Comparison of the community-acquired pneumonia and COVID-19 at the early stage: findings from two cohort studies
Introduction
Coronavirus disease-19 (COVID-19) caused by a novel beta-coronavirus has become a public health emergency of global concern, with severe cases leading to pneumonia, kidney failure, and even death, as well as bringing unexpected disasters and changes to human society (1-3). Full understanding and accurate diagnosis of COVID-19 development are key measures for its prevention and treatment.
Researchers all over the world have used real-time polymerase chain reaction (qRT-PCR), radiological features and epidemiological linkage to establish a clinical diagnosis method for COVID-19, with initial success, but many issues still need to be explored (4-6). (I) There remains much research into accurate diagnosis: RT-PCR is used to detect SARS-CoV-2 nucleotides from specimens such as oropharyngeal swab, nasopharyngeal swab, bronchoalveolar lavage, or tracheal aspirate. However, recent reports have revealed that RT-PCR produces unavoidable false negatives of 29–40% for detecting COVID-19 (7,8). (II) As far as radiological diagnostic value is concerned, the features of COVID-19 are often rather nonspecific (9). (III) As for treatment, biochemical indicators and other laboratory examination findings are the important keys to diagnosis, which in turn will optimize the treatment strategy. However, studies in this area have been scarce till now.
With regard to the laboratory examination, fortunately some studies had compared hematological and biochemical indexes of COVID-19patients with those of CAP patients, and the results showed that 9 laboratory parameters are highly predictive of a diagnosis of COVID-19 by multivariate analysis, including red blood cell distribution width (RDW), HGB, red blood cell count (RBC), albumin (ALB), eosinophil (EO), hematocrit (HCT), alkaline phosphatase (ALP), white blood cell count (WBC) and C-reactive protein (CRP) (10,11). However, laboratory parameters are influenced by variant variables, such as the host, environment, diet and stage of disease (12,13). To understand COVID-19 fully, continuous comparative studies among different cohorts are still necessary to analyze the relationships between these experimental indicators.In this study, 40 patients with early-stage COVID-19, 30 patients with CAP and 31 healthy people were recruited, and 48 different biochemical indexes were compared and analysed by bioinformatics and statistics to explore correlations among laboratory variables that might be an auxiliary diagnostic index and discriminate COVID-19 patients from CAP patients. Although many studies have been carried out on COVID-19 recently, the study on the difference of the early stage of COVID-19 and CAP is not clear. Based on the findings of the two cohorts, the present study focused on the difference between covid-19 and CAP for the first time. We present the following article in accordance with the STARD reporting checklist (available at https://dx.doi.org/10.21037/apm-21-2006).
Methods
Patients
We conducted a retrospective case sheet review of 40 patients with COVID-19, 30 patients with CAP admitted to Lanzhou Chest Hospital and 32 healthy subjects undergoing physical examination from Gansu People Hospital. Patients with COVID-19were confirmed to have SARS-CoV-2 by a positive result of RT-PCR. For patients with CAP, the diagnostic criteria were based on the 2017 Guidelines for the diagnosis and the treatment of community-acquired pneumonia (CAP) issued by the Chinese Society of Infectious Diseases and Parasitology. Patients were excluded if they had been diagnosed with severe pneumonia, critical pneumonia and comorbidity such as autoimmune disease, other infectious diseases, hepatorenal syndrome, or cardiovascular and cerebrovascular diseases.
The study was conducted in accordance with the Declaration of Helsinki (as revised in 2013). All the steps have been approved by the ethics committee of the First Hospital of Lanzhou University (No. LDYYLL2020-33). Because of the retrospective nature of the study, the requirement for informed consent was waived.
Data
Age, sex, hematological and biochemical laboratory parameters at presentation were recorded. Only those with laboratory parameters obtained at the time of admission were enrolled before initiation of therapy. A total of 48 laboratory variables were included in the data analysis. Clinical data collection from the patients with COVID-19 and CAP and the healthy subjects was approved by the Gansu people Hospital Ethics Committee.
