Prognostic value of the albumin-bilirubin score in critically ill patients with heart failure
Introduction
Heart failure (HF) is a condition that occurs when the heart is unable to pump enough oxygen-rich blood to the rest of the body. It can be caused by heart conditions, and non-cardiac diseases, such as a critical illness, severe pneumonia, bacterial sepsis, and chronic and acute renal failure (1). HF affects approximately 26 million people worldwide, and results in more than 1 million hospitalizations annually in both the United States (US) and Europe (2,3). HF is associated with high morbidity and mortality rates, and places high socioeconomic burdens on healthcare systems (4). Thus, it is important to develop reliable methods to identify the risk factors of death among critically ill patients with HF.
A number of clinical risk prediction models have been designed to predict adverse outcomes in hospitalized HF patients and estimate mortality risk, including the Acute Physiology and Chronic Health Evaluation-Heart Failure (APACHE-HF) (5), the AHEAD (A: atrial fibrillation; H: hemoglobin; E: elderly; A: abnormal renal parameters; D: diabetes mellitus) (6), the Acute Decompensated Heart Failure National Registry (ADHERE) (7), the Enhanced Feedback for Effective Cardiac Treatment (EFFECT) (8), the Organized Program to Initiate Lifesaving Treatment in Hospitalized Patients With Heart Failure (OPTIMIZE-HF) (9), and the Get With the Guidelines-Heart Failure (GWTG-HF) (10) models. Of these methods, the GWTG-HF model is the most recently published and externally validated risk model (11). It uses simple variables, and has been shown to be reasonably effective at predicting inpatient mortality in hospitalized patients with acute HF and 1-year mortality in a heterogeneous cohort of cardiac intensive care unit (ICU) patients (11). However, the GWTG-HF model does not perform well in critically ill patients with HF, presumably because of the complex and heterogeneous disease conditions of such patients, and it remains unclear if the GWTG-HF model is suitable for use in these patients.
A number of risk-scoring systems are used to predict adverse outcomes in critically ill patients, such as the Simplified Acute Physiology Score II (SAPSII) (12), the Acute Physiology Score III (ASPIII) (13), and the Sequential Organ Failure Assessment (SOFA) score (14). However, the value of these methods at predicting outcomes in patients with HF is unclear. Additionally, these methods use items such as the Glasgow Coma Score (GCS) (15) which are subjective and time-consuming to administer.
The albumin-bilirubin (ALBI) score was developed to assess liver function and predict survival in patients with hepatocellular carcinoma (HCC) (16). In addition to its use in predicting liver failure (17), the ALBI score has been used to predict acute kidney injury after chemotherapy (18). HF is usually complicated by liver dysfunction, and liver dysfunction can cause adverse cardiac reactions (19). Thus, the ALBI score has been reported to have clinical and prognostic value among patients with acute HF (20). However, it is still unknown whether the ALBI score is useful at predicting short- and long-term mortality in critically ill patients with HF. This study sought to use the data of HF patients admitted to the ICU to examine the predictive value of the ALBI score and other risk-factor scoring systems, and to develop a new model that combined ALBI and GWTG-HF to predict short-term and 4-year all-cause mortality in these patients. We present the following article in accordance with the STARD reporting checklist (available at https://dx.doi.org/10.21037/apm-21-3424).
Methods
Database
This study was based on data derived from the Medical Information Mart for Intensive Care III (MIMICIII) research database developed by researchers at the Laboratory for Computational Physiology at Massachusetts Institute of Technology (MIT), Cambridge, MA, USA, and the Department of Medicine at the Beth Israel Deaconess Medical Center (BIDMC) Boston, MA, USA (21,22). The database contains detailed information about patient ICU treatment, including data related to the International Classification of Diseases, 9th Revision (ICD-9) codes, age, sex, vital signs, laboratory results, radiology reports, therapeutic interventions, discharge summaries, and outcomes during the follow-up period (21,22). The data of 58,976 patients treated at the ICU at BIDMC from 2001 to 2012 are available in the database (21,22). The database is accessible to researches who have completed ‘protecting human subjects’ training. The Carevue system and the Metavision system are the 2 systems in the database from which follow-up data can be extracted, and both were used in this study. The study was conducted in accordance with the Declaration of Helsinki (as revised in 2013). Data extraction was performed using PostgreSQL tools V.1.12.3.
Study population and variables
Patients treated in the ICU between 2001 and 2012 who met all of the following criteria were included in the analysis: (I) were aged ≥18 years; (II) had a primary diagnosis of HF (ICD-9 code =40201, 40211, 40291, 40401, 0403, 40411, 40413, 40491, 40493, 4280, 4281, 42820, 42822, 42823, 42830, 42832, 42833, 42840, 42842, and 4289); (III) had an ICU stay ≥24 hours; and (IV)had Bilirubin level measurement data available. PostgreSQL tools V.1.12.3. was used to extract the following data from the first 24 hours after ICU admission: (I) the primary admission unit; (II) demographic information, including age, weight, high, sex, ethnicity, heart rate, and blood pressure; (III) disease severity as assessed by the SAPSII (12), the APSIII (13), the SOFA score (14), and GWTG-HF risk scores (10); and (IV) laboratory measurement data, including aspartate aminotransferase (AST) level, the international normalized ratio (INR), red cell volume distribution width (RDW), serum sodium level, serum blood urea nitrogen (BUN) level, serum albumin level, and serum total bilirubin level data. ALBI was calculated using the following equation: ALBI = [log10bilirubin (µmol/L) × 0.66 − 0.085 × albumin (g/L)] (12).
The presence of comorbidities related to HF, including diabetes, heart disease, chronic obstructive pulmonary disease (COPD), liver disease, chronic kidney disease, and cerebrovascular disease, were also extracted from the database.
