Changes in brain network properties in major depressive disorder following electroconvulsive therapy: a combined static and dynamic functional magnetic resonance imaging study
Introduction
Major depressive disorder (MDD), as a common mood disorder, is a kind of severe mental disease from which more than 264 million people worldwide suffer (1). It is well known that MDD is a major cause of disability and a significant risk factor for suicide (2-5), contributing to a growing global burden on the economy and health systems of the society (6).
Electroconvulsive therapy (ECT) provides effective and tolerable treatment to relieve depressive symptoms in patients with MDD (7-9), especially for patients who failed to respond to conventional pharmacotherapies (10,11). Because of such advantages, the use of ECT in psychiatric hospitals has largely increased over the past few years in China, the United States and the rest of the world (12). However, the ECT is not effective for all patients (13), and the underlying mechanisms are still poorly understood. To uncover the physiological markers of ECT response and advance the future treatment optimization, there is an urgent need to know how ECT plays a role in the treatment of MDD.
Whole-brain connectivity analysis based on functional magnetic resonance imaging (fMRI) (14,15) provides the possibility to explore whether and how ECT alters brain function in MDD. For instance, a study including 23 MDD patients and 25 healthy controls has reported significant increases in functional connectivity in the left anterolateral prefrontal cortex and dorsolateral prefrontal cortex after ECT compared with pre-treatment (16). Many recent studies have also suggested that the changes in the functional connectivity of the prefrontal cortex after ECT may be closely correlated with the alleviation of clinical symptoms of MDD (17-19). While the above results significantly deepen our understanding of ECT-related effects in MDD, there is an important limitation that all these studies were performed assuming the patterns of brain connectivity are static. Recent advances in fMRI studies have suggested that functional connectivity patterns are changing dynamically over time, and analysis of dynamic functional connectivity might provide important information on the biological indicators of brain networks in mental illness which is ignored by static fMRI measures (20), such as schizophrenia (21) and bipolar disorder (22). At present, it is believed that combining the dynamic and static functional network metrics could provide more powerful and sensitive classifications when compared to the conventional static functional connectivity-based methods (23,24). Notably, a number of studies have reported altered dynamic functional connectivity in MDD patients (25-28), bringing the possibility that these measures may be modulated by ECT, which remains to be tested.
The purpose of the present study was to explore the possible neurobiological mechanism of ECT in patients with MDD by using both the static and dynamic brain network properties. Resting-state fMRI data were scanned from MDD patients both before and after ECT and brain network analysis was conducted to (I) investigate the alterations in static and dynamic brain network induced by ECT, and (II) verify whether these alterations would be related to the alleviation of depressive symptoms in MDD patients. We present the following article in accordance with the STROBE reporting checklist (available at https://apm.amegroups.com/article/view/10.21037/apm-21-2723/rc).
Methods
Participants, designs and assessments
Eleven MDD patients were recruited to the study from The Second Xiangya Hospital, Central South University according to the following inclusion criteria at baseline: (I) being at least 16 years of age; (II) right-handed, Han Chinese ethnicity; (III) diagnosed with MDD according to the Diagnostic and Statistical Manual of Mental Disorders-Fifth Edition (DSM-5); (IV) had at least moderate current depression, as defined by the Hamilton Depression Scale (HAMD) score ≥17 (29); (V) met the clinical indications for ECT (suicide risk, refractory depression, etc.) (30).
All subjects underwent fMRI scans twice: once at baseline (T0) and once after completing a full course of ECT (T1). ECT was administered 3 times per week on Monday, Wednesday, and Friday with a break of weekends. In line with a previous study by Zeng et al. (31), all participants received at least 6 times of ECT sessions; after the 6th ECT session, ECTs were continued if patients’ depressive symptoms had not improved sufficiently as decided by experienced clinicians, but up to 8 ECT sessions in total. ECT and EEG monitoring were performed using a spECTrum-5000Q device (First Product Condar Technology Co., LTD, Beijing, China), and the stimulation parameters were set as follows: placing the original bitemporal stimulation, constant current =600–700 mA, pulse frequency =100 Hz, pulse width =1.0–1.2 ms, and stimulus dose adjusted by altering the duration of train. Two patients were lost because of refusing to complete a full course of ECT, and a total of nine patients were included in the final analysis.
At both time points (T0 and T1), the HAMD was used to measure the severity of current depressive symptoms; the Beck Suicidal Ideation Scale (BSIS) was used to assess the risk of suicide at the nearly 2 weeks (BSIS-2-weeks) and at the worst time of depression (BSIS-worst) (32); the Information (WAIS-I) and Digit Symbol (WAIS-DS) subtests of Wechsler Adult Intelligence Scale (WAIS), Chinese version was used to respectively evaluate two domains of cognitive function, verbal comprehension, and processing speed (33). Response to ECT was defined by at least a 50% reduction from the baseline HAMD scores (13).
The study was approved by the Ethics Committee of Second Xiangya Hospital, Central South University (No. 2016S024) and written informed consent was obtained from all participants. The study was conducted in accordance with the Declaration of Helsinki (as revised in 2013).