Statistical analysis
In the univariate analysis, the independent Student’s t-test was performed to compare the mean laboratory values. For data that were not normally distributed, we used a natural logarithmic transformation to normalize the distribution. We used Fisher’s exact test and/or Chi-square test to compare the proportions of patients with abnormal readings between groups of patients. Logistic regression models were used for multivariate analysis to determine the predictive and thus the distinguishing parameters between patients with COVID-19 and those with CAP. The area under the curve (AUC) from the receiver operating characteristic (ROC) curve was used to evaluate the model’s ability to diagnose COVID-19 patients and discriminate between COVID-19 and CAP patients. Odds ratios (ORs) and their 95% confidence intervals (CIs) were provided as estimates of effect size. A principal component analysis (PCA) was performed based on the Bray-Curtis distance matrix across the samples using the vegan package in R (version 3.4.4). Student’s t-test was performed to compare PC1/PC2 between groups using the stats package in R. Spearman’s correlation between laboratories variables was calculated using the Hmisc package in R. The correlation networks between laboratory variables were constructed using the Gene Net package in R, and further visualized using Cytoscape 3.4.0. The correlation matrices between laboratory variables were computed by the Kruskal test, Procrustes (PROTEST) test, and correlation matrix using the vegan and CCA package in R.
Results
Baseline characteristics
Descriptive statistics of the 40 patients with COVID-19, 30 patients with CAP and 32 healthy controls enrolled in this cohort study are summarized in Table S1. There were not no significant differences among groups in terms of age (P=0.478) and sex (P=0.579), which indicated that the distribution of sampling was balanced for age and sex.
AUC value of different laboratory variables and their combinations based on comparison of the COVID-19 group versus the Healthy and CAP groups
We used 14 and 7 different laboratory variables, yielded from a comparison of the COVID-19 group versus healthy controls and the COVID-19 group versus the CAP group, respectively (Tables 1,2) (P<0.05) to evaluate the AUC values of the individual variables and their combinations. ROC analysis was performed and the area under the curve showed the performance of hematological variables (Figures 1,2). Regarding the AUC value of single laboratory variables, LDH (P=0.635), CRP (P=0.783) and CK-MB (P=0.73) showed significant prediction probability compared with healthy controls, and CK-MB (P=0.825) and K (P=0.767) showed significant prediction probability compared with the CAP group. For the AUC values of combinations of laboratory variables, “LY + EO + PLT” (P=0.847) and “EO + WBC + NEUT” (P=0.74) of the hematological variables showed more significant prediction probability in the comparison of the COVID-19 group versus healthy controls and CAP group; “LDH + CK-MB + CRP” (P=0.854) and “CK-MB + K” (P=0.872) among the biochemical variables showed more significant prediction probability in the comparison of the COVID-19 patient group versus healthy controls and the CAP group. These results indicated that laboratory variables may have obvious value with different specificity and sensitivity for COVID-19 diagnosis and discrimination from CAP, and that combinations of laboratory variables can improve the prediction probability.
