Prediction of adverse maternal and perinatal outcomes in preeclampsia based on B-type natriuretic peptide: a retrospective study
Introduction
Preeclampsia (PE) is a common disease unique to pregnant women, with an incidence of about 3–10% among all pregnancies (1). PE is mainly characterized by new-onset hypertension (systolic blood pressure ≥140 mmHg and/or diastolic blood pressure ≥90 mmHg) after 20 weeks of gestation with proteinuria, and/or any of the maternal organs (such as heart, lung, liver and kidney) or systems (such as blood system, digestive system and nervous system) dysfunction. Globally, PE and its related complications are leading causes of maternal death. Approximately 12–25% of fetal growth restriction and 15–20% of preterm infants are attributable to PE (1). The pathophysiological changes of PE are complex, include genetic imprinting, maternal immune intolerance, chronic placental ischemia and hypoxia, lipoprotein toxicity, abnormal trophoblast apoptosis and necrosis, and excessive inflammatory response (2). Although medical progress has been made, giving birth remains the only treatment for PE (3). For PE women who are not at full term, premature termination of pregnancy will increase the incidence of adverse outcomes in preterm infants, but excessive prolongment of the expected treatment time will increase the risk of adverse maternal and fetal outcomes. How to maximize the gestational age as well as benefits for the fetus while ensuring the safety of both mothers and children is a still challenge.
Accurate risk prediction of high-risk obstetric patients would enable physicians to tailor obstetric surveillance, treatment, and birth plans for these patients. Therefore, early prediction of adverse outcomes of PE is essential to improve the prognosis of mothers and infants. In recent years, researchers from various countries have devoted themselves to exploring the prediction of adverse outcomes of PE, and established some prediction models (4-8). These predictive models have their own advantages and disadvantages. How to screen out predictive indicators with high efficiency from many clinical indicators, especially indicators that are simple to use and conducive to the detection of primary hospitals, is of great clinical significance.
The neuroendocrine hormone, B-type natriuretic peptide (BNP), is mainly synthesized and secreted by the cardiac ventricles, and was first confirmed to play an important role in the development of hypertension (9). In recent years, studies have shown that the increased BNP level is closely related to PE, and it has been believed that BNP plays an important role in the pathophysiology of PE (10-14). Some researchers have proposed that BNP can be used as an indicator of the severity of PE or a predictor of preeclampsia, and some clinicians use this to monitor the condition changes of pregnant women with PE (15). Therefore, BNP may be used as an indicator to predict the adverse outcome of PE; however, few studies to date have evaluated the role of BNP in the adverse outcomes of women with PE.
In this study, we assessed the relationship between BNP and adverse pregnancy outcomes of PE through retrospective analysis. Then, the predictors of adverse outcomes in PE were further explored, and the prediction model and nomogram for adverse outcomes based on BNP were established, aiming to provide reference for clinical prevention and treatment of PE complications and improve pregnancy outcomes. We present the following article in accordance with the STROBE reporting checklist (available at https://dx.doi.org/10.21037/apm-21-2981).
Methods
Study design
A retrospective analysis of pregnant women hospitalized in The Second Hospital of Shandong University from January 2017 to July 2019 was conducted. Women with PE who were singleton and underwent BNP testing at admission were selected as the research participants. Their clinical characteristics, laboratory indicators, and pregnancy outcomes were collected and we then studied the relationship between BNP and adverse outcomes of PE, and established the prediction models and nomograms for adverse outcomes based on BNP.
The diagnostic criteria of PE were defined according to the American College of Obstetricians and Gynecologists (ACOG) guidelines (2). The diagnosis of PE was based on new-onset hypertension (systolic blood pressure ≥140 mmHg and/or diastolic blood pressure ≥90 mmHg) after 20 weeks of gestation and the coexistence of 1 or more of the following new-onset conditions: proteinuria (300 mg or more per 24 h urine collection or urinary protein dipstick ≥1+ or protein/creatinine ratio of 0.3 or more); thrombocytopenia (platelet count less than 100×109/L); impaired liver function (blood concentration of liver enzymes more than twice normal concentration); renal insufficiency (serum creatinine concentration greater than 1.1 mg/dL or a doubling of the creatinine concentration in the absence of other renal disease); severe persistent right upper abdomen or epigastric pain (not accounted for by alternative diagnoses); new-onset headache (unresponsive to medicine and not accounted for by alternative diagnoses) and visual disturbances (2). Women with severe blood pressures (systolic blood pressure ≥160 mmHg, or diastolic blood pressure ≥110 mmHg) and/or with the conditions above were diagnosed with PE with severe features (2). According to the gestational age at disease onset, PE was divided into early-onset PE (before 34th gestational week) and late-onset PE (after 34th gestational week). The exclusion criteria were as follows: twin pregnancy, multiple pregnancy, fetal malformations, use of assisted reproductive technology, combined with diabetes, cardiovascular disease, immune system disease, liver and kidney disease, blood system disease, malignant tumor, and so on.
Finally, 284 women with PE were included in the study. According to the occurrence of adverse outcomes, women with PE were divided into a with adverse outcomes group and without adverse outcomes group (Figure 1). This study was conducted retrospectively from data obtained for clinical purposes. The women with PE were followed up from admission, and the end point of the follow-up was discharge. The study was performed in accordance with the Declaration of Helsinki (as revised in 2013) and was approved by the Ethics Committee of the Second Hospital of Shandong University (approval number: KYLL-2021LW012). As this was a retrospective study, the informed consent of each participant was not required.
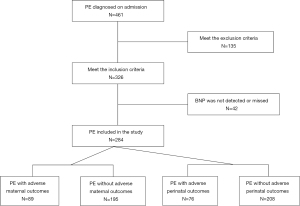
Data collection
The following data were collected from participant medical records: (I) basic characteristics at admission: maternal age, parity, body mass index (BMI), and gestational age (GA); (II) symptoms and signs at admission: systolic blood pressure (SBP), diastolic blood pressure (DBP), edema, fundus arteriovenous ratio, headache, visual disturbances, chest tightness, nausea, and vomiting; (III) laboratory indicators: BNP, proteinuria, hematocrit (HCT), hemoglobin (HB), platelets (PLT), fibrinogen (FIB), activated partial thromboplastin time (APTT), thrombin time (TT), prothrombin time (PT), alanine aminotransferase (ALT), aspartate aminotransferase (AST), lactate dehydrogenase (LDH), plasma albumin (ALB), blood urea nitrogen (BUN), blood creatinine (CR), and uric acid (UA); (IV) Perinatal infant characteristics: newborn weight and 1-minute Apgar scores.