Imaging data acquisition and preprocessing
Resting-state fMRI and three-dimensional T1-weighted (for accurately realigning images) imaging data were collected on a Philips Gyroscan Achieva 3.0 Tesla scanner (First Product Condar Technology Co., LTD) in the axial direction. A gradient-echo echoplanar imaging (EPI) sequence was used to obtain fMRI images with the following parameters: slice thickness =4 mm, gap =0 mm, matrix =64×64, slices =36, flip angle =90°, field of view (FOV) =240×240 mm, repetition time (TR) =2,000 ms, echo time (TE) =30 ms, and total number of time points =250. A turbo field echo sequence was used to collect T1-weighted images with the following parameters: TR =7.5 ms, TE =3.7 ms, flip angle =8°, FOV =240×240 mm, acquisition matrix =256×200, slice thickness =1 mm, gap =0 mm, and number of slices =180.
Imaging data preprocessing was performed using the DPARSF software (https://rfmri.org/DPARSF) (34,35) and a standard pipeline whose details can be found elsewhere (26,36). Briefly, the pipeline includes removing the first 10 volumes, slice timing, motion realignment, spatial normalization to the standard brain space, temporal filtering (0.01–0.10 Hz), and regressing out for white matter, cerebrospinal fluid, and global mean signals. To account for head motion, the slice timing for the remaining images was corrected, and images were realigned to the first volume. The mean framewise displacement (FD) (37) was calculated for estimating head motion. All participants had a mean FD <0.2, rotations <2° and translations <2 mm which suggest acceptable head motion, and there were no differences in mean FD between the before- and after-ECT fMRI scans at the group level (P>0.05, paired t-test).
Static and dynamic network metrics
After data preprocessing, both static and dynamic brain network metrics were calculated with nodes in the network defined by Craddock’s clustering 200 regions of interest (ROIs) (38). Static network metrics were computed using the Brain Connectivity Toolbox (BCT) (http://www.brain-connec-tivity-toolbox.net/) (39). The mean time series were extracted from each of the 200 ROIs and then a 200×200 symmetric matrix was created by calculating the Pearson correlation coefficient between each pair of ROIs. Since weak and spurious connections may obscure the topology of stronger meaningful connections, a proportional thresholding processing step is set up to remove spurious edges with lower connection strength from the connectivity matrix by convention (40,41). Multiple sparsity thresholds were set from 10% to 30% with an increment of 1%, to reduce the possibility that results would be biased by a single sparsity level (42,43). All metrics were calculated at all sparsity levels from derived binary networks, including four commonly used global network metrics: characteristic path length (LP), clustering coefficient (EP), global efficiency (Eglob), and local efficiency (Eloc); and a local network metric, nodal degree of each ROI (39).
Dynamic brain networks were constructed based on the same 200 ROIs and metric named flexibility (44-48) was computed at both global and local levels to quantify the temporal stability of the brain network. The computation was performed using an open-source MATLAB code package (https://github.com/GenLouvain/GenLouvain) (49) and strictly followed a previously published study (46). Briefly, the mean time series of 200 ROIs extracted earlier were firstly divided into a series of time windows with a window length of 40 seconds, which was suggested to be suitable for producing robust results and has been widely used in previous studies (45,46,50). Within each produced time window, a 200×200 connection matrix of the whole brain was calculated using the paired Pearson correlation. Then, the flexibility of constructed dynamic brain network was computed based on the temporal stability of its time-varying community structure, which included flexibility of the whole brain and nodal flexibility for each ROI (46,51). Since the computations of flexibility could produce slightly different results in each operation due to the algorithms, we set the number of operations to 100 and regarded the mean value as a final result. The value range of flexibility is 0 to 1, and a higher value suggests a more unstable community structure of the dynamic brain network.
Statistical analyses
All statistical analyses were performed using the SPSS (version 21) software. Paired t-tests were used to compare the differences in clinical characteristics and all brain network metrics of patients between two-time points (T0 and T1). For static network metrics, their values were averaged within the sparsity of 10–30% before being fed into the paired t-tests. Pearson correlation coefficients were used to further evaluate the correlations between the changes in clinical symptom severity and changes in network metrics. Significances were set at P value less than 0.05, with no multiple comparison corrections performed considering the relatively small sample size.
Results
Demographic and clinical characteristics
As shown in Table 1, the final analyzed sample consisted of 6 female and 3 male patients, with an average age of 33.78±11.01 years. The mean time interval between T0 and T1 was 46.11±49.60 days, ranging from 14 to 165 days. As shown in Table 2, after ECT, the HAMD scores were significantly decreased compared with before the ECT (t =7.517, P<0.001; all subjects had at least a 50% reduction from the baseline HAMD scores), while no significant differences were found in the WAIS-I and WAIS-DS scores (t =−0.153, P=0.881 and t =1.879, P=0.097, respectively). Scores of BSIS-2-weeks were decreased significantly after treatment (t =2.477, P=0.038), while there were no significant changes in scores of BSIS-worst (t =−1.049, P=0.325).