Table 1
Variables | Subtypes | COVID-19 (n=40) | Healthy controls (n=32) | P value | AUC |
---|---|---|---|---|---|
Hematological | LY (109/L) | 1.31±0.76 | 2.15±0.64 | 0 | 0.843 |
PLT (109/L) | 191.35±58.98 | 233.38±68.03 | 0.006 | 0.527 | |
EO (109/L) | 0.40±0.63 | 0.13±0.10 | 0.012 | 0.663 | |
LY + EO + PLT | 0.847 | ||||
Biochemical | CRP (mg/L) | 20.5±34.95 | 2.40±2.78 | 0.002 | 0.783 |
TP (g/L) | 64.47±7.25 | 72.65±3.64 | 0 | 0.696 | |
ALB (g/L) | 41.05±4.97 | 43.70±2.83 | 0.006 | 0.620 | |
Glb (g/L) | 26.42±3.69 | 29.61±3.32 | 0 | 0.743 | |
UA (mol/L) | 284.60±102.52 | 356.03±85.68 | 0.002 | 0.723 | |
P (mmol/L) | 1.02±0.29 | 1.15±0.25 | 0.038 | 0.628 | |
LDH (U/L) | 217.40±129.22 | 168.19±37.65 | 0.027 | 0.635 | |
CK-MB (U/L) | 15.17±5.53 | 11.37±3.30 | 0.001 | 0.730 | |
Cl (mmol/L) | 102.58±3.69 | 107.95±2.32 | 0 | 0.896 | |
Mg (mmol/L) | 0.72±0.20 | 0.85±0.09 | 0.001 | 0.687 | |
HCY (μmol/L) | 12.04±9.16 | 16.28±6.78 | 0.032 | 0.736 | |
LDH + CK-MB + CRP | 0.854 |
AUC, area under the curve; COVID-19, coronavirus disease-19; LY, lymphocyte count; PLT, platelets; EO, eosinophil; CRP, C-reactive protein; TP, total protein; ALB, albumin; Glb, globulin; UA, uric acid; P, phosphorus; LDH, lactate; CK-MB, creatine kinase isoenzyme; Cl, chlorine; Mg, magnesium; HCY, homocysteine.
Table 2
Variables | Subtypes | COVID-19 (n=40) | CAP (n=32) | P value | AUC |
---|---|---|---|---|---|
Hematological | WBC (109/L) | 5.15±2.35 | 7.30±3.78 | 0.009 | 0.690 |
EO (109/L) | 0.40±0.63 | 0.07±0.07 | 0.002 | 0.581 | |
NEUT (109/L) | 3.60±2.76 | 5.48±3.43 | 0.006 | 0.727 | |
EO + WBC + NEUT | 0.740 | ||||
Biochemical | CK-MB (U/L) | 15.17±5.53 | 10.04±4.02 | 0 | 0.825 |
K (mmol/L) | 4.30±0.61 | 3.84±0.31 | 0 | 0.767 | |
Mg (mmol/L) | 0.70±0.20 | 0.89±0.12 | 0 | 0.744 | |
HCY (μmol/L) | 12.04±9.16 | 20.43±12.94 | 0.002 | 0.755 | |
CK-MB + K | 0.872 |
AUC, area under the curve; COVID-19, coronavirus disease-19; CAP, community-acquired pneumonia; WBC, white blood cell count; EO, eosinophil; NEUT, neutrophil count; CK-MB, creatine kinase isoenzyme; Mg, magnesium; HCY, homocysteine.
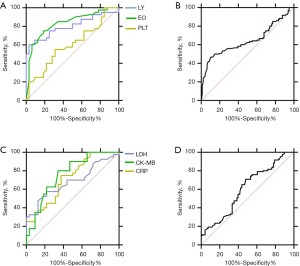
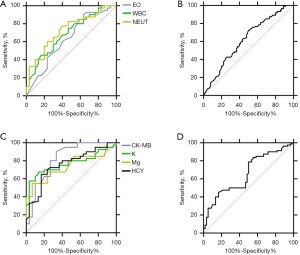
PCA of laboratory variables based on their values, heatmap and Procrustes analysis
We investigated the relationships among the levels of the laboratory variables in the three groups by PCA based on the Bray-Curtis distance to explore the characteristics of the metabolism and activity of the variables under the conditions of COVID-19 infection. The data showed that the COVID-19 and CAP generally overlapped, and had to be separated from the healthy controls (Figure 3). At the same time, it can be seen from the area diagram of the whole group that the area of the COVID-19 patients and healthy controls is smaller than that for CAP and appears to be concentrated. In order to observe the variation of the values of the laboratory indexes, we used Procrustes (PROTEST) test and k test to compare the laboratory variables’ values, the heatmap and Kruskal test showed no significant factor change among the three groups (Figure 4A), and the interactions among the laboratory variables were more complex in the COVID-19 group than in the other two groups (Figure 4B-4D).
