Integration of network pharmacology and molecular docking technology reveals the mechanism of the herbal pairing of Codonopsis Pilosula (Franch.) Nannf and Astragalus Membranaceus (Fisch.) Bge on chronic heart failure
Introduction
Chronic heart failure (CHF) is an advanced stage of cardiac dysfunction secondary to many diseases, and acts as the primary cause of death. Patients with CHF have mixed causes, which are not occur independently, and more than two-thirds of all cases of CHF can be attributed to four underlying aetiologies: ischaemic heart disease, hypertensive heart disease, chronic obstructive pulmonary disease and rheumatic heart disease (1). Patients with CHF mainly present with dyspnea, fatigue, limited exercise tolerance, and fluid retention, which significantly diminishes their quality of life (2). Despite advances in CHF treatment, morbidity and mortality remain high. It is reported that the global incidence of CHF ranges from 100 to 900 cases per 100,000 person-years (3) and is estimated 64.3 million individuals worldwide (4). Owing to the aging population, the prevalence of CHF is projected to increase 46% over the next 10 years. The total percentage of the population with CHF is predicted to increase from 2.42% to 2.97% in 2030 (5). Furthermore, the total medical expenses for patients with heart failure (HF) in the USA are estimated to reach US$53.1 billion by 2030 (6). CHF imposes an enormous burden on public health systems worldwide. Therefore, exploration of more effective treatments for CHF with fewer side effects is urgently needed.
Chinese herbal medicine (CHM) is characterized by multiple components, targets, and pathways, and has been widely applied to treat CHF in China for more than 2,000 years (7,8). Compared to Western medicine (WM) alone, integration of CHM and WM can better alleviate symptoms, more significantly improve exercise load, enhance quality of life, and has fewer side effects (9,10). In the theory of traditional Chinese medicine (TCM), Qi is the most basic substance of the human body and possesses the function of promotion, domination, defense, and warm. Qi deficiency syndrome is one of the basic syndromes in TCM and is characterized by physical weakness, shortness of breath, sweating, pale, low voice etc. (11). Qi deficiency syndrome is the major pathogenesis of CHF, encompassing its occurrence, development, and outcomes. Qi-boosting (YiQi) can be understood as supplementing Qi, the most basic substance of the human body, and restoring the function of Qi to alleviate the Qi deficiency syndrome (12). So Qi-boosting is an essential principle to treat disease with Qi deficiency syndrome such as CHF. The herbal pairing of Dangshen (DS) [Codonopsis pilosula (Franch.) Nannf.] and Huangqi (HQ) [Astragalus membranaceus (Fisch.) Bge.] (DHP) is compatible and exerts a synergistic effect on Qi-boosting, and has been widely used to treat disease with Qi deficiency syndrome such as CHF in China. Moreover, DHP is also the major herbal pairing of some Chinese formulas for CHF treatment, such as Qili Qiangxin capsules, Shenqi Fuzheng injection, and Qishen granules. These formulas can reduce the levels of N-terminal signal peptide of pro-B-type natriuretic peptide (NT-proBNP) and improve heart function, and thus, are widely used in combined therapy for the treatment of CHF (9,13-15). Our previous study and other animal experiments have shown that DHP has effects on the treatment of CHF, which may relate to regulating myocardial energy metabolism, inhibiting inflammation, improving cardiac remodeling, and enhancing myocardial contractility (16). However, due to the complexity of its chemical compounds, the mechanisms of DHP in the treatment of CHF have not fully been elucidated.
Network pharmacology is an emerging and powerful tool. It can predict the direct targets of the potential active compounds of CHM and systematically reveal the underlying mechanisms (17). Molecular docking can examine the interaction between the receptor and drug molecules, and predict its binding mode and affinity, and is a critical approach for structural molecular biology and computer-aided drug design for new drugs (18). In this study, we integrated network pharmacology and molecular docking technology to identify the complex mechanisms of DHP on CHF. The study flowchart is shown in Figure 1. We present the following article in accordance with the MDAR reporting checklist (available at https://dx.doi.org/10.21037/apm-21-1469).
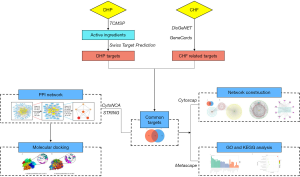
Methods
Active ingredients of DHP screening
The compounds of DS and HQ were collected from the Traditional Chinese Medicine Systems Pharmacology Database (TCMSP, https://tcmspw.com/tcmsp.php). This platform is designed for CHMs and includes pharmacochemistry, absorption, distribution, metabolism, and excretion (ADME) (19). Two ADME parameters, oral bioavailability (OB) and drug-likeness (DL) were used to screen the active ingredients of DHP.
Prediction of potential targets of DHP
The active ingredients of DHP were imported into the PubChem database (https://pubchem.ncbi.nlm.nih.gov/) (20) to obtain the two-dimensional (2D) structures of the compounds. Based on the SwissTargetPrediction database (https://www.swisstargetprediction.ch/) (21), the potential targets of DHP’s active ingredients in Homo sapiens were predicted.
CHF related targets screening
CHF-related targets were retrieved from the DisGeNET database (https://www.disgenet.org/) (22) and the GeneCards database (https://www.genecards.org/) (23). “Chronic Heart Failure” was used as the keyword for screening in both databases. This study was conducted in accordance with the Declaration of Helsinki (as revised in 2013).
Common targets between the potential targets of DHP and CHF-related targets
The common targets were obtained after DHP potential targets mapping onto CHF-related genes, and were displayed using a Venn diagram. These common targets play a vital role in medicinal treatment for disease and were used for subsequent analysis.