BNP measurement
Laboratory indicators including BNP were detected within 24 h after admission. The detection was carried out within 2–4 h after blood collection to ensure the accuracy and stability of the results. For BNP measurement, 3 mL of whole blood was collected into tubes containing ethylenediamine tetraacetic acid (EDTA). The BNP levels were measured by Alere Triage BNP test (Quidel Cardiovascular Inc., Shanghai, China). The test is a double antibody sandwich enzyme immunoassay using mouse anti-human BNP monoclonal antibody to quantitatively detect BNP. The measurements of BNP in the range from 1 to 5,000 pg/mL can be accurately measured. The 95% confidence limit of the analytical sensitivity is less than 1 pg/mL. Any HB, FIB, lipids, and bilirubin added to plasma containing BNP did not interfere with BNP results. The test showed coefficients of variation of 1.1–6.6% intra-assay imprecision and 2.1–6.7% total imprecision at 24.5–3,966.2 pg/mL.
Definition of adverse outcomes
In our study, adverse pregnancy outcomes include adverse maternal outcomes and adverse perinatal outcomes. Adverse maternal outcomes included eclampsia, placental abruption, hemolysis, elevated liver enzymes, and low platelets syndrome (HELLP syndrome), cardiac insufficiency, abnormal liver function (the liver enzyme greater than 3 times the normal value, and the normal value is about 40 U/L), acute renal insufficiency (creatinine greater than 150 µmol/L), central nervous system complications (such as cerebral hemorrhage and reversible posterior encephalopathy syndrome), body cavity effusion (new-onset pleural effusion, pericardial effusion and ascites), and pulmonary hypertension (the mean pulmonary artery pressure more than 25 mmHg at rest). Adverse perinatal outcomes included small for gestation age (SGA; an infant with birth weight below the 10th percentile of the average weight of the same gestational age), fetal distress, fetal death, stillbirth, oligohydramnios, and neonatal asphyxia (1-min Apgar scores <7 and umbilical blood pH <7.20).
Statistical analysis
The software SPSS version 22.0 (IBM Corp., Armonk, NY, USA) was used for data analysis. Categorical variables were recorded by absolute numbers (percentage), and the comparisons between groups were performed with the Pearson’s chi-square or Fisher’s exact tests. Continuous variables were calculated by median (interquartile ranges, IQR) or mean ± standard deviation (SD), and the comparisons between groups were analyzed using rank sum test or t-test.
To study the relationship between BNP and adverse outcomes, we stratified BNP according to the quartile. We also performed univariate logistic regression analysis and drew the receiver operating characteristic (ROC) curve between BNP and adverse outcomes to evaluate the predictive efficacy of BNP for adverse outcomes.
The univariate analysis was performed on the relevant variables for adverse outcomes, variables that were significant at a level of 0.10 were incorporated into the multivariate logistics regression analysis, and the stepwise method was used to determine the final prediction model. In order to evaluate the discriminative performance of the prediction model, the ROC curve was drawn and the area under the curve (AUC) was calculated. To evaluate the calibration performance of the prediction model, a calibration curve was drawn. Comparisons of AUCs were performed with MedCalc statistical software (version 19.7; https://www.medcalc.org/).
The variables included in the prediction model were introduced into R software (version 3.5.3; https://www.r-project.org/) to draw the nomogram. To use the nomogram, the position of each variable was located on the corresponding axis; a vertical line was drawn from this position to the points axis; the corresponding points of each variable were determined; the points of all variables were summed; and a vertical line was drawn from the total points axis to determine the occurrence probability of adverse events in the lower line of the nomogram.
All tests were 2-sided, and P value <0.05 was considered statistically significant.
Results
Characteristics of the population in the study
During the study period, 461 women were diagnosed with PE at admission, among whom 284 women were finally eligible for the study. Among the 284 women with PE, 89 (31.3%) cases had adverse maternal outcomes and 76 (26.8%) cases had perinatal adverse outcomes (Figure 1). The characteristics of the women with and without adverse outcomes are shown in Tables 1,2. There was no significant difference in age, parity, and BMI between PE women with or without adverse maternal outcomes. The PE women with adverse perinatal outcomes were older and had lower BMI, while their newborns had lower body weight and 1-min Apgar score.
Table 1
Variable | Adverse maternal outcomes | P value | |
---|---|---|---|
Without (n=195) | With (n=89) | ||
Age (years) | 31.95±5.80 | 32.21±5.42 | 0.716 |
BMI (kg/m2) | 32.04±4.78 | 31.70±4.84 | 0.582 |
GA at admission (weeks) | 36.60±2.73 | 33.19±3.46 | <0.001 |
Parity | 0.378 | ||
1 | 70 (35.90) | 26 (29.21) | |
2 | 114 (58.46) | 55 (61.80) | |
>2 | 11 (5.64) | 8 (8.99) |
Continuous variables are presented as mean ± SD; categorical variables are presented as number (%). P, Pearson’s chi-square test for categorical variables and t-test for continuous variables. BMI, body mass index; GA, gestational age.
Table 2
Variable | Perinatal outcomes | P value | |
---|---|---|---|
Without (n=208) | With (n=76) | ||
Age (years) | 31.57±5.59 | 33.30±5.74 | 0.022 |
BMI (kg/m2) | 32.38±4.80 | 30.71±4.58 | 0.009 |
GA at admission (weeks) | 36.36±3.00 | 33.26±3.28 | <0.001 |
Parity | 0.268 | ||
1 | 76 (36.54) | 20 (26.32) | |
2 | 119 (57.21) | 50 (65.79) | |
>2 | 13 (6.25) | 6 (7.89) | |
Newborn weight | 2,880.14±848.42 | 1,737.81±631.40 | <0.001 |
1-minute Apgar scores | 9.73±0.94 | 8.47±2.77 | <0.001 |
Continuous variables are presented as mean ± SD; categorical variables are presented as number (%). P, Pearson’s chi-square test for categorical variables and t test for continuous variables. BMI, body mass index; GA, gestational age.