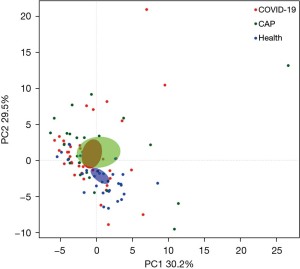
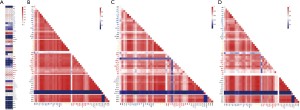
Correlations between laboratory variables
To further understand the differences in laboratory variables among the three groups, Spearman’s correlations were used to explore correlations among laboratory variables (Figure 5A-5C). In the healthy control group, the interactions among the laboratory variables contain more interactions between 2 indexes, such as ALT-AST, NRBC-NRBC% and LY%-NUET%, etc. There was just one interaction among 4 indexes: Glu-TBIL-DBIL-IBIL. In the CAP group, there were more complex interactions among indexes than in the healthy control group: there were 5 group interactions among 3 indexes: DBIL-TBIL-IBIL, Glb-TP-ALB, EO%-EO-MO, PCT-CK-MB-P, RBC-Hb-Hct and there was no interactions among more than 3 indexes; There were4 interactions of at least 4 indexes in the COVID-19 group: Na-CRP-Glu-Ur-LDH-sex, MCHC-MCH-MCV-RBC-Hct-Hb, ALB-TP-Glb-BO%-Ca, PDW-MPV-PLT-PCT, and 3 interactions among 3 indexes: LY-NUET%-LY%, CK-MB-CREA-Ua and DBIL-TBIL-IBIL. These results demonstrated that compared with the healthy and CAP groups, the laboratory variables had a variety of more intricate linkages in COVID-19.
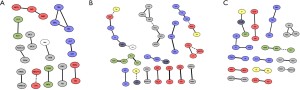
Discussion
The prevalence and fatality rate of COVID-19 are rapidly increasing but the medication options are still limited for this perilous disease. Advances in prevention and effective management of COVID-19 will require basic and clinical investigation and public health and clinical interventions. The pathogenesis of the new coronavirus is still not well defined. In the present study, we focused on the correlations among laboratory variables in COVID-19 and the differences from CAP and healthy controls. We found these correlations were more complex in the COVID-19 group than in the other two groups, and the changes in the correlations and the differences in laboratory variables involved may contribute to the multiple organ injury, especially the symptom of hypoxia during infection or development of COVID-19. These findings may have great clinical significance and value for detecting COVID-19 and raising the index of suspicion of COVID-19.
CAP refers to the inflammation of infectious lung parenchyma (including alveolar wall, i.e., lung stroma in a broad sense) outside the hospital, including pathogen infection with clear incubation period and pneumonia occurring in the incubation period after admission. Once the etiological results of CAP are obtained, targeted treatment with antibiotic drugs can be carried out with reference to the results of in vitro drug sensitivity test. For the pediatric patients with COVID-19, they may experience milder illness with atypical clinical manifestations and rare lymphopenia. High incidence of critical illness and vomiting symptoms reward attention in children under 1 year old (14). Viral pneumonia induced by non-new coronavirus mostly causes interstitial lung disease, which is more common in children, such as acute onset, continuous progress of respiratory symptoms, and high fever.