Study outcomes
The study outcomes were short-term and 4-year all-cause mortality. Short-term mortality was defined as death after ICU admission to 28 days after discharge. In-hospital mortality was also included in the category of short-term mortality for the analysis. Date of death was obtained from the Social Security Death Index records of the US government.
Statistical analysis and model building
The continuous variables are presented as the mean ± standard deviation or the median with the interquartile range (IQR). The categorical variables are presented as the number and percentage (%). Group means were tested using a Student’s independent t-test, Wilcoxon rank-sum test, or Kruskal-Wallis test according to the number of patients in the group and the normality assumption. Categorical variables were compared using the chi-square test or Fisher’s exact test.
Of all the variables in the data set, the missing value ratio of each variable was <20%. Any missing values were imputed using the expectation-maximization method using SPSS version 26 (IBM, Armonk, NY, USA).
A bar chart was used to illustrate the relationship between the ALBI score and the mortality rate, and a quadratic linear formula was used to fit the trend. Survival analyses were conducted using the Kaplan-Meier method and the log-rank test, and Cox proportional hazards-regression models were used to evaluate the relationship between the ALBI score and short-term and 4-year mortality. A univariate logistic regression analysis was used to screen risk factors, and factors with a P value <0.05 were used for the multivariate Cox proportional hazards-regression models. The final models were selected by stepwise selection.
Multivariate logistic regression models using a forward-selection modeling process were built to construct simpler and more effective clinical predictive models for predicting the short term and 4-year mortality of critically ill HF patients. The final logistic regression predictive model used the adjusted covariates of GWTG-HF and ALBI (GWTG-HF-ALBI) to predict mortality. A receiver operating characteristic (ROC) curve analysis was used to evaluate the ability of the ALBI and the new model to discriminate between survivors and non-survivors. The Hosmer and Lemeshow Goodness-of-fit test was performed to test the fitness of the models. All the statistical analyses were performed using Stata version 14.0 software, SPSS statistical software version 26.0, and R statistical software version 3.5.2). Two-tailed P values <0.05 were considered statistically significant.
Results
Baseline characteristics of study population
Of the 58,976 ICU patients included in the database for the study period, 12,456 were excluded because of data duplication. An additional 7,847 patients were excluded because they were aged <18 years old, and 29,797 were excluded because they had a diagnosis other than HF. Additionally, 346 patients with an ICU stay <24 hours, and 5,149 patients for whom no bilirubin data were available were excluded. Thus, ultimately 3,381 ICU patients who met the inclusion criteria were included in the analysis. In total, 1,986 patients from the Carevue system were followed up with for 4 years, and 1,395 patients from Metavision system were followed up with for 90 days after discharge. A flow diagram of patient inclusion is shown in Figure 1. In total, there were 937 non-survivors and 2,444 survivors, and the short-term mortality rate was 27.7%. Patients had a mean age of 70.02±12.55 years, and 1,817 (53.7%) patients were male.
The characteristics of all the patients, and those of survivors and non-survivors, are shown in Table 1. The ALBI score of survivors [−1.80 (−2.09 to −1.44)] was significantly lower than that of non-survivors [−1.43 (−1.80 to −0.99)] (P<0.001). Non-survivors were significantly older than survivors (73.12±11.46 vs. 68.83±12.75 years; P<0.001). Additionally, non-survivors had significantly higher SOFA score, SAPSII, and APSIII than survivors [i.e., 7 (4 to 10) vs. 4 (3 to 7), P<0.001; 49 (41 to 59) vs. 38 (30 to 47), P<0.001; 60 (47 to 77) vs. 45 (35 to 58), respectively, P<0.001]. Significant differences between survivors and non-survivors were also observed in the parameters, including differences in mean arterial pressure (MAP), AST, INR, and RDW (all P<0.001; Table 1).
Table 1
Parameters | All (N=3,381) | Survivor (n=2,444) | Non-survivor (n=937) | P |
---|---|---|---|---|
Age, years | 70.02±12.55 | 68.83±12.75 | 73.12±11.46 | <0.001 |
Sex | 0.378 | |||
Male | 1,817 (53.74) | 1,302 (53.27) | 515 (54.96) | |
Female | 1,564 (46.26) | 1,142 (46.73) | 422 (45.04) | |
BMI, kg/m2 | 28.67±7.84 | 28.85±7.84 | 28.22±7.80 | 0.036 |
Smoking | 178 (5.26) | 137 (5.61) | 41 (4.38) | 0.152 |
Ethnicity | <0.001 | |||
White | 2,410 (71.28) | 1,756 (71.85) | 654 (69.80) | |
Black | 265 (7.84) | 216 (8.84) | 49 (5.23) | |
Hispanic | 61 (1.80) | 47 (1.92) | 14 (1.49) | |
Asian | 74 (2.19) | 60 (2.45) | 14 (1.49) | |
Other | 571 (16.89) | 365 (14.93) | 206 (21.99) | |
ICU type | <0.001 | |||
CCU | 1,007 (29.78) | 768 (31.42) | 239 (25.51) | |