Protein-protein interaction (PPI) analysis
The common targets were imported into the Search Tool for the Retrieval of Interacting Genes/Proteins (STRING) database (https://string-db.org/) (24), which designs protein interaction analysis and PPI network construction. In our study, we set the interaction score as the highest confidence (>0.9). The PPI data were used for further topological properties analysis to identify the hub genes of DHP treatment of CHF.
Gene Ontology (GO) and Kyoto Encyclopedia of Genes and Genomes (KEGG) pathway enrichment analyses
To reveal the potential mechanisms of DHP in the treatment of CHF, we imported the common targets into the Metascape database (http://metascape.org/) (25) for GO and KEGG pathway enrichment analyses with Homo sapiens. GO constructs three different levels to describe genes: biological process (BP), molecular function (MF), and cellular component (CC) (26). KEGG analyzes the pathways involved in genes, which helps to better understand the relevant pathways (27). According to the corrected P value, the results of the GO and KEGG pathway enrichment analyses were sorted. R software (version 3.6.3) was used to visualize the results.
Network construction and analysis
Cytoscape 3.7.2 (https://cytoscape.org/) (28), a software platform used for visualizing molecular interaction networks and biological pathways, was utilized to draw the following networks: (I) common targets network; (II) Herb-Ingredient-Potential target-Disease network (H-I-P-D network); (III) PPI network; and (IV) Pathway-related targets network (P-R network). The Cytoscape plugins Network Analyzer and CytoNCA were used to analyze the topological properties of the networks (29,30). In the H-I-P-D network, ingredients with the top five degree values were identified as the key ingredients of DHP. After two rounds of screening the median using a series of parameters, including degree, betweenness centrality (BC), closeness centrality (CC), eigenvector centrality (EC), the local average connectivity-based method (LAC), and network centrality (NC), the hub genes of DHP in CHF treatment were filtered and used for subsequent molecular docking simulation.
Molecular docking validation
Molecular docking was performed with the key ingredients and hub genes to assess their binding affinities. The three-dimensional crystal structures of the hub genes were downloaded from the Research Collaboratory for Structural Bioinformatics Protein Data Bank (RCSB-PDB; https://www.rcsb.org) (31). The MOL2 format files of key compounds were retrieved from TCSMP. The downloaded proteins were processed by PyMol 2.4.0 to remove water molecules and extract ligands. All target proteins and compounds were saved as PDBQT format using AutoDock Tools (ADT) (32). AutoDock Vina software was then used to perform the molecular docking simulation, and PyMol 2.4.0 was used for visualization (33).
Statistical analysis
We used Cytoscape version 3.7.2 to analyse the topological data. GO and KEGG pathway enrichment analysis were conducted by Metascape database.
Results
Active ingredients and potential targets of DHP
According to the ADME parameters (OB ≥30% and DL ≥0.18) and invalid components exclusion, 33 active ingredients of DHP were obtained from the TCMSP database, 16 were obtained from DS, and 17 were obtained from HQ (Table 1). The 2D chemical structures of the 33 active ingredients were identified using PubChem. Based on these 2D structures, 795 targets were obtained from SwissTargetPrediction, including 385 targets from DS and 410 from HQ. Finally, we captured 561 targets as potential targets of DHP, after removing the duplicate values (https://cdn.amegroups.cn/static/public/apm-21-1469-1.pdf).
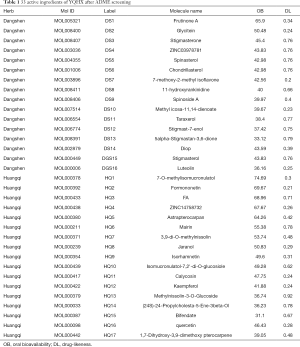
Full table
CHF- related targets
By screening the GeneCards and DisGeNET databases, 1,296 and 1,593 CHF-related genes were obtained, respectively. After removing duplications, 1,922 CHF-related genes were obtained (https://cdn.amegroups.cn/static/public/apm-21-1469-2.pdf).
Construction of the H-I-P-D network and screening out of key components
A total of 159 common targets between DHP and CHF were identified and displayed using a Venn diagram (Figure 2A, https://cdn.amegroups.cn/static/public/apm-21-1469-3.pdf). Next, we constructed the common targets network using Cytoscape 3.7.2 (Figure 2B). The common targets and the relevant active ingredients were input into Cytoscape 3.7.2 to construct the H-I-P-D network for visualization. The network comprised 193 nodes (including 32 active ingredients nodes, one disease node, one herb node, and 159 common targets nodes) and 853 edges (Figure 3). By using the Cytoscape Network Analyzer plug-in, the nodes were calculated based on the degree for topological analysis and sorted in descending order. The higher degree value, the more important corresponding ingredient was. Based on this principle, the top five nodes were screened out as key components, which included the following: 11-hydroxyrankinidine (degree =41), jaranol (degree =38), 7-methoxy-2-methylisoflavone (degree =37), astrapterocarpan (degree =36), and isorhamnetin (degree =36).