Firstly, we calculated the different single indexes with higher AUC value yielded from a comparison of the COVID-19 group versus the healthy controls and CAP group respectively (P<0.05). The elevated CRP in patients with COVID-19 than in healthy controls was consistent with previous studies (15), indicating that CRP was a core indicator of the inflammatory state in the body and might be a potential and reliable predictor of COVID-19 prognosis (16). We confirmed the data in other report that LDH was relatively higher in COVID-19 patients than in healthy controls (17), which is a biomarker of cardiovascular disease. The elevated LDH in COVID-19 conformed reports that suggested many patients with COVID 19 have accompanying heart damage (18-20). When we distinguished COVID-19 from CAP, though it is difficult based only on clinical and epidemiological evaluations, our results for the laboratory variables showed higher K levels in COVID-19 than CAP, indicating that an electrolyte imbalance is associated with the pathophysiology of COVID-19 and should be measured at initial presentation and serially monitored during hospitalization in order to establish timely and appropriate corrective actions (21). Strikingly, the higher CK-MB in COVID-19 cases was indicative of possible cardiac injury and highlights the importance of monitoring cardiac biomarkers in COVID-19 patients. Secondly, we tested the AUC value of combinations of laboratory variables and it is worth noting that the combinations of “LY + EO + PLT” (AUC =0.847), “LDH + CK-MB + CRP” (AUC =0.854), “EO + WBC + NEUT” (AUC =0.74), and “CK-MB + K” (AUC =0.872) showed more significant prediction probability for distinguishing COVID-19 from the healthy controls and CAP group. Taken together, application of this information with detailed epidemiological clues may help to avoid missing and misdiagnosis of COVID-19 patients, and shorten the time of isolation of patients with mild symptoms until a full diagnostic evaluation is completed.
Thirdly, we used PCA based on the Bray-Curtis distance and heatmap and Procrustes analyses to observe the relationships among the laboratory variables in the three groups with an omics view. The data indicated that the correlations among the laboratory variables may be a typical characteristic of COVID-19 and different from healthy controls but overlapped with CAP in overall performance. Furthermore, we used correlation network analysis to excavate the kinds of correlation-laboratory variables, and the data further confirmed that the correlations among laboratory variables were more complex in the COVID-19 group than in the other two groups. All these interactions may result in the complex organ damage of COVID-19. It is worth noting that COVID-19 targets many organs and tissues, such as the salivary glands, liver, kidneys, lungs and even the heart in the early stage. Once these organs are damaged, they will show their clinical characteristics in the physical and chemical hematological variables. Thus, laboratory variables in COVID-19 are significantly more complex than in CAP patients and health controls, which may be considered as one of the characteristics of COVID-19.
As for the composition of the correlation-laboratory variables in the COVID-19 group, multiple laboratory variables presented significant correlations that might indicate the multi-organ damage caused by COVID-19. Lung damage, the most common and earliest complication of COVID-19 infection, causes a series of stress reactions in the circulation of blood oxygen (22,23), which might explain why MCHC-MCH-MCV-RBC-Hct-Hb was the most typical network of correlation-laboratory variables in the present study. Beyond that, COVID-19 infection may damage the liver, kidneys, and heart muscle (24,25). Inflammation is closely related to coagulation function. Excessive inflammatory reaction causes intravascular thrombosis and increased capillary permeability. The coagulation function of alveoli and alveolar septum in patients with pneumonia is unbalanced. In our study, ALB-TP-Glb-BO%-Ca, CK-MB-CREA-Ua and DBIL-TBIL-IBIL were typical interconnected networks of laboratory variables. These interconnected networks were indicators of multiple organ damage and should be considered during COVID-19 diagnosis and surveillance.
In conclusion, the result of the present study suggested that the correlations and linkages among the laboratory variables in the COVID-19 group showed unique characteristics and the combined patterns of laboratory variables may have obvious value with different specificity and sensitivity for prediction probability of COVID-19. In managing epidemiologically high-risk patients with CAP, but without an immediate alternative diagnosis, certain routine laboratory findings may raise the index of suspicion of COVID-19.
Acknowledgments
The authors thank anonymous reviewers who helped in improving the article by their valuable comments. We also appreciate the study supported by the Fundamental Research Funds for the Central Universities and Project of the platform for innovation and service of Gansu Province.