Table 1
Demographics | Descriptive statistics |
---|---|
N | 9 |
Age (mean ± SD) | 33.78±11.01 |
Gender (male/female) | 3/6 |
Handedness (right/left) | 9/0 |
Months of illness duration (mean ± SD) | 64.67±46.38 |
Years of education (mean ± SD) | 11.83±2.83 |
Age of first onset (mean ± SD) | 29.50±11.20 |
Medications | |
Antidepressant | 9/0 |
Antipsychotic | 3/6 |
Benzodiazepine | 4/5 |
Other | 1/8 |
SD, standard deviation.
Table 2
Measures | Before ECT (mean ± SD) | After ECT (mean ± SD) | Comparisons |
---|---|---|---|
HAMD scores | 21.33±4.53 | 7.67±6.18 | t =7.517, P<0.001 |
WAIS-DS scores | 69.56±18.94 | 63.67±15.56 | t =1.879, P=0.097 |
WAIS-I scores | 16.28±6.51 | 16.56±7.28 | t =−0.153, P=0.881 |
BSIS-2 weeks scores | 16.56±8.43 | 10.67±4.18 | t =2.477, P=0.038 |
BSIS-worst scores | 18.67±9.73 | 22.11±8.07 | t =−1.049, P=0.325 |
ECT, electroconvulsive therapy; SD, standard deviation; HAMD, Hamilton Depression Scale; WAIS-DS, Digit Symbol subtest of Wechsler Adult Intelligence Scale; WAIS-I, Information subtest of Wechsler Adult Intelligence Scale; BSIS, Beck Suicidal Ideation Scale.
Changes in static network metrics
At global level, the Eloc and Ep were significantly increased (t =−2.861, P=0.021 and t =−2.450, P=0.039, respectively; shown in Figure 1) after the ECT, while the Eglob and Lp were not significantly changed (t =2.211, P=0.058 and t =−2.190, P=0.060, respectively; shown in Figure 1). At a local level, nodal degrees of several ROIs located in the cerebellum were found to be significantly changed after ECT; significantly decreased nodal degrees were also found in two separate regions of the temporal gyrus and olfactory cortex (see more details in Table 3 and Figure 2).
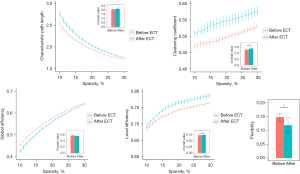
Table 3
Center of mass (MNI coordinate, x; y; z) | ROI label | Before ECT | After ECT | t | P |
---|---|---|---|---|---|
Nodal degree | |||||
62.9; −43.0; −7.8 | Right middle, inferior temporal gyrus | 0.00059 | −0.15587 | 2.349 | 0.047 |
−7.3; −45.4; −39.5 | Left cerebellum | −0.10308 | 0.00731 | −3.747 | 0.006 |
−28.4; −40.0; −30.7 | Left cerebellum | 0.00313 | 0.02049 | −2.521 | 0.036 |
35.7; −73.0; −30.6 | Right cerebellum peduncle | −0.00548 | −0.03143 | 4.748 | 0.001 |
0.1; 20.4; −8.0 | Bilateral olfactory cortex | 0.01789 | −0.00023 | 2.854 | 0.021 |
28.1; −49.1; −13.0 | Right fusiform gyrus | −0.01549 | −0.02977 | 2.459 | 0.039 |
Nodal flexibility | |||||
27.7; 58.2; −1.6 | Right middle frontal gyrus | 0.17040 | 0.10212 | 3.444 | 0.009 |
43.2; −73.6; −10.9 | Right inferior occipital gyrus | 0.14333 | 0.07050 | 3.326 | 0.012 |
−45.6; 8.0; 31.7 | Left precentral gyrus | 0.15020 | 0.09393 | 3.029 | 0.016 |
30.1; −39.3; −31.6 | Right cerebellum | 0.16646 | 0.09293 | 3.321 | 0.011 |
−39.1; 20.3; 42.2 | Left middle frontal gyrus | 0.11838 | 0.04889 | 3.666 | 0.006 |
−40.6; 12.9; −28.2 | Left superior and middle temporal gyrus | 0.13253 | 0.16030 | −3.315 | 0.011 |
37.8; −12.8; −26.0 | Right fusiform gyrus | 0.16222 | 0.09980 | 2.677 | 0.028 |
−28.4; −40.0; −30.7 | Left cerebellum | 0.19717 | 0.13162 | 2.350 | 0.047 |
28.6; 34.6; 42.0 | Right superior, middle frontal gyrus | 0.13374 | 0.08061 | 2.451 | 0.040 |
−27.6; −38.0; −11.4 | Left fusiform gyrus | 0.16838 | 0.11929 | 3.392 | 0.009 |
31.0; 18.0; 52.7 | Right superior, middle frontal gyrus | 0.13455 | 0.07242 | 2.486 | 0.038 |
−45.0; −0.7; −14.6 | Left middle, superior temporal gyrus | 0.16495 | 0.09545 | 3.923 | 0.004 |
−25.5; −92.9; 12.0 | Left middle occipital gyrus | 0.16253 | 0.09071 | 2.547 | 0.034 |
−31.9; −21.1; −18.8 | Left hippocampus | 0.16283 | 0.09051 | 4.007 | 0.004 |
51.9; 21.0; 21.1 | Right triangle inferior frontal gyrus | 0.15838 | 0.08778 | 2.641 | 0.030 |
13.5; 21.7; 59.8 | Right superior frontal gyrus | 0.16455 | 0.08859 | 2.549 | 0.034 |
−27.6; −58.3; −13.6 | Left fusiform gyrus | 0.15455 | 0.09010 | 2.339 | 0.047 |
−21.3; 30.0; 47.3 | Left superior, middle frontal gyrus | 0.09980 | 0.04515 | 2.727 | 0.026 |
−41.9; −31.5; 15.2 | Left superior temporal gyrus | 0.15434 | 0.08535 | 2.326 | 0.048 |
ROIs, regions of interest; ECT, electroconvulsive therapy; MNI, Montreal Neurological Institute.