In addition, we compared the indicators and adverse outcomes between PE with and without severe features, early-onset PE and late-onset PE. There was no significant difference in age, parity, and BMI between early-onset PE and late-onset PE (Table S1). Compared with late-onset PE, the risk of adverse maternal and perinatal outcomes in early-onset PE were increased, and the birth weight and 1-minute Apgar score of newborns were lower (Table S2). Compared with PE without severe features, PE with severe features were older, the rate of multipara was higher, the risk of adverse maternal and perinatal outcomes were increased, and the birth weight and 1-minute Apgar score of newborns were lower (Tables S3,S4).
BNP levels in PE with and without adverse outcomes
The difference of BNP levels between PE women with and without adverse outcomes were compared. The BNP levels in PE with adverse maternal outcomes (114 pg/mL) were higher than those without adverse maternal outcomes (51 pg/mL) (Figure 2A). The BNP levels in women with PE with adverse perinatal outcomes (81 pg/mL) were also higher than those without adverse perinatal outcomes (54 pg/mL) (Figure 2B).
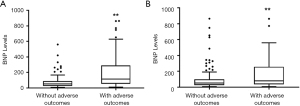
In addition, we compared the BNP levels between PE with and without severe features, early-onset PE and late-onset PE. Compared with late-onset PE (54 pg/mL), the BNP levels in early-onset PE (77.5 pg/mL) were higher (Table S1). Compared with PE without severe features (27 pg/mL), PE with severe features had higher BNP levels (80 pg/mL) (Table S3).
Prediction of adverse outcomes with BNP
To study the relationship between BNP and adverse outcomes, we stratified BNP according to the quartile. The univariate logistic regression showed that BNP is a risk factor for adverse maternal and perinatal outcomes. A level of BNP ≤30 pg/mL was used as the reference group, and as the level of BNP increased, the incidence of adverse outcomes increased. The risk of adverse maternal outcomes in PE with BNP >118 pg/mL was 9.941 times higher than in the reference group [odds ratio (OR) =9.941, 95% confidence interval (CI): 4.367–22.630] (Table 3). The risk of adverse perinatal outcomes in PE with BNP >118 pg/mL was 4.270 times higher than in the reference group (OR =4.270, 95% CI: 1.964–9.280) (Table 4). The ROC curve was used to analyze the predictive ability of BNP for adverse maternal outcomes, and the AUC was 0.739 (P<0.001, 95% CI: 0.684–0.789) (Figure 3A). The AUC of BNP for adverse perinatal outcomes was 0.642 (P<0.001, 95% CI: 0.583–0.698) (Figure 3B).
Table 3
Variable | β | OR (95% CI) | P value |
---|---|---|---|
BNP | 0.000 | ||
≤30 | Reference | Reference | |
>30–65 | 0.491 | 1.634 (0.679–3.933) | 0.273 |
>65–118 | 0.872 | 2.392 (1.027–5.571) | 0.043 |
>118 | 2.297 | 9.941 (4.367–22.630) | <0.001 |
BNP, brain natriuretic peptide; OR, odds ratio; CI, confidence interval.
Table 4
Variable | β | OR (95% CI) | P value |
---|---|---|---|
BNP | 0.000 | ||
≤30 | Reference | Reference | |
>30–65 | 0.275 | 1.317 (0.567–3.058) | 0.522 |
>65–118 | 0.358 | 1.430 (0.621–3.293) | 0.400 |
>118 | 1.452 | 4.270 (1.964–9.280) | <0.001 |
BNP, brain natriuretic peptide; OR, odds ratio; CI, confidence interval.
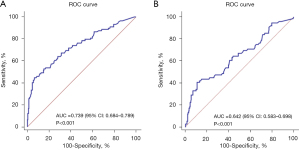
The univariate analysis of related factors affecting adverse outcomes
The univariate analysis was conducted on the related factors affecting adverse maternal outcomes, and significant factors were shown to include SBP, DBP, BNP, PLT, TT, AST, ALT, ALB, BUN, CR, UA, LDH, edema, proteinuria, headache, visual disturbances, nausea, and vomiting (Tables 1,5). Significant factors that affected the adverse outcome of perinatal infants were shown to include maternal age, BMI, SBP, DBP, BNP, HCT, HB, TT, AST, ALT, ALB, BUN, CR, UA, LDH, edema, and proteinuria (Tables 2,6).
Table 5
Variable | Adverse maternal outcomes | P value | |
---|---|---|---|
Without (n=195) | With (n=89) | ||
SBP (mmHg) | 158.80±18.35 | 170.25±20.81 | <0.001 |
DBP (mmHg) | 101.96±13.41 | 108.48±14.15 | <0.001 |
BNP (pg/mL) | 51.00 (25.00–89.00) | 114.00 (54.00–289.00) | <0.001 |
HCT (%) | 36.10±3.83 | 35.77±5.74 | 0.623 |
HB (g/L) | 118.54±14.87 | 118.46±21.86 | 0.974 |
PLT (109/L) | 219.86±62.72 | 198.40±88.45 | 0.041 |
FIB (g/L) | 4.12±0.70 | 4.03±0.92 | 0.360 |
APTT (s) | 27.03±2.30 | 27.55±2.71 | 0.098 |
TT (s) | 13.90±1.42 | 14.87±1.81 | <0.001 |
PT (s) | 10.73±0.54 | 10.77±0.56 | 0.514 |
AST (U/L) | 19.80 (13.00–25.80) | 24.70 (14.75–39.70) | 0.003 |
ALT (U/L) | 19.00 (16.00–26.00) | 26.30 (18.35–40.25) | <0.001 |
ALB (g/L) | 32.24±4.80 | 28.27±4.81 | <0.001 |
BUN (mmol/L) | 4.15±1.17 | 4.95±1.75 | <0.001 |
CR (μmol/L) | 52.42±11.24 | 62.16±16.00 | <0.001 |
UA (μmol/L) | 343.06±89.85 | 401.54±87.04 | <0.001 |
LDH (U/L) | 215.00 (190.00–272.00) | 266.00 (226.50–378.50) | <0.001 |
Edema | <0.001 | ||
0+ | 74 (37.95) | 15 (16.85) | |
1+ | 68 (34.87) | 24 (26.97) | |
2+ | 36 (18.46) | 29 (32.58) | |
3+ | 14 (7.18) | 14 (15.73) | |
4+ | 3 (1.54) | 7 (7.86) | |
A/V of fundus | 0.112 | ||
2:3 | 81 (52.94) | 29 (37.18) | |
1:2 | 48 (31.37) | 33 (42.31) | |
1:3 | 21 (13.73) | 15 (19.23) | |
1:4 | 3 (1.96) | 1 (1.28) | |
Proteinuria | <0.001 | ||
Negative | 20 (10.26) | 2 (2.25) | |
1+–2+ | 114 (58.46) | 28 (31.46) | |
3+ | 37 (18.97) | 37 (41.57) | |
4+ | 24 (12.31) | 22 (24.72) | |
Headache | 0.040 | ||
Yes | 20 (10.26) | 17 (19.10) | |
No | 175 (89.74) | 72 (80.90) | |
Visual disturbances | <0.001 | ||
Yes | 11 (5.64) | 17 (19.10) | |
No | 184 (94.36) | 72 (80.90) | |
Chest tightness | 0.303 | ||
Yes | 8 (4.10) | 7 (7.87) | |
No | 187 (95.90) | 82 (92.13) | |
Nausea or vomiting | 0.006 | ||
Yes | 1 (0.51) | 6 (6.74) | |
No | 194 (99.49) | 83 (93.26) |
Continuous variables are presented as mean ± SD or median (IQR); categorical variables are presented as number (%). P, Pearson’s chi-square test or Fisher’s exact tests for categorical variables and t-test or rank sum test for continuous variables. ALB, serum albumin; ALT, alanine transaminase; APTT, activated partial thromboplastin time; AST, aspartate transaminase; A/V, arteriovenous ratio; BNP, brain natriuretic peptide; BUN, blood urea nitrogen; CR, creatinine; DBP, diastolic blood pressure; FIB, fibrinogen; HB, hemoglobin; HCT, hematocrit; LDH, lactate dehydrogenase; PLT, platelets; PT, prothrombin time; SBP, systolic blood pressure; TT, thrombin time; UA, uric acid.