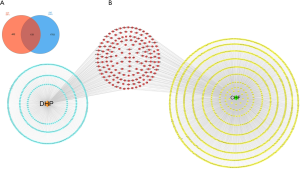
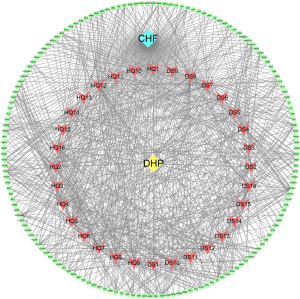
PPI network construction and hub genes screening
To reveal the mechanism of DHP in the treatment of CHF, a PPI network of the common targets was constructed using the STRING database, and was analyzed using the Cytoscape Network Analyzer and CytoNCA plug-ins for visualization (Figure 4). As shown in Figure 4A, the PPI network consisted of 119 nodes and 475 edges, which represented 119 interacting proteins and 475 interactions. Six topological parameters (“degree”, “BC”, “CC”, “EC”, “NC”, and “LAC”) were used as filters to screen the hub genes. The first threshold was degree >6, BC >41.52, CC >0.21, EC >0.028, NC >2.87, and LAC >2, which developed 30 nodes and 177 edges. These 30 key nodes were then further selected with the second threshold of degree >18, BC >326.22, CC >0.235, EC >0.147, NC >9.707, and LAC >6.238, and finally a total of six nodes and 13 edges were involved in the cluster network. These six nodes were identified as hub genes, which likely exert principal effects on therapeutic mechanisms, and were used in the subsequent molecular docking. The top six hub genes were PIK3CA (degree =40), PIK3R1 (degree =38), SRC (degree =37), HRAS (degree =32), MAPK1 (degree =32), and AKT1 (degree =31) (Table 2).
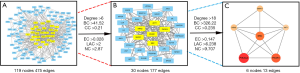
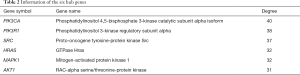
Full table
GO and KEGG pathway enrichment analysis
A total of 159 common targets were used to perform GO and KEGG pathway enrichment analyses using Metascape database and R software 3.6.3 for visualization.
According to the P value (P<0.05) and counts, the top 10 enriched BP terms, CC terms, and MF terms were displayed (Figure 5A and Table S1). The top 5 BP terms were as follows: positive regulation of kinase activity, cellular response to nitrogen compound, response to wounding, blood circulation, and response to inorganic substance. The top 5 CC terms were as follows: membrane raft, receptor complex, axon, lytic vacuole, and perinuclear region of cytoplasm. The top 5 MF terms were as follows: protein kinase activity, phosphatase binding, protein domain-specific binding, kinase binding, and protein homodimerization activity.
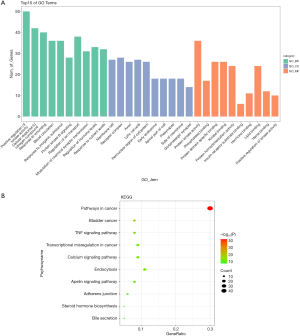
Furthermore, KEGG pathway analysis was also performed to identify pathways that exert a significant function on the therapeutic mechanism (Figure 5B and Table 3). The top 5 pathways included those in cancer, bladder cancer, the TNF signaling pathway, transcriptional misregulation in cancer, and the calcium signaling pathway. Based on the counts of targets involved in each pathway, a P-R network was established and analyzed using Cytoscape 3.7.2 (Figure 6). The P-R network comprised 97 nodes and 159 edges. The purple nodes represented the top 10 pathways, and the blue nodes represented the related-targets.
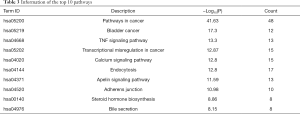
Full table
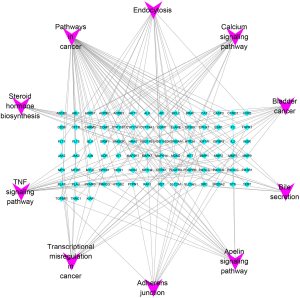
Molecular docking analysis
In the present study, we simulated the docking of six hub genes (PIK3CA, PIK3R1, SRC, HRAS, MAPK1, and AKT1) and five key ingredients of DHP (11-hydroxyrankinidine, jaranol, 7-methoxy-2-methylisoflavone, astrapterocarpan, and isorhamnetin) to assess the protein-ligand binding potential. The related proteins of PIK3CA, PIK3R1, SRC, HRAS, MAPK1, and AKT1 were obtained from PDB, and the PDB IDs were 6OAC, 5UBT, 1KSW, 2C5L, 6G9J, and 6HHH, respectively. Furthermore, given that previous experiments had shown that drugs such as buparlisib (34,35), LY294002 (36,37), dasatinib (38,39), FTI-277 (40), camptothecin (41,42), and 3,3'-diindolylmethane (43,44) could interact with these six hub genes, they were selected as the positive control group. Similar to the positive group (Table 4), the five key ingredients exhibited strong affinity with the six hub genes, and their average docking affinity was −7.37 kcal/mol, indicating strong binding energy (Table S2). For the targets PIK3CA, SRC, and HRAS, their affinity with most of the key components was higher than the corresponding positive drugs, and AKT1-11-hydroxyrankinidine exhibited the best binding activity. Taking this pair as an example, a small molecule ligand of 11-hydroxyrankinidine could potentially fit into the interface pocket of AKT1, and the details of the docking are displayed in Figure 7. The binding modes of the six hub genes with the key ingredients are displayed in Figure 7 and Table 5.
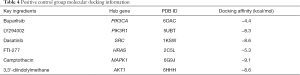
Full table
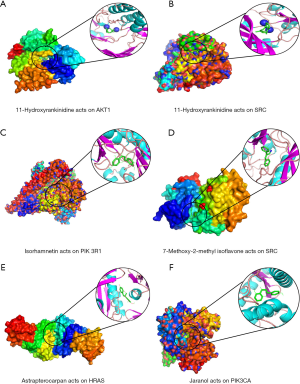

Full table
Discussion
As the major herb pairing for Qi-boosting in TCM, DHP plays an important role in the treatment of CHF. Clinical studies have shown that formulas with DHP as the main herb pairing exert significant therapeutic effects on CHF (9,13-15). Our previous experiment demonstrated that DHP improves heart function in a rat model of HF (after myocardial infarction caused by coronary artery ligation) through regulation of myocardial energy metabolism (16). However, the complex mechanism of DHP in the treatment of CHF has not been fully elucidated. In this study, we aimed to explore the molecular mechanism of DHP in CHF using network pharmacology and molecular docking technology.