MICU | 1,558 (46.08) | 1,062 (43.45) | 496 (52.93) | |
SICU | 300 (8.87) | 218 (8.92) | 82 (8.75) | |
CSRU | 369 (10.91) | 293 (11.99) | 76 (8.11) | |
TSICU | 147 (4.35) | 103 (4.21) | 44 (4.70) | |
Comorbidities | ||||
AMI | 314 (9.29) | 237 (9.70) | 77 (8.22) | 0.185 |
Coronary heart disease | 1,274 (37.68) | 991 (40.55) | 283 (30.20) | <0.001 |
Hypertension | 1,283 (37.95) | 986 (40.34) | 297 (31.70) | <0.001 |
Atrial fibrillation | 1,460 (43.18) | 997 (40.79) | 463 (49.41) | <0.001 |
Diabetes | 1,160 (34.31) | 856 (35.02) | 304 (32.44) | 0.157 |
Liver cirrhosis | 163 (4.82) | 86 (3.52) | 77 (8.22) | <0.001 |
CKD | 639 (18.90) | 487 (19.93) | 152 (16.22) | 0.014 |
Cerebrovascular disease | 196 (5.80) | 119 (4.87) | 77 (8.22) | <0.001 |
COPD | 169 (5.00) | 122 (4.99) | 47 (5.02) | 0.977 |
Scorning system | ||||
SOFA | 5 (3–8) | 4 (3–7) | 7 (4–10) | <0.001 |
SAPSII | 41 (33–51) | 38 (30–47) | 49 (41–59) | <0.001 |
APSIII | 49 (38–62) | 45 (35–58) | 60 (47–77) | <0.001 |
GWTG-HF | 45 (39–50) | 44 (38–49) | 48 (42–53) | <0.001 |
MAP | 80.67 (70–92.33) | 81.67 (71.67–93.33) | 77.33 (67–89.50) | <0.001 |
ALBI | –1.72 (–2.04 to –1.32) | –1.80 (–2.09 to –1.44) | –1.43 (–1.80 to –0.99) | <0.001 |
AST | 69 (32–232) | 64 (31–198.75) | 99 (36–358.07) | <0.001 |
INR | 1.40 (1.20–1.96) | 1.40 (1.20–1.82) | 1.60 (1.30–2.30) | <0.001 |
RDW | 16.10 (14.90–17.70) | 15.86 (14.70–17.20) | 16.90 (15.50–19.10) | <0.001 |
Data are presented as the mean ± standard deviation, count (percentage), or median (interquartile range). BMI, body mass index; ICU, intensive care unit; CCU, cardiovascular care unit; MICU, medical intensive care unit; SICU, surgery intensive care unit; CSRU, cardiac surgery intensive care unit; TSICU, trauma surgery intensive care unit; AMI, acute myocardial infarction; CKD, chronic kidney disease; COPD, chronic obstructive pulmonary disease; SOFA, sequential organ failure assessment; SAPSII, simplified acute physiology score II; ASPIII, acute physiology score III; GWTG-HF, Get With the Guidelines-Heart Failure risk score; MAP, mean arterial pressure; ALBI, albumin-bilirubin score; AST, aspartate aminotransferase; INR, international normalized ratio; RDW, red cell volume distribution width.
Comparisons based on ALBI quartiles
A quadratic linear relationship between the ALBI score and short-term mortality was found (Figure 2). Based on the relationship, the patients were divided into the following 3 groups: (I) Group A (ALBI score <−2.0); (II) Group B (ALBI score from −2.0 to −1.25); and (III) Group C (ALBI score >−1.25). Unadjusted outcomes according to the ALBI scores are shown in Table 2. Without adjusting for covariates, the in-hospital (11.39%, 19.57%, and 41.35%, respectively; P<0.001) and short-term (15.19%, 25.48%, and 48.35%, respectively; P<0.001) mortality rates increased significantly as ALBI scores increased. ICU length of stay, hospital length of stay, and the SOFA score, SAPSII, and APSIII also increased as ALBI scores increased (all P<0.001; Table 2).
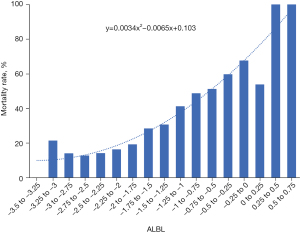
Table 2
Parameters | Group A (n=948) | Group B (n=1,676) | Group C (n=757) | P |
---|---|---|---|---|
ICU LOS, days | 3.02 (1.92–5.09) | 3.66 (2.03–7.05) | 6.40 (3.12–13.71) | <0.001 |
Hospital LOS, days | 6.92 (4.34–10.66) | 8.88 (5.26–14.45) | 15.58 (7.98–25.38) | <0.001 |
In-hospital mortality | 108 (11.39%) | 328 (19.57%) | 313 (41.35%) | <0.001 |
Follow-up, days | 34 (31.22–37.96) | 34.92 (30.13–40.32) | 35.21 (14.79–48.60) | 0.016 |
Short-term mortality | 144 (15.19%) | 427 (25.48%) | 366 (48.35%) | <0.001 |
SOFA | 4 (2–6) | 5 (3–7) | 8 (5–10) | <0.001 |
SAPSII | 37 (29–45) | 41 (33–50) | 48 (38–59) | <0.001 |
APSIII | 42 (33–55) | 48 (37–60) | 61 (48–77.50) | <0.001 |
GWTG-HF | 43 (38–48) | 45 (39–50) | 47 (41–52) | <0.001 |
The in-hospital mortality and short-term mortality data are the presented as count (percentage); the other data presented as the median (interquartile range). LOS, length of stay; SOFA, sequential organ failure assessment; SAPSII, simplified acute physiology score III; APSIII, acute physiology score III; MAP, mean arterial pressure; GWTG-HF, Get With the Guidelines-Heart Failure risk score; ALBI, albumin-bilirubin score. Group A, ALBI score <−2.0, Group B, ALBI score ≥−2.0 and ALBI score <−1.25, Group C, ALBI score ≥−1.25.
Associations between the ALBI score and outcomes
Of the 3381 patients, 937 all-cause deaths were observed from admission to 28 days after discharge; thus, the short-term all-cause mortality rate was 27.71%. Kaplan-Meier survival curves for short-term mortality were constructed for each ALBI group (Figure 3A), and the log-rank test revealed statistically significant differences among the groups (P<0.001). Of the patients, 1,986 were followed up with for 4 years. During the 4-year follow-up period there were 1,089 all-cause deaths; thus, the 4-year all-cause mortality rate was 54.83%. A higher ALBI score was associated with an increasing risk of 4-year all-cause mortality (Figure 3B). The same trend was also observed when patients with liver cirrhosis were excluded (Figure 3C,3D).