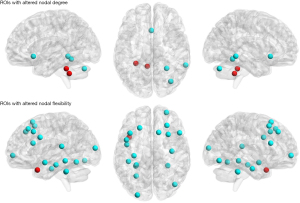
Changes in dynamic network metrics
As shown in Figure 1, brain network flexibility at the global level was significantly decreased after ECT (t =2.334, P=0.048). At the local level, the flexibility of 19 ROIs was found to be significantly changed (mostly decreased) after ECT; these ROIs mainly involved the cerebellum and hippocampus areas, as well as frontal and temporal cortices (Table 3 and Figure 2).
Correlations with clinical improvements
No significant correlations were found between the changes in brain network metrics and changes in clinical characteristics (all P>0.05); nevertheless, the decreases in HAMD scores were associated with trends of changes in all network metrics after the ECT (Figure 3).
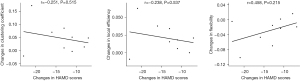
Supplementary analysis
In order to exclude the influence of the selection of threshold (sparsity) range on the results, we further compared the difference in all static network metrics before and after ECT in the threshold range from 10% to 40% and from 10% to 50%, respectively. The results showed that within each threshold range, both Eloc and Ep remained significantly increased (10% to 40%: t =−2.742, P=0.025 and t =−2.453, P=0.040 for Eloc and Ep, respectively; 10% to 50%: t =−2.659, P=0.029 and t =−2.431, P=0.041 for Eloc and Ep, respectively) after ECT, while Eglob and Lp remained not significantly different.
Discussion
To the best of our knowledge, the current research is the first one to explore the mechanism of ECT in MDD by combining both static and dynamic brain network analyses. Overall, our research illustrated that ECT significantly alters both the static and dynamic properties of brain networks in MDD patients.
In respect of static brain network properties, our results suggested that at the global level, both the Eloc and Ep were significantly increased after ECT (Figure 1), which is highly consistent with the results in several previous studies (52-54). For example, a recent study including 17 patients with depression has reported that the Ep of the brain network was significantly increased after ECT (53). Compared with healthy subjects, MDD patients are generally thought to have a shift toward randomization in their brain networks, reflecting a less optimal topological organization which may be due to overactivated connections in the network (55). The increases in Eloc and Ep reflect that the brain network is more regularized with enhanced information transfer efficiency between nodes and less energy costs (56). Thus, it could be hypothesized that ECT may have effects to decrease the randomness of brain network as reflected by elevated levels of Eloc and Ep, which has been reported in a series of previous studies (52-54) and confirmed in the present study again.
Beyond static network properties, our study revealed that an important dynamic brain network metric named flexibility was significantly decreased, which suggests a significantly decreased temporal variability (increased temporal stability) of brain network after the ECT in MDD patients (Figure 1). Previous hypotheses (21) suggest that an increase in brain network flexibility may imply excessive brain activity, which may lead to a decrease in network remodeling and a further decrease in the ability to adapt to the environment (57). Such an increase in brain network flexibility has been reported in multiple psychiatric diseases such as schizophrenia (58) and autism (59). Although not fully consistent, a number of previous dynamic fMRI studies have shown that the temporal variability of FC in the brain network is significantly increased in MDD patients compared to normal subjects, which is correlated with their clinical symptoms (26,60,61). Similar to what was found in static brain networks, such an alteration may also indicate a more randomized brain network organization attributed to increased aberrant brain connections (26). Therefore, ECT may make the brain network more regularized and stable over time, which may explain why ECT can alleviate the depressive symptoms in MDD patients in the context of a dynamic brain network for the first time.