In this study, five key ingredients of DHP were screened from the H-I-P-D network, including 11-hydroxyrankinidine, jaranol, 7-methoxy-2-methyl isoflavone, astrapterocarpan, and isorhamnetin. All of these belong to flavonoids, except for 11-hydroxyrankinidine, which is a kind of Gelsemium alkaloids (45), indicating that flavonoids are the main compounds of DHP in CHF treatment. Flavonoids can protect the cardiovascular system through anti-inflammatory, antioxidant, and anti-platelet aggregation effects (46,47). They can also reduce the downregulation of endothelial nitric oxide synthase (eNOS) and the level of reactive oxygen species (ROS), and increase the bioavailability of nitric oxide (NO), thereby improving endothelial function (48). Sun et al. reported that flavonoids extracted from propolis could reduce pathological myocardial hypertrophy via the PI3K/AKT signaling pathway (49). Endothelial dysfunction and cardiac hypertrophy are two important pathophysiological mechanisms, which serve as the main risk factors of CHF (50). Astrapterocarpan may improve endothelial dysfunction by inhibiting the activation of the MAPK3/1 signaling pathway (51). Isorhamnetin protects against endothelial dysfunction and cardiac hypertrophy via the PI3K/AKT pathway (52,53). Based on the results of topological analysis and literature retrieval, flavonoids may be the main compounds of DHP in the treatment of CHF. Numerous studies also shown that isorhamnetin may play an important role in CHF treatment.
After screening of the PPI network, six hub genes were identified, including PIK3CA, PIK3R1, SRC, HRAS, MAPK1, and AKT1. Phosphatidylinositol 3-kinases (PI3Ks) are involved in cellular functions such as cell proliferation, survival, growth, differentiation, and apoptosis (54). PIK3CA and PIK3R1 are the class I PIK3s. Inhibition of PIK3s can prevent many age-related changes in the heart and protect the heart function of aged mice (55). PIK3CA, PIK3R1, and AKT1 are associated with PI3K/AKT pathways. As mentioned above, isorhamnetin can improve endothelial dysfunction and cardiac hypertrophy via the PI3K/AKT pathway (52,53). The rat sarcoma (RAS) protein exists in cardiac myocytes as well as in cancer cells, and its high level of expression relates to numerous growth responses, such as promotion of cardiac hypertrophy, and is an important risk factor for CHF (56). HRAS, an isoform of Ras proteins, can regulate the PI3K-AKT signaling pathway and may be an important modulator of cardiac growth (57,58). It has been reported that the expression of the MAPK1 [also known as extracellular regulated protein kinase 2 (ERK2)] gene and protein increases rapidly in CHF rats, and the process of cardiac remodeling is delayed by inhibiting the expression of ERK2 (59). The ERK1/2 pathway is also closely related to myocardial hypertrophy and endothelial dysfunction (60,61). SRC is a non-receptor protein tyrosine kinase, and plays a key role in many cellular processes, including cell growth, proliferation, differentiation, and neuronal signal (62,63). SRC is involved in the PI3K/Akt and ERK1/2 signaling pathways, and plays an important role in the occurrence and development of endothelial dysfunction and cardiac hypertrophy (64-68). Therefore, DHP may alleviate endothelial dysfunction and myocardial hypertrophy via the PI3K/Akt or ERK1/2 signaling pathways, thereby improving CHF. PIK3CA, PIK3R1, SRC, HRAS, MAPK1, and AKT1 may also play critical roles in this process.
Meanwhile, the six hub genes and five key ingredients of DHP were investigated by molecular docking simulation. Compared to the positive group, the five key ingredients exhibited strong affinities to the six hub genes. Therefore, they can be explored as the main components of new natural medicines of DHP in the treatment of CHF in the future.
The top five pathways after KEGG enrichment analysis included pathways in cancer, bladder cancer, the TNF signaling pathway, transcriptional misregulation in cancer, and the calcium signaling pathway, which mainly relates to cancer, inflammation, and calcium regulation. Due to critical roles of SRC, HRAS, and the PI3K/Akt or ERK1/2 signaling pathway in the occurrence and development of tumors, this may explain why the enrichment results were closely related to cancer pathways (69-72). The TNF signaling pathway is associated with inflammation and is mainly involved in regulating immune cells. TNF can also mediate many downstream pathways, such as the PI3K/Akt or ERK1/2 signaling pathways, resulting in cardiac hypertrophy and endothelial dysfunction (73-76). A previous study showed that restricting the TNF-α can inhibit the process of CHF, which may become a novel way to treat CHF (77). The excitation-contraction coupling of cardiomyocytes is closely related to calcium ion (Ca2+) regulation. Abnormal Ca2+ handling results in impaired Ca2+ cycling that affect both systolic and diastolic functions, and is considered as an important mechanism of CHF (78,79). Our previous study found that Astragalus granules could improve cardiac function in HF mice caused by thoracic aortic constriction (TAC) by reversing Ca2+/calmodulin-dependent protein kinase II (CaMKII) overexpression-induced Ca2+ handling disorder (80).
The results of BP in the GO enrichment analysis were primarily associated with the positive regulation of kinase activity and the cellular response to nitrogen compound. Many kinases are involved in PI3K/Akt, ERK1/2, and calcium signaling pathways, such as AKT1, MAPK1, and CaMKII. Abnormal regulation of these kinases plays an important role in the development of CHF (81-83). Flavonoids can improve endothelial function by increasing the bioavailability of NO (48).