Table 6
Variable | Adverse perinatal outcomes | P value | |
---|---|---|---|
Without (n=208) | With (n=76) | ||
SBP (mmHg) | 160.44±18.71 | 167.71±21.92 | 0.011 |
DBP (mmHg) | 102.53±13.44 | 108.04±14.63 | 0.003 |
BNP (pg/mL) | 54.00 (27.00–99.25) | 81.10 (37.50–257.25) | <0.001 |
HCT (%) | 35.58±4.39 | 37.14±4.65 | 0.010 |
HB (g/L) | 116.51±16.69 | 124.03±17.93 | 0.001 |
PLT (109/L) | 215.10±68.69 | 207.76±81.65 | 0.450 |
FIB (g/L) | 4.13±0.75 | 3.99±0.83 | 0.155 |
APTT (s) | 27.13±2.45 | 27.37±2.42 | 0.472 |
TT (s) | 14.04±1.68 | 14.66±1.32 | 0.004 |
PT (s) | 10.74±0.54 | 10.74±0.57 | 0.951 |
AST (U/L) | 19.45 (13.00–27.18) | 24.60 (17.93–34.00) | 0.001 |
ALT (U/L) | 20.00 (16.00–27.00) | 23.00 (18.00–37.15) | 0.002 |
ALB (g/L) | 31.56±5.07 | 29.45±5.03 | 0.002 |
BUN (mmol/L) | 4.19±1.14 | 4.98±1.90 | 0.001 |
CR (μmol/L) | 53.83±12.40 | 59.98±15.88 | 0.003 |
UA (μmol/L) | 352.79±89.24 | 384.91±99.60 | 0.010 |
LDH (U/L) | 229.00 (197.00–276.75) | 266.00 (214.25–361.75) | 0.010 |
Edema | 0.055 | ||
0+ | 67 (32.21) | 22 (28.95) | |
1+ | 74 (35.58) | 18 (23.68) | |
2+ | 46 (22.12) | 19 (25.00) | |
3+ | 15 (7.21) | 13 (17.11) | |
4+ | 6 (2.88) | 4 (5.26) | |
A/V of fundus | 0.907 | ||
2:3 | 81 (48.50) | 29 (45.31) | |
1:2 | 56 (33.53) | 25 (39.06) | |
1:3 | 27 (16.17) | 9 (14.06) | |
1:4 | 3 (1.80) | 1 (1.56) | |
Proteinuria | <0.001 | ||
Negative | 19 (9.13) | 3 (3.95) | |
1+–2+ | 122 (58.65) | 20 (26.31) | |
3+ | 43 (20.67) | 31 (40.79) | |
4+ | 24 (11.54) | 22 (28.95) | |
Headache | 0.217 | ||
Yes | 24 (11.54) | 13 (17.10) | |
No | 184 (88.46) | 63 (82.90) | |
Visual disturbances | 0.115 | ||
Yes | 17 (8.17) | 11 (14.47) | |
No | 191 (91.83) | 65 (85.53) | |
Chest tightness | 0.132 | ||
Yes | 14 (6.73) | 1 (1.32) | |
No | 194 (93.27) | 75 (98.68) | |
Nausea or vomiting | 0.588 | ||
Yes | 4 (1.92) | 3 (3.95) | |
No | 204 (98.08) | 73 (96.05) |
Continuous variables are presented as mean ± SD or median (IQR); categorical variables are presented as number (%). P value, Pearson’s chi-square test or Fisher’s exact tests for categorical variables and t-test or rank sum test for continuous variables. ALB, serum albumin; ALT, alanine transaminase; APTT, activated partial thromboplastin time; AST, aspartate transaminase; A/V, arteriovenous ratio; BNP, brain natriuretic peptide; BUN, blood urea nitrogen; CR, creatinine; DBP, diastolic blood pressure; FIB, fibrinogen; HB, hemoglobin; HCT, hematocrit; LDH, lactate dehydrogenase; PLT, platelets; PT, prothrombin time; SBP, systolic blood pressure; TT, thrombin time; UA, uric acid.
Prediction of adverse outcomes with BNP combined with multiple factors
After multivariate logistics regression analysis, the factors including BNP, SBP, AST, ALB, and CR were incorporated in the predictive model of adverse maternal outcomes (Table 7). These factors were applied to construct the nomogram (Figure 4A). The ROC curve and calibration curve of the model were drawn. As a result, the model showed good discriminating ability, and the AUC was 0.844 (P<0.001, 95% CI: 0.796–0.884) (Figure 4B). The calibration curve indicated good calibration between observed probability and predicted probability (Figure 4C).