Funding: We also thank the grants of the National Natural Science Foundation (grants No. 81860372, 81800528, and 31860598), Gansu Provincial COVID-19 Science and Technology Major Project, China (20YF2FA034, 20YF2FA011). Gansu Provincial Natural Science Foundation Project (20JR0RA683, 20JR5RA364), Lanzhou University First Hospital Intra-hospital Fund (ldyyyn2018-41).
Footnote
Reporting Checklist: The authors have completed the STARD reporting checklist. Available at https://dx.doi.org/10.21037/apm-21-2006
Data Sharing Statement: Available at https://dx.doi.org/10.21037/apm-21-2006
Conflicts of Interest: All authors have completed the ICMJE uniform disclosure form (available at https://dx.doi.org/10.21037/apm-21-2006). The authors have no conflicts of interest to declare.
Ethical Statement: The authors are accountable for all aspects of the work in ensuring that questions related to the accuracy or integrity of any part of the work are appropriately investigated and resolved. The study was conducted in accordance with the Declaration of Helsinki (as revised in 2013). All the steps used in the study have been approved by the ethics committee of the First Hospital of Lanzhou University (No. LDYYLL2020-33). Because of the retrospective nature of the study, the requirement for informed consent was waived.
Open Access Statement: This is an Open Access article distributed in accordance with the Creative Commons Attribution-NonCommercial-NoDerivs 4.0 International License (CC BY-NC-ND 4.0), which permits the non-commercial replication and distribution of the article with the strict proviso that no changes or edits are made and the original work is properly cited (including links to both the formal publication through the relevant DOI and the license). See: https://creativecommons.org/licenses/by-nc-nd/4.0/.
References
- Cao L, Zhang S, Luo X, et al. Myocardium injury biomarkers predict prognosis of critically ill coronavirus disease 2019 (COVID-19) patients. Ann Palliat Med 2020;9:4156-65. [Crossref] [PubMed]
- Chan JF, Yuan S, Kok KH, et al. A familial cluster of pneumonia associated with the 2019 novel coronavirus indicating person-to-person transmission: a study of a family cluster. Lancet 2020;395:514-23. [Crossref] [PubMed]
- Zhu N, Zhang D, Wang W, et al. A Novel Coronavirus from Patients with Pneumonia in China, 2019. N Engl J Med 2020;382:727-33. [Crossref] [PubMed]
- Shi F, Yu Q, Huang W, et al. 2019 Novel Coronavirus (COVID-19) Pneumonia with Hemoptysis as the Initial Symptom: CT and Clinical Features. Korean J Radiol 2020;21:537-40. [Crossref] [PubMed]
- Wang P, Anderson N, Pan Y, et al. The SARS-CoV-2 Outbreak: Diagnosis, Infection Prevention, and Public Perception. Clin Chem 2020; [Crossref] [PubMed]
- Ai T, Yang Z, Hou H, et al. Correlation of Chest CT and RT-PCR Testing for Coronavirus Disease 2019 (COVID-19) in China: A Report of 1014 Cases. Radiology 2020;296:E32-40. [Crossref] [PubMed]
- Liu R, Han H, Liu F, et al. Positive rate of RT-PCR detection of SARS-CoV-2 infection in 4880 cases from one hospital in Wuhan, China, from Jan to Feb 2020. Clin Chim Acta 2020;505:172-5. [Crossref] [PubMed]
- Fang Y, Zhang H, Xie J, et al. Sensitivity of Chest CT for COVID-19: Comparison to RT-PCR. Radiology 2020;296:E115-7. [Crossref] [PubMed]