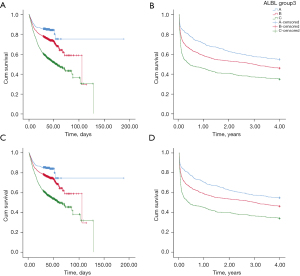
A multivariate logistic regression analysis was performed to assess potential risk factors for short-term all-cause mortality and 4-year all-cause mortality (Table 3). The multivariate analysis was adjusted for age, sex, body mass index, smoking, coronary heart disease, hypertension, diabetes, liver cirrhosis, acute myocardial infarction, atrial fibrillation, liver disease, chronic kidney disease, cerebrovascular disease, COPD, ALBI, MAP, AST, INR, RDW, and SAPSII. The odds ratio (OR) for the short-term all-cause mortality of ALBI Group B was 1.42 [95% confidence interval (CI): 1.13 to 1.77, P=0.002] and the OR for ALBI Group C 2.41 (95% CI: 1.85–3.15, P<0.001) (Group A as the reference). The OR for 4-year all-cause mortality of ALBI Group B was 1.17 (95% CI: 0.92–1.48, P=0.213) and for ALBI Group C was 1.37 (95% CI: 1.00–1.86, P=0.048) (Group A as the reference).
Table 3
Parametersa | Short-term mortality | 4-year mortality | |||
---|---|---|---|---|---|
OR (95% CI) | P | OR (95% CI) | P | ||
ALBI group | <0.001 | 0.138 | |||
A | Ref. | – | Ref. | – | |
B | 1.42 (1.13–1.77) | 0.002 | 1.17 (0.92–1.48) | 0.213 | |
C | 2.41 (1.85–3.15) | <0.001 | 1.37 (1.00–1.86) | 0.048 | |
Age | 1.03 (1.02–1.04) | <0.001 | 1.05 (1.04–1.06) | <0.001 | |
Sex | |||||
Male | Ref. | – | |||
Female | 0.82 (0.69–0.97) | 0.024 | |||
Smoking | |||||
No | Ref. | – | |||
Yes | 0.51 (0.23–1.11) | 0.091 | |||
Coronary heart disease | |||||
No | Ref. | – | Ref. | – | |
Yes | 0.75 (0.63–0.90) | 0.002 | 0.70 (0.56–0.87) | 0.001 | |
Hypertension | |||||
No | Ref. | – | Ref. | – | |
Yes | 0.71 (0.59–0.86) | <0.001 | 0.78 (0.63–0.96) | 0.020 | |
Liver cirrhosis | |||||
No | Ref. | – | |||
Yes | 1.72 (1.18–2.49) | 0.005 | |||
CKD | |||||
No | Ref. | – | |||
Yes | 0.59 (0.46–0.74) | <0.001 | |||
Cerebrovascular disease | |||||
No | Ref. | – | |||
Yes | 2.20 (1.57–3.09) | <0.001 | |||
COPD | |||||
No | Ref. | – | |||
Yes | 1.74 (1.08–2.81) | 0.022 | |||
AST | 1.00 (1.00–1.00) | <0.001 | |||
RDW | 1.13 (1.09–1.17) | <0.001 | 1.18 (1.13–1.24) | <0.001 | |
SAPSIIb | 1.05 (1.04–1.05) | <0.001 | 1.03 (1.02–1.04) | <0.001 |
a, only variables with a P value <0.05 in the logistic regression analysis are presented in this table. b, SAPSII includes age, heart rate, systolic blood pressure, body temperature, PaO2/FiO2, urine volume, white blood cell count, blood potassium concentration, serum sodium concentration, blood HCO−3 concentration, blood bilirubin concentration, Glasgow Coma Scale score, chronic disease, and the type of ICU. To avoid including too many repetitive variables, SOFA score, APSIII, GWTG-HF score, and age were not included in the multivariate linear logistic regression analysis. AST, aspartate aminotransferase; CKD, chronic kidney disease; RDW, red cell volume distribution width; OR, odds ratio; CI, confidence interval; ICU, intensive care unit.
The Kaplan-Meier survival curves showed a cross between ALBI Group B and ALBI Group C in relation to both short-term mortality and 4-year mortality, which indicates that the data did not meet the proportional-hazards assumption. Thus, a time-dependent Cox regression model was used to evaluate the relationship between the ALBI score and short-term and 4-year all-cause mortality that included time-dependent covariates. The results showed that the ALBI score exhibited good predictive value, and was an independent predictive factor for short-term all-cause mortality (Table 4). The hazard ratio (HR) for ALBI Group B was 1.26 (95% CI: 1.04–1.53, P=0.020) and for ALBI Group C was 1.43 (95% CI: 1.13–1.83, P=0.004) (Group A the reference). The results also showed that a higher SAPSII was associated with higher short-term mortality (HR =1.04, 95% CI: 1.03–1.04, P<0.001) (Table 5).