Above all, our network pharmacology results indicate that flavonoids may be the main compounds of DHP in the treatment of CHF. The role of isorhamnetin in CHF warrants further study. Firstly, the flavonoids in DHP may regulate the TNF pathway and act on hub genes to regulate TNF-mediated downstream PI3K/Akt or ERK1/2 signaling pathways, thereby alleviating endothelial dysfunction and cardiac hypertrophy and improving CHF. They may also improve excitation-contraction coupling by regulating the calcium signaling pathway (Figure 8). These pathways are all related to the BPs of positive regulation of kinase activity and cellular response to nitrogen compound. Furthermore, our molecular docking results showed that five key ingredients exhibited strong affinities to six hub genes.
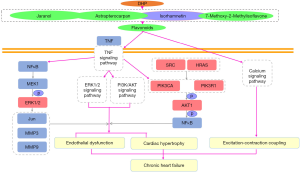
Conclusions
This study revealed the molecular mechanism of DHP in the treatment of CHF by utilizing network pharmacology and molecular docking. However, further experiments are required to conform these findings and provide insights for future research.
Acknowledgments
Funding: The authors were supported by grants from the project of National Natural Science Foundation of China (No. 81903993 and No. 81973622).
Footnote
Reporting Checklist: The authors have completed MDAR reporting checklist. Available at https://dx.doi.org/10.21037/apm-21-1469
Conflicts of Interest: All authors have completed the ICMJE uniform disclosure form (available at https://dx.doi.org/10.21037/apm-21-1469). The authors have no conflicts of interest to declare.
Ethical Statement: The authors are accountable for all aspects of the work in ensuring that questions related to the accuracy or integrity of any part of the work are appropriately investigated and resolved. This study was conducted in accordance with the Declaration of Helsinki (as revised in 2013).
Open Access Statement: This is an Open Access article distributed in accordance with the Creative Commons Attribution-NonCommercial-NoDerivs 4.0 International License (CC BY-NC-ND 4.0), which permits the non-commercial replication and distribution of the article with the strict proviso that no changes or edits are made and the original work is properly cited (including links to both the formal publication through the relevant DOI and the license). See: https://creativecommons.org/licenses/by-nc-nd/4.0/.
References
- Groenewegen A, Rutten FH, Mosterd A, et al. Epidemiology of heart failure. Eur J Heart Fail 2020;22:1342-56. [Crossref] [PubMed]
- Ponikowski P, Voors AA, Anker SD, et al. 2016 ESC Guidelines for the diagnosis and treatment of acute and chronic heart failure: The Task Force for the diagnosis and treatment of acute and chronic heart failure of the European Society of Cardiology (ESC). Developed with the special contribution of the Heart Failure Association (HFA) of the ESC. Eur J Heart Fail 2016;18:891-975. [Crossref] [PubMed]
- Cheng H, Wu X, Ni G, et al. Citri Reticulatae Pericarpium protects against isoproterenol-induced chronic heart failure via activation of PPARγ. Ann Transl Med 2020;8:1396. [Crossref] [PubMed]
- . GBD 2017 Disease and Injury Incidence and Prevalence Collaborators. Global, regional, and national incidence, prevalence, and years lived with disability for 354 diseases and injuries for 195 countries and territories, 1990–2017: a systematic analysis for the Global Burden of Disease Study 2017. Lancet 2018;392:1789-858. [Crossref] [PubMed]
- Benjamin EJ, Muntner P, Alonso A, et al. Heart Disease and Stroke Statistics-2019 Update: A Report From the American Heart Association. Circulation 2019;139:e56-e528. [Crossref] [PubMed]
- Hao G, Wang X, Chen Z, et al. Prevalence of heart failure and left ventricular dysfunction in China: the China Hypertension Survey, 2012-2015. Eur J Heart Fail 2019;21:1329-37. [Crossref] [PubMed]
- Hao P, Jiang F, Cheng J, et al. Traditional Chinese Medicine for Cardiovascular Disease: Evidence and Potential Mechanisms. J Am Coll Cardiol 2017;69:2952-66. [Crossref] [PubMed]
- Wang Y, Wang Q, Li C, et al. A Review of Chinese Herbal Medicine for the Treatment of Chronic Heart Failure. Curr Pharm Des 2017;23:5115-24. [PubMed]
- Li X, Zhang J, Huang J, et al. A multicenter, randomized, double-blind, parallel-group, placebo-controlled study of the effects of qili qiangxin capsules in patients with chronic heart failure. J Am Coll Cardiol 2013;62:1065-72. [Crossref] [PubMed]
- Wang J, Yang R, Zhang F, et al. The Effect of Chinese Herbal Medicine on Quality of Life and Exercise Tolerance in Heart Failure With Preserved Ejection Fraction: A Systematic Review and Meta-Analysis of Randomized Controlled Trials. Front Physiol 2018;9:1420. [Crossref] [PubMed]
- Liu X, Fu J, Fan T, et al. The Efficacy and Safety of Shen Guo Lao Nian Granule for Common Cold of Qi-Deficiency Syndrome: Study Protocol for a Randomized, Double-Blind, Placebo-Controlled, Multicenter, Phase II Clinical Trial. Evid Based Complement Alternat Med 2017;2017:1806461 [Crossref] [PubMed]
- Shu Q, Sun D, Wang H, et al. Differences of acupuncture and moxibustion on heart rate variability in qi-deficiency syndrome: a randomized controlled trial. Zhongguo Zhen Jiu 2017;37:25-30. [PubMed]
- Wang KH, Wu JR, Zhang D, et al. Comparative efficacy of Chinese herbal injections for treating chronic heart failure: a network meta-analysis. BMC Complement Altern Med 2018;18:41. [Crossref] [PubMed]
- Gao S, Zhang Q, Tian C, et al. The roles of Qishen granules recipes, Qingre Jiedu, Wenyang Yiqi and Huo Xue, in the treatment of heart failure. J Ethnopharmacol 2020;249:112372 [Crossref] [PubMed]
- Ren W, Gao S, Zhang H, et al. Decomposing the Mechanism of Qishen Granules in the Treatment of Heart Failure by a Quantitative Pathway Analysis Method. Molecules 2018;23:1829. [Crossref] [PubMed]
- Li Y, Wu Q, Lin Q. Effect of Radix Codonopsitis and Milkvetch Root on Hemodynamics in Chronic Heart Failure Rats. Chinese Journal of Basic Medicinein Traditional Chinese Medicine 2010;16:597-8.