Table 7
Variable | β | OR (95% CI) | P value |
---|---|---|---|
BNP | 0.008 | 1.008 (1.004–1.011) | 0.000 |
ALB | −0.085 | 0.918 (0.857–0.984) | 0.016 |
AST | 0.013 | 1.013 (1.002–1.023) | 0.015 |
CR | 0.041 | 1.042 (1.015–1.069) | 0.002 |
SBP | 0.019 | 1.020 (1.002–1.037) | 0.025 |
BNP, brain natriuretic peptide; ALB, serum albumin; AST, aspartate transaminase; CR, creatinine; SBP, systolic blood pressure; OR, odds ratio; CI, confidence interval.
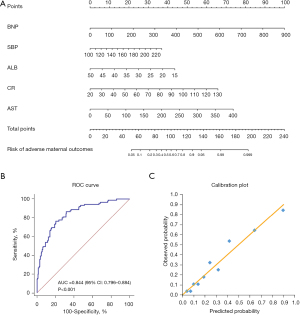
The factors included in the predictive model of adverse perinatal outcomes were: BNP, age, HB, BMI, and proteinuria (Table 8). The nomogram was constructed based on the above factors (Figure 5A). The model was also found to have good discriminating ability, and its AUC was 0.792 (P<0.001, 95% CI: 0.740–0.838) (Figure 5B). The calibration of the model was also good (Figure 5C).
Table 8
Variable | β | OR (95% CI) | P value |
---|---|---|---|
BNP | 0.003 | 1.003 (1.001–1.005) | 0.003 |
Age | 0.069 | 1.072 (1.015–1.132) | 0.013 |
BMI | −0.089 | 0.915 (0.854–0.980) | 0.011 |
HB | 0.031 | 1.032 (1.012–1.052) | 0.001 |
Proteinuria | 0.000 | ||
Negative | Reference | Reference | |
1+–2+ | −0.008 | 0.992 (0.255–3.859) | 0.991 |
3+ | 1.323 | 3.753 (0.957–14.719) | 0.058 |
4+ | 1.456 | 4.288 (1.020–18.036) | 0.047 |
BNP, brain natriuretic peptide; BMI, body mass index; HB, hemoglobin; OR, odds ratio; CI, confidence interval.
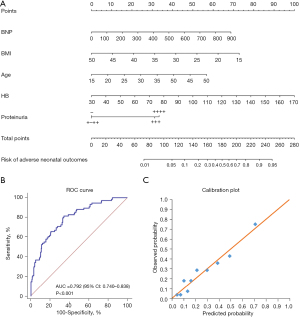
In order to clarify the important role of BNP in the predictive model, we drew the ROC curves of other factors in the predictive model except BNP, calculated their AUCs, and then compared them with the AUCs of the models containing BNP. For the adverse maternal outcomes, the AUC of the model with BNP was 0.844, which was significantly higher than that of the model without BNP (AUC =0.790, P=0.0017; Figure 6A). For the adverse perinatal outcomes, the AUC of the model with BNP (AUC =0.792) was also higher than that of the model without BNP (AUC =0.764, P=0.0374; Figure 6B).
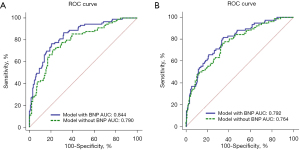
Discussion
Main findings
First, our data show that the PE women with adverse outcomes had higher BNP levels on admission. Second, BNP was shown to be a risk factor for adverse outcomes, and as the level of BNP increased, the incidence of adverse outcomes increased. The BNP was shown to have a certain predictive value for adverse maternal outcomes (AUC =0.739). Third, BNP can combine with clinical features and laboratory indicators to construct predictive models for adverse maternal and perinatal outcomes, and BNP was found to play an important role in the models. Both the discrimination and calibration capabilities of the predictive models were good (AUCs were 0.844 and 0.792, respectively).
Interpretation
The BNP is mainly synthesized and secreted by ventricular myocytes, and it also shows paracrine effects in many other tissues, such as placenta, amniotic membrane, and amniotic fluid (14,16-18). During normal pregnancy, BNP is maintained at a relatively low level (a median level of <20 pg/mL) (12,19). However, the PE women were shown to have significantly higher BNP levels in the third trimester (10-14). The BNP level of PE with severe features was higher than that of PE without severe features (10,12), while the BNP of early-onset PE was higher than late-onset PE (11,14). Our results were consistent with those of the previous studies. Previous studies had also shown that BNP levels in women with PE were higher than those in women with gestational hypertension, but the comparison of BNP levels between gestational hypertension and normal pregnancy were inconsistent. Some studies showed that the BNP levels in gestational hypertension were higher than that in normal pregnancy (20-22), while others considered that there was no significant difference in BNP levels between them (12,23). Our study was retrospective, and there were few patients with gestational hypertension who received BNP testing at admission, so we did not analyze this population.
To date, it is still unclear why BNP is increased in PE, but some reasoning may explain that. On the one hand, the placenta can produce BNP, and hypoxia can induce the expression of extracardiac BNP, suggesting that the increase of BNP in PE may be the consequence and defense mechanism of placental hypoxia (11). On the other hand, the effects of cardiovascular changes, placental dysfunction, and increased placental circulation resistance on the cardiac system in PE are thought to be responsible for the increase release of BNP by ventricle (10).
Studies on BNP in PE have mainly focused on its diagnosis or prediction of the disease and its relationship with the associated cardiovascular complications. In PE, the BNP levels reflect the severity of the PE (10-14), and elevated BNP levels are associated with ventricular systolic or diastolic dysfunction (24-26). Kumari et al. (13) found that BNP levels in women with chronic hypertension and gestational hypertension who later superimposed or progressed to PE were significantly higher than those who did not develop PE, and the ROC curve analysis showed that BNP had a good value in predicting the development of PE in the study population (AUC =0.7406, 95% CI: 0.6543–0.827, P<0.0001). Therefore, BNP can be used as an indicator of the severity of PE and to monitor the condition changes.
However, few studies have focused on the relationship between BNP and adverse pregnancy outcomes of PE. A retrospective analysis showed that the increase of BNP was related to cardiovascular complications and preterm birth in PE (27). Giannubilo et al. (28) showed that the BNP level in PE was negatively correlated with the weight of the newborn, while the BNP level in those patients with intrauterine growth restriction (IUGR) was even higher. Another study showed that BNP had a relatively high predictive value for the occurrence of adverse outcomes in early-onset PE (AUC =0.944), but the outcomes were limited to giving birth in short time, stillbirth, or early neonatal death (29). Pulmonary interstitial syndrome (PIS) was detected in 25% of PE women with severe features, which was associated with increased maternal and neonatal morbidity and mortality (30). Cardiac dysfunction plays an important role in the occurrence of PIS, and the BNP level has good specificity in predicting cardiac dysfunction. Therefore, the BNP level can indirectly reflect the occurrence of PIS (24). The study by Yu et al. (31) used velocity vector imaging combined with BNP levels to study the ventricular functions of fetuses in women with PE, showed that the fetuses of mothers with PE had biventricular diastolic and systolic dysfunction, and the increase of fetal umbilical vein BNP levels were significantly correlated with the dysfunction (Spearman coefficient was 0.615–0.703).