At the local level, several ROIs with significant changes after ECT were found in both the static and dynamic networks, which mainly involve the cerebellum, hippocampus, frontal gyrus, and temporal gyrus (Table 3 and Figure 2). These regions may thus play key roles in the effects of ECT on depressive symptoms in MDD. Previous studies have found that frontal and temporal cortices play important roles in the development of MDD (62); the disrupted functions of the cerebellum and hippocampus were also repeatedly reported in patients with MDD (55,63,64), which are consistent with our results. It is also noteworthy that in this study, more ROIs with significant local-level alterations were revealed by the dynamic brain network analysis method compared to static network metrics (Table 3 and Figure 2); such results may partly support the opinion that the dynamic network model may provide a more sensitive tool for detecting the brain dysfunctions than conventional static network model (23,24).
In this study, we failed to find any significant correlation between the relief in clinical symptoms and changes in brain network metrics. Nevertheless, the decreases in HAMD scores were associated with trends of changes in all network metrics after the ECT (Figure 3). Future explorations with a larger sample and a higher statistical power may be needed to confirm such associations.
The current research mainly has the following limitations for future research reference. Firstly, as mentioned above, the sample size of this study is small. Further studies are needed to verify our results in a larger sample with higher statistical power and reliability. Secondly, we only analyzed changes in brain network connectivity before and after ECT and were unable to further analyze long-term dynamic changes after the acute treatment period (65). In addition, because few participants were discharged from the hospital after completing the ECT course, the interval of the second scan was delayed for a part of the time, which may lead to unstable results. Future researches with a longer follow-up period, if undertaken, will provide more insight into the prognostic benefits of ECT for MDD. Thirdly, problems regarding the lack of control group (46,65), possible impacts of the heterogeneity of different subtypes of depression (such as patients with/without somatic or psychotic symptoms) (66), different ECT regimens (67) and varied time intervals between the two assessments have not been effectively addressed in this research, which may be investigated in future research. Lastly, while we focused on the flexibility in this study, there are some other dynamic brain network measures (68,69) which can be further investigated.
Conclusions
Briefly, this study explores the neurobiological mechanism by which ECT may play its role in relieving depressive symptoms in MDD by investigating the changes in both static and dynamic brain network properties after ECT. We found that the clustering coefficient and local efficiency in static brain networks were increased, while the flexibility of the dynamic brain network was decreased after ECT. Several ROIs that changed significantly at the local level were also identified, which involved regions of the cerebellum, hippocampus as well as frontal and temporal cortices. Moreover, although not significant, the decrease of HAMD scores were associated with trends of changes in all network metrics after ECT. Our results suggest that ECT may alleviate the depressive symptoms of MDD by decreasing the randomness of brain network as reflected by changes in both static and dynamic network metrics, which might extend our knowledge of mechanisms underlying ECT for the clinical treatments of MDD. However, this study is limited by a relatively small sample size and the results should be confirmed in larger samples.
Acknowledgments
Funding: This work was supported by the National Natural Science Foundation of China (Nos. 81801353, 81701325, and 82071506) and the Natural Science Foundation of Hunan Province, China (No. 2021JJ4085).
Footnote
Reporting Checklist: The authors have completed the STROBE reporting checklist. Available at https://apm.amegroups.com/article/view/10.21037/apm-21-2723/rc
Data Sharing Statement: Available at https://apm.amegroups.com/article/view/10.21037/apm-21-2723/dss
Conflicts of Interest: All authors have completed the ICMJE uniform disclosure form (available at https://apm.amegroups.com/article/view/10.21037/apm-21-2723/coif). The authors have no conflicts of interest to declare.
Ethical Statement: The authors are accountable for all aspects of the work in ensuring that questions related to the accuracy or integrity of any part of the work are appropriately investigated and resolved. The study was approved by the Ethics Committee of Second Xiangya Hospital, Central South University (No. 2016S024) and written informed consent was obtained from all participants. The study was conducted in accordance with the Declaration of Helsinki (as revised in 2013).
Open Access Statement: This is an Open Access article distributed in accordance with the Creative Commons Attribution-NonCommercial-NoDerivs 4.0 International License (CC BY-NC-ND 4.0), which permits the non-commercial replication and distribution of the article with the strict proviso that no changes or edits are made and the original work is properly cited (including links to both the formal publication through the relevant DOI and the license). See: https://creativecommons.org/licenses/by-nc-nd/4.0/.
