-induced blood-brain barrier compromise and increased stroke size are rescued by VE-PTP-dependent restoration of Tie2 signaling. Acta Neuropathol 2016;131:753-73. [Crossref] [PubMed]
- Sahni M, Yeboah B, Das P, et al. Novel biomarkers of bronchopulmonary dysplasia and bronchopulmonary dysplasia-associated pulmonary hypertension. J Perinatol 2020;40:1634-43. [Crossref] [PubMed]
- Halaby R, Popma CJ, Cohen A, et al. D-Dimer elevation and adverse outcomes. J Thromb Thrombolysis 2015;39:55-9. [Crossref] [PubMed]
- Kruger PC, Eikelboom JW, Douketis JD, et al. Deep vein thrombosis: update on diagnosis and management. Med J Aust 2019;210:516-24. [Crossref] [PubMed]
- Liang LR, Zhang Z, Wang H, et al. Proportion and prevention of venous thromboembolism among hospitalized patients with acute exacerbation of chronic obstructive pulmonary disease in Beijing. Zhonghua Yi Xue Za Zhi 2013;93:2337-40. [PubMed]