- Kanne JP, Little BP, Chung JH, et al. Essentials for Radiologists on COVID-19: An Update-Radiology Scientific Expert Panel. Radiology 2020;296:E113-4. [Crossref] [PubMed]
- Skevaki C, Fragkou PC, Cheng C, et al. Laboratory characteristics of patients infected with the novel SARS-CoV-2 virus. J Infect 2020;81:205-12. [Crossref] [PubMed]
- Pan Y, Ye G, Zeng X, et al. Can routine laboratory tests discriminate SARS-CoV-2-infected pneumonia from other causes of community-acquired pneumonia? Clin Transl Med 2020;10:161-8. [Crossref] [PubMed]
- Saathoff E, Schneider P, Kleinfeldt V, et al. Laboratory reference values for healthy adults from southern Tanzania. Trop Med Int Health 2008;13:612-25. [Crossref] [PubMed]
- Kibaya RS, Bautista CT, Sawe FK, et al. Reference ranges for the clinical laboratory derived from a rural population in Kericho, Kenya. PLoS One 2008;3:e3327 [Crossref] [PubMed]
- Cui X, Zhao Z, Zhang T, et al. A systematic review and meta-analysis of children with coronavirus disease 2019 (COVID-19). J Med Virol 2021;93:1057-69. [Crossref] [PubMed]
- Li R, Tian J, Yang F, et al. Clinical characteristics of 225 patients with COVID-19 in a tertiary Hospital near Wuhan, China. J Clin Virol 2020;127:104363 [Crossref] [PubMed]
- Liu YP, Li GM, He J, et al. Combined use of the neutrophil-to-lymphocyte ratio and CRP to predict 7-day disease severity in 84 hospitalized patients with COVID-19 pneumonia: a retrospective cohort study. Ann Transl Med 2020;8:635. [Crossref] [PubMed]
- Chen L, Liu HG, Liu W, et al. Analysis of clinical features of 29 patients with 2019 novel coronavirus pneumonia. Zhonghua Jie He He Hu Xi Za Zhi 2020;43:203-8. [PubMed]
- Chen C, Chen C, Yan JT, et al. Analysis of myocardial injury in patients with COVID-19 and association between concomitant cardiovascular diseases and severity of COVID-19. Zhonghua Xin Xue Guan Bing Za Zhi 2020;48:567-71. [PubMed]
- Cui Y, Tian M, Huang D, et al. A 55-Day-Old Female Infant Infected With 2019 Novel Coronavirus Disease: Presenting With Pneumonia, Liver Injury, and Heart Damage. J Infect Dis 2020;221:1775-81. [Crossref] [PubMed]
- Liu Y, Yang Y, Zhang C, et al. Clinical and biochemical indexes from 2019-nCoV infected patients linked to viral loads and lung injury. Sci China Life Sci 2020;63:364-74. [Crossref] [PubMed]
- Lippi G, South AM, Henry BM. Electrolyte imbalances in patients with severe coronavirus disease 2019 (COVID-19). Ann Clin Biochem 2020;57:262-5. [Crossref] [PubMed]
- Xu Q, Jiang X, Wang T, et al. Study on the extracorporeal membrane oxygenation inter-hospital transport during coronavirus disease 2019 epidemic: based on the transport experience of 6 cases of severe H1N1 influenza virus pneumonia on extracorporeal membrane oxygenation. Zhonghua Wei Zhong Bing Ji Jiu Yi Xue 2020;32:430-4. [PubMed]
- Polak SB, Van Gool IC, Cohen D, et al. A systematic review of pathological findings in COVID-19: a pathophysiological timeline and possible mechanisms of disease progression. Mod Pathol 2020;33:2128-38. [Crossref] [PubMed]
- Duarte-Neto AN, Monteiro RAA, da Silva LFF, et al. Pulmonary and systemic involvement in COVID-19 patients assessed with ultrasound-guided minimally invasive autopsy. Histopathology 2020;77:186-97. [Crossref] [PubMed]
- Li MY, Li L, Zhang Y, et al. Expression of the SARS-CoV-2 cell receptor gene ACE2 in a wide variety of human tissues. Infect Dis Poverty 2020;9:45. [Crossref] [PubMed]