References
- GBD 2017 Disease and Injury Incidence and Prevalence Collaborators. Global, regional, and national incidence, prevalence, and years lived with disability for 354 diseases and injuries for 195 countries and territories, 1990-2017: a systematic analysis for the Global Burden of Disease Study 2017. Lancet 2018;392:1789-858. [Crossref] [PubMed]
- Hawton K, Casañas I, Comabella C, Haw C, et al. Risk factors for suicide in individuals with depression: a systematic review. J Affect Disord 2013;147:17-28. [Crossref] [PubMed]
- Tan L, Xia T, Reece C. Social and individual risk factors for suicide ideation among Chinese children and adolescents: A multilevel analysis. Int J Psychol 2018;53:117-25. [Crossref] [PubMed]
- Bae SM. Individual and social stress, social capital, and depressive symptoms as predictors of adolescent suicide in South Korea: A mediated moderation model. J Health Psychol 2019;24:1909-19. [Crossref] [PubMed]
- Lumontod RZ III. Seeing the invisible: Extracting signs of depression and suicidal ideation from college students’ writing using LIWC a computerized text analysis. International Journal of Research Studies in Education 2020;9:31-44. [Crossref]
- Ren X, Yu S, Dong W, et al. Burden of depression in China, 1990-2017: Findings from the global burden of disease study 2017. J Affect Disord 2020;268:95-101. [Crossref] [PubMed]
- Jakobsen JC, Katakam KK, Schou A, et al. Selective serotonin reuptake inhibitors versus placebo in patients with major depressive disorder. A systematic review with meta-analysis and Trial Sequential Analysis. BMC Psychiatry 2017;17:58. [Crossref] [PubMed]
- Cipriani A, Furukawa TA, Salanti G, et al. Comparative Efficacy and Acceptability of 21 Antidepressant Drugs for the Acute Treatment of Adults With Major Depressive Disorder: A Systematic Review and Network Meta-Analysis. Focus (Am Psychiatr Publ) 2018;16:420-9. [Crossref] [PubMed]
- Read J, Bentall R. The effectiveness of electroconvulsive therapy: a literature review. Epidemiol Psichiatr Soc 2010;19:333-47. [Crossref] [PubMed]
- Brown S, Rittenbach K, Cheung S, et al. Current and Common Definitions of Treatment-Resistant Depression: Findings from a Systematic Review and Qualitative Interviews. Can J Psychiatry 2019;64:380-7. [Crossref] [PubMed]
- Khalid N, Atkins M, Tredget J, et al. The effectiveness of electroconvulsive therapy in treatment-resistant depression: a naturalistic study. J ECT 2008;24:141-5. [Crossref] [PubMed]
- Ma Y, Rosenheck R, Fan N, et al. Rates and Patient Characteristics of Electroconvulsive Therapy in China and Comparisons With the United States. J ECT 2019;35:251-7. [Crossref] [PubMed]
- Socci C, Medda P, Toni C, et al. Electroconvulsive therapy and age: Age-related clinical features and effectiveness in treatment resistant major depressive episode. J Affect Disord 2018;227:627-32. [Crossref] [PubMed]
- Smitha KA, Akhil Raja K, Arun KM, et al. Resting state fMRI: A review on methods in resting state connectivity analysis and resting state networks. Neuroradiol J 2017;30:305-17. [Crossref] [PubMed]
- Lin Z, Long Y, Wu Z, et al. Associations between brain abnormalities and common genetic variants for schizophrenia: a narrative review of structural and functional neuroimaging findings. Ann Palliat Med 2021;10:10031-52. [Crossref] [PubMed]
- Wang J, Ji Y, Li X, et al. Improved and residual functional abnormalities in major depressive disorder after electroconvulsive therapy. Prog Neuropsychopharmacol Biol Psychiatry 2020;100:109888. [Crossref] [PubMed]
- Perrin JS, Merz S, Bennett DM, et al. Electroconvulsive therapy reduces frontal cortical connectivity in severe depressive disorder. Proc Natl Acad Sci U S A 2012;109:5464-8. [Crossref] [PubMed]
- van Waarde JA, Scholte HS, van Oudheusden LJ, et al. A functional MRI marker may predict the outcome of electroconvulsive therapy in severe and treatment-resistant depression. Mol Psychiatry 2015;20:609-14. [Crossref] [PubMed]
- Bai T, Wei Q, Zu M, et al. Functional plasticity of the dorsomedial prefrontal cortex in depression reorganized by electroconvulsive therapy: Validation in two independent samples. Hum Brain Mapp 2019;40:465-73. [Crossref] [PubMed]
- Hutchison RM, Womelsdorf T, Allen EA, et al. Dynamic functional connectivity: promise, issues, and interpretations. Neuroimage 2013;80:360-78. [Crossref] [PubMed]
- Braun U, Schäfer A, Bassett DS, et al. Dynamic brain network reconfiguration as a potential schizophrenia genetic risk mechanism modulated by NMDA receptor function. Proc Natl Acad Sci U S A 2016;113:12568-73. [Crossref] [PubMed]