Our study is the first to evaluate the value of BNP in predicting the comprehensive adverse maternal and perinatal outcomes of PE. The PE women with adverse outcomes had significantly higher BNP levels than PE women without adverse outcomes (Figure 2). With the increase of BNP, the risk of adverse outcomes of PE increased, and PE with BNP >118 pg/mL was associated with a significantly increased risk of adverse maternal (OR =9.941) and perinatal outcomes (OR =4.270), in comparison with BNP <30 pg/mL. The BNP had better predictive ability for adverse maternal outcomes (AUC =0.739), but poorer predictive ability for adverse perinatal outcomes (AUC =0.642). Therefore, we cannot predict adverse outcomes only by BNP, and it is necessary to combine multiple factors to predict the occurrence of adverse outcomes. Finally, we established the predictive models based on BNP and multiple clinical indicators, and ROC curves and calibration curves were used to evaluate the discrimination ability and calibration ability of the prediction models. The results showed that both the discrimination and calibration capabilities of the models are good (AUCs were 0.844 and 0.792, respectively). Therefore, we believe that monitoring BNP levels can identify high-risk women, for whom we can then provide further examination and treatment, and terminate the pregnancy in time to prevent serious adverse pregnancy outcomes.
Our prediction model includes not only BNP, but also some established markers of adverse outcomes. Other factors in the prediction model of maternal outcomes are SBP, AST, CR, and ALB. The results of our model are consistent with those of some established models (4-8,32-34), indicating that elevated SBP, AST, and CR levels as well as reduced ALB levels predict the increase of adverse maternal outcomes, so changes in these indicators need to be closely monitored during clinical treatment. Other factors in the prediction model of perinatal outcomes were maternal age, HB, BMI, and proteinuria. There is an “inverted U-shaped” association between maternal hemoglobin levels and newborn birth weight (35), whereby the higher the hemoglobin (HB ≥120 g/L) of women with preeclampsia, the higher the incidence of low birth-weight infants (36,37), which is consistent with the results of our study. There is controversy regarding the predictive role of proteinuria on adverse outcomes in PE. Some studies have suggested that proteinuria is a good indicator for predicting adverse outcomes (6,32,38), but others have shown otherwise (39). In PE, the women with proteinuria >3 g/24 h had a lower birth weight, while the women with proteinuria >5 g/24 h had a significantly higher incidence of fetal growth restriction or stillbirth (40). Proteinuria was closely associated with adverse fetal outcomes (r=0.336, P<0.001), which suggested that proteinuria may have indirect predictive value for adverse outcomes (41).Our study showed that when PE women have obvious proteinuria, the risk of perinatal adverse outcomes will increase. Although the latest guidelines have diminished the role of proteinuria in PE, it does not refute the significance of it in the disease. The degree of proteinuria cannot be used as a single indication for termination of pregnancy, but it can still reflect renal damage, and large amounts of proteinuria can lead to hypoalbuminemia, thereby affecting the outcome of PE. Increasing age was a risk factor for adverse perinatal outcomes in our study (OR: 1.072, 95% CI: 1.015–1.132), which was the same as other studies (6,7). Study had shown that in women ≥35 years old, the risk of adverse outcomes such as stillbirth, preterm birth and low birth weight was higher than that in the control group (20–34 years old) (42). And the incidence of low birth weight in women > 40 years old was 1.51 times higher than that in women ≥35 years old (43). Our study showed that the GA at admission for PE with adverse outcomes were smaller than those without adverse outcomes, so that adverse outcomes were occurring in PE at earlier GA. But we wanted to study that whether we could use the indicators other than GA to predict adverse outcomes. Therefore, at the same GA, adverse outcomes can be predicted based on the severity of these indicators, allowing individualized treatment. So, GA was not included in our final models. However, we also conducted ROC curve analysis and calculated the AUC for the prediction model containing GA, and found that there was no significant statistical difference between the AUC of the prediction model containing GA and that of our final prediction model.
In recent years, researchers have established some multi-factor prediction models for the adverse outcomes of PE (4-8). These models all have good prediction performance, and their AUCs range from 0.76 to 0.88. Our prediction model achieved similar prediction performance to previous models (AUC was 0.844 and 0.792). We constructed a nomogram using the factors in the prediction model. We assigned scores to each factor according to its contribution to adverse outcomes, add the scores of each factor we combined to obtain a total score, which was then converted to the incidence of adverse outcomes to calculate the individual probability of adverse outcomes. In this way, the risk assessment of adverse outcomes can be more intuitively provided for individuals.
Strengths and limitations of research
Our study was the first to comprehensively research the role of BNP in predicting adverse outcomes of PE, establish a prediction model for adverse outcomes based on BNP, and construct a nomogram. The factors we selected in the predictive models are commonly detected by most laboratories and easily collected by physicians. Using them, we can predict the adverse pregnancy outcomes in PE women on admission and high-risk women can be identified early, which can give physicians sufficient time for risk assessment and clinical intervention. However, the performance of the prediction model depends heavily on the study population and the selected predictors, so further external validation of our prediction models will be required before applying them throughout different healthcare institutions and the wider population.
The definition of adverse outcomes in this study included not only the severe maternal complications in the fullPIERS model (4), but also some non-serious adverse outcomes (such as body cavity effusion, pulmonary hypertension, and so on). We also predicted the adverse perinatal outcomes. These non-serious adverse outcomes may have short- and long-term adverse effects on mothers and newborns, and are also worthy of clinical monitoring and attention.