Table 4
Parameters | β (SE) | HR (95% CI) | P |
---|---|---|---|
Short-term mortality | |||
ALBI group | |||
A | – | Ref. | 0.014 |
B | 0.23 (0.10) | 1.26 (1.04–1.53) | 0.020 |
C | 0.36 (0.12) | 1.43 (1.13–1.83) | 0.004 |
SAPSII | 0.04 (0.00) | 1.04 (1.03–1.04) | <0.001 |
4-year mortality | |||
ALBI group | |||
A | – | Ref. | <0.001 |
B | 0.30 (0.09) | 1.34 (1.13–1.59) | <0.001 |
C | 0.49 (0.11) | 1.63 (1.32–2.00) | <0.001 |
SAPSII | 0.03 (0.00) | 1.03 (1.03–1.03) | <0.001 |
Hypertension | −0.20 (0.07) | 0.82 (0.72–0.93) | 0.002 |
T_COV_ | −0.19 (0.05) | 0.82 (0.74–0.91) | <0.001 |
Omnibus test of model coefficients, P<0.001. ALBI, albumin-bilirubin score; SAPSII, simplified acute physiology score II; HR, hazards ratio; SE, standard error; CI, confidence interval.
Table 5
Score system | AUC (SE) | 95% CI of AUC | Pa | Pb | Pc | Suggestive cut-off | Sensitivity | Specificity | Youden index |
---|---|---|---|---|---|---|---|---|---|
Short-term mortality | |||||||||
ALBI | 0.676 (0.010) | 0.655–0.696 | <0.001 | <0.001 | <0.001 | −1.745 | 0.71 | 0.55 | 0.26 |
GWTG-HF-ALBI | 0.713 (0.010) | 0.693–0.732 | <0.001 | 0.059 | – | 0.288 | 0.65 | 0.69 | 0.34 |
GWTG-HF | 0.643 (0.011) | 0.622–0.664 | <0.001 | <0.001 | <0.001 | 47.5 | 0.52 | 0.70 | 0.22 |
SOFA | 0.673 (0.010) | 0.653–0.693 | <0.001 | <0.001 | <0.001 | 6.5 | 0.52 | 0.73 | 0.25 |
APSIII | 0.711 (0.010) | 0.691–0.730 | <0.001 | 0.008 | 0.876 | 51.5 | 0.68 | 0.64 | 0.32 |
SAPSII | 0.733 (0.009) | 0.715–0.752 | <0.001 | – | 0.059 | 42.5 | 0.70 | 0.65 | 0.35 |
4-year mortality | |||||||||
ALBI | 0.596 (0.013) | 0.571–0.622 | <0.001 | <0.001 | <0.001 | −1.812 | 0.68 | 0.48 | 0.15 |
GWTG-HF-ALBI | 0.650 (0.013) | 0.626–0.675 | <0.001 | 0.204 | – | 0.288 | 0.53 | 0.69 | 0.23 |
GWTG-HF | 0.638 (0.013) | 0.613–0.663 | <0.001 | 0.004 | 0.002 | 45.5 | 0.55 | 0.66 | 0.22 |
SOFA | 0.592 (0.013) | 0.567–0.618 | <0.001 | <0.001 | <0.001 | 4.5 | 0.63 | 0.50 | 0.13 |
APSIII | 0.644 (0.013) | 0.620–0.669 | <0.001 | 0.001 | 0.219 | 48.5 | 0.61 | 0.61 | 0.23 |
SAPSII | 0.681 (0.012) | 0.657–0.705 | <0.001 | – | 0.204 | 39.5 | 0.68 | 0.60 | 0.29 |
Pa: AUC progressive significance of each score system; Pb: comparison of the AUC of the SAPSII with that of other scoring systems (chi-square test); Pc: comparison of the AUC of the GWTG-HF-ALBI score with that of other scoring systems (chi-square test). ALBI, albumin-bilirubin score; AUC, area under the receiver operating characteristic curve; GWTG-HF-ALBI, logistic regression predictive model with adjusted covariates combining GWTG-HF and ALBI; GWTG-HF, Get With the Guidelines-Heart Failure risk score; SOFA, sequential organ failure assessment; APSIII, acute physiology score III; SAPSII, simplified acute physiology score II.
A higher ALBI score was also an independent risk factor for higher 4-year all-cause mortality. The HR for ALBI Group B was 1.34 (95% CI: 1.13–1.59, P<0.001) and for ALBI Group C was 1.63 (95% CI: 1.32–2.00, P<0.001) (Group A the reference). The results also showed that a higher SAPSII was predictive of higher 4-year all-cause mortality (HR =1.03, 95% CI: 1.03–1.03, P<0.001). However, the presence of hypertension was associated with a lower 4-year all-cause mortality rate (HR =0.82, 95% CI: 0.72–0.93, P=0.001; Table 4).
New models and evaluation of predictive value
The ROC curves showing the performance of the ALBI score, the GWTG-HF score, the GWTG-HF-ALBI risk score, the SOFA score, the APSIII, and the SAPSII in predicting short-term and 4-year all-cause mortality are shown in Figure 4, and the area under the ROC curves (AUCs) of these methods are shown in Table 5. The results showed that the ALBI score and the GWTG-HF score exhibited the best predictive value for short-term all-cause mortality [ALBI score AUC =0.676 (95% CI: 0.655–0.696, P<0.001]; GWTG-HF score AUC =0.643 (95% CI: 0.622–0.664; P<0.001)]. After adjusting for the ALBI score, the new GWTG-HF-ALBI model exhibited the highest predictive value (AUC =0.713, 95% CI: 0.0.693–0.732, P<0.001). The AUC of the SOFA score for predicting short-term mortality was 0.673 (95% CI: 0.653–0.693, P<0.001), that of the APSIII was 0.711(95% CI: 0.691–0.730, P<0.001), and that of the SAPSII was 0.733 (95% CI: 0.715–0.752, P<0.001). Of these 3 methods, the predictive value of the SAPSII was significantly greater than that of the SOFA and the APSIII (P<0.001 and P=0.008, respectively). The predictive value of the GWTG-HF-ALBI model was higher than that of the ALBI score, GWTG-HF score, and SOFA core (all; P<0.001), and similar to that of the APSIII and the SAPSII (P=0.876 and P=0.059, respectively).