- Long Y, Liu Z, Chan CKY, et al. Altered Temporal Variability of Local and Large-Scale Resting-State Brain Functional Connectivity Patterns in Schizophrenia and Bipolar Disorder. Front Psychiatry 2020;11:422. [Crossref] [PubMed]
- Rashid B, Arbabshirani MR, Damaraju E, et al. Classification of schizophrenia and bipolar patients using static and dynamic resting-state fMRI brain connectivity. Neuroimage 2016;134:645-57. [Crossref] [PubMed]
- Pang Y, Zhang H, Cui Q, et al. Combined static and dynamic functional connectivity signatures differentiating bipolar depression from major depressive disorder. Aust N Z J Psychiatry 2020;54:832-42. [Crossref] [PubMed]
- Wang S, Wen H, Hu X, et al. Transition and Dynamic Reconfiguration of Whole-Brain Network in Major Depressive Disorder. Mol Neurobiol 2020;57:4031-44. [Crossref] [PubMed]
- Long Y, Cao H, Yan C, et al. Altered resting-state dynamic functional brain networks in major depressive disorder: Findings from the REST-meta-MDD consortium. Neuroimage Clin 2020;26:102163. [Crossref] [PubMed]
- Qiao D, Zhang A, Sun N, et al. Altered Static and Dynamic Functional Connectivity of Habenula Associated With Suicidal Ideation in First-Episode, Drug-Naïve Patients With Major Depressive Disorder. Front Psychiatry 2020;11:608197. [Crossref] [PubMed]
- Han S, Cui Q, Wang X, et al. Resting state functional network switching rate is differently altered in bipolar disorder and major depressive disorder. Hum Brain Mapp 2020;41:3295-304. [Crossref] [PubMed]
- Zimmerman M, Martinez JH, Young D, et al. Severity classification on the Hamilton Depression Rating Scale. J Affect Disord 2013;150:384-8. [Crossref] [PubMed]
- Ross EL, Zivin K, Maixner DF. Cost-effectiveness of Electroconvulsive Therapy vs Pharmacotherapy/Psychotherapy for Treatment-Resistant Depression in the United States. JAMA Psychiatry 2018;75:713-22. [Crossref] [PubMed]
- Zeng J, Luo Q, Du L, et al. Reorganization of Anatomical Connectome following Electroconvulsive Therapy in Major Depressive Disorder. Neural Plast 2015;2015:271674. [Crossref] [PubMed]
- Esfahani M, Hashemi Y, Alavi K. Psychometric assessment of beck scale for suicidal ideation (BSSI) in general population in Tehran. Med J Islam Repub Iran 2015;29:268. [PubMed]
- Long Y, Ouyang X, Liu Z, et al. Associations Among Suicidal Ideation, White Matter Integrity and Cognitive Deficit in First-Episode Schizophrenia. Front Psychiatry 2018;9:391. [Crossref] [PubMed]
- Chao-Gan Y, Yu-Feng Z. DPARSF: A MATLAB Toolbox for "Pipeline" Data Analysis of Resting-State fMRI. Front Syst Neurosci 2010;4:13. [Crossref] [PubMed]
- Yan CG, Wang XD, Zuo XN, et al. DPABI: Data Processing & Analysis for (Resting-State) Brain Imaging. Neuroinformatics 2016;14:339-51. [Crossref] [PubMed]
- Yan CG, Chen X, Li L, et al. Reduced default mode network functional connectivity in patients with recurrent major depressive disorder. Proc Natl Acad Sci U S A 2019;116:9078-83. [Crossref] [PubMed]
- Jenkinson M, Bannister P, Brady M, et al. Improved optimization for the robust and accurate linear registration and motion correction of brain images. Neuroimage 2002;17:825-41. [Crossref] [PubMed]
- Craddock RC, James GA, Holtzheimer PE 3rd, et al. A whole brain fMRI atlas generated via spatially constrained spectral clustering. Hum Brain Mapp 2012;33:1914-28. [Crossref] [PubMed]
- Rubinov M, Sporns O. Complex network measures of brain connectivity: uses and interpretations. Neuroimage 2010;52:1059-69. [Crossref] [PubMed]
- Tan W, Liu Z, Xi C, et al. Decreased integration of the frontoparietal network during a working memory task in major depressive disorder. Aust N Z J Psychiatry 2021;55:577-87. [Crossref] [PubMed]
- Cao H, Bertolino A, Walter H, et al. Altered Functional Subnetwork During Emotional Face Processing: A Potential Intermediate Phenotype for Schizophrenia. JAMA Psychiatry 2016;73:598-605. [Crossref] [PubMed]
- Meng Q, Han Y, Ji G, et al. Disrupted topological organization of the frontal-mesolimbic network in obese patients. Brain Imaging Behav 2018;12:1544-55. [Crossref] [PubMed]
- Duan S, Liu L, Li G, et al. Altered Functional Connectivity Within and Between Salience and Sensorimotor Networks in Patients With Functional Constipation. Front Neurosci 2021;15:628880. [Crossref] [PubMed]
- Bassett DS, Wymbs NF, Porter MA, et al. Dynamic reconfiguration of human brain networks during learning. Proc Natl Acad Sci U S A 2011;108:7641-6. [Crossref] [PubMed]
- Betzel RF, Satterthwaite TD, Gold JI, et al. Positive affect, surprise, and fatigue are correlates of network flexibility. Sci Rep 2017;7:520. [Crossref] [PubMed]