There are some limitations in this study. Our study included urine protein dipstick as a variable rather than 24 h urine protein, because the 24 h urine collections had been conducted in fewer than 70% of the participants. In the real word, we believe that clinicians faced with PE women with proteinuria on dipstick analysis at term or PE women with severe symptoms will decide to proceed with birth as soon as possible as opposed to waiting for the additional 24 h urine collection (4). Therefore, we suggest that dipstick proteinuria, despite its shortcomings, can also be used to screen and identify women at risk. The sample size of the study population and adverse outcomes was limited. As this study was a single-center, retrospective study, we can only analyze the recorded variables, which may have caused selection bias. In addition, only internal verification was performed in our study. Hence, hopefully in the future, multi-center, large-sample, prospective research, and external verification can be conducted to further clarify the role of BNP in the predicting adverse pregnancy outcomes. A further limitation of the study is that BNP has already been used as one of the monitoring indicators for the severity of PE in our research institution, where BNP levels affect the decisions on clinical intervention and the timing of termination of pregnancy. Therefore, the relatively low prediction accuracy of BNP may have been partly due to intervention bias. However, we believe that the real-world data approach should be conducted in this way.
Conclusions
The level of BNP is one of the important risk factors for evaluating the adverse outcomes of PE. It can combine with multiple clinical indicators to predict the adverse pregnancy outcomes of PE. Therefore, the presence of significantly elevated BNP in PE accompanied by abnormalities of multiple indicators often suggests poor pregnancy outcomes, suggesting that early identification and prevention of adverse outcomes should be paid attention to during treatment.
Acknowledgments
Funding: None.
Footnote
Reporting Checklist: The authors have completed the STROBE reporting checklist. Available at https://dx.doi.org/10.21037/apm-21-2981
Data Sharing Statement: Available at https://dx.doi.org/10.21037/apm-21-2981
Conflicts of Interest: All authors have completed the ICMJE uniform disclosure form (available at https://dx.doi.org/10.21037/apm-21-2981). The authors have no conflicts of interest to declare.
Ethics Statement: The authors have promised to protect the privacy of participants. The authors are accountable for all aspects of the work in ensuring that questions related to the accuracy or integrity of any part of the work are appropriately investigated and resolved. The study was performed in accordance with the Declaration of Helsinki (as revised in 2013) and was approved by the Ethics Committee of the Second Hospital of Shandong University (approval number: KYLL-2021LW012). As this was a retrospective study, the informed consent of each participant was not required.
Open Access Statement: This is an Open Access article distributed in accordance with the Creative Commons Attribution-NonCommercial-NoDerivs 4.0 International License (CC BY-NC-ND 4.0), which permits the non-commercial replication and distribution of the article with the strict proviso that no changes or edits are made and the original work is properly cited (including links to both the formal publication through the relevant DOI and the license). See: https://creativecommons.org/licenses/by-nc-nd/4.0/.
References
- Duley L. The global impact of pre-eclampsia and eclampsia. Semin Perinatol 2009;33:130-7. [Crossref] [PubMed]
- ACOG Practice Bulletin No. 202: Gestational Hypertension and Preeclampsia. Obstet Gynecol 2019;133:e1-25.
- Le Y, Ye J, Lin J. Expectant management of early-onset severe preeclampsia: a principal component analysis. Ann Transl Med 2019;7:519. [Crossref] [PubMed]
- von Dadelszen P, Payne B, Li J, et al. Prediction of adverse maternal outcomes in pre-eclampsia: development and validation of the fullPIERS model. Lancet 2011;377:219-27. [Crossref] [PubMed]
- Payne BA, Hutcheon JA, Ansermino JM, et al. A risk prediction model for the assessment and triage of women with hypertensive disorders of pregnancy in low-resourced settings: the miniPIERS (Pre-eclampsia Integrated Estimate of RiSk) multi-country prospective cohort study. PLoS Med 2014;11:e1001589. [Crossref] [PubMed]
- Zwertbroek EF, Broekhuijsen K, Langenveld J, et al. Prediction of progression to severe disease in women with late preterm hypertensive disorders of pregnancy. Acta Obstet Gynecol Scand 2017;96:96-105. [Crossref] [PubMed]
- Thangaratinam S, Allotey J, Marlin N, et al. Prediction of complications in early-onset pre-eclampsia (PREP): development and external multinational validation of prognostic models. BMC Med 2017;15:68. [Crossref] [PubMed]
- Li X, Zhang W, Lin J, et al. Risk factors for adverse maternal and perinatal outcomes in women with preeclampsia: analysis of 1396 cases. J Clin Hypertens (Greenwich) 2018;20:1049-57. [Crossref] [PubMed]
- Licata A, Corrao S, Petta S, et al. NT pro BNP plasma level and atrial volume are linked to the severity of liver cirrhosis. PLoS One 2013;8:e68364. [Crossref] [PubMed]
- Bakacak M, Serin S, Ercan O, et al. Association of serum N-terminal pro-brain natriuretic peptide levels with the severity of preeclampsia. J Matern Fetal Neonatal Med 2016;29:2802-6. [PubMed]
- Szabó G, Molvarec A, Nagy B, et al. Increased B-type natriuretic peptide levels in early-onset versus late-onset preeclampsia. Clin Chem Lab Med 2014;52:281-8. [Crossref] [PubMed]
- Seong WJ, Kim SC, Hong DG, et al. Amino-terminal pro-brain natriuretic peptide levels in hypertensive disorders complicating pregnancy. Hypertens Pregnancy 2011;30:287-94. [Crossref] [PubMed]
- Kumari M, Kovach T, Sheehy B, et al. Circulating NT-proBNP but not soluble corin levels were associated with preeclampsia in pregnancy-associated hypertension. Clin Biochem 2019;67:12-5. [Crossref] [PubMed]