- Li S, Zhang B. Traditional Chinese medicine network pharmacology: theory, methodology and application. Chinese Journal of Natural Medicines 2013;11:110-20. [Crossref] [PubMed]
- Saikia S, Bordoloi M. Molecular Docking: Challenges, Advances and its Use in Drug Discovery Perspective. Curr Drug Targets 2019;20:501-21. [Crossref] [PubMed]
- Ru J, Li P, Wang J, et al. TCMSP: a database of systems pharmacology for drug discovery from herbal medicines. J Cheminform 2014;6:13. [Crossref] [PubMed]
- Kim S, Chen J, Cheng T, et al. PubChem 2019 update: improved access to chemical data. Nucleic Acids Res 2019;47:D1102-D1109. [Crossref] [PubMed]
- Daina A, Michielin O, Zoete V. SwissTargetPrediction: updated data and new features for efficient prediction of protein targets of small molecules. Nucleic Acids Res 2019;47:W357-W364. [Crossref] [PubMed]
- Piñero J, Ramirez-Anguita JM, Sauch-Pitarch J, et al. The DisGeNET knowledge platform for disease genomics: 2019 update. Nucleic Acids Res 2020;48:D845-D855. [PubMed]
- Safran M, Dalah I, Alexander J, et al. GeneCards Version 3: the human gene integrator. Database (Oxford) 2010;2010:baq020 [Crossref] [PubMed]
- Szklarczyk D, Morris JH, Cook H, et al. The STRING database in 2017: quality-controlled protein-protein association networks, made broadly accessible. Nucleic Acids Res 2017;45:D362-D368. [Crossref] [PubMed]
- Zhou Y, Zhou B, Pache L, et al. Metascape provides a biologist-oriented resource for the analysis of systems-level datasets. Nat Commun 2019;10:1523. [Crossref] [PubMed]
- Ashburner M, Ball CA, Blake JA, et al. Gene ontology: tool for the unification of biology. The Gene Ontology Consortium. Nat Genet 2000;25:25-9. [Crossref] [PubMed]
- Kanehisa M, Furumichi M, Sato Y, et al. KEGG: integrating viruses and cellular organisms. Nucleic Acids Res 2021;49:D545-D551. [Crossref] [PubMed]
- Shannon P, Markiel A, Ozier O, et al. Cytoscape: a software environment for integrated models of biomolecular interaction networks. Genome Res 2003;13:2498-504. [Crossref] [PubMed]
- Tang Y, Li M, Wang J, et al. CytoNCA: a cytoscape plugin for centrality analysis and evaluation of protein interaction networks. Biosystems 2015;127:67-72. [Crossref] [PubMed]
- Kapetis D, Sassone J, Yang Y, et al. Network topology of NaV1.7 mutations in sodium channel-related painful disorders. BMC Syst Biol 2017;11:28. [Crossref] [PubMed]
- Burley SK, Berman HM, Bhikadiya C, et al. RCSB Protein Data Bank: biological macromolecular structures enabling research and education in fundamental biology, biomedicine, biotechnology and energy. Nucleic Acids Res 2019;47:D464-D474. [Crossref] [PubMed]
- Morris GM, Huey R, Lindstrom W, et al. AutoDock4 and AutoDockTools4: Automated docking with selective receptor flexibility. J Comput Chem 2009;30:2785-91. [Crossref] [PubMed]
- Seeliger D, de Groot BL. Ligand docking and binding site analysis with PyMOL and Autodock/Vina. J Comput Aided Mol Des 2010;24:417-22. [Crossref] [PubMed]
- Vasan N, Razavi P, Johnson JL, et al. Double PIK3CA mutations in cis increase oncogenicity and sensitivity to PI3Kα inhibitors. Science 2019;366:714-23. [Crossref] [PubMed]
- Nixon MJ, Formisano L, Mayer IA, et al. PIK3CA and MAP3K1 alterations imply luminal A status and are associated with clinical benefit from pan-PI3K inhibitor buparlisib and letrozole in ER+ metastatic breast cancer. NPJ Breast Cancer 2019;5:31. [Crossref] [PubMed]
- Mazumder AG, Kumari S, Singh D. Anticonvulsant action of a selective phosphatidylinositol-3-kinase inhibitor LY294002 in pentylenetetrazole-mediated convulsions in zebrafish. Epilepsy Res 2019;157:106207 [Crossref] [PubMed]
- Zheng S, Xiao L, Liu Y, et al. DZNep inhibits H3K27me3 deposition and delays retinal degeneration in the rd1 mice. Cell Death Dis 2018;9:310. [Crossref] [PubMed]
- Qian XL, Zhang J, Li PZ, et al. Dasatinib inhibits c-src phosphorylation and prevents the proliferation of Triple-Negative Breast Cancer (TNBC) cells which overexpress Syndecan-Binding Protein (SDCBP). PLoS One 2017;12:e0171169 [Crossref] [PubMed]
- Montero JC, Seoane S, Ocana A, et al. Inhibition of SRC family kinases and receptor tyrosine kinases by dasatinib: possible combinations in solid tumors. Clin Cancer Res 2011;17:5546-52. [Crossref] [PubMed]