- Long Y, Chen C, Deng M, et al. Psychological resilience negatively correlates with resting-state brain network flexibility in young healthy adults: a dynamic functional magnetic resonance imaging study. Ann Transl Med 2019;7:809. [Crossref] [PubMed]
- Huang D, Liu Z, Cao H, et al. Childhood trauma is linked to decreased temporal stability of functional brain networks in young adults. J Affect Disord 2021;290:23-30. [Crossref] [PubMed]
- Huang X, Wu Z, Liu Z, et al. Acute Effect of Betel Quid Chewing on Brain Network Dynamics: A Resting-State Functional Magnetic Resonance Imaging Study. Front Psychiatry 2021;12:701420. [Crossref] [PubMed]
- Jeub LGS, Bazzi M, Jutla IS, et al. A generalized Louvain method for community detection implemented in MATLAB, 2019. Available online: https://github.com/GenLouvain/GenLouvain
- Zheng H, Li F, Bo Q, et al. The dynamic characteristics of the anterior cingulate cortex in resting-state fMRI of patients with depression. J Affect Disord 2018;227:391-7. [Crossref] [PubMed]
- Mucha PJ, Richardson T, Macon K, et al. Community structure in time-dependent, multiscale, and multiplex networks. Science 2010;328:876-8. [Crossref] [PubMed]
- Wang J, Wei Q, Wang L, et al. Functional reorganization of intra- and internetwork connectivity in major depressive disorder after electroconvulsive therapy. Hum Brain Mapp 2018;39:1403-11. [Crossref] [PubMed]
- Sinha P, Reddy RV, Srivastava P, et al. Network neurobiology of electroconvulsive therapy in patients with depression. Psychiatry Res Neuroimaging 2019;287:31-40. [Crossref] [PubMed]
- Hill AT, Hadas I, Zomorrodi R, et al. Modulation of functional network properties in major depressive disorder following electroconvulsive therapy (ECT): a resting-state EEG analysis. Sci Rep 2020;10:17057. [Crossref] [PubMed]
- Zhang J, Wang J, Wu Q, et al. Disrupted brain connectivity networks in drug-naive, first-episode major depressive disorder. Biol Psychiatry 2011;70:334-42. [Crossref] [PubMed]
- Suo XS, Lei DL, Li LL, et al. Psychoradiological patterns of small-world properties and a systematic review of connectome studies of patients with 6 major psychiatric disorders J Psychiatry Neurosci 2018;43:427. [Crossref] [PubMed]
- Kays JL, Hurley RA, Taber KH. The dynamic brain: neuroplasticity and mental health. J Neuropsychiatry Clin Neurosci 2012;24:118-24. [Crossref] [PubMed]
- Gifford G, Crossley N, Kempton MJ, et al. Resting state fMRI based multilayer network configuration in patients with schizophrenia. Neuroimage Clin 2020;25:102169. [Crossref] [PubMed]
- Harlalka V, Bapi RS, Vinod PK, et al. Atypical Flexibility in Dynamic Functional Connectivity Quantifies the Severity in Autism Spectrum Disorder. Front Hum Neurosci 2019;13:6. [Crossref] [PubMed]
- Demirtaş M, Tornador C, Falcón C, et al. Dynamic functional connectivity reveals altered variability in functional connectivity among patients with major depressive disorder. Hum Brain Mapp 2016;37:2918-30. [Crossref] [PubMed]
- Kaiser RH, Whitfield-Gabrieli S, Dillon DG, et al. Dynamic Resting-State Functional Connectivity in Major Depression. Neuropsychopharmacology 2016;41:1822-30. [Crossref] [PubMed]
- Jung J, Choi S, Han KM, et al. Alterations in functional brain networks in depressed patients with a suicide attempt history. Neuropsychopharmacology 2020;45:964-74. [Crossref] [PubMed]
- Liu L, Zeng LL, Li Y, et al. Altered cerebellar functional connectivity with intrinsic connectivity networks in adults with major depressive disorder. PLoS One 2012;7:e39516. [Crossref] [PubMed]
- Guo W, Liu F, Liu J, et al. Increased cerebellar-default-mode-network connectivity in drug-naive major depressive disorder at rest. Medicine (Baltimore) 2015;94:e560. [Crossref] [PubMed]
- Wei Q, Bai T, Chen Y, et al. The Changes of Functional Connectivity Strength in Electroconvulsive Therapy for Depression: A Longitudinal Study. Front Neurosci 2018;12:661. [Crossref] [PubMed]
- Wang YZ, Han Y, Zhao JJ, et al. Brain activity in patients with deficiency versus excess patterns of major depression: A task fMRI study. Complement Ther Med 2019;42:292-7. [Crossref] [PubMed]
- Lihua P, Su M, Ke W, et al. Different regimens of intravenous sedatives or hypnotics for electroconvulsive therapy (ECT) in adult patients with depression. Cochrane Database Syst Rev 2014;CD009763. [PubMed]
- Dini H, Sendi MSE, Sui J, et al. Dynamic Functional Connectivity Predicts Treatment Response to Electroconvulsive Therapy in Major Depressive Disorder. Front Hum Neurosci 2021;15:689488. [Crossref] [PubMed]
- Fu Z, Sui J, Espinoza R, et al. Whole-Brain Functional Connectivity Dynamics Associated With Electroconvulsive Therapy Treatment Response. Biol Psychiatry Cogn Neurosci Neuroimaging 2021; Epub ahead of print. [Crossref] [PubMed]