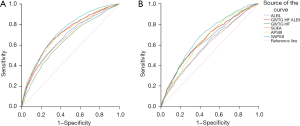
The ALBI score and GWTG-HF-ALBI score exhibited good predictive value for 4-year all-cause mortality; however, the predictive values of all the score systems used to predict 4-year all-cause mortality were generally lower than those used to predict short-term all-cause mortality. The AUC of the ALBI score for predicting 4-year all-cause mortality was 0.596 (95% CI: 0.571–0.622, P<0.001), that of the GWTG-HF model was 0.638 (95% CI: 0.613–0.663, P<0.001), that of the GWTG-HF-ALBI model was 0.650 (95% CI: 0.626–0.675, P<0.001), that of SOFA score was 0.592 (95% CI: 0.0.567–0.618, P<0.001), that of the APSII was 0.644 (95% CI: 0.620–0.669, P<0.001), and that of the SAPSII was 0.681 (95% CI: 0.657–0.705, P<0.001). The predictive values of the GWTG-HF-ALBI model and the SAPSII for 4-year all-cause mortality were similar (P=0.204).
Nomograms for the GWTG-HF + ALBI model and single GWTG-HF-ALBI model are shown in Figure 5, respectively. Through the nomograms, the predictive value for mortality was constructed according to the ALBI and GWTG-HF scores. A goodness-of-fit test was performed to determine any discrepancy between the observed values and those that would be expected of the GWTG-HF-ALBI in a normal distribution. The results showed that GWTG-HF-ALBI exhibited good fitness for predicting short-term and 4-year all-cause mortality (P=0.392 and P=0.302, respectively).
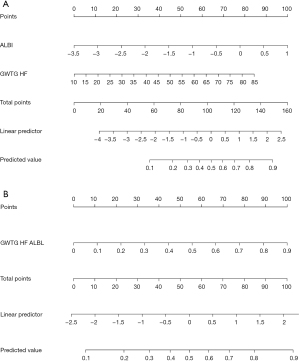
Discussion
In this study we used a large ICU dataset (from MIMICIII) to determine the predictive value of the ALBI score in critically ill patients with HF. The results showed that the ALBI score was predictive of both short-term and 4-year all-cause mortality in critical ill patients with HF, but its predictive value was higher for short-term mortality than 4-year mortality. The findings were similar when patients with liver cirrhosis were excluded. Further, a high ALBI score was an independent risk factor for both short-term and 4-year all-cause mortality. We also found that the predictive value of the GWTG-HF score for short-term mortality was not as high as previously reported (10,11). When we developed a new model that included both the ALBI and GWTG-HF (GWTG-HF-ALBI) scores, we found that its predictive value for short-term all-cause mortality was higher than that of the GWTG-HF model alone, the ALBI score alone, and the SOFA score alone, and was equally as good as the APSIII and SAPS II at predicting short-term all-cause mortality. The predictive value of the GWTG-HF-ALBI model for 4-year all-cause mortality was lower than for short-term all-cause mortality, but comparable to that of other scoring systems.
Hepatomegaly, elevated bilirubin and hypoalbuminemia usually accompany advanced HF because of passive congestion (23,24), and elevated bilirubin and hypoalbuminemia are associated with increased risk of death in HF patients (25-27). The ALBI score, which includes serum bilirubin and albumin levels, was developed as a new model to assess liver function and predict the survival of patients with liver disease. Since it was first reported in 2015, the ALBI score has been widely used in clinical practice (16). Research has shown that the ALBI score can reliably predict the prognosis and overall survival of patients with liver disease (28). Other research has shown that a high ALBI score is related to elevated serum creatinine levels in patients with HCC after arterial chemoembolization and arterial implantation chemotherapy (18). It has also been shown to be associated with in-hospital mortality in critically ill patients with acute pancreatitis (29). However, few studies have examined the predictive value of the ALBI score in critically ill patients with HF.
Recently, Matsue et al. (20) conducted a prospective, multi-center registry study on the prognostic value of the ALBI score in relation to acute HF presentation in the emergency department. The results showed that the ALBI score is a promising marker for predicting the prognosis of such patients, as it includes information on fluid overload. Our results showed that a high ALBI score was associated with higher short-term and 4-year all-cause mortality in critically ill patients with HF. We also found that the predictive value of the ALBI score in relation to short-term and 4-year all-cause mortality in critically ill patients with HF was better than that of the GWTG-HF score, which is the method commonly used to predict in-hospital mortality in patients with HF (10).
The GWTG-HF risk-score model uses race (black vs. non-black), age, heart rate, COPD, blood pressure, BUN level, and serum sodium level. It was developed using GWTG-HF program data from the American Heart Association to predict the in-hospital mortality of patients with acute HF (10). Compared to other HF scoring systems (5,7-9), the items in the GWTG-HF are easy to administer; however, its predictive value has been shown to vary with the study population. Based on data from the American Heart Association, the GWTG-HF risk-score AUC was reported to be 0.75 for the mortality of in-hospital patients with HF (10). In unselected cardiac ICU patients, the GWTG-HF risk-score AUC for the hospital mortality of all HF patients was 0.73, the GWTG-HF risk-score AUC for patients with a discharge diagnosis of HF was 0.69, and the GWTG-HF risk-score AUC for patients with a no discharge diagnosis of HF was 0.75; values which were similar to those of the EFFECT, OPTIMIZE-HF, and ADHERE risk scores (11). Another study reported the AUC of the GWTG-HF risk score for all-cause death in patients with HF after discharge was 0.687 (30). In our study, we found that the AUC of the GWTG-HF risk score for short-term mortality was 0.643 and for 4-year all-cause mortality was 0.638 in critically ill patients with HF; however, it should be noted that these values are lower than those previously reported. The lower prediction values of our study may be due to the more complicated medical conditions and complications of ICU patients.