- Lee KH, Koh M, Moon A. Farnesyl transferase inhibitor FTI-277 inhibits breast cell invasion and migration by blocking H-Ras activation. Oncol Lett 2016;12:2222-6. [Crossref] [PubMed]
- Lee S, Lee HS, Baek M, et al. MAPK signaling is involved in camptothecin induced cell death. Mol Cells 2002;14:348-54. [PubMed]
- Miltyk W, Karna E, Palka JA. Prolidase-independent mechanism of camptothecin-induced inhibition of collagen biosynthesis in cultured human skin fibroblasts. J Biochem 2007;141:287-92. [Crossref] [PubMed]
- Jin H, Park MH, Kim SM. 3,3'-Diindolylmethane potentiates paclitaxel-induced antitumor effects on gastric cancer cells through the Akt/FOXM1 signaling cascade. Oncol Rep 2015;33:2031-6. [Crossref] [PubMed]
- Lopez-Vazquez A, Garcia-Banuelos JJ, Gonzalez-Garibay AS, et al. IRS-1 pY612 and Akt-1/PKB pT308 Phosphorylation and Antiinflammatory Effect of Diindolylmethane in Adipocytes Cocultured with Macrophages. Med Chem 2017;13:727-33. [Crossref] [PubMed]
- Sun MX, Cui Y, Li Y, et al. Indole alkaloids from Gelsemium elegans. Phytochemistry 2019;162:232-40. [Crossref] [PubMed]
- Faggio C, Sureda A, Morabito S, et al. Flavonoids and platelet aggregation: A brief review. Eur J Pharmacol 2017;807:91-101. [Crossref] [PubMed]
- Vazhappilly CG, Ansari SA, Al-Jaleeli R, et al. Role of flavonoids in thrombotic, cardiovascular, and inflammatory diseases. Inflammopharmacology 2019;27:863-9. [Crossref] [PubMed]
- Yamagata K, Yamori Y. Inhibition of Endothelial Dysfunction by Dietary Flavonoids and Preventive Effects Against Cardiovascular Disease. J Cardiovasc Pharmacol 2020;75:1-9. [Crossref] [PubMed]
- Sun GW, Qiu ZD, Wang WN, et al. Flavonoids Extraction from Propolis Attenuates Pathological Cardiac Hypertrophy through PI3K/AKT Signaling Pathway. Evid Based Complement Alternat Med 2016;2016:6281376 [Crossref] [PubMed]
- Zuchi C, Tritto I, Carluccio E, et al. Role of endothelial dysfunction in heart failure. Heart Fail Rev 2020;25:21-30. [Crossref] [PubMed]
- Ohkawara S, Okuma Y, Uehara T, et al. Astrapterocarpan isolated from Astragalus membranaceus inhibits proliferation of vascular smooth muscle cells. Eur J Pharmacol 2005;525:41-7. [Crossref] [PubMed]
- Gong G, Guan YY, Zhang ZL, et al. Isorhamnetin: A review of pharmacological effects. Biomed Pharmacother 2020;128:110301 [Crossref] [PubMed]
- Gao L, Yao R, Liu Y, et al. Isorhamnetin protects against cardiac hypertrophy through blocking PI3K-AKT pathway. Mol Cell Biochem 2017;429:167-77. [Crossref] [PubMed]
- Aoyagi T, Matsui T. Phosphoinositide-3 kinase signaling in cardiac hypertrophy and heart failure. Curr Pharm Des 2011;17:1818-24. [Crossref] [PubMed]
- Inuzuka Y, Okuda J, Kawashima T, et al. Suppression of phosphoinositide 3-kinase prevents cardiac aging in mice. Circulation 2009;120:1695-703. [Crossref] [PubMed]
- Hunter JJ, Chien KR. Signaling pathways for cardiac hypertrophy and failure. N Engl J Med 1999;341:1276-83. [Crossref] [PubMed]
- Matsuda T, Jeong JI, Ikeda S, et al. H-Ras Isoform Mediates Protection Against Pressure Overload-Induced Cardiac Dysfunction in Part Through Activation of AKT. Circ Heart Fail 2017;10:e003658 [Crossref] [PubMed]
- Thorburn A, Thorburn J, Chen SY, et al. HRas-dependent pathways can activate morphological and genetic markers of cardiac muscle cell hypertrophy. J Biol Chem 1993;268:2244-9. [Crossref] [PubMed]
- Brietz A, Schuch KV, Wangorsch G, et al. Analyzing ERK 1/2 signalling and targets. Mol Biosyst 2016;12:2436-46. [Crossref] [PubMed]
- Souza DS, Barreto TO, Menezes-Filho JER, et al. Myocardial hypertrophy is prevented by farnesol through oxidative stress and ERK1/2 signaling pathways. Eur J Pharmacol 2020;887:173583 [Crossref] [PubMed]
- Campos-Mota GP, Navia-Pelaez JM, Araujo-Souza JC, et al. Role of ERK1/2 activation and nNOS uncoupling on endothelial dysfunction induced by lysophosphatidylcholine. Atherosclerosis 2017;258:108-18. [Crossref] [PubMed]
- Ingley E. Src family kinases: regulation of their activities, levels and identification of new pathways. Biochim Biophys Acta 2008;1784:56-65. [Crossref] [PubMed]