- Junus K, Wikström AK, Larsson A, et al. Placental expression of proBNP/NT-proBNP and plasma levels of NT-proBNP in early- and late-onset preeclampsia. Am J Hypertens 2014;27:1225-30. [Crossref] [PubMed]
- Verlohren S, Perschel FH, Thilaganathan B, et al. Angiogenic Markers and Cardiovascular Indices in the Prediction of Hypertensive Disorders of Pregnancy. Hypertension 2017;69:1192-7. [Crossref] [PubMed]
- Itoh H, Sagawa N, Hasegawa M, et al. Brain natriuretic peptide is present in the human amniotic fluid and is secreted from amnion cells. J Clin Endocrinol Metab 1993;76:907-11. [PubMed]
- Cameron VA, Aitken GD, Ellmers LJ, et al. The sites of gene expression of atrial, brain, and C-type natriuretic peptides in mouse fetal development: temporal changes in embryos and placenta. Endocrinology 1996;137:817-24. [Crossref] [PubMed]
- Walther T, Stepan H. C-type natriuretic peptide in reproduction, pregnancy and fetal development. The Journal of endocrinology 2004;180:17-22. [Crossref] [PubMed]
- Resnik JL, Hong C, Resnik R, et al. Evaluation of B-type natriuretic peptide (BNP) levels in normal and preeclamptic women. Am J Obstet Gynecol 2005;193:450-4. [Crossref] [PubMed]
- Sadlecki P, Grabiec M, Walentowicz-Sadlecka M. Prenatal Clinical Assessment of NT-proBNP as a Diagnostic Tool for Preeclampsia, Gestational Hypertension and Gestational Diabetes Mellitus. PLoS One 2016;11:e0162957. [Crossref] [PubMed]
- Fleming SM, O'Byrne L, Grimes H, et al. Amino-terminal pro-brain natriuretic peptide in normal and hypertensive pregnancy. Hypertens Pregnancy 2001;20:169-75. [Crossref] [PubMed]
- Moghbeli N, Srinivas SK, Bastek J, et al. N-terminal pro-brain natriuretic Peptide as a biomarker for hypertensive disorders of pregnancy. Am J Perinatol 2010;27:313-9. [Crossref] [PubMed]
- Borghi C, Cicero AF, Degli Esposti D, et al. Hemodynamic and neurohumoral profile in patients with different types of hypertension in pregnancy. Intern Emerg Med 2011;6:227-34. [Crossref] [PubMed]
- Ortner CM, Krishnamoorthy V, Neethling E, et al. Point-of-Care Ultrasound Abnormalities in Late-Onset Severe Preeclampsia: Prevalence and Association With Serum Albumin and Brain Natriuretic Peptide. Anesth Analg 2019;128:1208-16. [Crossref] [PubMed]
- Borges VTM, Zanati SG, Peraçoli MTS, et al. Maternal left ventricular hypertrophy and diastolic dysfunction and brain natriuretic peptide concentration in early- and late-onset pre-eclampsia. Ultrasound Obstet Gynecol 2018;51:519-23. [Crossref] [PubMed]
- Ghomian N, Vakilian F, Shahri B, et al. Can brain natriuretic peptide predict cardiovascular complications in severe preeclampsia? A case-control study. Int J Reprod Biomed 2019;17:271-8. [Crossref] [PubMed]
- Afshani N, Moustaqim-Barrette A, Biccard BM, et al. Utility of B-type natriuretic peptides in preeclampsia: a systematic review. Int J Obstet Anesth 2013;22:96-103. [Crossref] [PubMed]
- Giannubilo SR, Pasculli A, Tidu E, et al. Relationship between maternal hemodynamics and plasma natriuretic peptide concentrations during pregnancy complicated by preeclampsia and fetal growth restriction. J Perinatol 2017;37:484-7. [Crossref] [PubMed]
- Álvarez-Fernández I, Prieto B, Rodríguez V, et al. N-terminal pro B-type natriuretic peptide and angiogenic biomarkers in the prognosis of adverse outcomes in women with suspected preeclampsia. Clin Chim Acta 2016;463:150-7. [Crossref] [PubMed]
- Ambrozic J, Brzan Simenc G, Prokselj K, et al. Lung and cardiac ultrasound for hemodynamic monitoring of patients with severe pre-eclampsia. Ultrasound Obstet Gynecol 2017;49:104-9. [Crossref] [PubMed]
- Yu L, Zhou Q, Peng Q, et al. Velocity vector imaging echocardiography and NT-proBNP study of fetal cardiac function in pregnancy-induced maternal hypertension. J Clin Ultrasound 2019;47:285-91. [Crossref] [PubMed]
- Liao Y, Liu XH, Tan J, et al. Development of a Predictive Model for Adverse Outcomes of Preeclampsia. Sichuan Da Xue Xue Bao Yi Xue Ban 2018;49:797-802. [PubMed]
- Paul TD, Hastie R, Tong S, et al. Prediction of adverse maternal outcomes in preeclampsia at term. Pregnancy Hypertens 2019;18:75-81. [Crossref] [PubMed]
- Chen R, Han Q, Zheng L, et al. Establishment and assessment of a nomogram for predicting adverse outcomes of preterm preeclampsia. J Int Med Res 2020;48:300060520911828. [Crossref] [PubMed]
- Laflamme EM. Maternal hemoglobin concentration and pregnancy outcome: a study of the effects of elevation in el alto, bolivia. Mcgill J Med 2011;13:47. [PubMed]
- Amburgey OA, Ing E, Badger GJ, et al. Maternal hemoglobin concentration and its association with birth weight in newborns of mothers with preeclampsia. J Matern Fetal Neonatal Med 2009;22:740-4. [Crossref] [PubMed]
- Young MF, Oaks BM, Tandon S, et al. Maternal hemoglobin concentrations across pregnancy and maternal and child health: a systematic review and meta-analysis. Ann N Y Acad Sci 2019;1450:47-68. [Crossref] [PubMed]
- Homer CS, Brown MA, Mangos G, et al. Non-proteinuric pre-eclampsia: a novel risk indicator in women with gestational hypertension. J Hypertens 2008;26:295-302. [Crossref] [PubMed]
- Fishel Bartal M, Lindheimer MD, Sibai BM. Proteinuria during pregnancy: definition, pathophysiology, methodology, and clinical significance. Am J Obstet Gynecol 2020; Epub ahead of print. [Crossref] [PubMed]
- Dong X, Gou W, Li C, et al. Proteinuria in preeclampsia: Not essential to diagnosis but related to disease severity and fetal outcomes. Pregnancy Hypertension. 2017;8:60-64. [Crossref] [PubMed]
- Lei T, Qiu T, Liao W, et al. Proteinuria may be an indicator of adverse pregnancy outcomes in patients with preeclampsia: a retrospective study. Reprod Biol Endocrinol 2021;19:71. [Crossref] [PubMed]
- Debelo BT, Hunie Asratie M, Solomon AA. Risk of Selected Fetal Adverse Pregnancy Outcomes at Advanced Maternal Age: A Retrospective Cohort Study in Debre Markos Referral Hospital, Northwest Ethiopia. Obstet Gynecol Int 2020;2020:1875683. [Crossref] [PubMed]
- Guarga Montori M, Álvarez Martínez A, Luna Álvarez C, et al. Advanced maternal age and adverse pregnancy outcomes: A cohort study. Taiwan J Obstet Gynecol 2021;60:119-24. [Crossref] [PubMed]