Based on the high predictive value of the ALBI score, we developed a new model that combined the ALBI score and the GWTG-HF (GWTG-HF-ALBI) score to predict short-term and 4-year all-cause mortality in critically ill patients with HF. After adjusting for covariates, the AUC of the GWTG-HF-ALBI risk score was 0.713 for short-term all-cause mortality and 0.650 for 4-year all-cause mortality; values which were higher than those of the GWTG-HF, ALBI, and SOFA scores alone, and similar to those of the APSIII and SAPSII scores.
Our study also showed that the SOFA score, the APSIII, and the SAPSII were relatively accurate at predicting short-term all-cause mortality in our patient population (AUCs =0.673, 0.711, and 0.733, respectively), but were less accurate at predicting 4-year all-cause mortality (AUCs =0.592, 0.644, and 0.681, respectively). Thus, the SAPSII had the highest predictive value for both short-term and 4-year all-cause mortality and was similar to that of the new GWTG-HF-ALBI risk score. However, the SAPSII contains 15 items (12), 1 of which is the GCS (15) which is subjective and time-consuming to administer. Conversely, the GWTG-HF-ALBI risk-score model contains only 9 items, all of which are easy to obtain and not subjective. Thus, the GWTG-HF-ALBI risk score is much simpler to derive and may be more suitable than SAPSII at predicting short-term and 4-year all-cause mortality in critically ill patients with HF.
This study had several limitations. First, it was a retrospective cohort study; thus, the results need to be verified in future prospective studies. Second, because of the nature of the database and follow-up systems, it was not possible to distinguish between chronic and acute HF patients; thus, we studied “HF” as a whole. In addition, due to database limitations, we could only extract all-cause mortality data. Based on the results of this study, we plan to conduct a prospective cohort study to examine the use of the ALBI score and GWTG-HF-ALBI risk score in chronic and acute HF patients, and specifically examine short- and long-term cardiovascular death rates.
Conclusions
An elevated ALBI score is a readily available independent predictor of short-term and 4-year all-cause mortality in critically ill patients with HF. The newly developed model that combines the ALBI score and GWTG-HF score (i.e., the GWTG-HF-ALBI risk-score model) has good predictive value for short-term and 4-year all-cause mortality for critically ill patients with HF. Notably, the GWTG-HF-ALBI risk score is based on data that are easy to obtain and not subjective, making it more convenient than other risk-scoring systems. Future prospective studies should be conducted on the GWTG-HF-ALBI risk score.
Acknowledgments
Funding: This work was supported by the Guangzhou Science and Technology Project (201802010048), the National Natural Science Foundation of China (81773176), the National Natural Science Foundation of China (81900320), the Medical Science and Technology Research Project of Guangdong Province (C2019107), basic research funding from Sun Yat-Sen University (19ykpy40), the Natural Science Foundation of Guangdong Province (2020A1515010599), and the Guangdong Natural Science Foundation (2018A0303130070).
Footnote
Reporting Checklist: The authors have completed the STARD reporting checklist. Available at https://dx.doi.org/10.21037/apm-21-3424
Conflicts of Interest: All authors have completed the ICMJE uniform disclosure form (available at https://dx.doi.org/10.21037/apm-21-3424). The authors have no conflicts of interest to declare.
Ethical Statement: The authors are accountable for all aspects of the work in ensuring that questions related to the accuracy or integrity of any part of the work are appropriately investigated and resolved. The study was conducted in accordance with the Declaration of Helsinki (as revised in 2013).
Open Access Statement: This is an Open Access article distributed in accordance with the Creative Commons Attribution-NonCommercial-NoDerivs 4.0 International License (CC BY-NC-ND 4.0), which permits the non-commercial replication and distribution of the article with the strict proviso that no changes or edits are made and the original work is properly cited (including links to both the formal publication through the relevant DOI and the license). See: https://creativecommons.org/licenses/by-nc-nd/4.0/.
References
- Yancy CW, Jessup M, Bozkurt B, et al. 2017 ACC/AHA/HFSA Focused Update of the 2013 ACCF/AHA Guideline for the Management of Heart Failure: A Report of the American College of Cardiology/American Heart Association Task Force on Clinical Practice Guidelines and the Heart Failure Society of America. Circulation 2017;136:e137-61. [Crossref] [PubMed]
- Ponikowski P, Anker SD, AlHabib KF, et al. Heart failure: preventing disease and death worldwide. ESC Heart Fail 2014;1:4-25. [Crossref] [PubMed]
- Ambrosy AP, Fonarow GC, Butler J, et al. The global health and economic burden of hospitalizations for heart failure: lessons learned from hospitalized heart failure registries. J Am Coll Cardiol 2014;63:1123-33. [Crossref] [PubMed]
- Lee JH, Kim MS, Kim EJ, et al. KSHF Guidelines for the Management of Acute Heart Failure: Part I. Definition, Epidemiology and Diagnosis of Acute Heart Failure. Korean Circ J 2019;49:1-21. [Crossref] [PubMed]