- Roskoski R Jr. Src protein-tyrosine kinase structure and regulation. Biochem Biophys Res Commun 2004;324:1155-64. [Crossref] [PubMed]
- Nemoto S, Kobayashi T, Taguchi K, et al. Losartan improves aortic endothelium-dependent relaxation via proline-rich tyrosine kinase 2/Src/Akt pathway in type 2 diabetic Goto-Kakizaki rats. Am J Physiol Heart Circ Physiol 2011;301:H2383-94. [Crossref] [PubMed]
- Abeyrathna P, Su Y. The critical role of Akt in cardiovascular function. Vascul Pharmacol 2015;74:38-48. [Crossref] [PubMed]
- Takeishi Y, Huang Q, Abe J, et al. Src and multiple MAP kinase activation in cardiac hypertrophy and congestive heart failure under chronic pressure-overload: comparison with acute mechanical stretch. J Mol Cell Cardiol 2001;33:1637-48. [Crossref] [PubMed]
- Hao HF, Liu LM, Pan CS, et al. Rhynchophylline Ameliorates Endothelial Dysfunction via Src-PI3K/Akt-eNOS Cascade in the Cultured Intrarenal Arteries of Spontaneous Hypertensive Rats. Front Physiol 2017;8:928. [Crossref] [PubMed]
- García-Redondo AB, Briones AM, Martinez-Revelles S, et al. c-Src, ERK1/2 and Rho kinase mediate hydrogen peroxide-induced vascular contraction in hypertension: role of TXA2, NAD(P)H oxidase and mitochondria. J Hypertens 2015;33:77-87. [Crossref] [PubMed]
- Massari F, Ciccarese C, Santoni M, et al. Metabolic phenotype of bladder cancer. Cancer Treat Rev 2016;45:46-57. [Crossref] [PubMed]
- Zhou B, Der CJ, Cox AD. The role of wild type RAS isoforms in cancer. Semin Cell Dev Biol 2016;58:60-9. [Crossref] [PubMed]
- Sharma VR, Gupta GK, Sharma AK, et al. PI3K/Akt/mTOR Intracellular Pathway and Breast Cancer: Factors, Mechanism and Regulation. Curr Pharm Des 2017;23:1633-8. [Crossref] [PubMed]
- Wang K, Ji W, Yu Y, et al. FGFR1-ERK1/2-SOX2 axis promotes cell proliferation, epithelial-mesenchymal transition, and metastasis in FGFR1-amplified lung cancer. Oncogene 2018;37:5340-54. [Crossref] [PubMed]
- Zhang X, Yang K, Zhang H, et al. Effect of typhaneoside on ventricular remodeling and regulation of PI3K/Akt/mTOR pathway. Herz 2020;45:113-22. [Crossref] [PubMed]
- Liu Y, Tie L. Apolipoprotein M and sphingosine-1-phosphate complex alleviates TNF-alpha-induced endothelial cell injury and inflammation through PI3K/AKT signaling pathway. BMC Cardiovasc Disord 2019;19:279. [Crossref] [PubMed]
- Kanaji N, Nelson A, Wang X, et al. Differential roles of JNK, ERK1/2, and p38 mitogen-activated protein kinases on endothelial cell tissue repair functions in response to tumor necrosis factor-alpha. J Vasc Res 2013;50:145-56. [Crossref] [PubMed]
- Zhang C, Zhou G, Chen Y, et al. Human umbilical cord mesenchymal stem cells alleviate interstitial fibrosis and cardiac dysfunction in a dilated cardiomyopathy rat model by inhibiting TNFalpha and TGFbeta1/ERK1/2 signaling pathways. Mol Med Rep 2018;17:71-8. [PubMed]
- Chen C, Zong M, Lu Y, et al. Differentially expressed lnc-NOS2P3-miR-939-5p axis in chronic heart failure inhibits myocardial and endothelial cells apoptosis via iNOS/TNFalpha pathway. J Cell Mol Med 2020;24:11381-96. [Crossref] [PubMed]
- Cho GW, Altamirano F, Hill JA. Chronic heart failure: Ca(2+), catabolism, and catastrophic cell death. Biochim Biophys Acta 2016;1862:763-77. [Crossref] [PubMed]
- Bers DM. Cardiac sarcoplasmic reticulum calcium leak: basis and roles in cardiac dysfunction. Annu Rev Physiol 2014;76:107-27. [Crossref] [PubMed]
- Li S, Nong Y, Gao Q, et al. Astragalus Granule Prevents Ca(2+) Current Remodeling in Heart Failure by the Downregulation of CaMKII. Evid Based Complement Alternat Med 2017;2017:7517358 [Crossref] [PubMed]
- Zhou H, Dickson ME, Kim MS, et al. Akt1/protein kinase B enhances transcriptional reprogramming of fibroblasts to functional cardiomyocytes. Proc Natl Acad Sci U S A 2015;112:11864-9. [Crossref] [PubMed]
- Naguib YW, Yu Y, Wei SG, et al. An Injectable Microparticle Formulation Provides Long-Term Inhibition of Hypothalamic ERK1/2 Activity and Sympathetic Excitation in Rats with Heart Failure. Mol Pharm 2020;17:3643-8. [Crossref] [PubMed]
- Pyun JH, Kim HJ, Jeong MH, et al. Cardiac specific PRMT1 ablation causes heart failure through CaMKII dysregulation. Nat Commun 2018;9:5107. [Crossref] [PubMed]
(English Language Editor: A. Kassem)