- Okazaki H, Shirakabe A, Hata N, et al. New scoring system (APACHE-HF) for predicting adverse outcomes in patients with acute heart failure: evaluation of the APACHE II and Modified APACHE II scoring systems. J Cardiol 2014;64:441-9. [Crossref] [PubMed]
- Chen YJ, Sung SH, Cheng HM, et al. Performance of AHEAD Score in an Asian Cohort of Acute Heart Failure With Either Preserved or Reduced Left Ventricular Systolic Function. J Am Heart Assoc 2017;6:e004297. [Crossref] [PubMed]
- Fonarow GC, Adams KF Jr, Abraham WT, et al. Risk stratification for in-hospital mortality in acutely decompensated heart failure: classification and regression tree analysis. JAMA 2005;293:572-80. [Crossref] [PubMed]
- Lee DS, Austin PC, Rouleau JL, et al. Predicting mortality among patients hospitalized for heart failure: derivation and validation of a clinical model. JAMA 2003;290:2581-7. [Crossref] [PubMed]
- Abraham WT, Fonarow GC, Albert NM, et al. Predictors of in-hospital mortality in patients hospitalized for heart failure: insights from the Organized Program to Initiate Lifesaving Treatment in Hospitalized Patients with Heart Failure (OPTIMIZE-HF). J Am Coll Cardiol 2008;52:347-56. [Crossref] [PubMed]
- Peterson PN, Rumsfeld JS, Liang L, et al. A validated risk score for in-hospital mortality in patients with heart failure from the American Heart Association get with the guidelines program. Circ Cardiovasc Qual Outcomes 2010;3:25-32. [Crossref] [PubMed]
- Lyle M, Wan SH, Murphree D, et al. Predictive Value of the Get With The Guidelines Heart Failure Risk Score in Unselected Cardiac Intensive Care Unit Patients. J Am Heart Assoc 2020;9:e012439. [Crossref] [PubMed]
- Le Gall JR, Lemeshow S, Saulnier F. A new Simplified Acute Physiology Score (SAPS II) based on a European/North American multicenter study. JAMA 1993;270:2957-63. [Crossref] [PubMed]
- Beck DH, Smith GB, Pappachan JV, et al. External validation of the SAPS II, APACHE II and APACHE III prognostic models in South England: a multicentre study. Intensive Care Med 2003;29:249-56. [Crossref] [PubMed]
- Vincent JL, de Mendonça A, Cantraine F, et al. Use of the SOFA score to assess the incidence of organ dysfunction/failure in intensive care units: results of a multicenter, prospective study. Working group on "sepsis-related problems" of the European Society of Intensive Care Medicine. Crit Care Med 1998;26:1793-800. [Crossref] [PubMed]
- Chesnut RM. Glasgow Coma Score versus severity systems in head trauma. Crit Care Med 1998;26:10-1. [Crossref] [PubMed]
- Johnson PJ, Berhane S, Kagebayashi C, et al. Assessment of liver function in patients with hepatocellular carcinoma: a new evidence-based approach-the ALBI grade. J Clin Oncol 2015;33:550-8. [Crossref] [PubMed]
- Mai RY, Wang YY, Bai T, et al. Combination Of ALBI And APRI To Predict Post-Hepatectomy Liver Failure After Liver Resection For HBV-Related HCC Patients. Cancer Manag Res 2019;11:8799-806. [Crossref] [PubMed]
- Hayashi M, Abe K, Fujita M, et al. Acute kidney injury after platinum-based transcatheter arterial chemoembolization and transarterial infusion chemotherapy in patients with hepatocellular carcinoma. Jpn J Clin Oncol 2020;50:36-43. [Crossref] [PubMed]
- Carvalho MVH, Kroll PC, Kroll RTM, et al. Cirrhotic cardiomyopathy: the liver affects the heart. Braz J Med Biol Res 2019;52:e7809. [Crossref] [PubMed]
- Matsue Y, Kagiyama N, Yamaguchi T, et al. Clinical and Prognostic Values of ALBI Score in Patients With Acute Heart Failure. Heart Lung Circ 2020;29:1328-37. [Crossref] [PubMed]
- Saeed M, Villarroel M, Reisner AT, et al. Multiparameter Intelligent Monitoring in Intensive Care II: a public-access intensive care unit database. Crit Care Med 2011;39:952-60. [Crossref] [PubMed]
- Johnson AE, Pollard TJ, Shen L, et al. MIMIC-III, a freely accessible critical care database. Sci Data 2016;3:160035. [Crossref] [PubMed]
- Ford RM, Book W, Spivey JR. Liver disease related to the heart. Transplant Rev (Orlando) 2015;29:33-7. [Crossref] [PubMed]
- Durante-Mangoni E, Parrella A, Pafundi PC, et al. Liver histopathological findings in advanced heart failure: a reappraisal of cardiac cirrhosis concept. Intern Emerg Med 2019;14:931-40. [Crossref] [PubMed]
- Allen LA, Felker GM, Pocock S, et al. Liver function abnormalities and outcome in patients with chronic heart failure: data from the Candesartan in Heart Failure: Assessment of Reduction in Mortality and Morbidity (CHARM) program. Eur J Heart Fail 2009;11:170-7. [Crossref] [PubMed]
- Uthamalingam S, Kandala J, Daley M, et al. Serum albumin and mortality in acutely decompensated heart failure. Am Heart J 2010;160:1149-55. [Crossref] [PubMed]
- Horwich TB, Kalantar-Zadeh K, MacLellan RW, et al. Albumin levels predict survival in patients with systolic heart failure. Am Heart J 2008;155:883-9. [Crossref] [PubMed]
- Deng M, Ng SWY, Cheung ST, et al. Clinical application of Albumin-Bilirubin (ALBI) score: The current status. Surgeon 2020;18:178-86. [Crossref] [PubMed]
- Shi L, Zhang D, Zhang J. Albumin-bilirubin score is associated with in-hospital mortality in critically ill patients with acute pancreatitis. Eur J Gastroenterol Hepatol 2020;32:963-70. [Crossref] [PubMed]
- Suzuki S, Yoshihisa A, Sato Y, et al. Clinical Significance of Get With the Guidelines-Heart Failure Risk Score in Patients With Chronic Heart Failure After Hospitalization. J Am Heart Assoc 2018;7:e008316. [Crossref] [PubMed]
(English Language Editor: L. Huleatt)